CS186 Berkeley
This is the course notes of CS186 online course
SQL
Create Table
CREATE TABLE Sailors(
sid INTEGER,
sname CHAR(20),
rating INTEGER,
age FLOAT,
PRIMARY KEY (sid)
);
Primary key cannot have any duplicate values, and it can be made up of >1 colume
E.g. (firstname, lastname) as private key.
CREATE TABLE Reserves(
sid INTEGER,
bid INTEGER,
day DATE,
PRIMARY KEY (sid, bid, day),
FOREIGN KEY (sid) REFERENCES Sailors
);
Foreign key is like a point, pointing from Reserves to Sailors
A table can have more than one foreign key.
Select Distinct
SELECT DISTINCT S.name S.gpa
FROM students S -- rename
WHERE S.dept = 'CS'
-
String in sql should use
single quotation marks
-
There is no
double quotation marks
in sql, in general.
Order by
SELECT S.name, S.gpa, S.age * 2 AS a2
FROM Student S
WHERE S.dept = 'CS'
ORDER BY S.gpa, S.name, a2 -- ASC for default
Here order by S.gpa, S.name, a2
means sql should order by S.gpa
. If there is a tie in S.gpa
, then order by S.name
.
SELECT S.name, S.gpa, S.age * 2 AS a2
FROM Student S
WHERE S.dept = 'CS'
ORDER BY S.gpa, S.name ASC, a2
- ASC: ascent
- DESC: descent
LIMIT
SELECT S.name, S.gpa, S.age * 2 AS a2
FROM Student S
WHERE S.dept = 'CS'
ORDER BY S.gpa, S.name ASC, a2
LIMIT 3 -- get the first three record
Limit
is typically used with order by
Aggregates
SELECT DISTINCT AVG(S.gpa)
FROM students S
WHERE S.dept = 'CS'
Other aggregates: SUM, COUNT, MAX, MIN
Group by
SELECT DISTINCT AVG(S.gpa) S.dept
FROM students S
Group by S.dept
SELECT S.dept, COUNT(*)FROM students SGroup by S.dept
Having
SELECT DISTINCT AVG(S.gpa) S.deptFROM students SGroup by S.deptHAVING COUNT(*) > 2
Having can only be used in aggregate queries
OR , UNION
SELECT R.sidFROM boats B, Reserves RWHERE R.bid = B.bid AND(B.color = 'red' OR B.color = 'green')
VS.
SELECT R.sidFROM boats B, Reserves RWHERE R.bid = B.bid AND B.color = 'red'UNION ALLSELECT R.sidFROM boats B, Reserves RWHERE R.bid = B.bid AND B.color = 'green'
AND, INTERSECT
SELECT R.sidFROM boats B, Reserves RWHERE R.bid = B.bid AND(B.color = 'red' AND B.color = 'green')
VS.
SELECT R.sidFROM boats B, Reserves RWHERE R.bid = B.bid AND B.color = 'red'INTERSECTSELECT R.sidFROM boats B, Reserves RWHERE R.bid = B.bid AND B.color = 'green'
Join Variants
Join types:[INNER | NATURAL | {LEFT | RIGHT | FULL } {OUTER}]
INNER is default
Inner and natural join
SELECT s.*, r.bidFROM Sailors s, Revserve rWHERE s.sid = r.sid
SELECT s.*, r.bidFROM Sailors s INNER JOIN Revserves rON s.sid = r.sid
SELECT s.*, r.bidFROM Sailors s NATURAL JOIN Revserves r
ALL three are equivalent
Left outer join
SELECT s.*, r.bidFROM Sailors s LEFT OUTER JOIN Revserves rON s.sid = r.sid
Preserve all unmatched rows from the table on the left
Right outer join
Preserve all unmatched rows from the table on the right
Full Outer join
Return all(matched or unmatched) rows from the tables on both sides.
Views
CREATE VIEW view_nameAS SELECT B.bid, COUNT(*) AS scount FROM Boats2 B, Reserves2 R WHERE R.bid = B.bid AND B.color = 'red' GROUP BY B.bid
NULL Values
NULL
Architecture of a DBMS
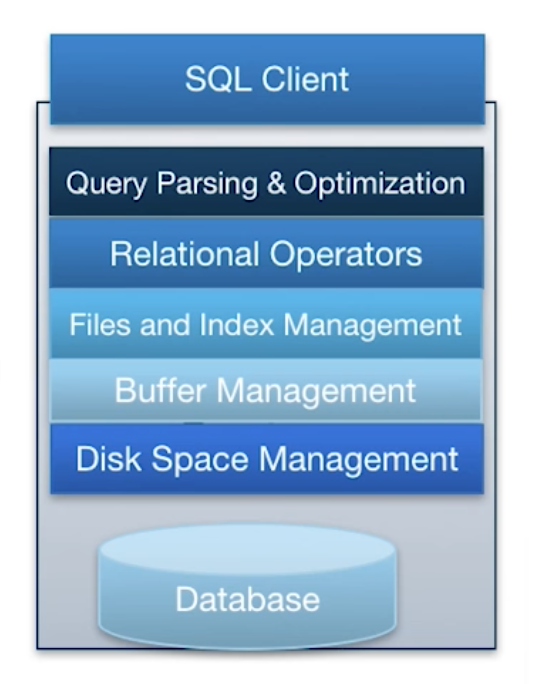
- Query Parsing & Optimization: Parse, check and verify the SQL
- Relational Operators: Execute a dataflow by operating on records and files
- Files and Index Management: Organize tables and Records as groups of pages in a lopgical file
- Buffer Management: Provide the illusion of operating in memory
- Disk Space Management: Translate page requests into physical bytes on one or more device(s)
Storage media
Disk
Most DB are originally designed for magnetic disks ( so old ) ==> slow
- Mag Disk: ~40TB for $1000
- SSD : ~2.3TB for $1000
- RAM: 80GB for $1000
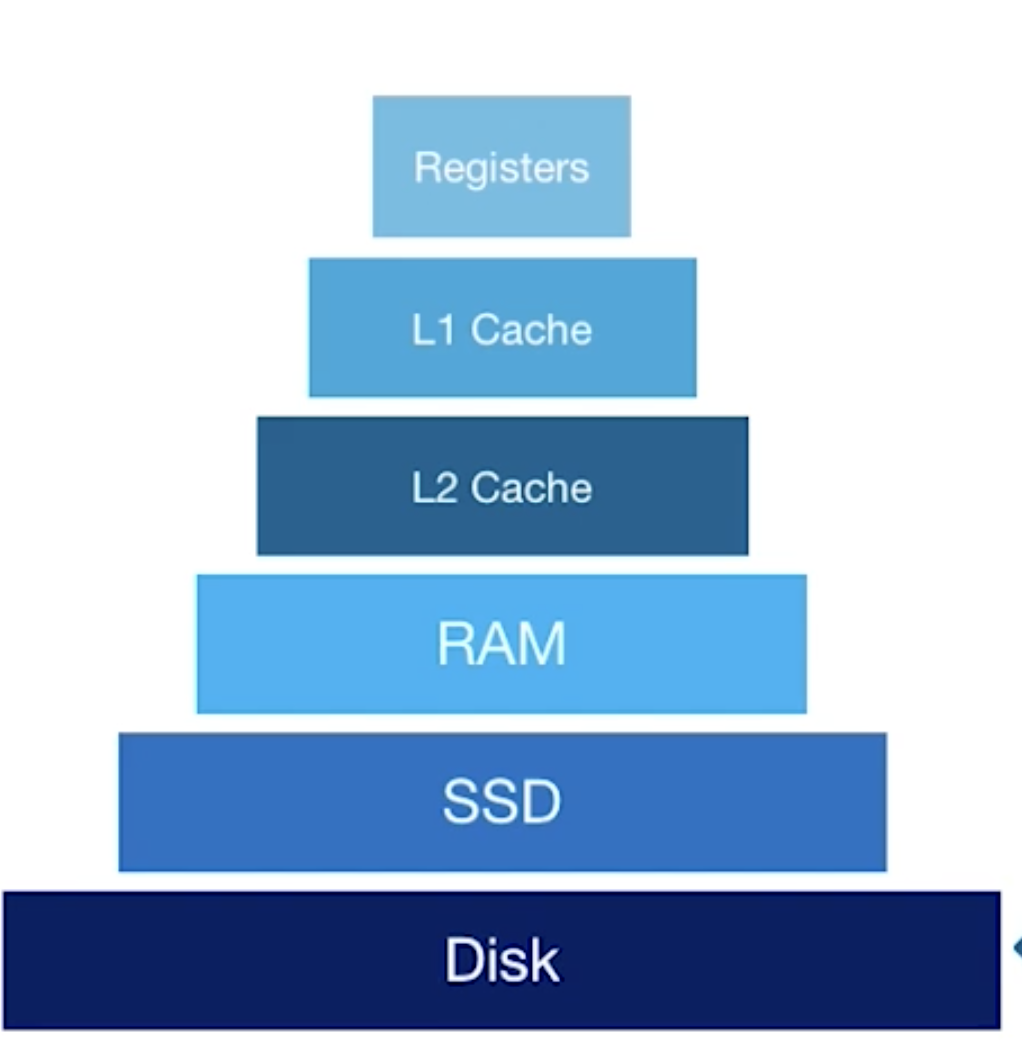
Lowest layer of DBMA, manages space on disk
- Block: Unit of transfer for disk read/write
- 64-128KB is a good number today
- Book says 4kb
- Page: a common synonym for “block”
- In some texts, “page” = a block-sized chuck of RAM
Overview: Representations
-
Tables stored as logical files consist of pages(each page contains a collection of records).
-
Pages are managed:
- on disk by the disk space manager: pages read/written to phtsical disk/files
- In memory by the buffer manager: higher levels of DBMS only operate in memory
-
DB file: like lofical files, is a collection of pages and each page contains a collection of record.
DB File structures
- Unordered Heap Files: recoreds placed arbitrarily across pages
- Clustered Heap Files: Records and pages are grouped
- Sorted Files: Pages and recoreds are in sorted order
- Index Files: B+ Tree, Linear Hashing…
Heap File Implemented as List
Use linked list
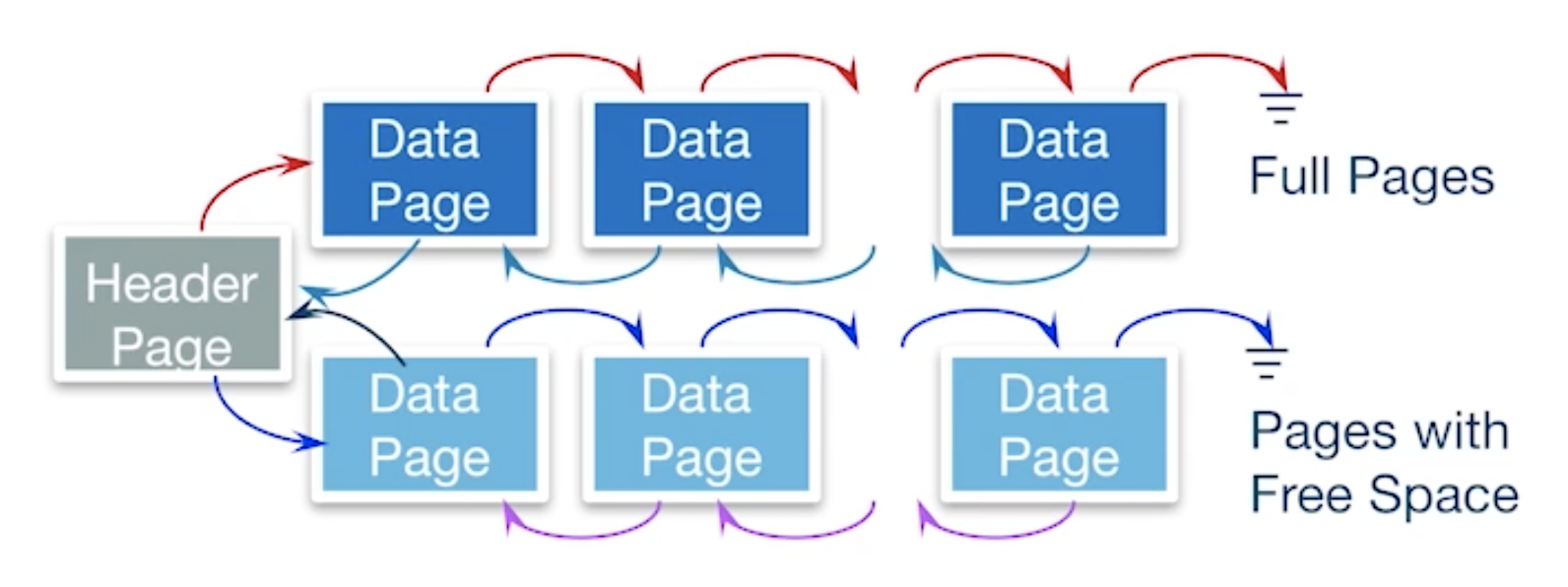
Each page has 2 pointers plus free space and data
But the problem is how do I find a page with enough space for a 20 byte records?
In this structure, we need walk through the linked list to find the proper page, which is inefficient.
