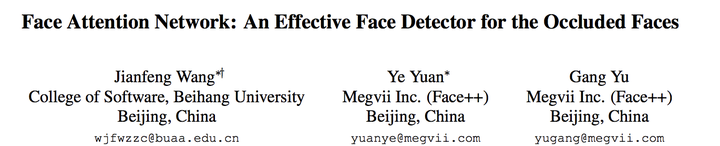
人脸检测的性能近期由于深度学习的发展大幅度提高, 然而对于人脸的遮挡问题一直是人脸检测中一个比较有挑战的问题, 这种场景多出现于佩戴口罩、太阳镜和其他人的遮挡等。
这篇文章提出了Face Attention Network (FAN), 有效提升了有遮挡人脸的召回。提出了a new anchor-level attention,增强人脸区域的特征. 结合anchor assign strategy and data augmentation techniques,在WiderFace and MAFA上达到了state-of-the-art的效果。
Base Framework
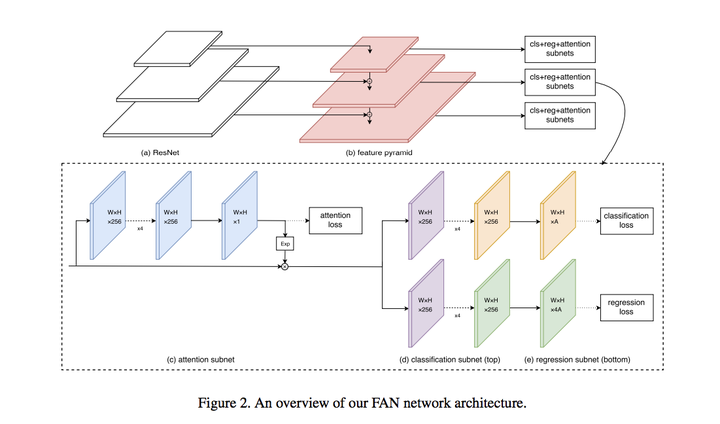
U-shape的结构能够很好地融合底层丰富的特征信息和High-level的语义信息. 基础结构借鉴RetinaNet(FPN + ResNet). RetinaNet包括两个subnet, 一个用于分类, 另一个用于回归。
分类subnet使用4个 3*3 conv layers (each with 256 filters), followed by a 3×3 convolution
layer with KA filters where K means the number of classes and A means the number of anchors per location.
For face detection K = 1 since we use sigmoid activation, and we use A = 6 in most experiments.
回归subnet terminates 4A conv filters with liner activation.
Attention Network
- Anchor Assign Strategy
- Attention Function
- Data Augmentation
Anchor Assign Strategy
在FAN中,共有5个detector layers,每一个都有特定的scale anchor. 另外, anchor的长宽比都是1和1.5,因为大多数的人脸都接近1:1.5的长宽比. 论文统计了WiderFace人脸的像素大小占比, 用于调整anchors的大小。
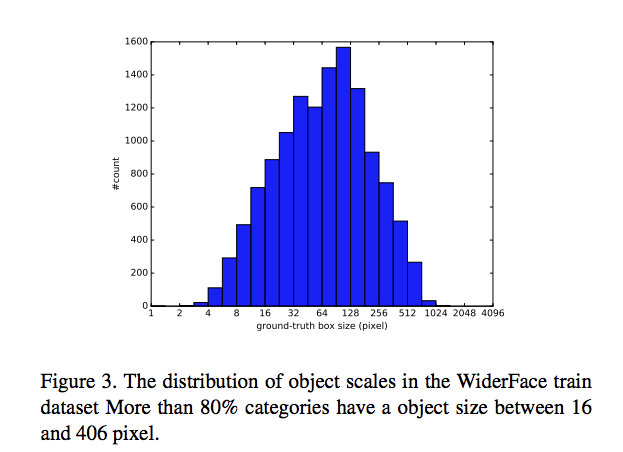
Attention Function
为了解决遮挡的问题, 提出了novel anchor-level attention.
The attention supervision information is obtained by filling the ground-truth box.
可以近似为加了一个segment的branch.
