1.tf.multiply()两个矩阵中对应元素各自相乘
格式: tf.multiply(x, y, name=None)
参数:
x: 一个类型为:half, float32, float64, uint8, int8, uint16, int16, int32, int64, complex64, complex128的张量。
y: 一个类型跟张量x相同的张量。
返回值: x * y element-wise.
注意:
(1)multiply这个函数实现的是元素级别的相乘,也就是两个相乘的数元素各自相乘,而不是矩阵乘法,注意和tf.matmul区别。
(2)两个相乘的数必须有相同的数据类型,不然就会报错。
2.tf.matmul()将矩阵a乘以矩阵b,生成a * b。
格式: tf.matmul(a, b, transpose_a=False, transpose_b=False, adjoint_a=False, adjoint_b=False, a_is_sparse=False, b_is_sparse=False, name=None)
参数:
a: 一个类型为 float16, float32, float64, int32, complex64, complex128 且张量秩 > 1 的张量。
b: 一个类型跟张量a相同的张量。
transpose_a: 如果为真, a则在进行乘法计算前进行转置。
transpose_b: 如果为真, b则在进行乘法计算前进行转置。
adjoint_a: 如果为真, a则在进行乘法计算前进行共轭和转置。
adjoint_b: 如果为真, b则在进行乘法计算前进行共轭和转置。
a_is_sparse: 如果为真, a会被处理为稀疏矩阵。
b_is_sparse: 如果为真, b会被处理为稀疏矩阵。
name: 操作的名字(可选参数)
返回值: 一个跟张量a和张量b类型一样的张量且最内部矩阵是a和b中的相应矩阵的乘积。
注意:
(1)输入必须是矩阵(或者是张量秩 >2的张量,表示成批的矩阵),并且其在转置之后有相匹配的矩阵尺寸。
(2)两个矩阵必须都是同样的类型,支持的类型如下:float16, float32, float64, int32, complex64, complex128。
引发错误:
ValueError: 如果transpose_a 和 adjoint_a, 或 transpose_b 和 adjoint_b 都被设置为真
References:
tf.matmul() 和tf.multiply() 的区别www.cnblogs.com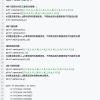