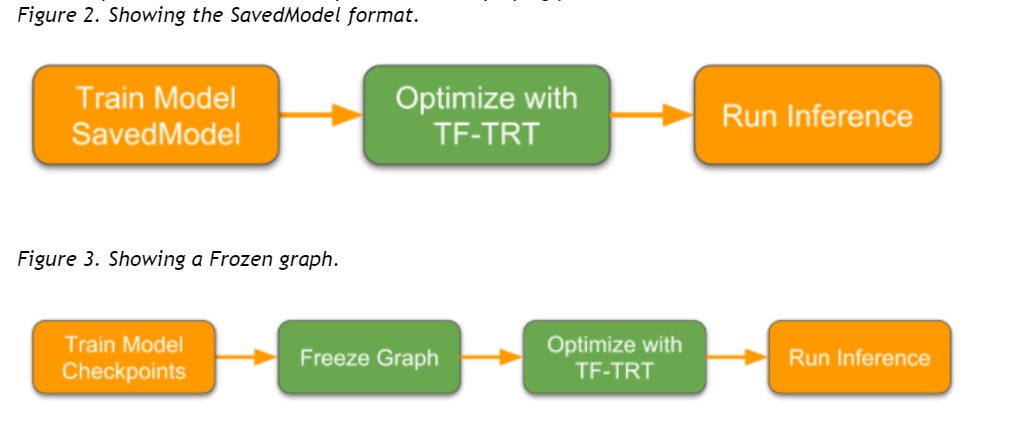
1 常用优化
官方提供的优化请参考官方文档:https://tensorflow.google.cn/guide/performance/overview?hl=zh-cn,列出的都是些最基本的常用优化方法,效果明显,更细的优化就不具体介绍了,像算子粒度的优化.
1.1 增加并发量提高算子计算图
提高算子和算子间的并发量对提高性能最明显,简单有效,是最常用的优化方法,尤其对CPU计算来说,下面给出三个修改并发量的参数,CPU计算时一定不要忘记这三个参数.
config.proto
// Map from device type name (e.g., "CPU" or "GPU" ) to maximum
// number of devices of that type to use. If a particular device
// type is not found in the map, the system picks an appropriate
// number.
map<string, int32> device_count = 1;
// The execution of an individual op (for some op types) can be
// parallelized on a pool of intra_op_parallelism_threads.
// 0 means the system picks an appropriate number.
int32 intra_op_parallelism_threads = 2;
// Nodes that perform blocking operations are enqueued on a pool of
// inter_op_parallelism_threads available in each process.
//
// 0 means the system picks an appropriate number.
//
// Note that the first Session created in the process sets the
// number of threads for all future sessions unless use_per_session_threads is
// true or session_inter_op_thread_pool is configured.
int32 inter_op_parallelism_threads = 5;
解释:
1)device_count, 告诉tf Session使用CPU数量上限,如果你的CPU数量较多,可以适当加大这个值;
2) inter_op_parallelism_threads和intra_op_parallelis