Better: Use a page directory
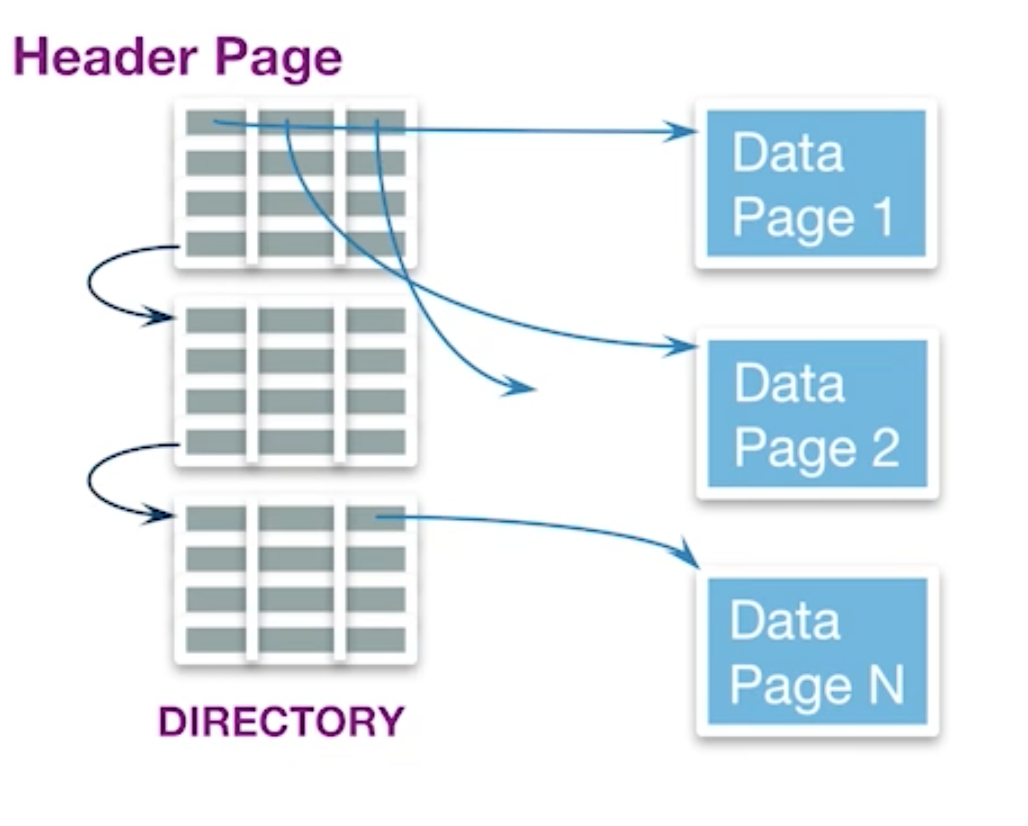
- Directory entries include: # free bytes on the referenced page
- Header pages accessed often ==> likely in cache
- better than linked list
Page layout
Header may contain:
- # records
- Free space
- maybe a next/last pointer
- bitmaps, slot table
Fixed length records, packed
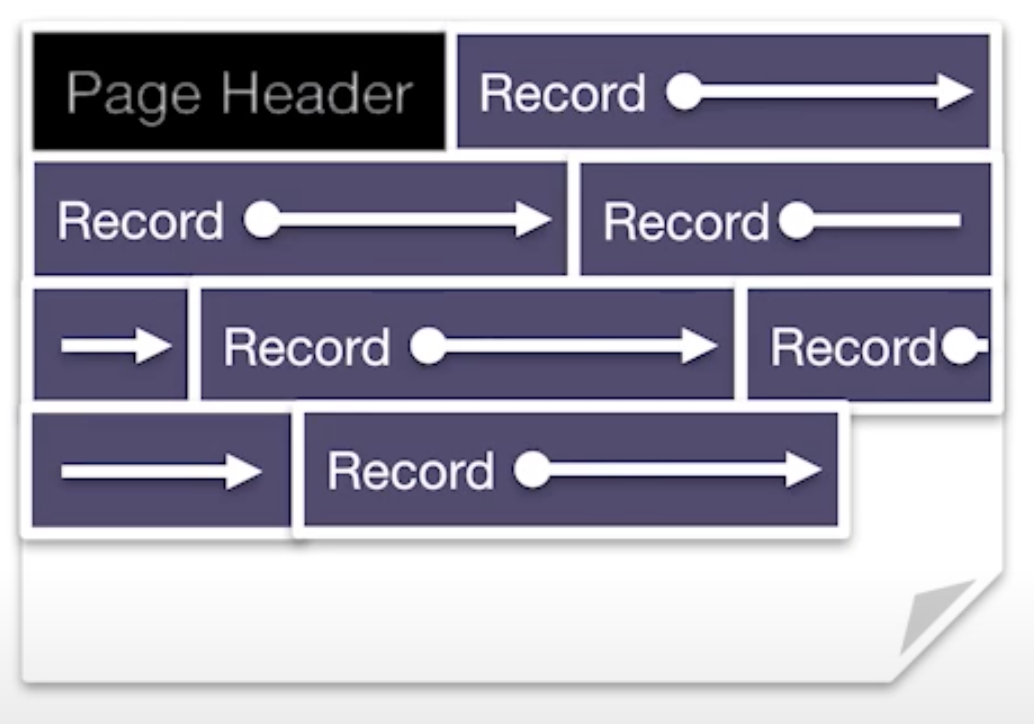
- Record id and offset
- Easy to add
- Problem: how to delete? ==> packed implies re-arrange, and record id pointers need to be updated
Fixed Length Records: Unpacked
- Bitmap denotes
slots
with records - Insert: find the first empty slot
- Delete: clear bit
Variable Length Records
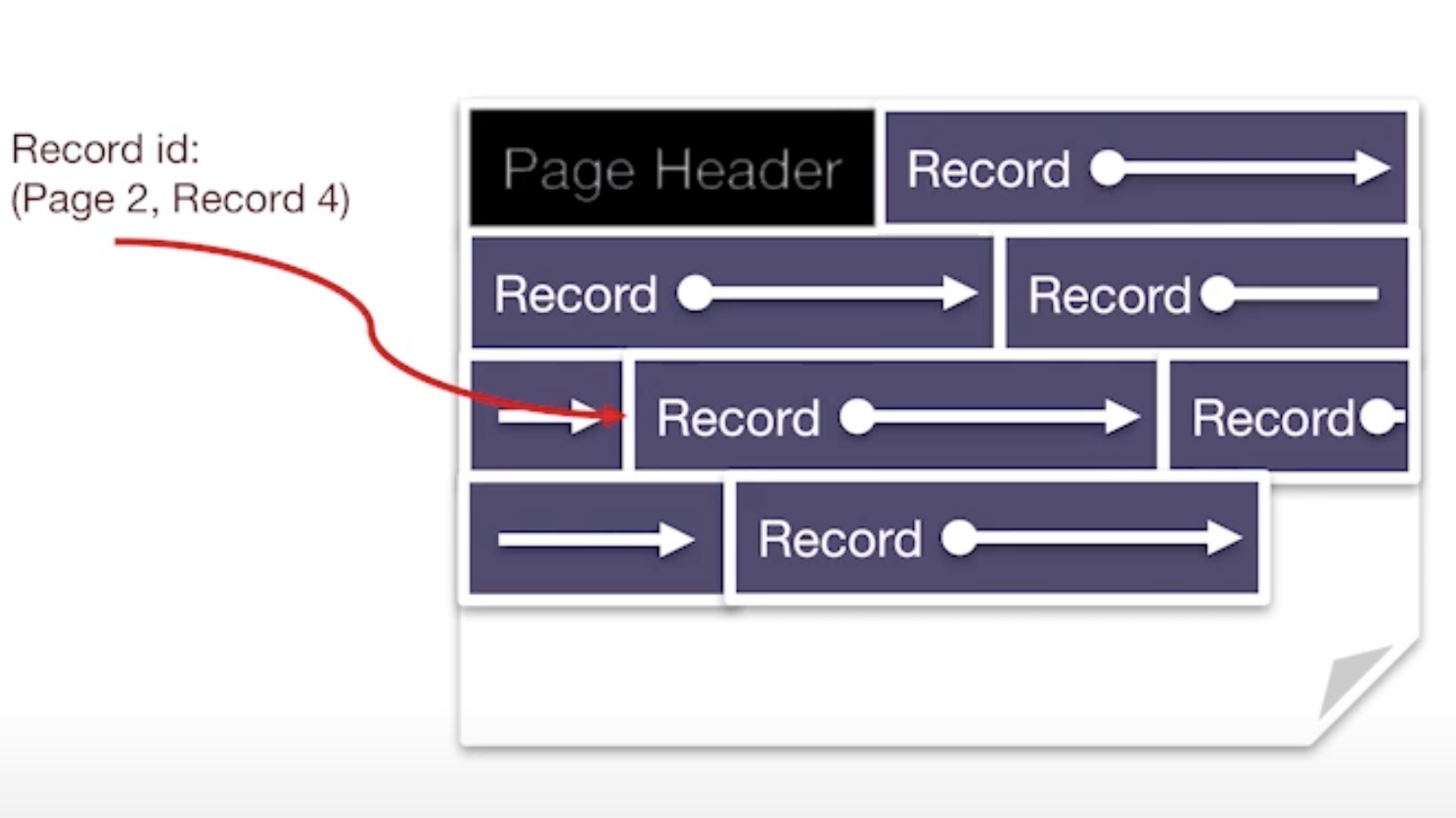
The problem is
- how to locate each record?
- how to insert and delete?
Slotted Page can address this issue
Slotted Page
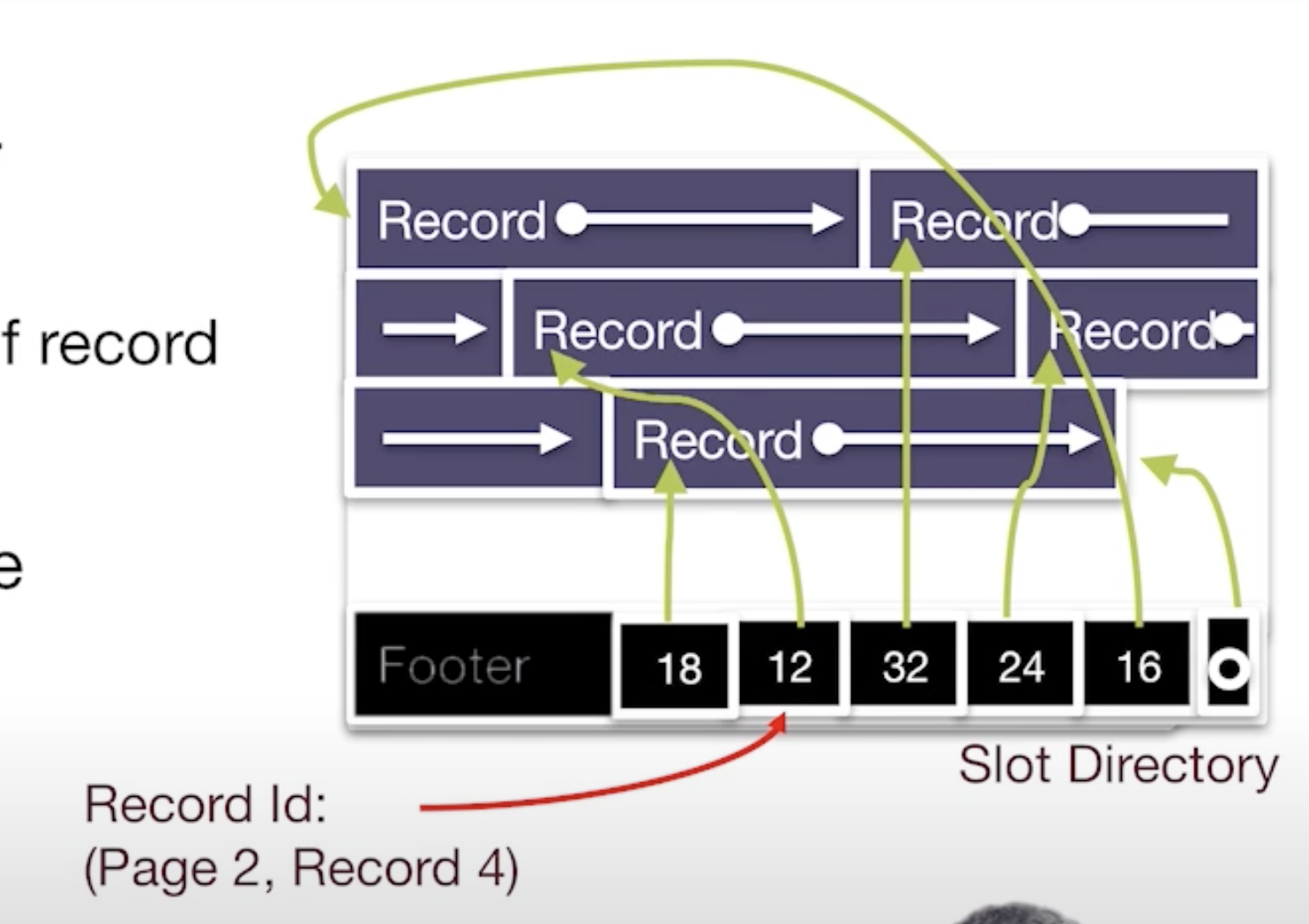
-
Slot directory in footer
- pointer to fre space
- Length + pointer to beginning of record (reverse order)
-
Delete: set 4th directory pointer to null
-
Insert:
- place record in free space on page,
- create pointer/length pair in the next open slot in slot directory
- Update the free space pointer
- Fragmentation ? ==> reorganize data on page
-
Reorganize
#$%^&*
Record layout
Record formats: fixed length
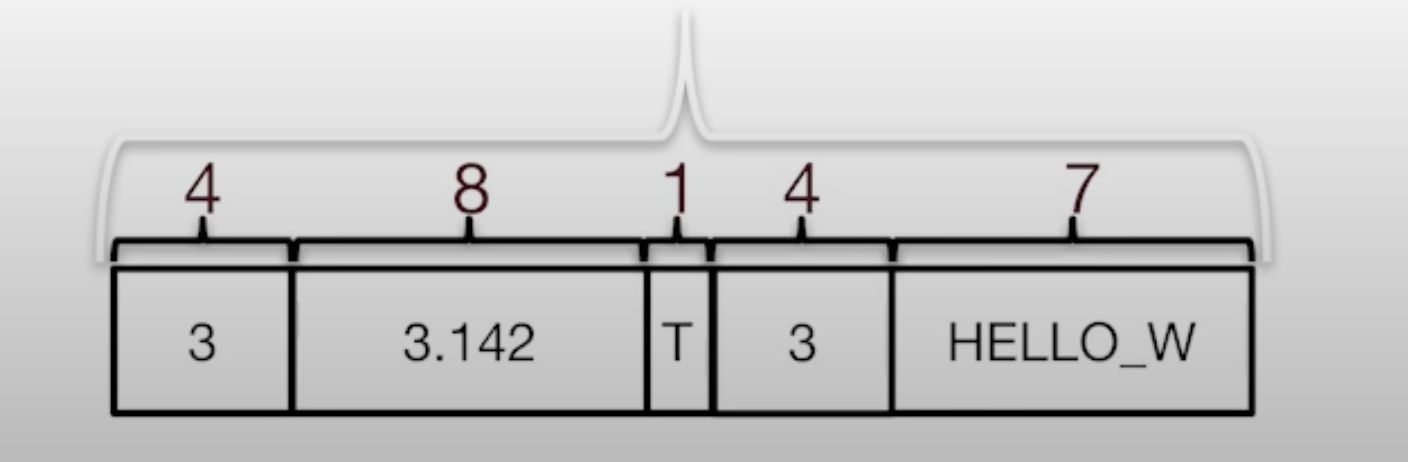
- field types same foir all record in a file
- Type info stored separately in system catalog
- On disk byte representation same as in memory
- Finding i’th field ==> done via arithmetic (fast)
- Compact?
