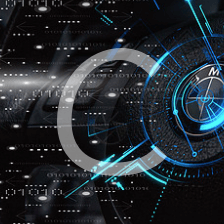
medical report generation
文章平均质量分 92
ying wong
你没资格懈怠和偷懒
展开
-
Competence-based Multimodal Curriculum Learning for Medical Report Generation (ACL 2021) 解读+总结
摘要:针对两个问题:【1】严重的数据偏差:视觉数据偏差:数据集中正常样本图像的比例远超于异常样本图像;此外,每个异常样本图像中,正常区域的占比远超于异常区域的占比。 文本数据偏差:放射学家标注的参考报告,通常遍历所有部位生成了描述,使得整个报告中正常本文描述占了绝大多数。此外,描述同一个部位的许多句子重复率极高。【2】有限的医学数据: 大部分现有的方法不会考虑它们的难度,而统一进行随机采样。有限数据的不平衡的数据偏差将会误导模型训练。所以,提出了CMCL(具体做法):...原创 2021-12-10 20:47:56 · 3253 阅读 · 1 评论 -
Generating Radiology Reports via Memory-driven Transformer (EMNLP-2020)
Generating Radiology Reports via Memory-driven Transformer文章链接:https://www.aclweb.org/anthology/2020.emnlp-main.112.pdf摘要医学图像常被用于临床诊断和医疗诊断的实验。写医学报告对于无经验的医生来说是一个耗时且容易出错的。因此,自动地生成医疗报告被赋予高期望去减轻放射科专家的工作量,并推动临床自动,这对于应用人工智能到医学领域是一个关键任务。本文,我们提出利用memory-driven的原创 2021-06-25 18:36:04 · 2441 阅读 · 17 评论 -
Hybrid Retrieval-Generation Reinforced Agent for Medical Image Report Generation(NIPS 2018)总结
Hybrid Retrieval-Generation Reinforced Agent for Medical Image Report Generation混合检索生成强化的代理----------医学报告生成文章链接:https://proceedings.neurips.cc/paper/2018/file/e07413354875be01a996dc560274708e-Paper.pdf此博客适合对于image captioning 和 reinforcement learning 具有一原创 2021-06-25 18:01:05 · 790 阅读 · 1 评论 -
On the Automatic Generation of Medical Imaging Reports Github源码复现
On the Automatic Generation of Medical Imaging Reports (ACL2018) Github源码复现数据集获取地址:https://github.com/nlpaueb/bio_image_caption代码地址:https://github.com/ZexinYan/Medical-Report-Generation文章目录On the Automatic Generation of Medical Imaging Reports (ACL2018)原创 2021-04-19 19:51:21 · 1488 阅读 · 27 评论