按照数据集进行划分:
关于自动分割工具,婴儿脑MR图像来自单个时间点,其中纵向数据集不可用,因此必须开发不针对纵向数据集的分割工具,目前提出了一些机器学习方法,但这些方法的效果并不令人满意。
iseg-2017:
竞赛结果一共评估了两次,第一次评估中的TOP-1为MSL_SKKU,第二次评估中降为了第二名,但由于第二次评估的时间较晚,因此已发表的论文大多与第一次评估中的冠军作对比,在以下的总结中,把第一次评估的第一名作为挑战赛的冠军。
第二次评估结果入下:
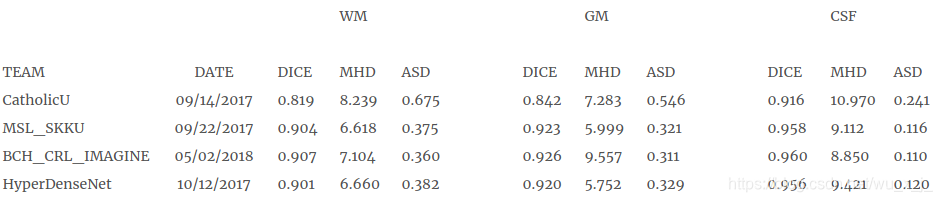
MICCAI iseg-2017挑战赛结果: 第一名为MSL_SKKU
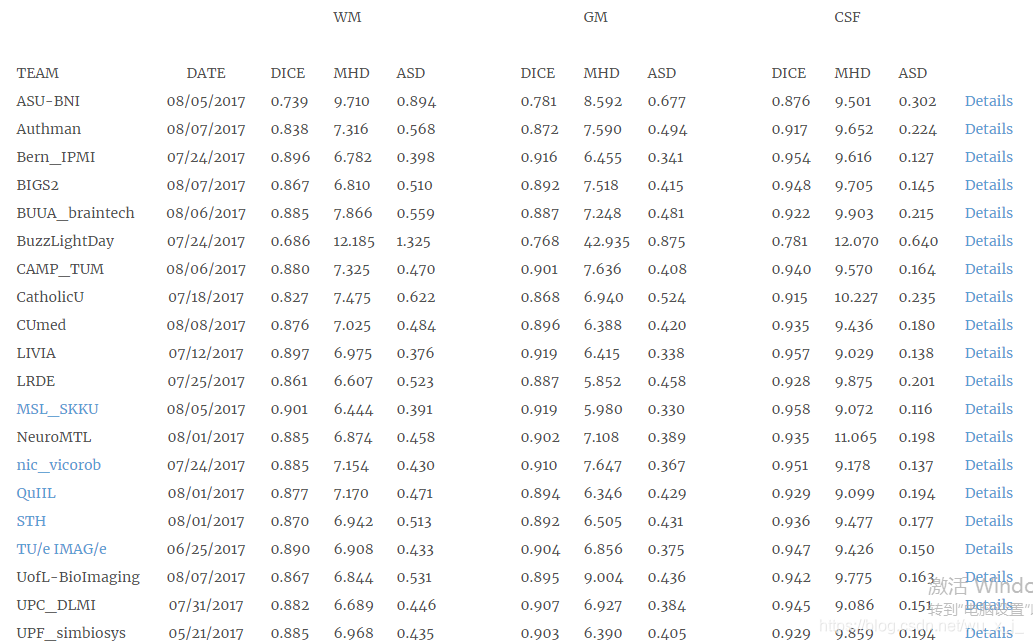
DICE结果排名如下:
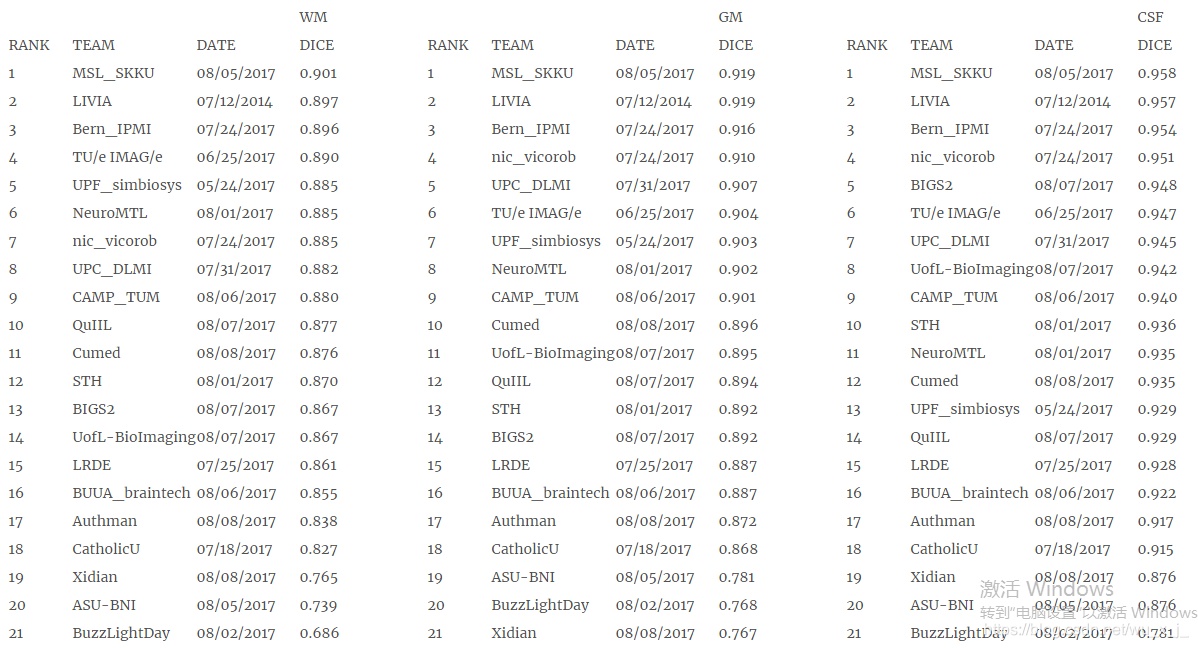
MHD排名结果如下:
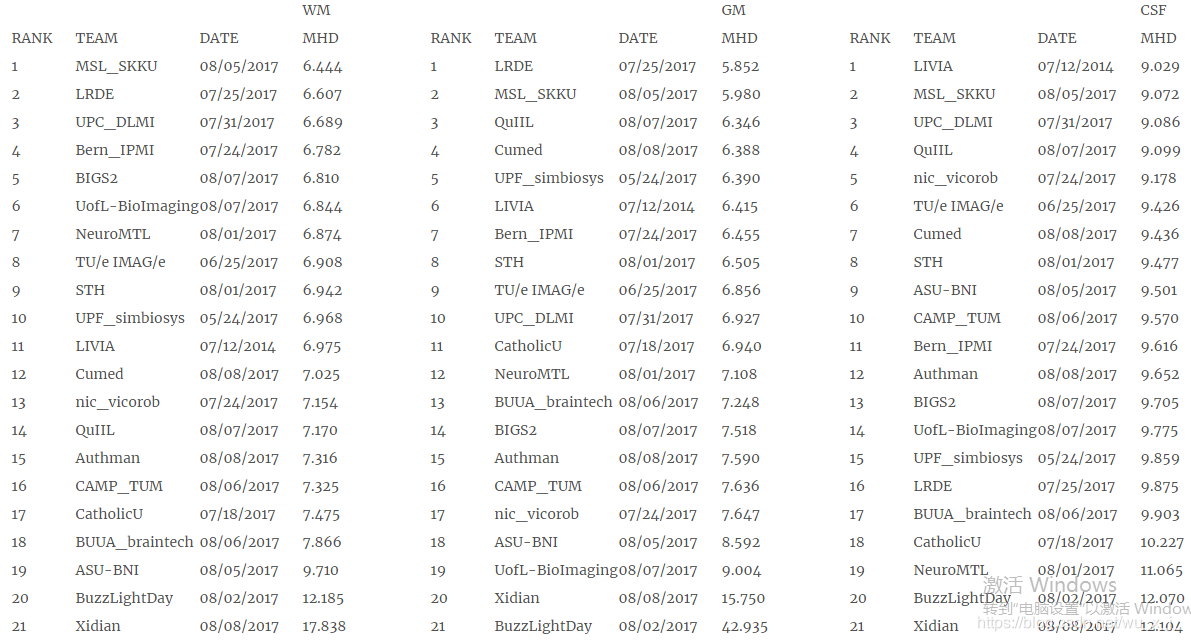
ASD排名结果如下:
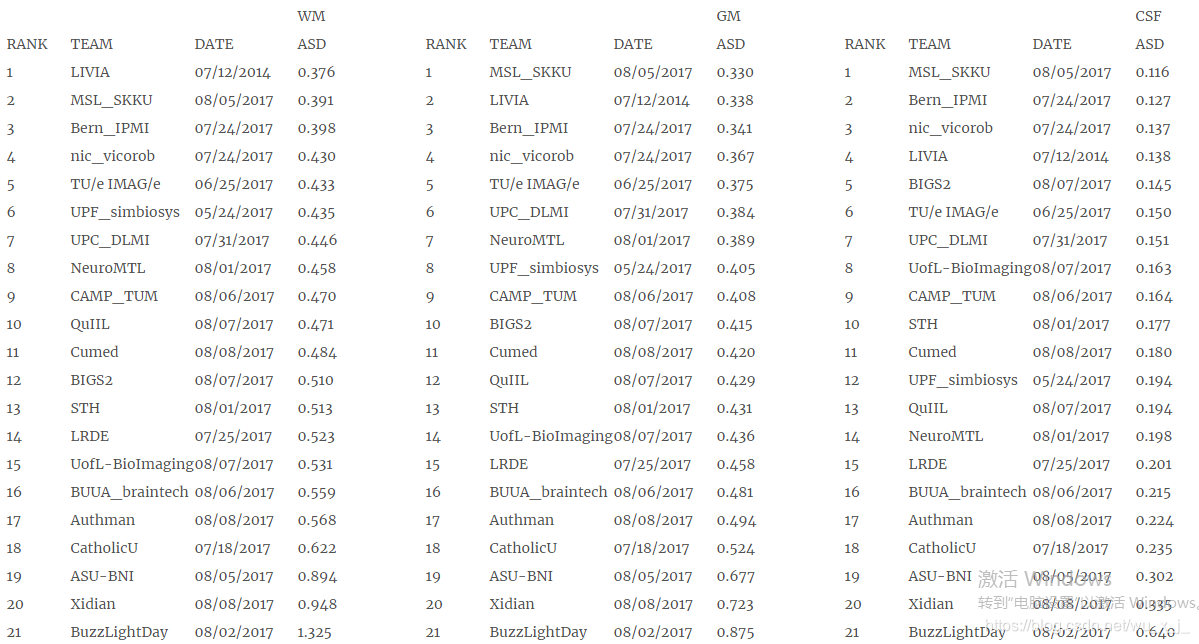
前五名的结果为:
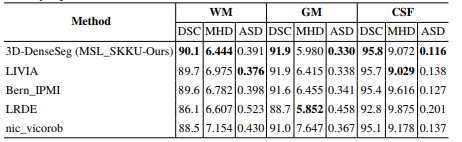
与数据集相关的论文:
