import sys from PyQt5.QtWidgets import QApplication, QMainWindow, QLabel, QPushButton from PyQt5.QtGui import QPixmap, QImage import numpy as np import cv2 import os import time import tensorflow as tf from object_detection.utils import label_map_util from object_detection.utils import visualization_utils as vis_utils class MainWindow(QMainWindow): def __init__(self): super(MainWindow, self).__init__() # 创建一个标签用于显示图像 self.label = QLabel(self) self.label.setGeometry(10, 10, 640, 480) # 创建一个按钮用于打开摄像头 self.open_camera_button = QPushButton('Open Camera', self) self.open_camera_button.setGeometry(10, 500, 100, 30) self.open_camera_button.clicked.connect(self.on_open_camera_button_clicked) # 创建一个按钮用于保存图像 self.save_image_button = QPushButton('Save Image', self) self.save_image_button.setGeometry(120, 500, 100, 30) self.save_image_button.clicked.connect(self.on_save_image_button_clicked) # 初始化摄像头 self.cap = cv2.VideoCapture(0) # 加载模型 self.MODEL_NAME = 'ssdlite_mobilenet_v2_coco_2018_05_09' self.PATH_TO_CKPT = "ssdlite_mobilenet_v2_coco_2018_05_09/frozen_inference_graph.pb" self.PATH_TO_LABELS = os.path.join('data', 'mscoco_label_map.pbtxt') self.NUM_CLASSES = 90 self.detection_graph = tf.Graph() with self.detection_graph.as_default(): od_graph_def = tf.compat.v1.GraphDef() with open(self.PATH_TO_CKPT, 'rb') as fid: serialized_graph = fid.read() od_graph_def.ParseFromString(serialized_graph) tf.import_graph_def(od_graph_def, name='') label_map = label_map_util.load_labelmap(self.PATH_TO_LABELS) categories = label_map_util.convert_label_map_to_categories(label_map, max_num_classes=self.NUM_CLASSES, use_display_name=True) self.category_index = label_map_util.create_category_index(categories) # 开始计时 self.t_start = time.time() self.fps = 0 def on_open_camera_button_clicked(self): sess = tf.compat.v1.Session(graph=self.detection_graph) # 创建 TensorFlow 会话对象 while True: ret, frame = self.cap.read() # 进行目标检测 image_np_expanded = np.expand_dims(frame, axis=0) image_np_rgb = cv2.cvtColor(frame, cv2.COLOR_BGR2RGB) # 将帧转换为 RGB 格式 image_tensor = self.detection_graph.get_tensor_by_name('image_tensor:0') detection_boxes = self.detection_graph.get_tensor_by_name('detection_boxes:0') detection_scores = self.detection_graph.get_tensor_by_name('detection_scores:0') detection_classes = self.detection_graph.get_tensor_by_name('detection_classes:0') num_detections = self.detection_graph.get_tensor_by_name('num_detections:0') (boxes, scores, classes, num) = sess.run( [detection_boxes, detection_scores, detection_classes, num_detections], feed_dict={image_tensor: image_np_expanded}) # 在图像上绘制结果 vis_utils.visualize_boxes_and_labels_on_image_array( image_np_rgb, np.squeeze(boxes), np.squeeze(classes).astype(np.int32), np.squeeze(scores), self.category_index, use_normalized_coordinates=True, line_thickness=8) # 显示图像 self.display_image(image_np_rgb) # 更新FPS self.fps += 1 mfps = self.fps / (time.time() - self.t_start) cv2.putText(image_np_rgb, "FPS " + str(int(mfps)), (10, 10), cv2.FONT_HERSHEY_SIMPLEX, 0.5, (0, 0, 255), 2) # 检查是否按下了ESC键 k = cv2.waitKey(30) & 0xff if k == 27: # 按下ESC退出循环 break sess.close() # 关闭 TensorFlow 会话对象 def on_save_image_button_clicked(self): # 保存图像 image = self.label.pixmap().toImage() image.save('image.jpg') def display_image(self, frame): # 将OpenCV图像转换为Qt图像 h, w, ch = frame.shape bytes_per_line = ch * w qt_image = QImage(frame.data, w, h, bytes_per_line, QImage.Format_RGB888).rgbSwapped() # 在标签中显示图像 pixmap = QPixmap.fromImage(qt_image) self.label.setPixmap(pixmap) if __name__ == '__main__': app = QApplication(sys.argv) window = MainWindow() window.show() sys.exit(app.exec_())
11111
最新推荐文章于 2024-08-19 14:41:29 发布
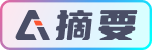