Record formats: Variable length
e.g. varchar data is mutable
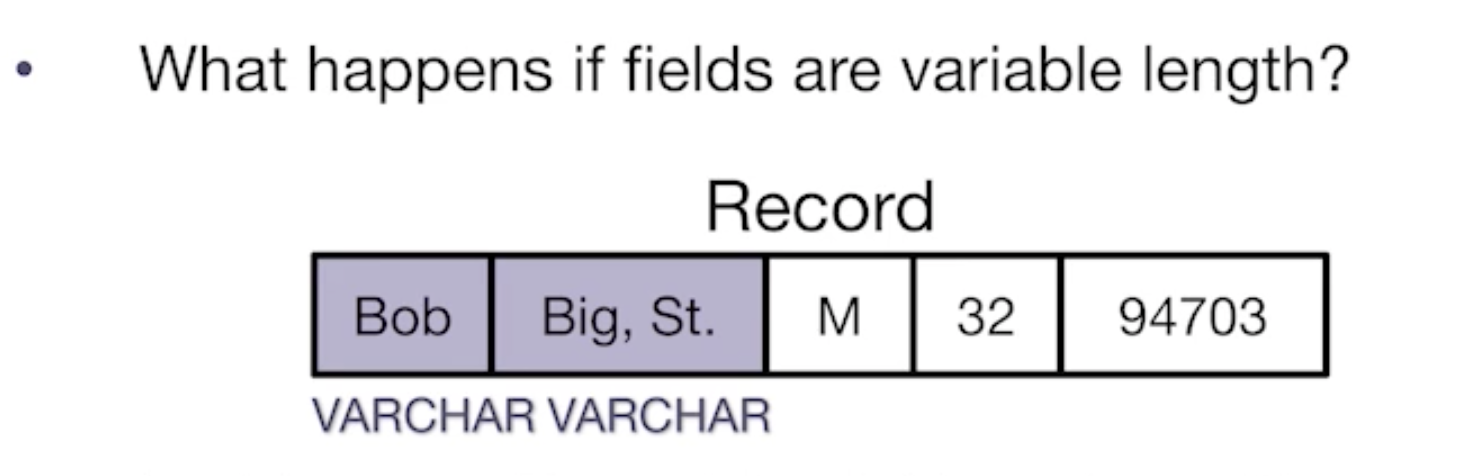
- could store with padding
- could use delimiters (i.e., CSV)
- could use record header (Best)
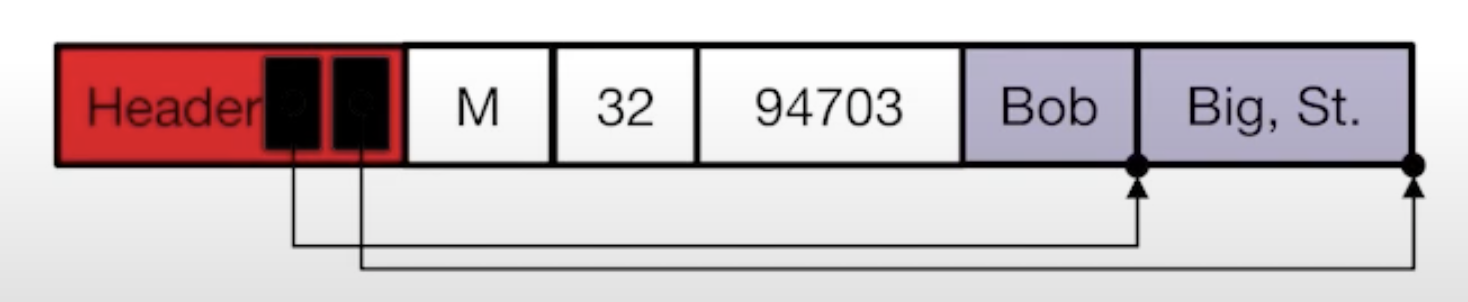
Record header use point to variables
Cost Model for Analysis
- B: the number of data blocks in the file
- R: Number of records per block
- D: (average) time to read/write disk block
heap files & sorted files
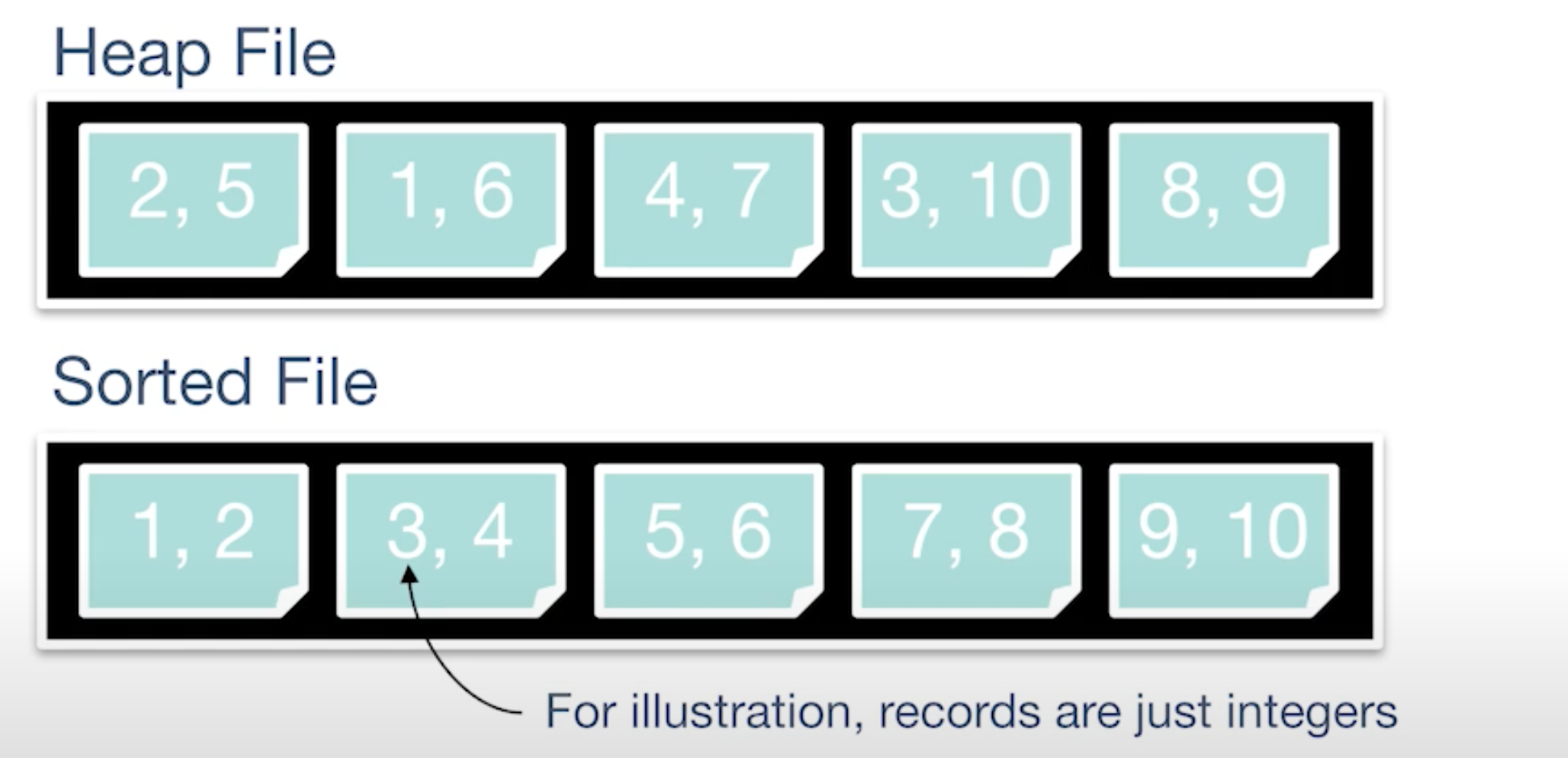
Cost of opetations
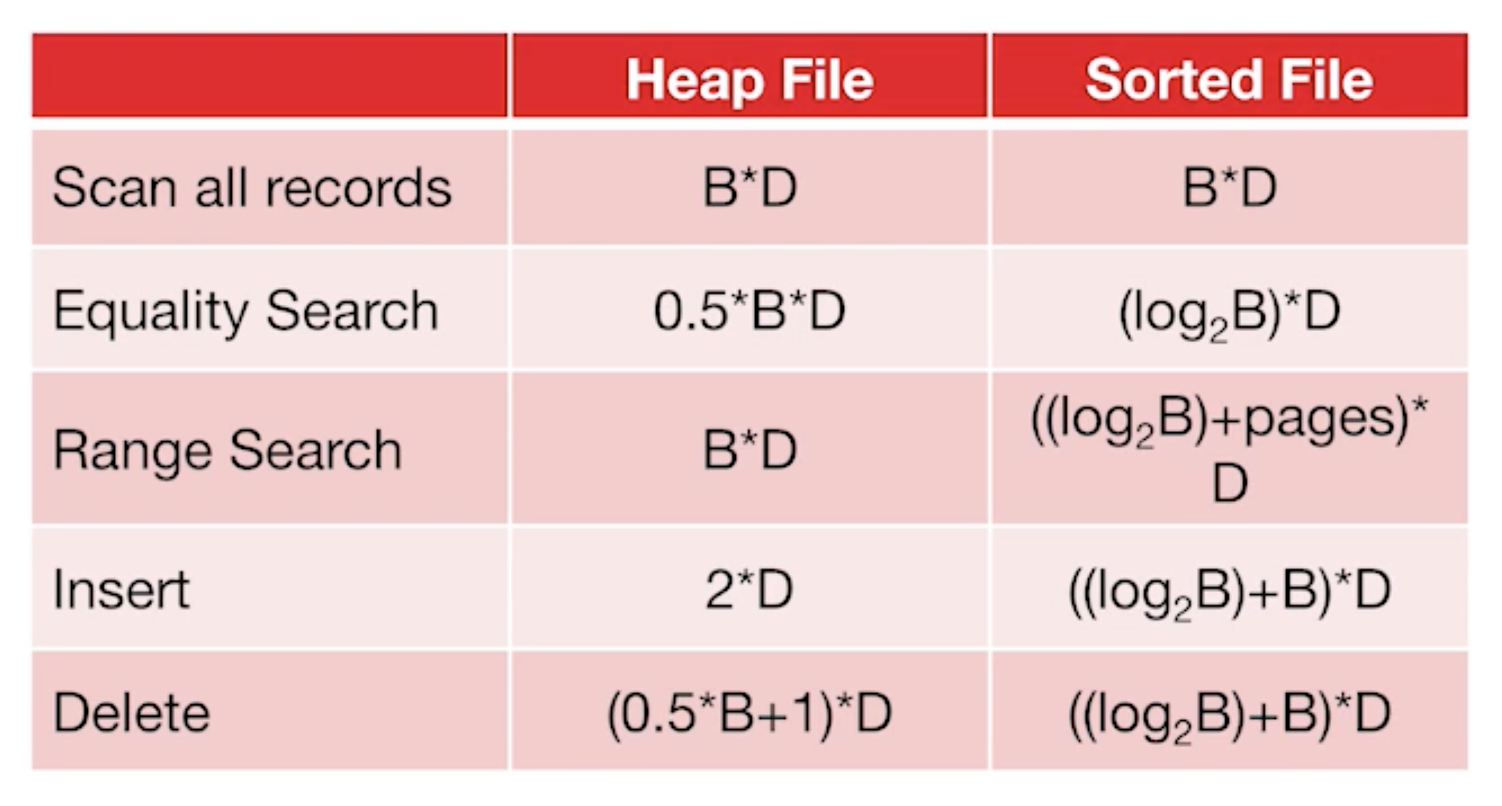
-
range search in sorted files: logB + pages
- logB denotes the cost to find the left border
- Pages denotes the cost to find the right border: scan from the end to find the right border.
-
Insert in heap file : 2 * D
- one for read. Read records from pages to memory
- one for write. Write records from memory to disk
Tree Index
to imporve Better: Use a page directory
-
ISAM is a static structure (static means inserting is not allowed)
- Only leaf pages modified, overflow pages needed
- Overflow chains can degrade performance unless size of data set and data distribution stay constant
-
B+ tree is a dynamic structure
- Inserts/deletes leave tree height-balanced: logN cost
- High fanout means depth rarely more than 3 or 4
- Almost always better than maintaining a sorted file
- Typically, 67% occupancy on average
- Usually perferable to ISAM
-
Bulk loading can be much faster than repeated insers for creating a B+ tree on a large data set
Index
An index is data structure taht enables fast lookup and modification of data entries by search key
B+ Tree
[外链图片转存失败,源站可能有防盗链机制,建议将图片保存下来直接上传(img-ldP6ai20-1655482515290)(https://raw.githubusercontent.com/Yukun4119/BlogImg/main/img/Screenshot%202021-09-15%20at%201.01.53%20PM.png)]
- d: order of tree (max fan-out = 2d + 1)
- d <= # entries <= 2d
- root is special, other node should be restricted with d <= # entries <= 2d
- e.g. from the figure above, we can find there are 5 pointers per page.