Bui T D, Shin J, Moon T. 3d densely convolution networks for volumetric segmentation[J]. arXiv preprint arXiv:1709.03199, 2017.
---10引用 采用DenseNet 挑战赛中排名第一 队伍名:MSL-SKKU
取小数点后3位的情况下9项指标6项第一
Dolz J, Desrosiers C, Wang L, et al. Deep CNN ensembles and suggestive annotations for infant brain MRI segmentation[J]. arXiv preprint arXiv:1712.05319, 2017.
---6引用 采用SemiDenseNet 在某些指标中排名第一或者第二,结果如下:
Dolz J, Ayed I B, Yuan J, et al. Isointense infant brain segmentation with a hyper-dense connected convolutional neural network[C]//Biomedical Imaging (ISBI 2018), 2018 IEEE 15th International Symposium on. IEEE, 2018: 616-620.
---3引用 采用DenseNet 9个指标中6个排名前三
该文章基于另外两篇文章:
Konstantinos Kamnitsas et al., "Efficient multi-scale 3D CNN with fully connected CRF for accurate brain lesion segmentation", Medical image analysis, vol. 36, pp. 61-78, 2017.
Jose Dolz et al., "3D fully convolutional networks for subcortical segmentation in MRI: A large-scale study", NeuroImage, 2017.
Dolz J, Gopinath K, Yuan J, et al. HyperDense-Net: A hyper-densely connected CNN for multi-modal image segmentation[J]. arXiv preprint arXiv:1804.02967, 2018.