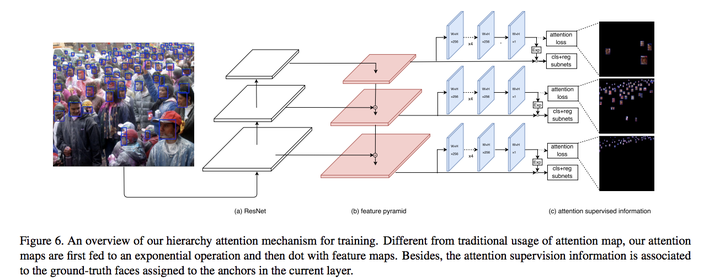
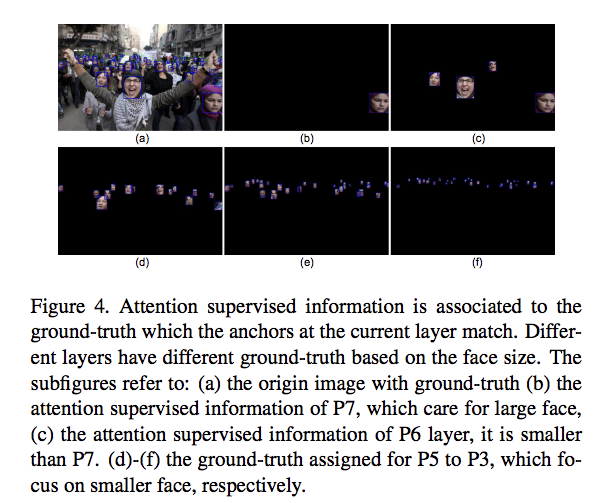
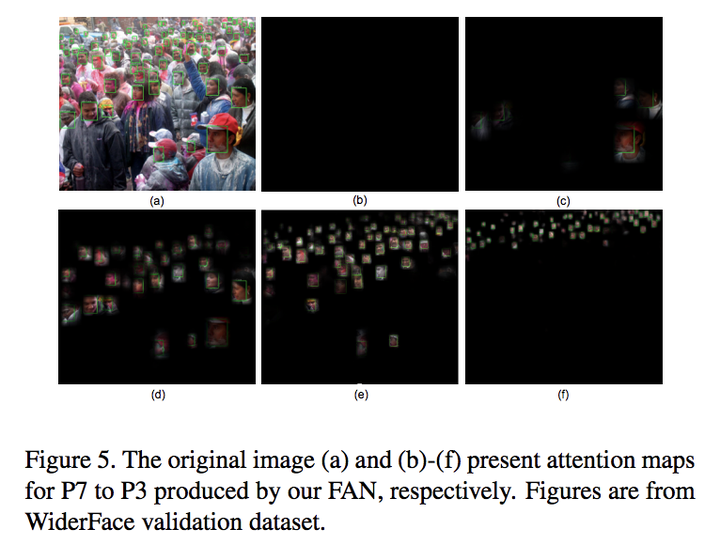
Data Augmentation
提出了随机crop策略, 来模拟训练数据中的遮挡.Besides from the random crop dataset
augmentation, we also employ augmentation from random flip and color jitter.
Loss function分为三部分:
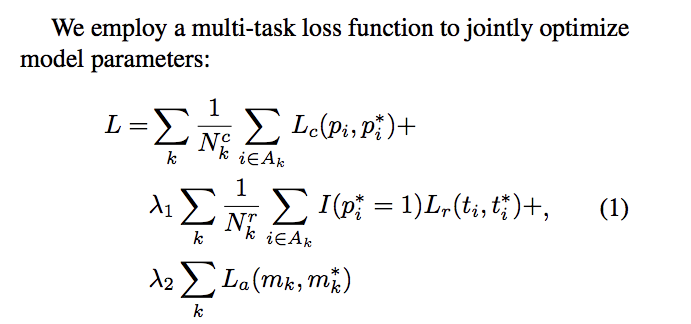
多pyramid level的分类, 回归和mask的pixel-wise sigmoid cross entropy.
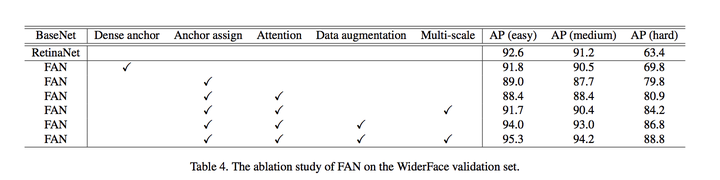
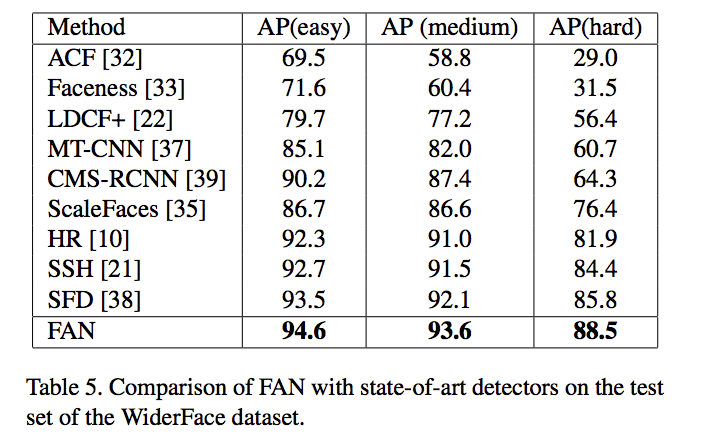