==> 2d+1 = 5
==> d = 2
B+ Tree and Scale
Height is 1
[外链图片转存失败,源站可能有防盗链机制,建议将图片保存下来直接上传(img-xmXHlliW-1655482515290)(https://raw.githubusercontent.com/Yukun4119/BlogImg/main/img/Screenshot%202021-09-21%20at%202.35.23%20PM.png)]
max fan-out = 5
d = 2
max leaf entries = 2d = 4
# Records: 5 * 4 = 20
Height is 3
[外链图片转存失败,源站可能有防盗链机制,建议将图片保存下来直接上传(img-719q6smu-1655482515291)(https://raw.githubusercontent.com/Yukun4119/BlogImg/main/img/Screenshot%202021-09-21%20at%202.37.02%20PM.png)]
max fan-out = 5
d = 2
max leaf entries = 2d = 4
# Records: 5^3 * 4 = 625
- B+ Tree Visualization website: https://www.cs.usfca.edu/~galles/visualization/BPlusTree.html
Search in B+ Tree
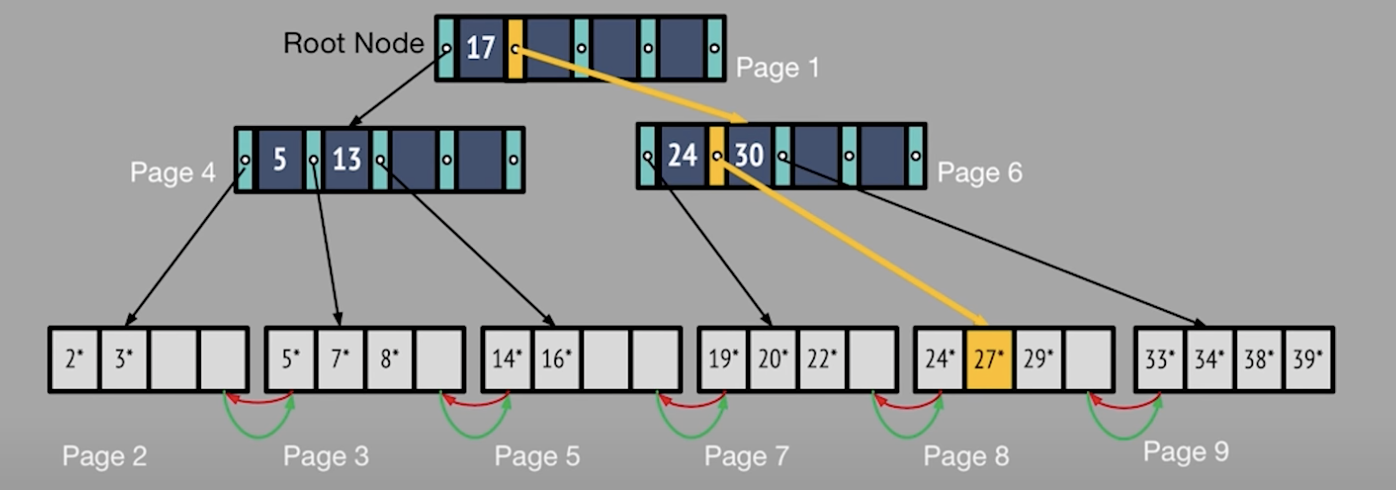
e.g. If we need to find the 3
at the height of 1, find key 3 and go to the its right child
Inserting in B+ Tree
Original tree:
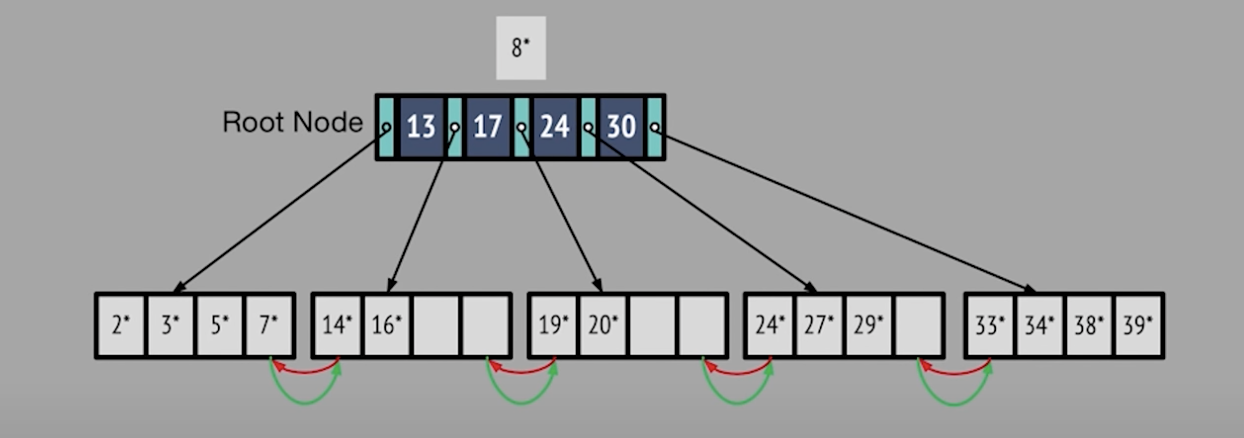
After inserting 8*
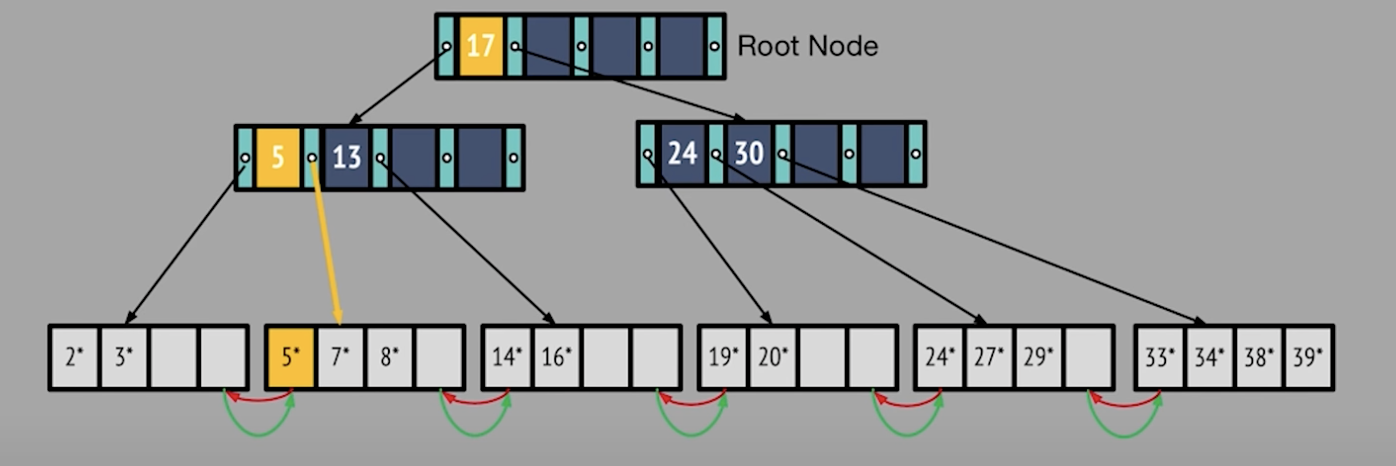
Leaf
5 was copied upIndex
17 was pushed up
Index Files and B+ Tree Refinements
Indexes: Basic Selection
- Basic Selection includes equality selections(op is =) and range selections (op is one of <, >, <=, >= BETWEEN)
- B+ tree provide both
- Linear Hash indexes provide only equality
Three alternatives for data entries in any index
- By Value
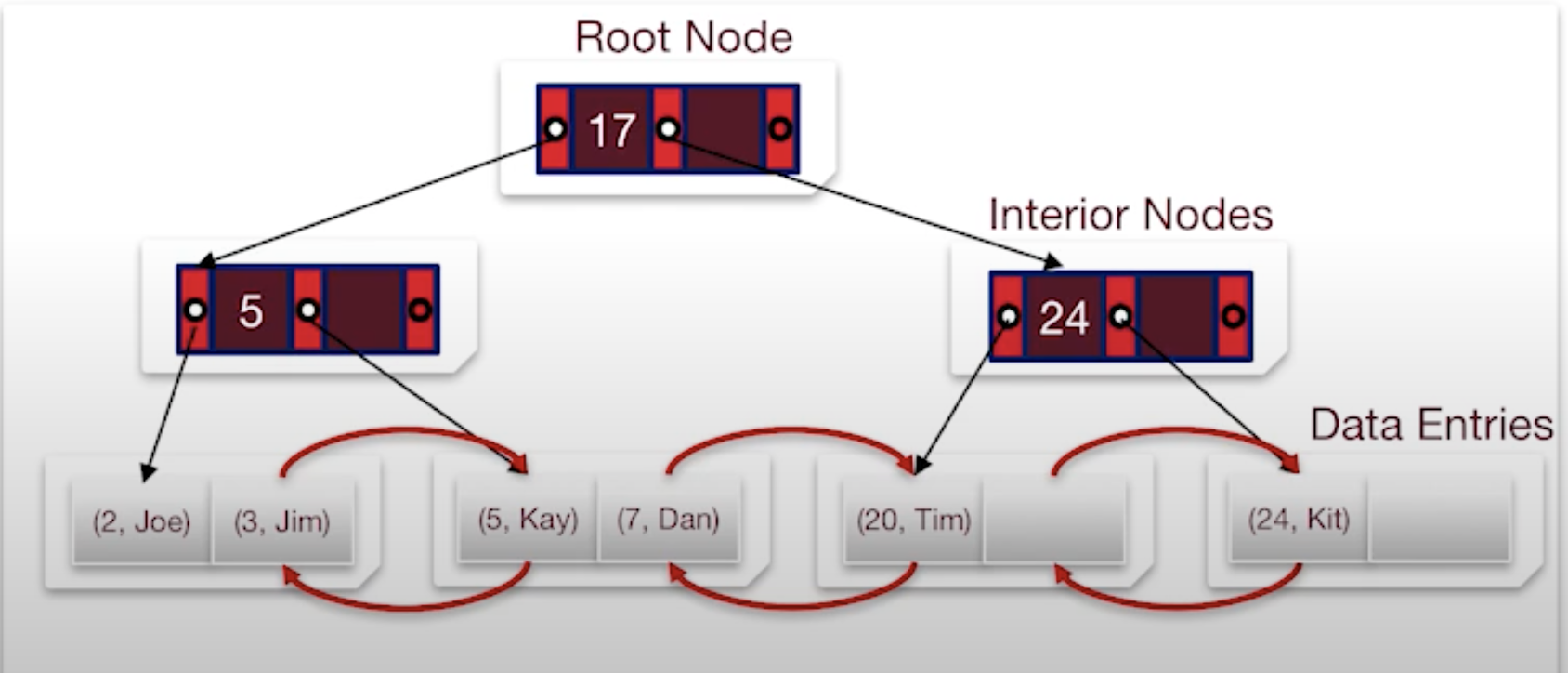
- By Reference
[外链图片转存失败,源站可能有防盗链机制,建议将图片保存下来直接上传(img-hhkSR9vi-1655482515292)(https://raw.githubusercontent.com/Yukun4119/BlogImg/main/img/Screenshot%202021-09-16%20at%206.42.26%20PM.png)]
- By List of references
Index By Reference vs. index By List of references
[外链图片转存失败,源站可能有防盗链机制,建议将图片保存下来直接上传(img-e7n765T0-1655482515293)(https://raw.githubusercontent.com/Yukun4119/BlogImg/main/img/Screenshot%202021-09-16%20at%206.44.32%20PM.png)]
Clustered vs. unclustered index
By Reference indexes(Alt 2 and 3) can be clustered or unclustered.
It is different definitions of “clustering” in AI
-
Clustered index:
- Heap file records are kept mostly ordered according to search keys in index
-
Unclustered index:
[外链图片转存失败,源站可能有防盗链机制,建议将图片保存下来直接上传(img-jO2neWaj-1655482515293)(https://raw.githubusercontent.com/Yukun4119/BlogImg/main/img/Screenshot%202021-09-16%20at%206.50.04%20PM.png)]
Cost Table
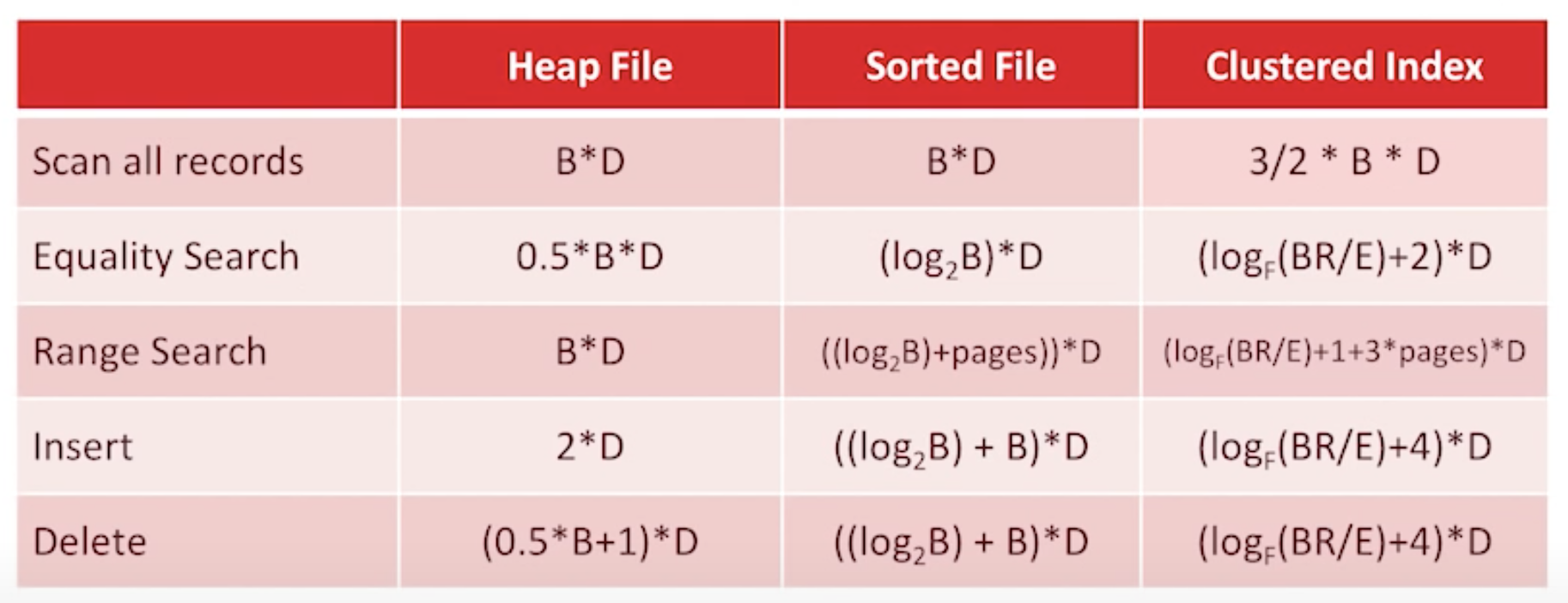
Big O notation:
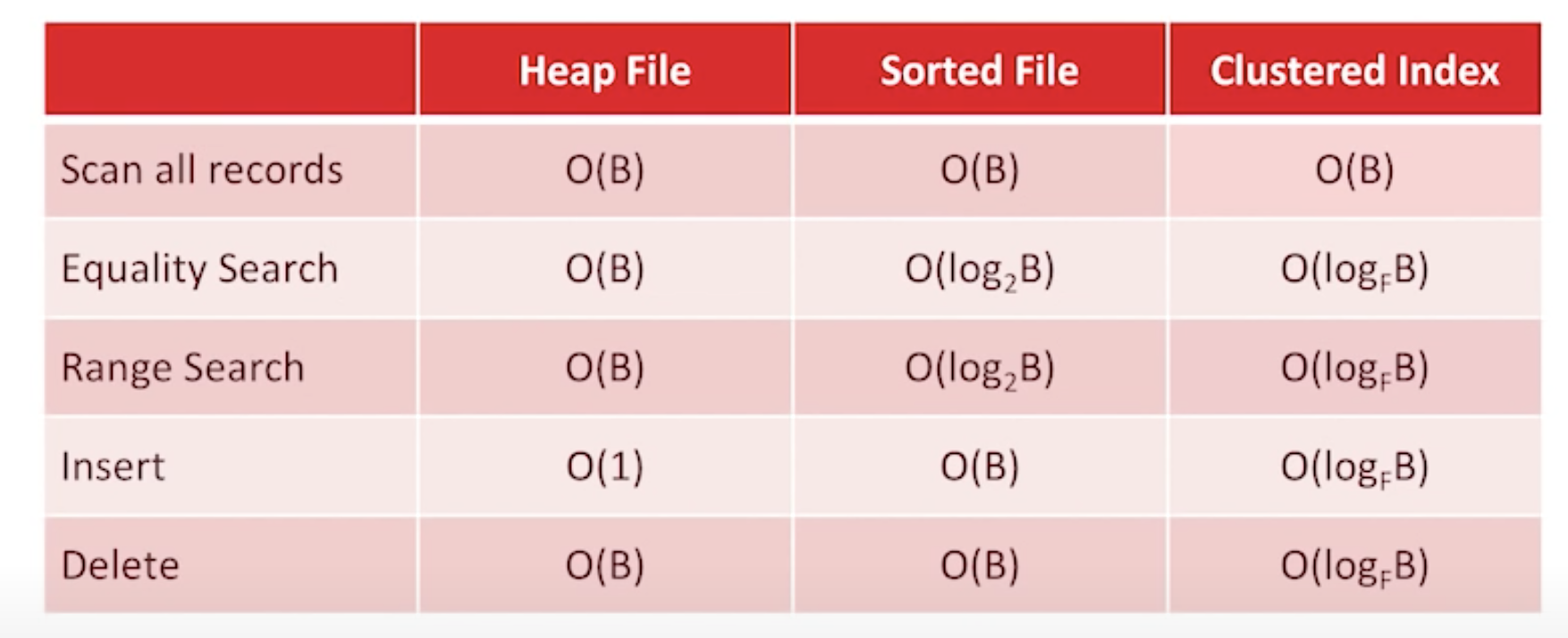
From Cow Book:
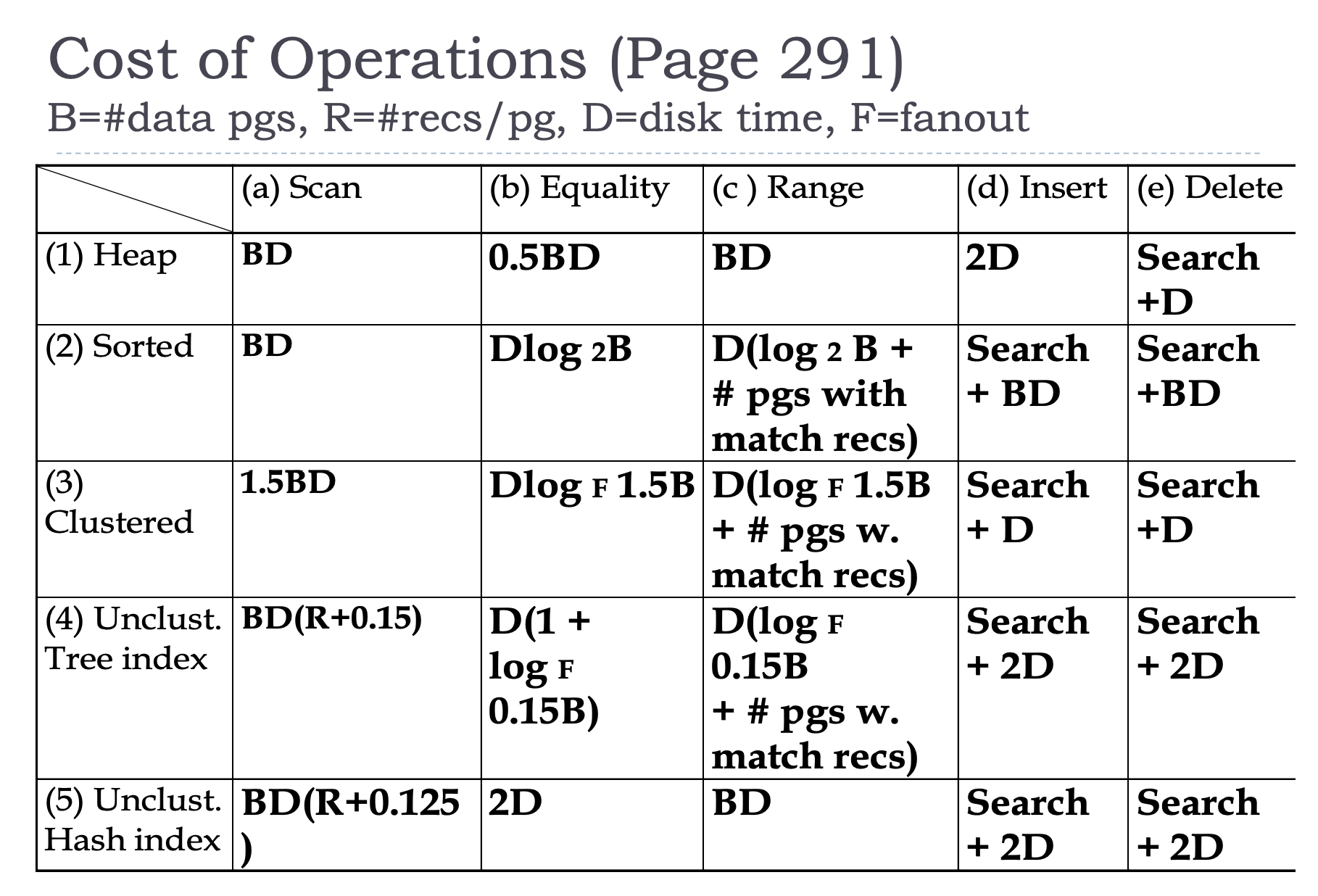
Buffer Management
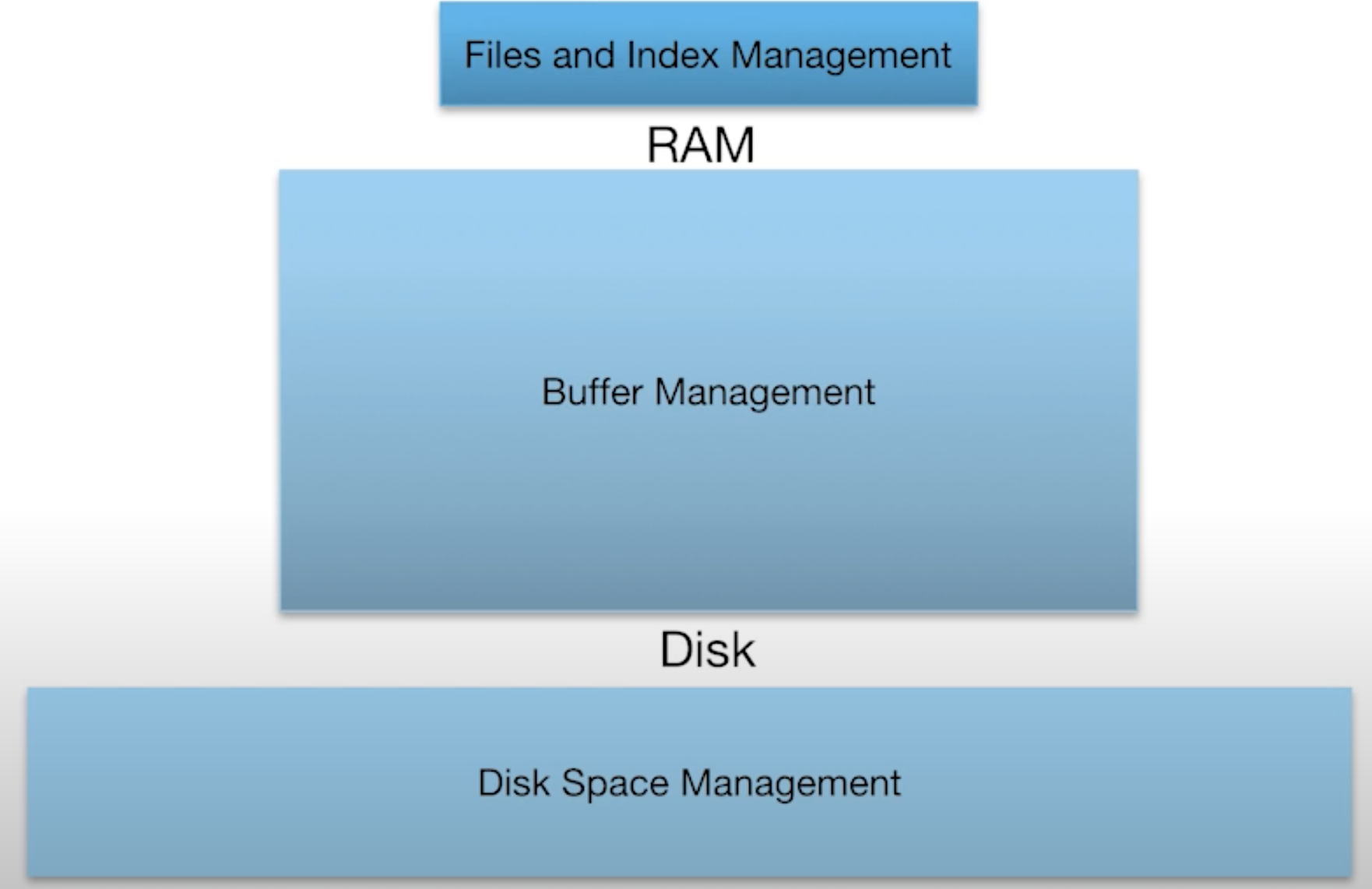
Handing dirty pages
- How will the buffer manager find out?
- Dirty bit on page
- What do with a dirty page?
- Write back via disk manager
Page Replacement
- How will the buffer mgr know if a page is in use
- Page pin count
- If buffer manager is full, which page should be replaced?
- page replacement policy
replacement policy
- LRU(Least Recently Used), clock
- MRU(Most-recently-used)
Tips for LRU and Clock
- LRU may be cost in squential flooding
- MRU performs better in squential flooding