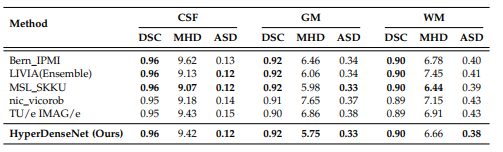
取小数点后两位的情况下,都为9项指标7项第一
对于MRBrainS2013:
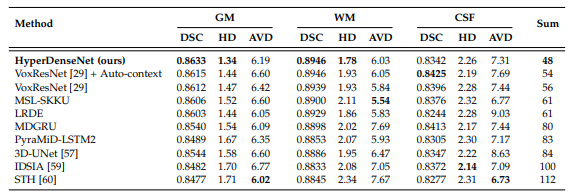
提交时间为18.02.16,提交时为第一名,目前排名第6,对比结果如下:
HyperDenseNet(top-6) | 0.8633 | 1.34 | 6.19 | 0.8946 | 1.78 | 6.03 | 0.8342 | 2.26 | 7.31 |
XMU_SmartDSP2(top-1) | 0.865 | 1.29 | 5.75 | 0.899 | 1.73 | 5.47 | 84.8 | 1.84 | 6.83 |
Dolz J, Ayed I B, Yuan J, et al. HyperDense-Net: A hyper-densely connected CNN for multi-modal image semantic segmentation[J]. arXiv preprint arXiv:1710.05956, 2017.
---未了解(上一篇的效果较好)
Fonov V, Doyle A, Evans A C, et al. NeuroMTL iSEG challenge methods[J]. bioRxiv, 2018: 278465.
---排名较靠前 队伍名:NeuroMTL
Sanroma G, Benkarim O M, Piella G, et al. Learning to combine complementary segmentation methods for fetal and 6-month infant brain MRI segmentation[J]. Computerized Medical Imaging and Graphics, 2018, 69: 52-59.
---非顶会,效果不好但拿不到数据集
Zeng G, Zheng G. Multi-stream 3D FCN with multi-scale deep supervision for multi-modality isointense infant brain MR image segmentation[C]//Biomedical Imaging (ISBI 2018), 2018 IEEE 15th International Symposium on. IEEE, 2018: 136-140.
---排名第三 队伍名:Bern_IPMI 采用FCN
Wang Z, Zou N, Shen D, et al. Global Deep Learning Methods for Multimodality Isointense Infant Brain Image Segmentation[J]. arXiv preprint arXiv:1812.04103, 2018.
-----预印本,目前最新效果最好的的论文(WM与GM为最佳性能,CSF具有可比性),提交时间为2018.12.10,未参与挑战赛排名,实验结果为:
WM | GM | CSF | |
top-1 | 0.901 | 0.919 | 0.958 |
论文 | 0.9044 | 0.9219 | 0.9557 |
使用的数据集为 iseg-2017,使用的网络为FCNN,实验结果接近第一名,实验结果如下:
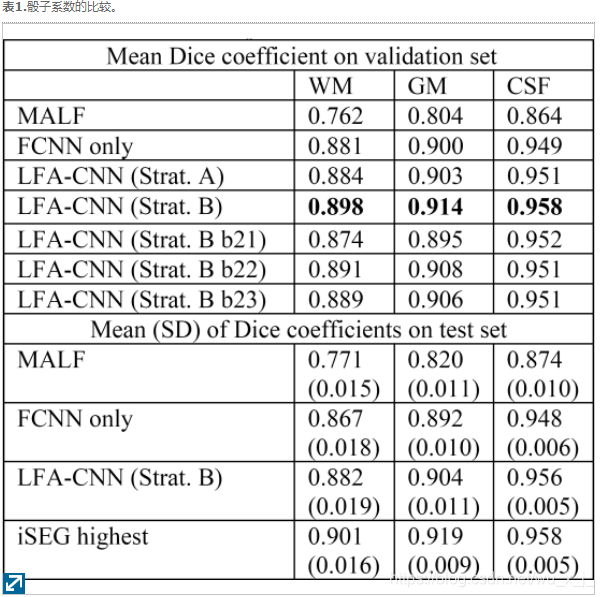
数据集来源于BCP项目,该数据集作为iseg-2017挑战赛数据集的一部分(总数,训练测试集数一样),结果比挑战赛TOP-1低2%,但参数减半。
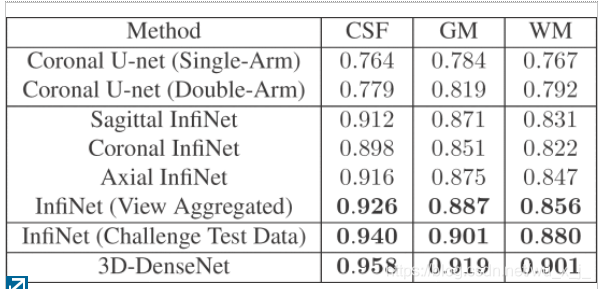
推荐的文章为:但3篇文章都为预印本
1.Bui T D, Shin J, Moon T. 3d densely convolution networks for volumetric segmentation[J]. arXiv preprint arXiv:1709.03199, 2017.
