1 构建项目途径
- Look at ACL anthology for NLP papers: https://aclanthology.info
- Also look at the online proceedings of major ML conferences: NeurIPS, ICML, ICLR
- Look at online preprint servers, especially: aixiv.org
Arxiv Sanity Preserver by Stanford grad Andrej Karpathy of cs231n http://www.arxiv-sanity.com
Great new site – a much needed resource for this – lots of NLP tasks :https://paperswithcode.com/sota
2 数据集
Linguistic Data Consortium
https://catalog.ldc.upenn.edu/
https://linguistics.stanford.edu/resources/resources-corpora
Machine translation
http://statmt.org
Dependency parsing: Universal Dependencies
https://universaldependencies.org
Look at lists of datasets
• https://machinelearningmastery.com/datasets-natural-language-processing/
• https://github.com/niderhoff/nlp-datasets
3 GRU Review
vanilla RNN的BPTT算法有梯度消失的问题,难以学习到长距离依赖(评估过去对未来的影响),因此提出了LSTM GRU这种门控网络,以解决长距离依赖问题。想法很简单,给残差往后传播一个捷径(short-cut),这样可以直接计算长距离的残差,GRU这种网络本质上就是一种适应性捷径(adaptive shortcut),根据目前的状态,通过0~1的门,有比重的建立一些shortcut。
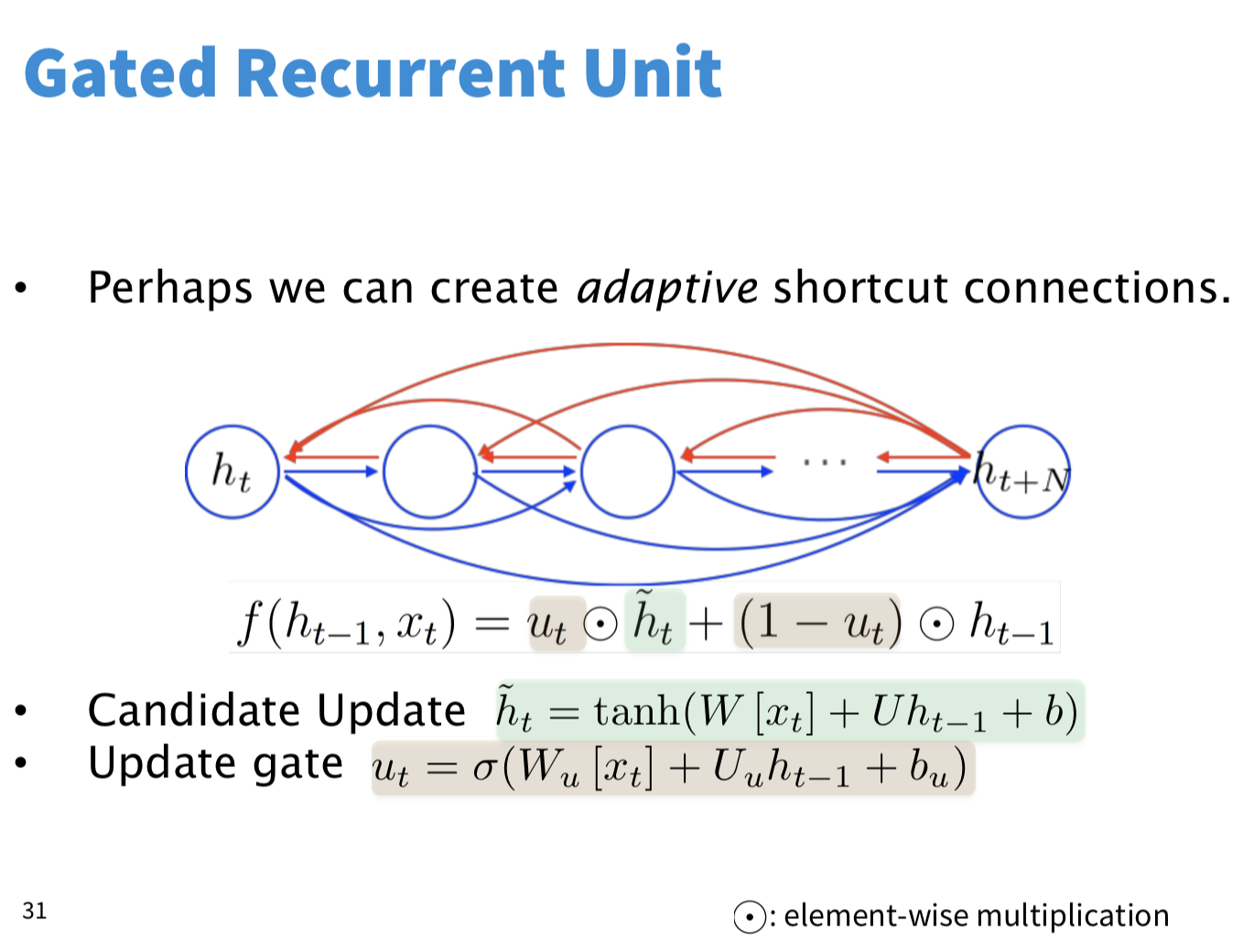
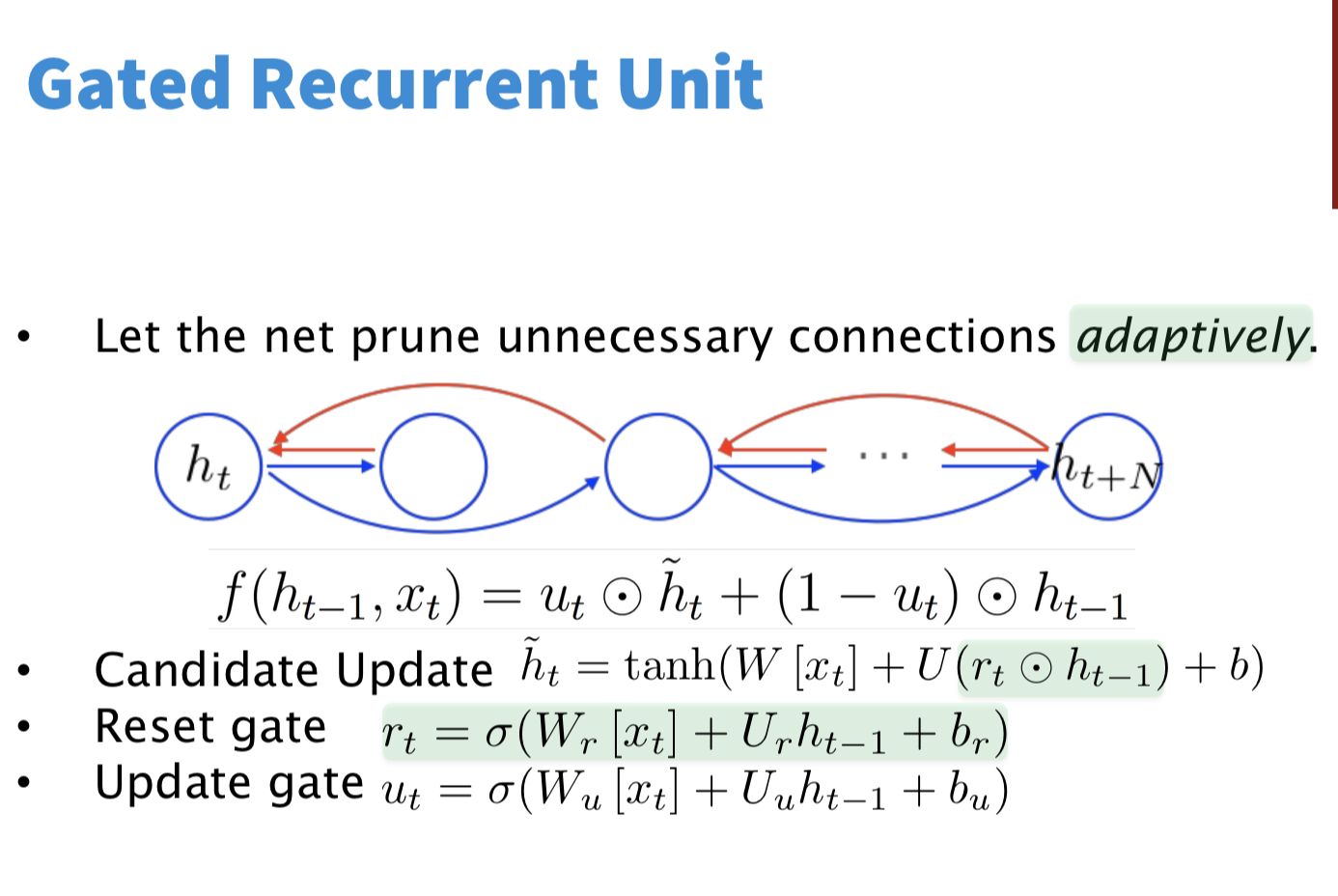
传统的RNN机制是读取所有信息,然后根据这些信息更新所有信息,如下图