- Clock may be cheap (why?)
Sorting and hashing
Hashing pros:
- For duplicate elimination, scales with # of values
- Delete dups in first pass while partitioning on hp
- Vs. sort which scales with # of iterms
- Easy to shuffle qeually in parallel case
Sorting
- Great if we need output to be sorted anyway
- Not sensitive to duplicates or bad hash functions
Relational Algebra
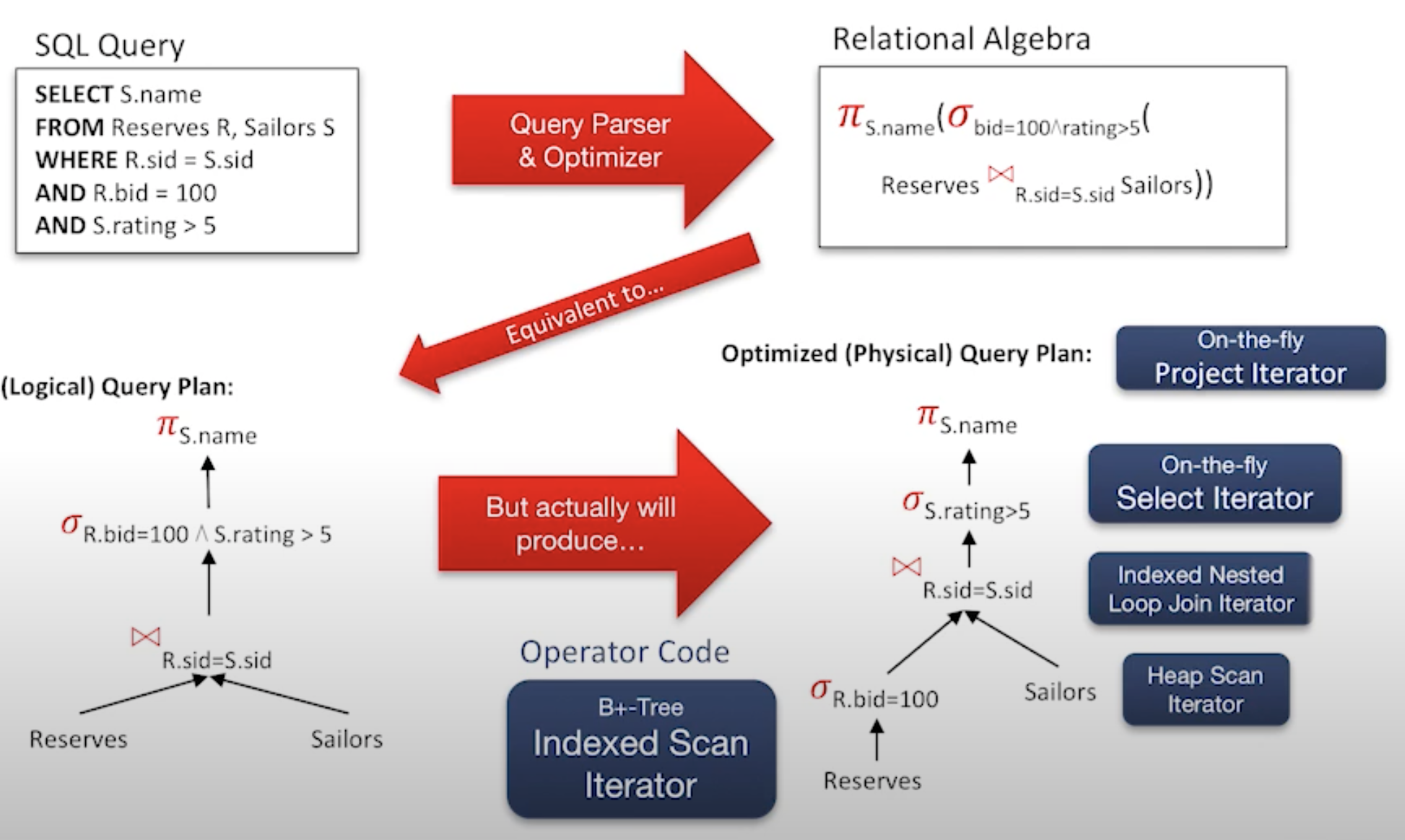
Terminolog | Symbol | Meaning |
---|---|---|
Projection | π | Retains only desired columns(vertical) |
Selection | σ | Selects a subset of rows(horizontal) |
Renaming | ρ | Rename attributes and relations |
Union | υ | Tuple in r1 or in r2 |
Set-difference | - | Tuples in r1, but not in r2 |
Cross-product | x | Allow us to combine two relations |
Intersection | ∩ \cap ∩ | Tuples in r1 and in r2 |
Joins | ⋈ | combine relations that satisfy predictions |
[外链图片转存失败,源站可能有防盗链机制,建议将图片保存下来直接上传(img-GLfy0aEZ-1655482515295)(https://raw.githubusercontent.com/Yukun4119/BlogImg/main/img/Screenshot%202021-09-17%20at%204.52.35%20PM.png)]
[外链图片转存失败,源站可能有防盗链机制,建议将图片保存下来直接上传(img-jcknE0z1-1655482515296)(https://raw.githubusercontent.com/Yukun4119/BlogImg/main/img/Screenshot%202021-09-17%20at%204.54.22%20PM.png)]
[外链图片转存失败,源站可能有防盗链机制,建议将图片保存下来直接上传(img-hwAm3wjv-1655482515297)(https://raw.githubusercontent.com/Yukun4119/BlogImg/main/img/Screenshot%202021-09-17%20at%204.56.00%20PM.png)]
[外链图片转存失败,源站可能有防盗链机制,建议将图片保存下来直接上传(img-laMFiKPX-1655482515297)(https://raw.githubusercontent.com/Yukun4119/BlogImg/main/img/Screenshot%202021-09-17%20at%204.56.23%20PM.png)]
[外链图片转存失败,源站可能有防盗链机制,建议将图片保存下来直接上传(img-1ztl5Q8q-1655482515298)(https://raw.githubusercontent.com/Yukun4119/BlogImg/main/img/Screenshot%202021-09-17%20at%204.58.24%20PM.png)]
[外链图片转存失败,源站可能有防盗链机制,建议将图片保存下来直接上传(img-cO3Va9ZN-1655482515299)(https://raw.githubusercontent.com/Yukun4119/BlogImg/main/img/Screenshot%202021-09-17%20at%205.00.56%20PM.png)]
[外链图片转存失败,源站可能有防盗链机制,建议将图片保存下来直接上传(img-yLmRPBw3-1655482515300)(https://raw.githubusercontent.com/Yukun4119/BlogImg/main/img/Screenshot%202021-09-17%20at%205.03.06%20PM.png)]
[外链图片转存失败,源站可能有防盗链机制,建议将图片保存下来直接上传(img-yZ6eBfjL-1655482515300)(https://raw.githubusercontent.com/Yukun4119/BlogImg/main/img/Screenshot%202021-09-17%20at%205.03.53%20PM.png)]
[外链图片转存失败,源站可能有防盗链机制,建议将图片保存下来直接上传(img-5X1NYssk-1655482515301)(https://raw.githubusercontent.com/Yukun4119/BlogImg/main/img/Screenshot%202021-09-17%20at%205.05.05%20PM.png)]
Iterators
omitted
Join Operations
Schema for Examples
- Cost Notation
- [R]: the number of pages to store R
- p R p_R pR: number of records per page of R
- |R|: the cardinality ( number of records) of R
- ==> |R| = p R p_R pR * [R]
Parallel Query Processing
A little history
- Relational revolution
- declarative set-oriented primitives
- 1970s
- Parallel relkational database systems
- on commodity hardware
- 1980s
- Bit data: MapReduce, Spark, etc.
