Python学习教程(Python学习路线):Pandas库基础分析-详解时间序列的处理
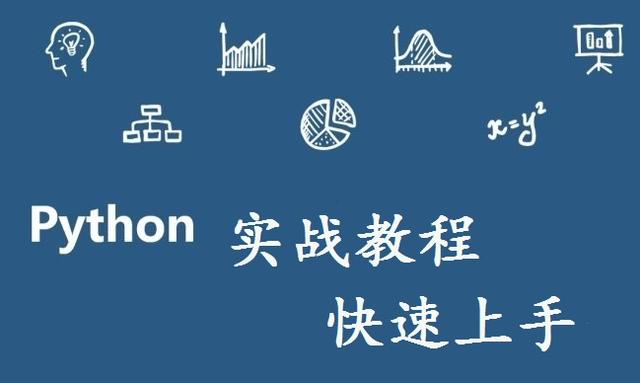
在使用Python进行数据分析时,经常会遇到时间日期格式处理和转换,特别是分析和挖掘与时间相关的数据,比如量化交易就是从历史数据中寻找股价的变化规律。Python中自带的处理时间的模块有datetime,NumPy库也提供了相应的方法,Pandas作为Python环境下的数据分析库,更是提供了强大的日期数据处理的功能,是处理时间序列的利器。
1、生成日期序列
主要提供pd.data_range()和pd.period_range()两个方法,给定参数有起始时间、结束时间、生成时期的数目及时间频率(freq='M’月,'D’天,‘W’,周,'Y’年)等。
两种主要区别在于pd.date_range()生成的是DatetimeIndex格式的日期序列;pd.period_range()生成的是PeriodIndex格式的日期序列。
以下通过生成月时间序列和周时间序列来对比下:
date_rng = pd.date_range('2019-01-01', freq='M', periods=12)print(f'month date_range():{date_rng}')"""date_range():DatetimeIndex(['2019-01-31', '2019-02-28', '2019-03-31', '2019-04-30', '2019-05-31', '2019-06-30', '2019-07-31', '2019-08-31', '2019-09-30', '2019-10-31', '2019-11-30', '2019-12-31'], dtype='datetime64[ns]', freq='M')"""period_rng = pd.period_range('2019/01/01', freq='M', periods=12)print(f'month period_range():{period_rng}')"""period_range():PeriodIndex(['2019-01', '2019-02', '2019-03', '2019-04', '2019-05', '2019-06', '2019-07', '2019-08', '2019-09', '2019-10', '2019-11', '2019-12'], dtype='period[M]', freq='M')"""date_rng = pd.date_range('2019-01-01', freq='W-SUN', periods=12)print(f'week date_range():{date_rng}')"""week date_range():DatetimeIndex(['2019-01-06', '2019-01-13', '2019-01-20', '2019-01-27', '2019-02-03', '2019-02-10', '2019-02-17', '2019-02-24', '2019-03-03', '2019-03-10', '2019-03-17', '2019-03-24'], dtype='datetime64[ns]', freq='W-SUN')"""period_rng=pd.period_range('2019-01-01',freq='W-SUN',periods=12)print(f'week period_range():{period_rng}')"""week period_range():PeriodIndex(['2018-12-31/2019-01-06', '2019-01-07/2019-01-13', '2019-01-14/2019-01-20', '2019-01-21/2019-01-27', '2019-01-28/2019-02-03', '2019-02-04/2019-02-10', '2019-02-11/2019-02-17', '2019-02-18/2019-02-24', '2019-02-25/2019-03-03', '2019-03-04/2019-03-10', '2019-03-11/2019-03-17', '2019-03-18/2019-03-24'], dtype='period[W-SUN]', freq='W-SUN')"""date_rng = pd.date_range('2019-01-01 00:00:00', freq='H', periods=12)print(f'hour date_range():{date_rng}')"""hour date_range():DatetimeIndex(['2019-01-01 00:00:00', '2019-01-01 01:00:00', '2019-01-01 02:00:00', '2019-01-01 03:00:00', '2019-01-01 04:00:00', '2019-01-01 05:00:00', '2019-01-01 06:00:00', '2019-01-01 07:00:00', '2019-01-01 08:00:00', '2019-01-01 09:00:00', '2019-01-01 10:00:00', '2019-01-01 11:00:00'], dtype='datetime64[ns]', freq='H')"""period_rng=pd.period_range('2019-01-01 00:00:00',freq='H',periods=12)print(f'hour period_range():{period_rng}')"""hour period_range():PeriodIndex(['2019-01-01 00:00', '2019-01-01 01:00', '2019-01-01 02:00', '2019-01-01 03:00', '2019-01-01 04:00', '2019-01-01 05:00', '2019-01-01 06:00', '2019-01-01 07:00', '2019-01-01 08:00', '2019-01-01 09:00', '2019-01-01 10:00', '2019-01-01 11:00'], dtype='period[H]', freq='H')"""
2、生成Timestamp对象及转换
创建一个Timestamp时间戳对象有pd.Timestamp()方法和pd.to_datetime()方法。如下所示:
ts=pd.Timestamp(2019,1,1)print(f'pd.Timestamp()-1:{ts}')#pd.Timestamp()-1:2019-01-01 00:00:00ts=pd.Timestamp(dt(2019,1,1,hour=0,minute=1,second=1))print(f'pd.Timestamp()-2:{ts}')#pd.Timestamp()-2:2019-01-01 00:01:01ts=pd.Timestamp("2019-1-1 0:1:1")print(f'pd.Timestamp()-3:{ts}')#pd.Timestamp()-3:2019-01-01 00:01:01print(f'pd.Timestamp()-type:{type(ts)}')#pd.Timestamp()-type:#dt=pd.to_datetime(2019,1,1) 不支持dt=pd.to_datetime(dt(2019,1,1,hour=0,minute=1,second=1))print(f'pd.to_datetime()-1:{dt}')#pd.to_datetime()-1:2019-01-01 00:01:01dt=pd.to_datetime("2019-1-1 0:1:1")print(f'pd.to_datetime()-2:{dt}')#pd.to_datetime()-2:2019-01-01 00:01:01print(f'pd.to_datetime()-type:{type(dt)}')#pd.to_datetime()-type:#pd.to_datetime生成自定义时间序列dtlist=pd.to_datetime(["2019-1-1 0:1:1