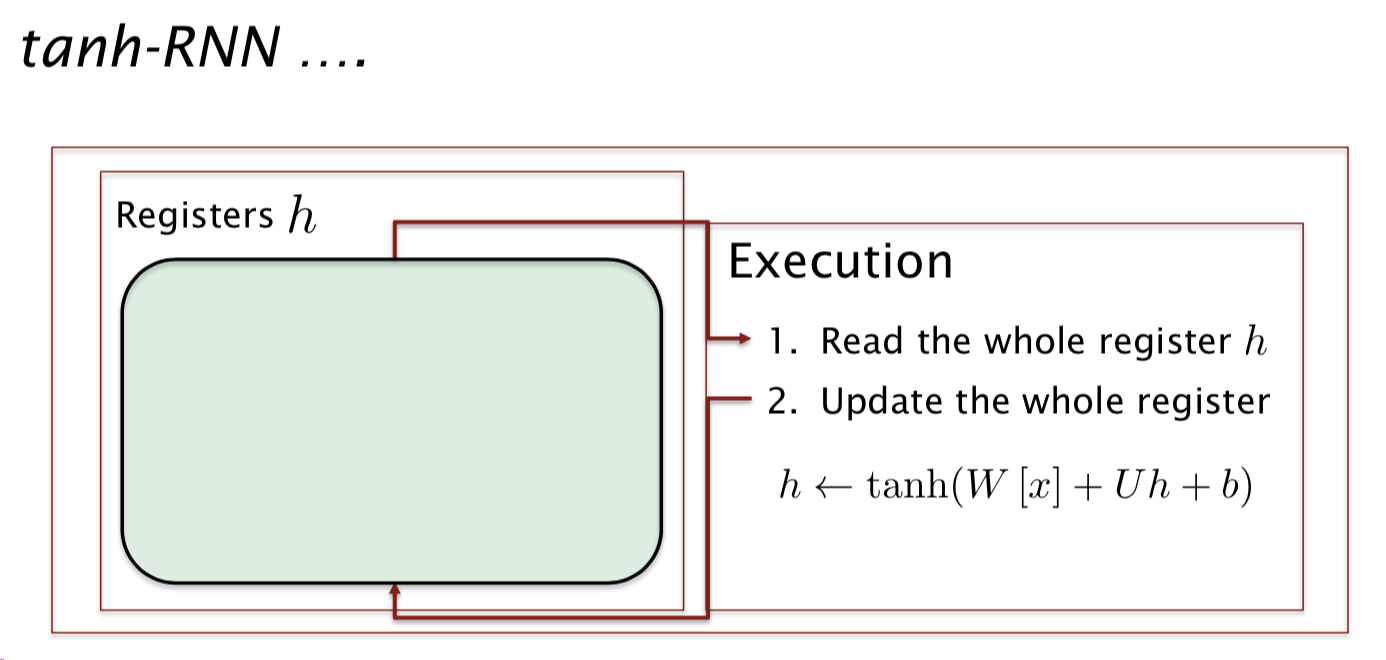
如下图所示,GRU的机制其实就是挑选(读取)一部分隐层信息,然后更新(写入)一部分隐层信息。读取写入的操作都是基于门实现。这个想法一定程度上和attention机制相似。
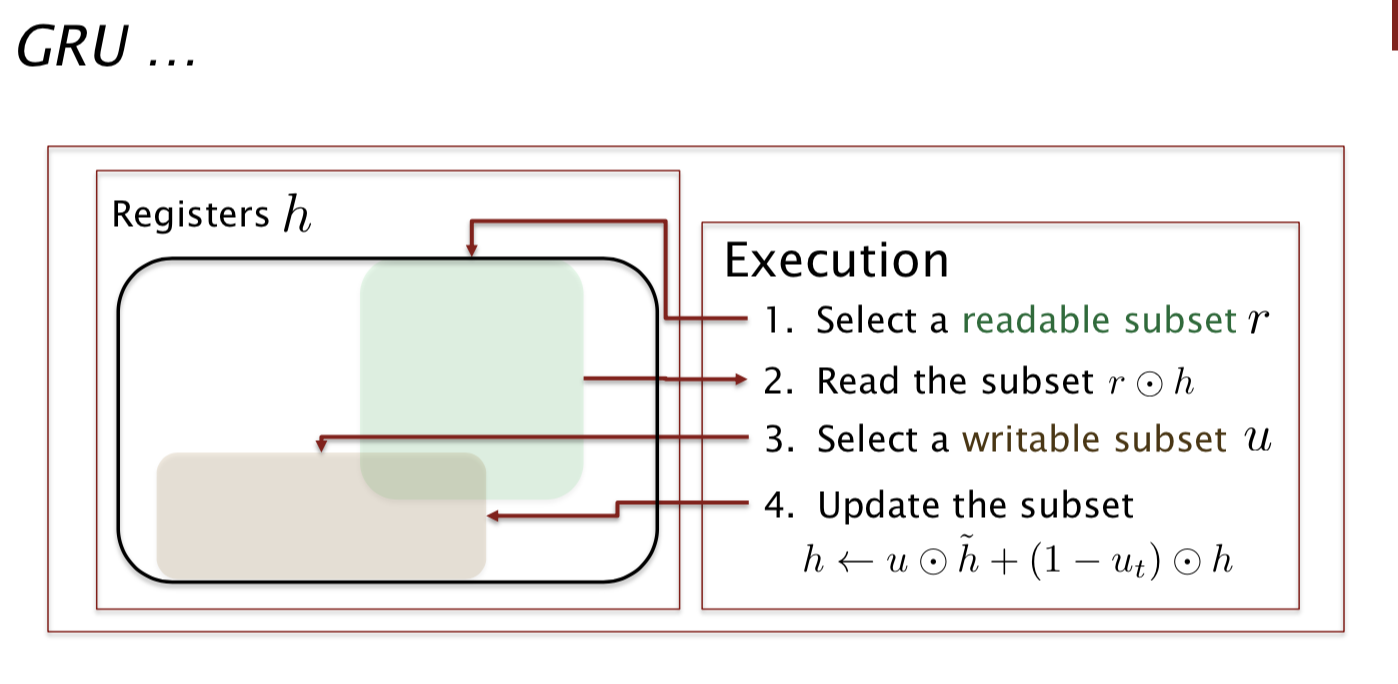
4 The large output vocabulary problem in NMT (or all NLG)
NMT和很多NLP任务面临的一个计算问题,就是softmax生成概率的计算量过大,因为要所有 词汇的概率,词汇的量又比较大,通常有五万多的词汇,这样softmax计算非常昂贵。解决这个问题的方法大概有以下几种:
-
Hierarchical softmax: tree-structuredvocabulary
-