- scaling to thousands of machiens and beyond
- 2005-2015
Roughly 2 Kinds of Parallelism
- Pipeline
- Partition
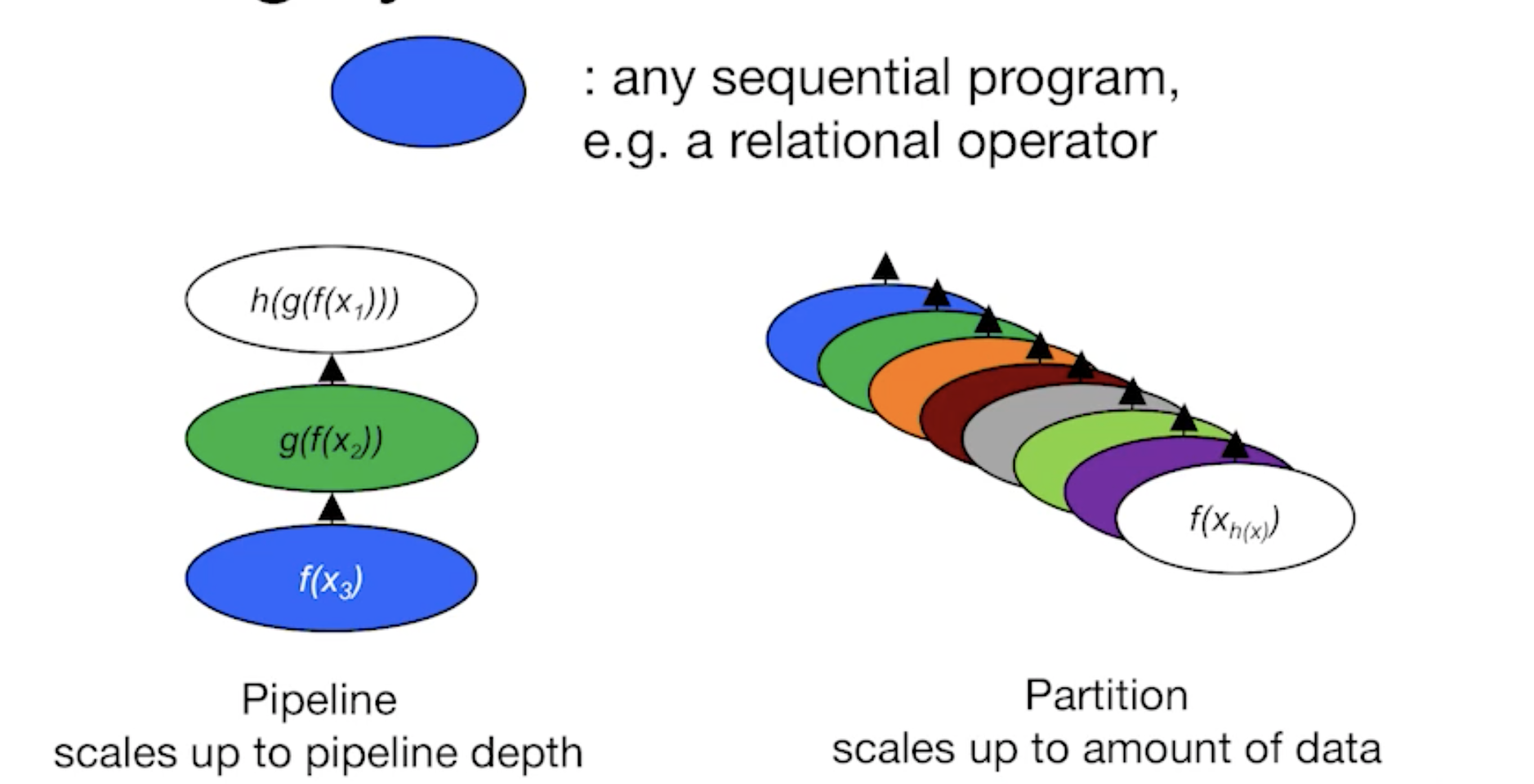
Parallel Architectures
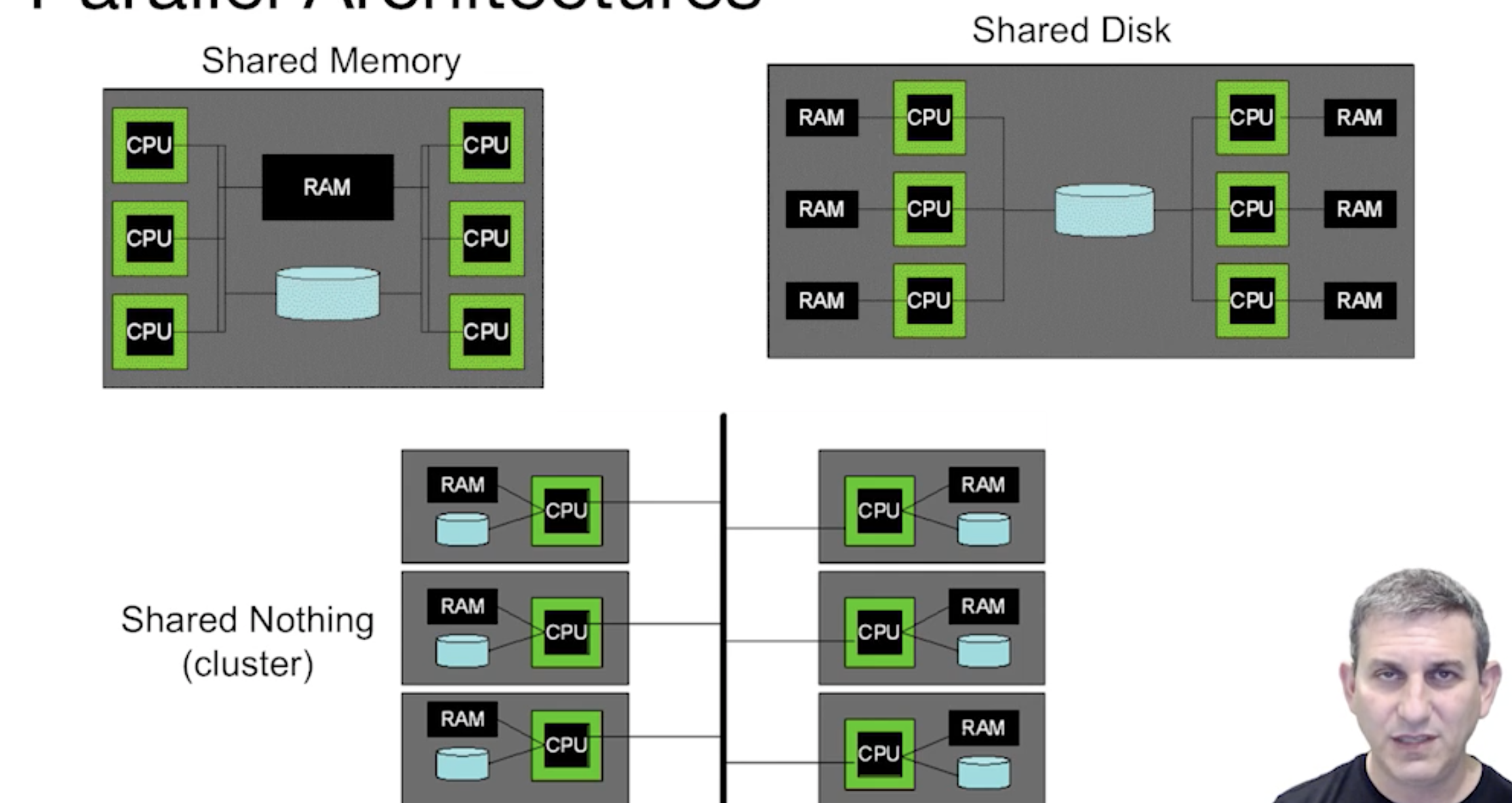
Kinds of Query Parallelism
-
Inter: “between”,“across”
-
Intra: “within”
-
Each query runs on a separate processor
Intra Query - Inter operator
[外链图片转存失败,源站可能有防盗链机制,建议将图片保存下来直接上传(img-kqR97ldf-1655482515301)(https://raw.githubusercontent.com/Yukun4119/BlogImg/main/img/Screenshot%202021-10-11%20at%204.15.39%20PM.png)]
Data Partitioning
todo
Parallel DBMS Summary
- Parallelism natural to query processing
- Both pipeline and partition
- Shared-Nothing vs. Shard-Mem vs. Shard Disk
- Shared-mem easiest SW, costliest HW.
- Doesn’t scale indefinitely
- Shared-nothing cheap, scales well, harder to implement
- Shared disk a middle ground
- For updates, introduces icky stuff related to concurrency control
- Shared-mem easiest SW, costliest HW.
