01.根据数据集ex0.txt,画出样本点。并根据线性回归画出拟合直线。
注:可以采用sklearn里面的线性回归算法也可以自己写线性回归算法
import numpy as np
import matplotlib.pyplot as plt
dataSet=np.genfromtxt('ex0.txt')
x_data=dataSet[:,:-1]
y_data=dataSet[:,-1]
def plot():
plt.scatter(x_data[:,1],y_data)
plot()
plt.show()
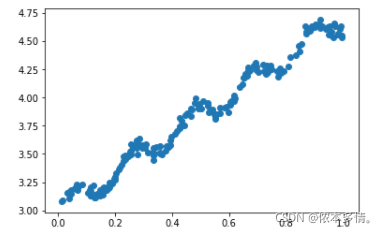
def standRegres(xArr,yArr):
xMat=np.mat(xArr)
yMat=np.mat(yArr).T
xTx=xMat.T*xMat
if np.linalg.det(xTx) == 0.0:
print("这个矩阵是奇异矩阵,矩阵不可逆")
return
ws=(xTx).I*(xMat.T*yMat)
return ws
ws=standRegres(x_data,y_data)
print(ws)
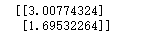
yHat=x_data*ws
plt.plot(x_data[:,1],yHat,c='r')
plot()
plt.show()
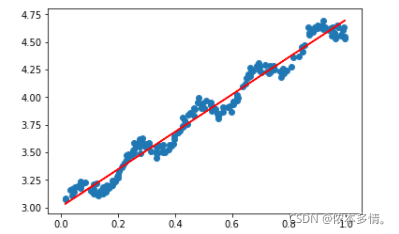
import numpy as np
import matplotlib.pyplot as plt
dataSet = np.genfromtxt('ex0.txt')
x_data=dataSet[:,:-1]
y_data=dataSet[:,-1]
def plot():
plt.scatter(x_data[:,1],y_data,c='blue',s=10)
plt.xlabel('X')
plt.ylabel('Y')
plot()
plt.show()
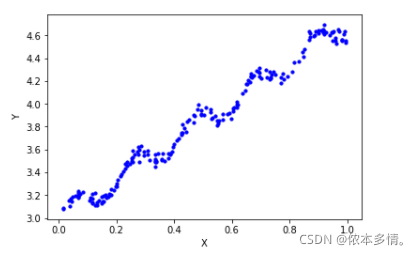
from sklearn.linear_model import LinearRegression
LR=LinearRegression()
XData=x_data[:,1,np.newaxis]
LR.fit(XData,y_data)
print(LR.coef_)
print(LR.intercept_)
print(LR.score(XData,y_data))
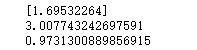
ws=np.zeros((2,1))
ws[0]=LR.intercept_
ws[1]=LR.coef_
print(ws)
yHat=np.mat(x_data)*ws
plot()
import matplotlib.pyplot as plt
plt.plot(x_data[:,1],yHat,'r')
plt.show()
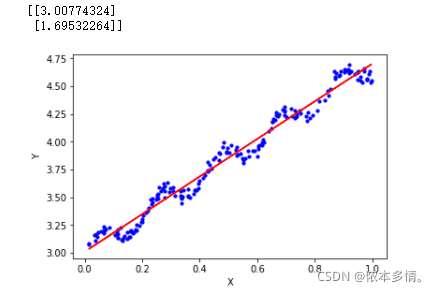
import mglearn
from sklearn.linear_model import LinearRegression
from sklearn.model_selection import train_test_split
X,y=mglearn.datasets.load_extended_boston()
X_train,X_test,y_train,y_test=train_test_split(X,y)
lr=LinearRegression().fit(X_train,y_train)
print("数据集的分值:",lr.score(X_train,y_train))
print("数据集的分值:",lr.score(X_test,y_test))