Noise-contrastive estimation: binary classification
-
Train on a subset of the vocabulary at at ime; test on a smart on the set of possible translations
• Jean,Cho,Memisevic,Bengio.ACL2015
-
Use attention to work out what you are translating:
You can do something simple like dictionary lookup
-
*More ideas we will get to:*Word pieces;char.models
5 训练RNN的技巧
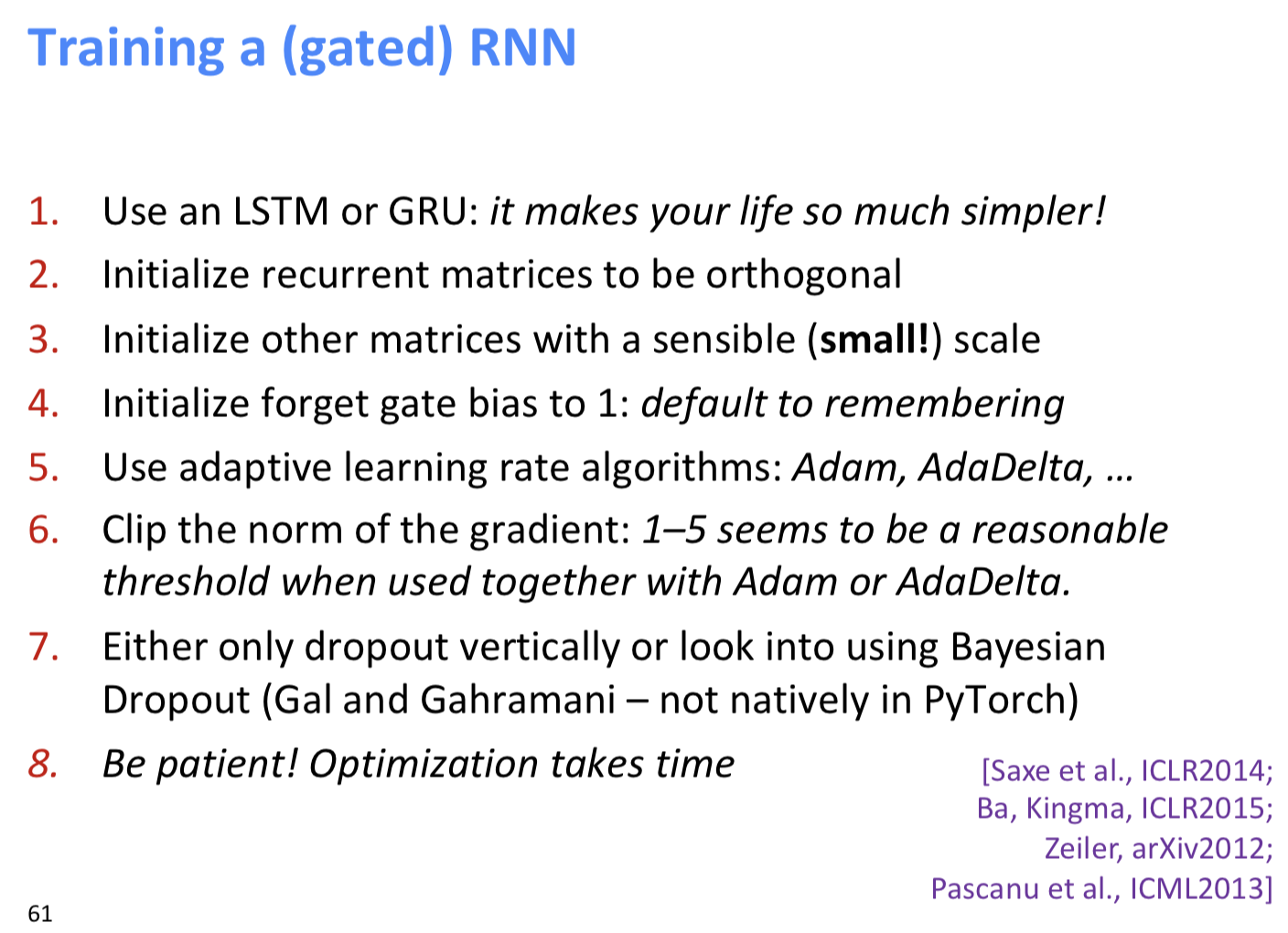