- intra-op, inter-op, & inter-query parallelism all possible
- Data layout choices important
- Most DB operations can be done partition-parallel
- Sort, Hash
- sort-merge join, hash-join
- Complex plans
- Allow for pipeline-parallelism, but sorts, hashes block the pipeline
- Inter-op parallelism alos achieved via bushy trees
- Transactions require introducing some new protocols
- distributed deadlock detection
- Two-phase commit (2PC)
- 2PC not great for availability, latency
- single failure stalls the whole system
- transaction commit waits for the slowest worker
Relational Query Optimizaiton 1: The Plan Space
- Overview
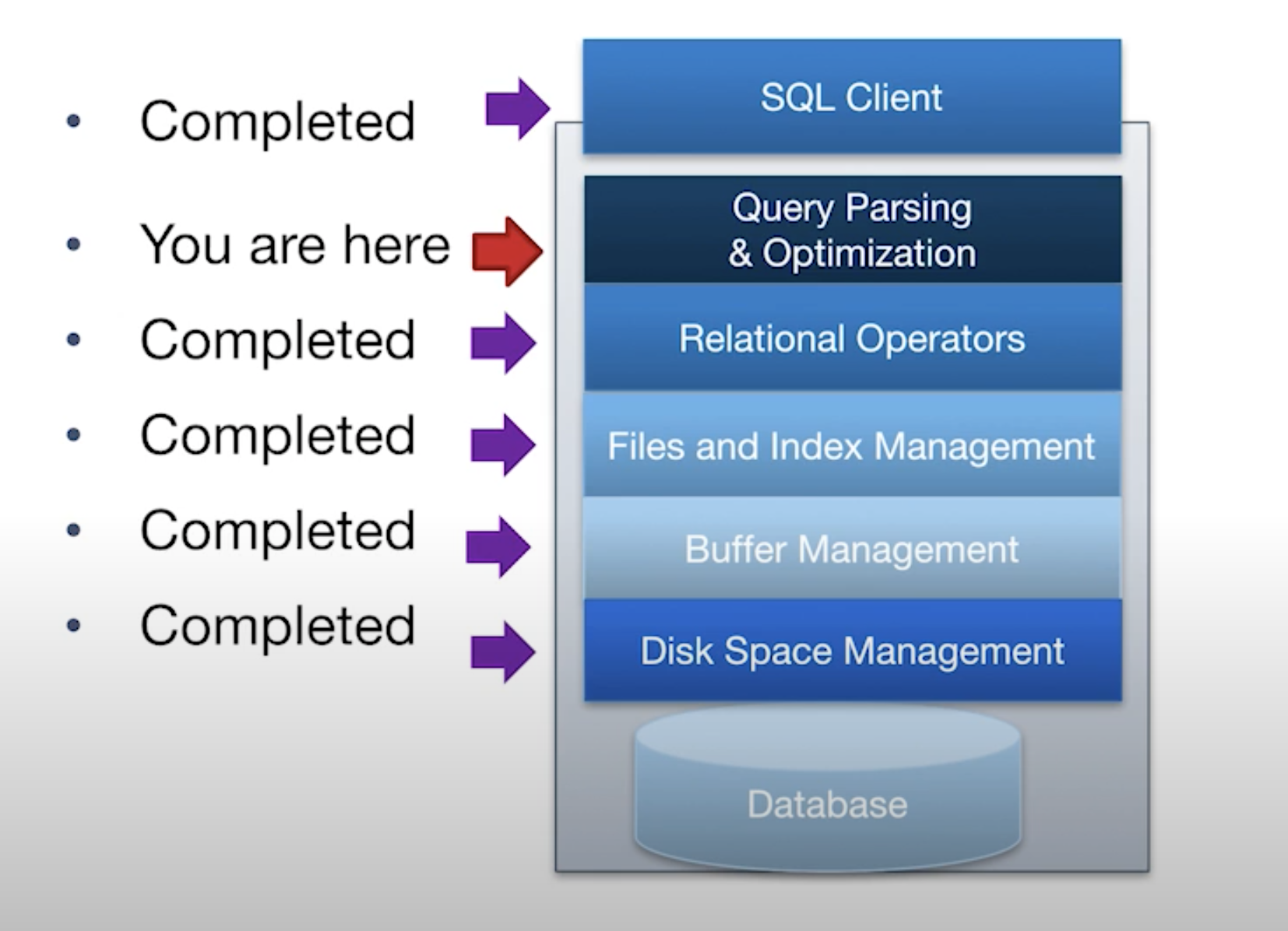
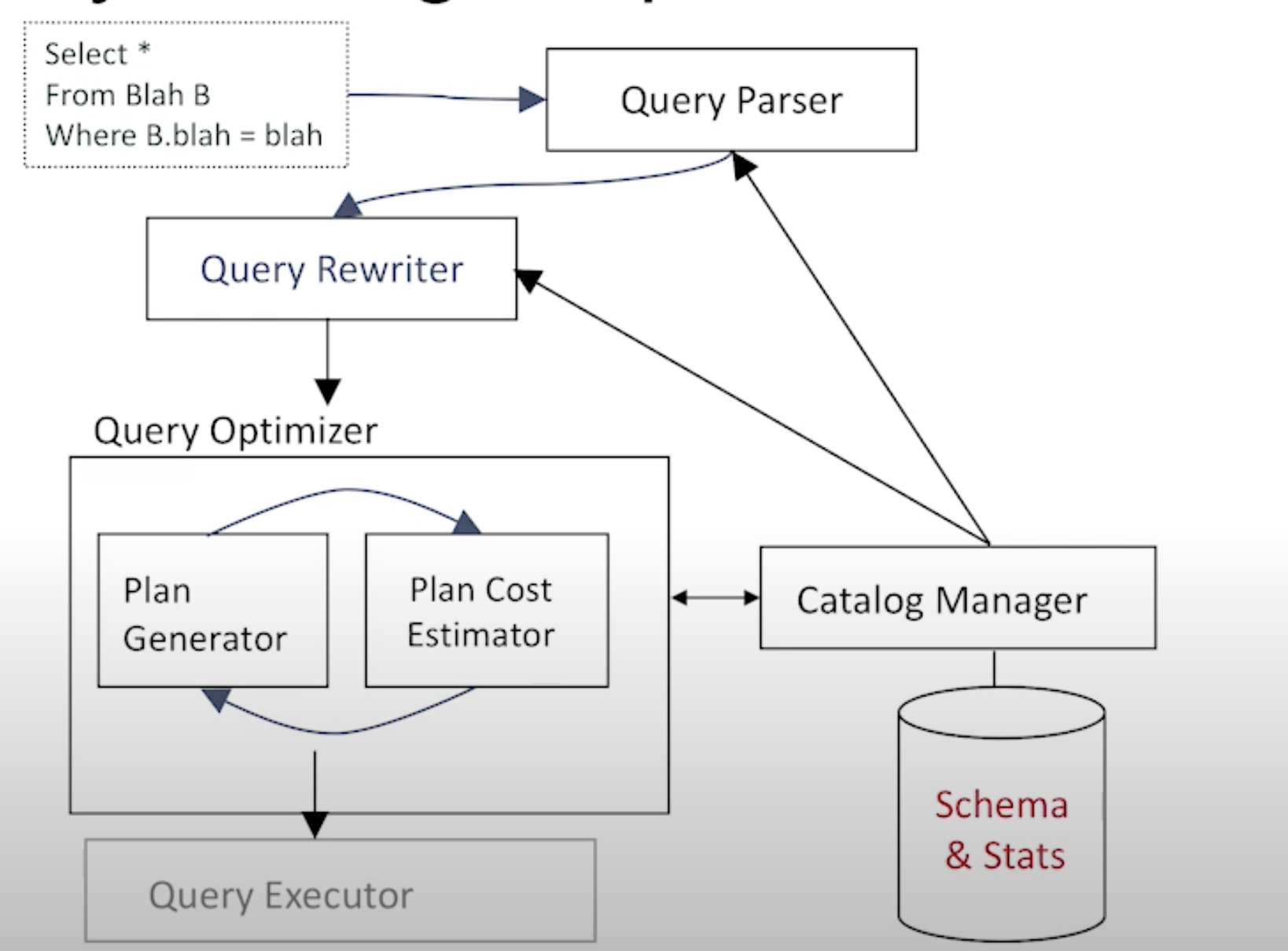
-
Query parser
- Checks correctness, authorisation
- Generates a parse tree
- straightforward
-
Query rewriter
- Converts queries to canonical form
- flatten views
- Subqueries into fewer query blocks
- weak spot in many open-source DBMSs
- Converts queries to canonical form
-
“Cost-based” Query Optimizer
- Optimizes 1 query block at a time
- Select, Project, Join
- GroupBy/Agg
- Order By(if top-most block)
- Uses catalog states to find least-“cost” plan per query block
- “Soft underbelly” of every DBMS
- Sometimes not trully “optimal”
- Optimizes 1 query block at a time
Relational Algebra Equivalences: Selections
-
Selections
-
Projections

-
Cartesian product
Some common heuristics
todo
