在解决了cpu条件下编译fastrcnn的错误后,
菜园子:youtu one stage yolo make -j32 报错zhuanlan.zhihu.com就可以安排上训练等事宜了。
第一件事就是将自己要用的数据集转换成模型训练需要用的voc数据集的格式。VOC2007文件夹下文件组织目录如图
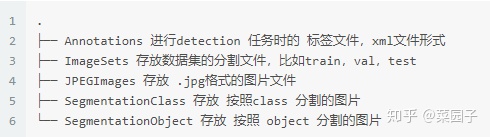
由于我要做检测任务,所以就不创建最后两个文件夹了。同时ImageSets文件夹下创建Main文件夹.
我在affect数据集目录下创建了notebook。同时将VOC2007文件夹也放在这里.
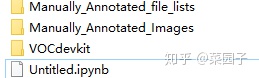
忽略这个VOCdevkit名字,其实是就是上面说的VOC2007,因为后面代码需要,所以改名了,但是代码里都是VOC2007.
然后开始上代码啦!
- 首先是读取csv文件,将train与test的csv 标签都放在一起
import pandas as pd
import os
train_labels = pd.read_csv('./Manually_Annotated_file_lists/training.csv')
train_len = len(train_labels['subDirectory_filePath'])
validation_labels = pd.read_csv('./Manually_Annotated_file_lists/validation.csv')
vali_len = len(validation_labels['subDirectory_filePath'])
print(train_len)
print(vali_len)
labels = pd.concat([train_labels,validation_labels])
print(len(labels))
2.然后就是将源文件的名字修改,同时复制到./VOC2007/JPEGImages/下,然后生成xml文件放到./VOC2007/Annotations/下
from lxml.etree import Element, SubElement, tostring
from xml.dom.minidom import parseString
import shutil
import matplotlib.image as mpimg
def getxml(label,image_name,shape):
node_root = Element('annotation')
node_folder = SubElement(node_root, 'folder')
node_folder.text = 'JPEGImages'
node_filename = SubElement(node_root, 'filename')
node_filename.text = image_name
node_size = SubElement(node_root, 'size')
node_width = SubElement(node_size, 'width')
node_width.text = '%s' % shape[0]
node_height = SubElement(node_size, 'height')
node_height.text = '%s' % shape[1]
node_depth = SubElement(node_size, 'depth')
node_depth.text = '%s' % shape[2]
left, top, right, bottom = label[1], label[2], label[1]+label[3], label[2] + label[4]
node_object = SubElement(node_root, 'object')
node_name = SubElement(node_object, 'name')
node_name.text = '%s' % label[6]
node_difficult = SubElement(node_object, 'difficult')
node_difficult.text = '0'
node_bndbox = SubElement(node_object, 'bndbox')
node_xmin = SubElement(node_bndbox, 'xmin')
node_xmin.text = '%s' % left
node_ymin = SubElement(node_bndbox, 'ymin')
node_ymin.text = '%s' % top
node_xmax = SubElement(node_bndbox, 'xmax')
node_xmax.text = '%s' % right
node_ymax = SubElement(node_bndbox, 'ymax')
node_ymax.text = '%s' % bottom
xml = tostring(node_root, pretty_print=True)
dom = parseString(xml)
save_xml = os.path.join('./VOC2007/Annotations/', image_name.replace('jpg', 'xml'))
with open(save_xml, 'wb') as f:
f.write(xml)
return
def getJPG_MV(labels):
mv_dir = './VOC2007/JPEGImages/'
# 将每个图片重命名移动到jpeg路径下
for i in range(235929,len(labels)):
ori_name = labels.iloc[i][0]
try:
I = mpimg.imread(os.path.join('./Manually_Annotated_Images/',ori_name))
except IOError:
continue
shape = I.shape
ori_dir = os.path.join('./Manually_Annotated_Images/',ori_name)
target_name = str(i).zfill(6)+'.jpg'
target_dir = os.path.join(mv_dir,target_name)
if os.path.exists(mv_dir):
shutil.copy(ori_dir,target_dir)
getxml(labels.iloc[i],target_name,shape)
getJPG_MV(labels)
3.划分train test val trainval
import random
def _main():
trainval_percent = 0.1
train_percent = 0.9
xmlfilepath = './VOC2007/Annotations'
total_xml = os.listdir(xmlfilepath)
num = len(total_xml)
list = range(num)
tv = int(num * trainval_percent)
tr = int(tv * train_percent)
trainval = random.sample(list, tv)
train = random.sample(trainval, tr)
ftrainval = open('./VOC2007/ImageSets/Main/trainval.txt', 'w')
ftest = open('./VOC2007/ImageSets/Main/test.txt', 'w')
ftrain = open('./VOC2007/ImageSets/Main/train.txt', 'w')
fval = open('./VOC2007/ImageSets/Main/val.txt', 'w')
for i in list:
name = total_xml[i][:-4] + 'n'
if i in trainval:
ftrainval.write(name)
if i in train:
ftest.write(name)
else:
fval.write(name)
else:
ftrain.write(name)
ftrainval.close()
ftrain.close()
fval.close()
ftest.close()
if __name__ == '__main__':
_main()
结束!