Running Example
- Plan1
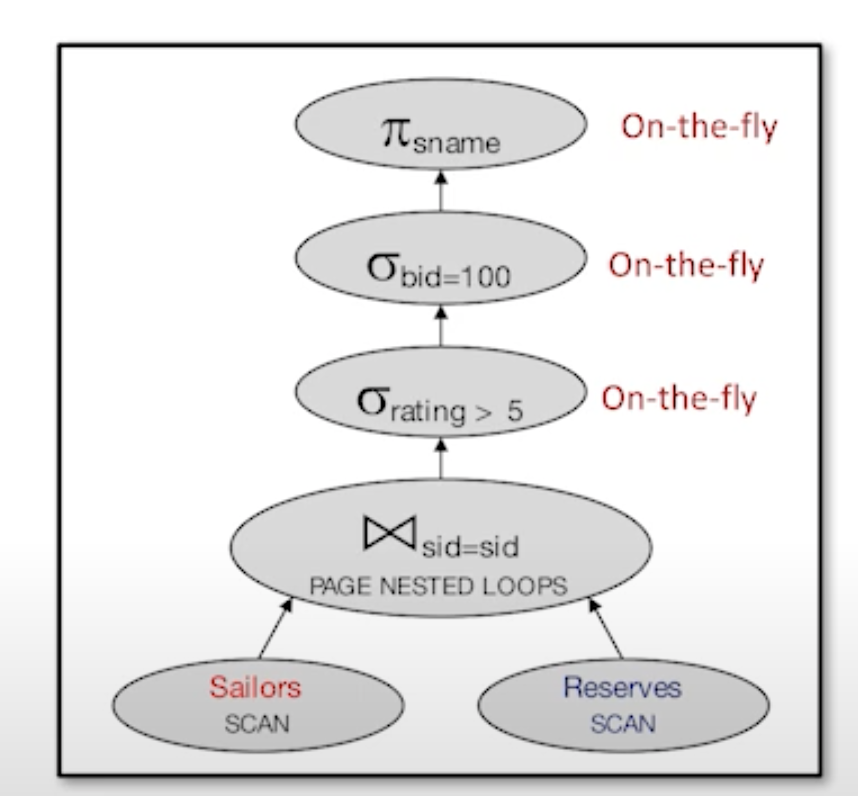
Cost: 500 + 500 * 1000 I/Os
- Plan2:
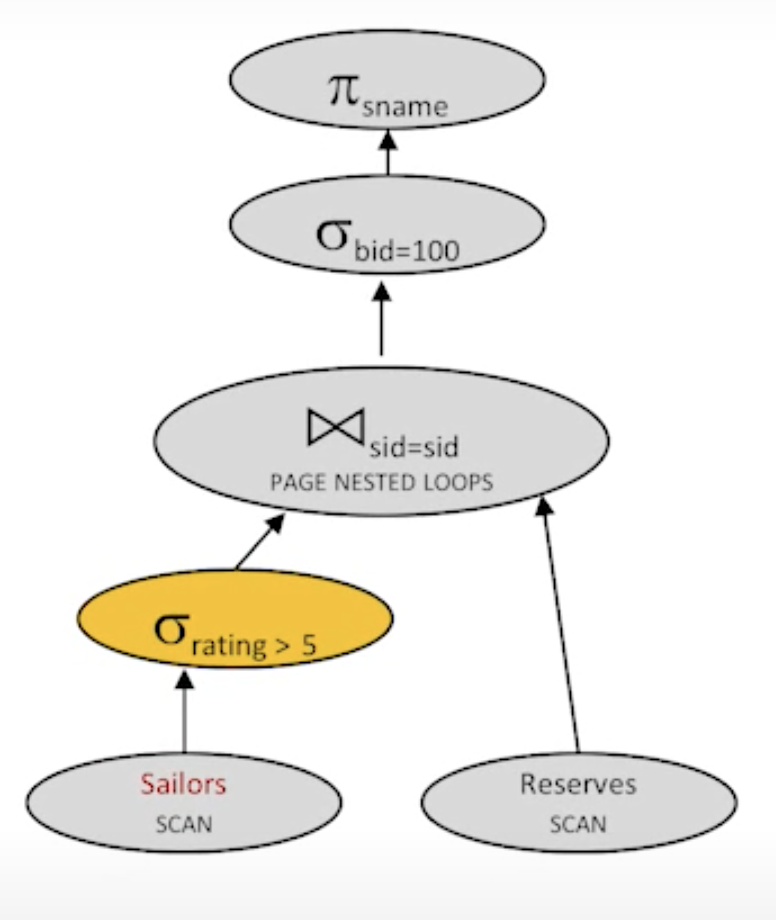
cost: 500 + 250 * 1000 I/Os
(Assumed that data is evenly distributed)
- Plan3:
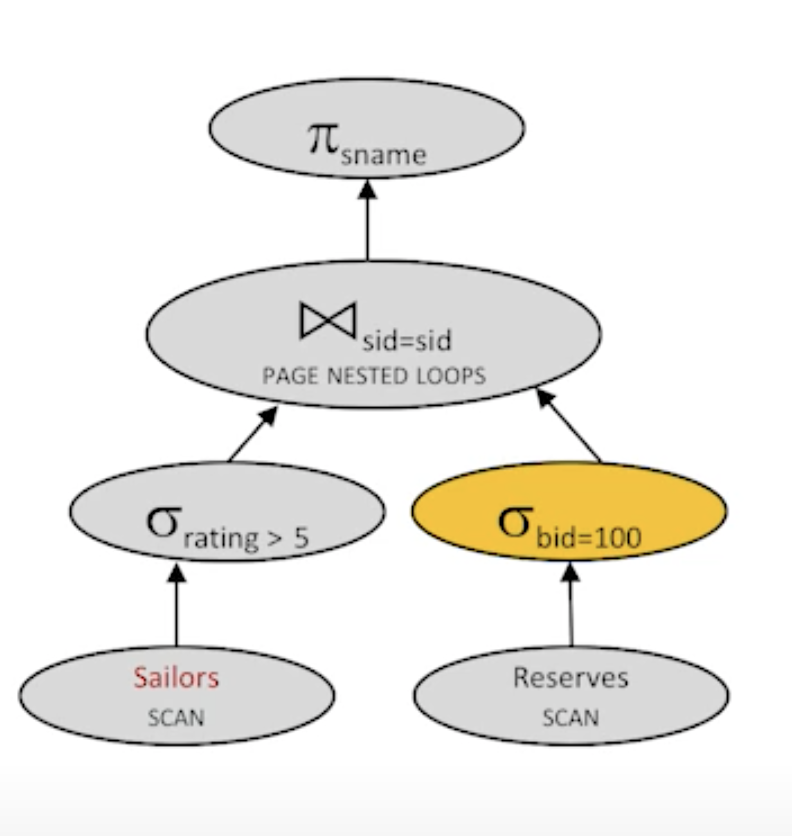
cost : 500 + 250 * 1000 I/Os
- plan4 (exchange join sequence)

cost: 1000 + 10 * 500 I/Os
- Plan5:
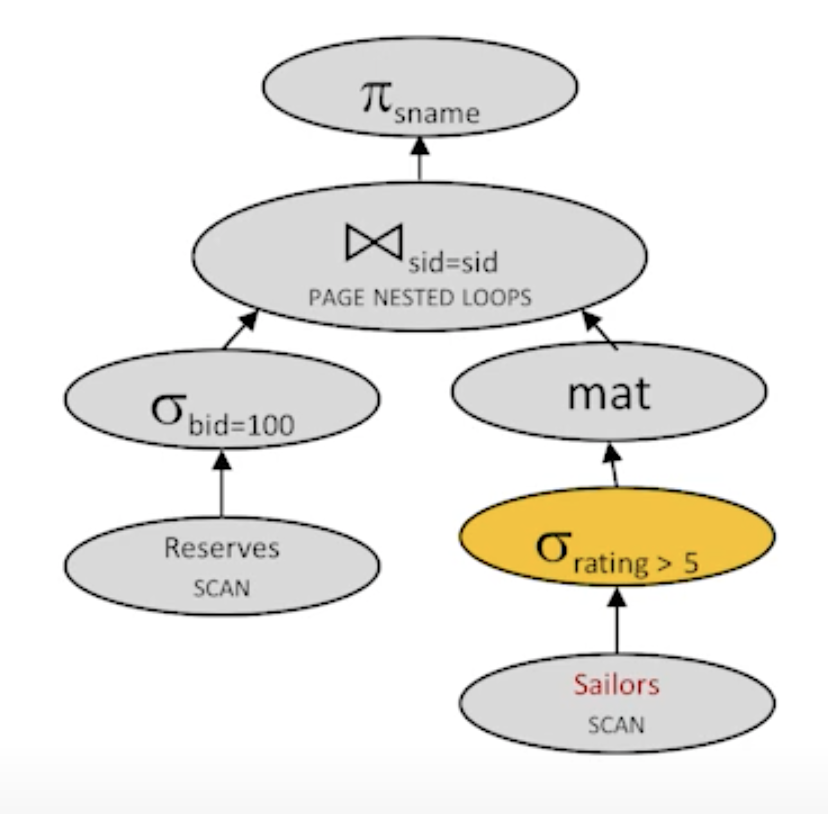
cost: 1000 + 500 + 250 + 10 * 250
- plan6
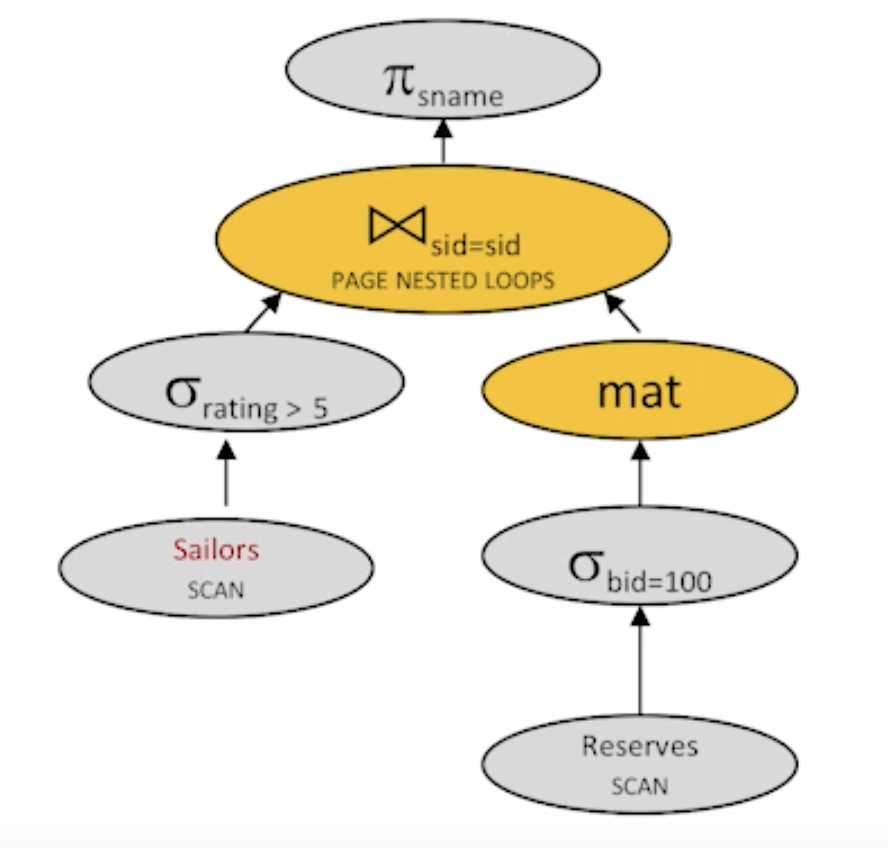
cost: 500 + 1000 + 10 + 250 * 10
- Plan7:
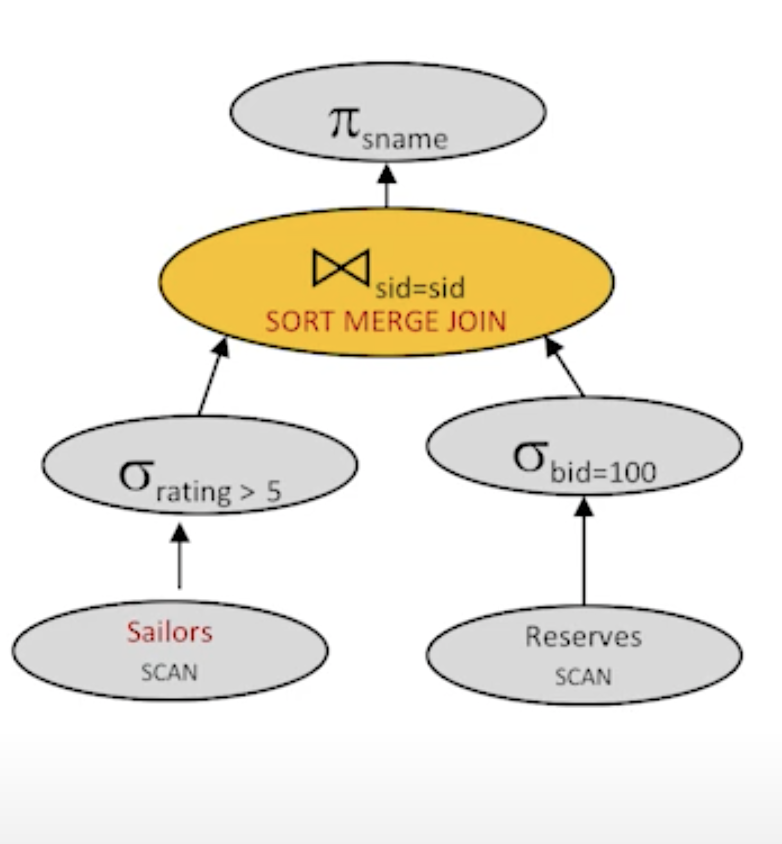
cost : 1000 + 500 + sort reserves(10 + 10 * 2) + sort sailors (250 + 3 * 2 * 250) + merge(10 + 250) = 3630 I/Os
- plan 8:
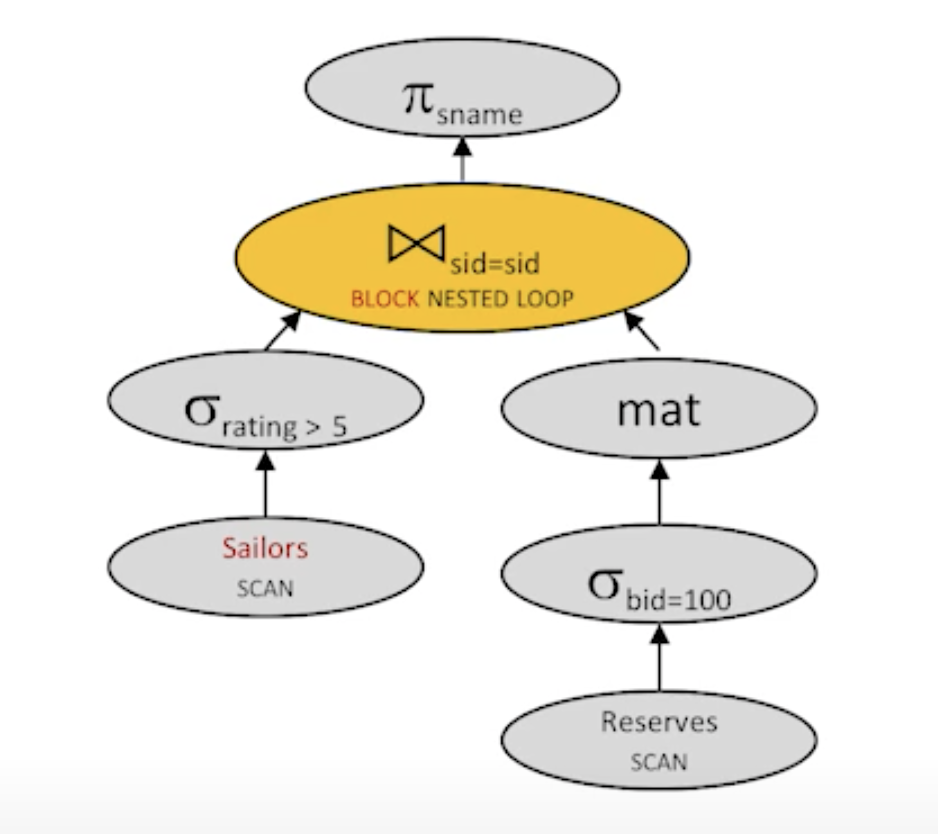
cost: 500 + 1000 + 10 + (ceil(250 / 4) * 10) = 2140 I/Os
- Plan9:
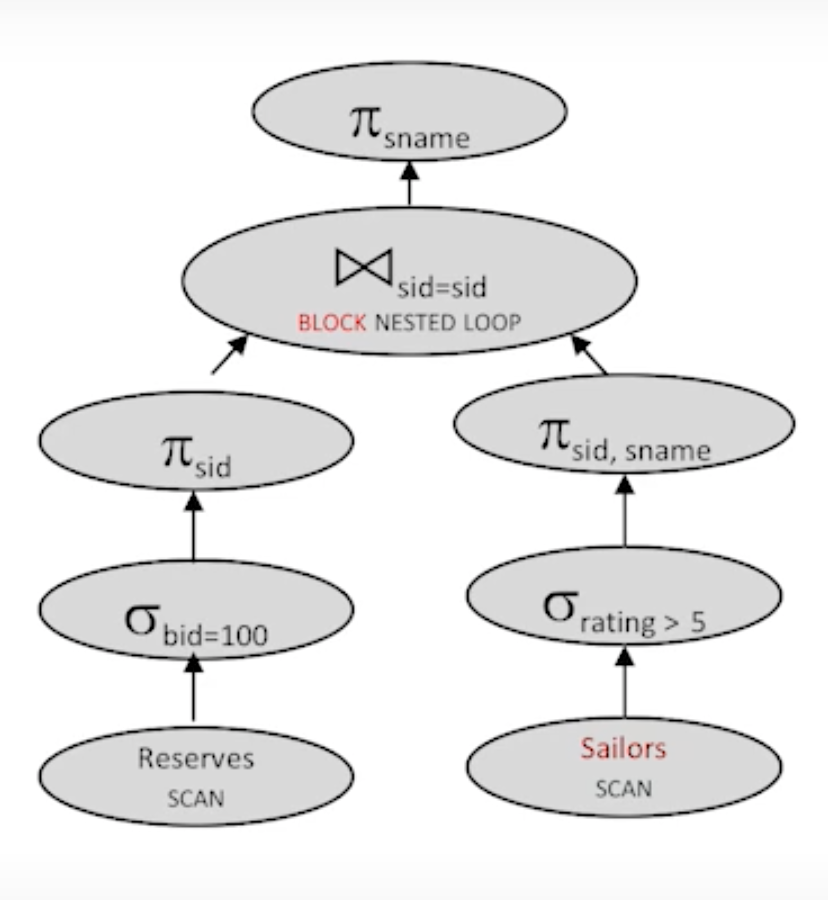
Total : 1500
===> It is the best plan so far.
- plan 10
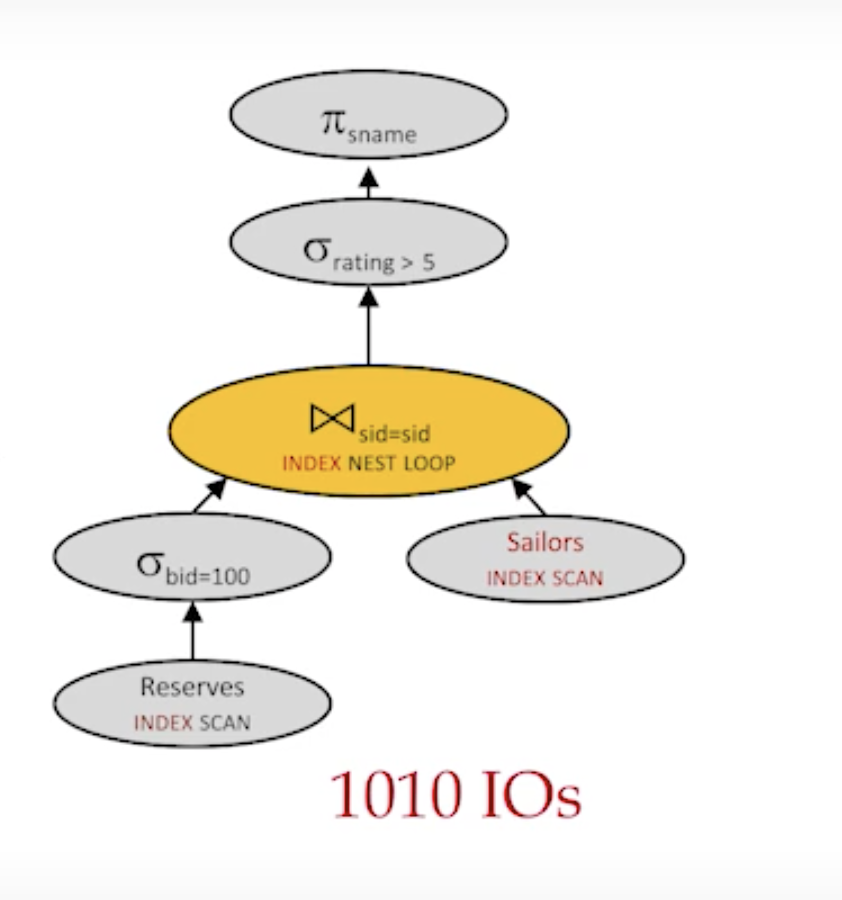
cost: 1010 I/Os
(with index)
Relational Query Optimization 2: Costing and Searching
- Plan Space
- Cost Estimation
- Search Algorithm
Query Blocks: Units of Optimization
- Beark query into query blocks
- Optimize one block at at time
- Uncorrelated nested blocks computed once
- Correlated nested blocks are like function calls
- But sometimes can be “decorrelated”
Information Retrieval (IR)
IR vs. DBMS
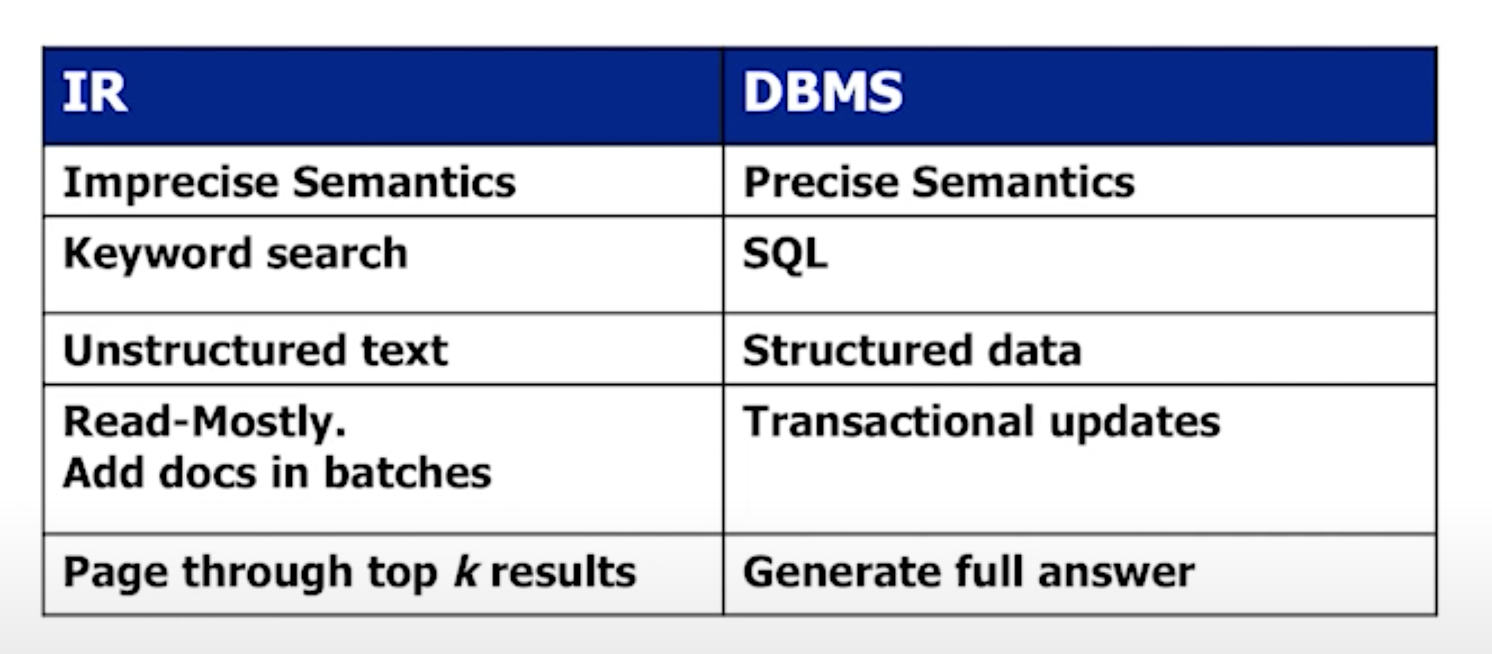
Not as different as they might seem