---10引用 采用DenseNet 挑战赛中排名第一 队伍名:MSL-SKKU
取小数点后3位的情况下9项指标6项第一
2.Dolz J, Gopinath K, Yuan J, et al. HyperDense-Net: A hyper-densely connected CNN for multi-modal image segmentation[J]. arXiv preprint arXiv:1804.02967, 2018.
--取小数点后2为的情况下都为9项指标7项第一
3.Wang Z, Zou N, Shen D, et al. Global Deep Learning Methods for Multimodality Isointense Infant Brain Image Segmentation[J]. arXiv preprint arXiv:1812.04103, 2018.
-----预印本,目前最新效果最好的的论文(WM与GM为最佳性能,CSF具有可比性),提交时间为2018.12.10,未参与挑战赛排名
NDAR数据集
Wang L, Li G, Shi F, et al. Volume-based analysis of 6-month-old infant brain MRI for autism biomarker identification and early diagnosis[C]//International Conference on Medical Image Computing and Computer-Assisted Intervention. Springer, Cham, 2018: 411-419.
采用U-Net,数据集来源于NDAR,包含18名受试者,分割结果很好------未找到数据集
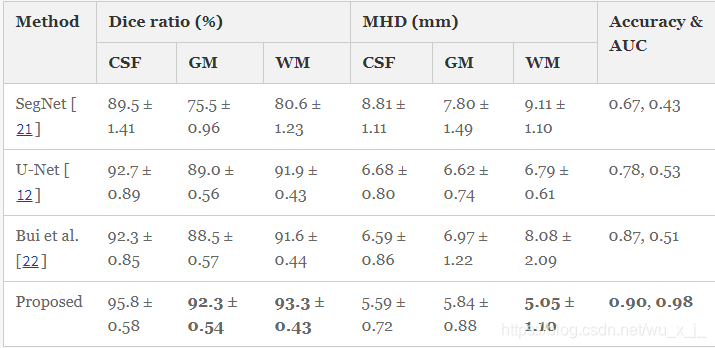
neobrains(类别较多)--相关文章较少
第一名排名结果如下(在大多数指标中排名第一)提交时间为2018.09
CoGM | UMW | CSF | Vent | CB | BS | BGT | MWM | UWM+MWM | CSF+Vent | |
DC | 0.89 | 0.94 | 0.85 | 0.90 | 0.95 | 0.86 | 0.94 | 0.53(2) | 0.93(3) | 0.85 |
MSD | 0.1 | 0.09 | 0.16 | 0.14 | 0.26 | 0.24 | 0.30 | 0.60 | 0.10 | 0.16 |
HD | 18.79 | 10.57 | 8.66 | 13.76 | 16.40 | 7.13 | 19.84 | 9.19 | 7.83 | 8.27 |
CoGM | UWM | CSF | Vent | CB | BS | BGT | MWM | UMW+MWM | SCF+Vent | |
DC | 0.79 | 0.95 | 0.89 | 0.87 | 0.91 | 0.84 | 0.89 | - | 0.95 | 0.91 |
MSD | 0.14 | 0.14 | 0.13 | 0.33 | 0.30 | 0.37 | 0.42 | - | 0.14 | 0.10 |
HD | 13.81 | 9.16 | 6.43 | 13.69 | 7.09 | 7.54 | 7.98 | - | 9.99 | 4,96 |
CoGM | UWM | CSF | Vent | CB | BS | BGT | MWM | UWM+MWM | CSF+Vent | |
DC | 0.79 | 0.91 | 0.82 | 0.82 | 0.89 | 0.68 | 0.86 | 0.04 | 0.91 | 0.83 |
MSD | 0.21 | 0.18 | 0.30 | 0.41 | 0.65 | 1.13 | 0.98 | 7.07 | 0.22 | 0.28 |
HD | 27.20 | 24.00 | 12.86 | 15.42 | 26.18 | 15.15 | 25.91 | 27.92 | 13.75 | 12.52 |
使用了该数据集的部分文章:
- Sanroma et al., In: Machine Learning in Medical Imaging (MICCAI), 2016, 27–35
- Moeskops et al., IEEE Transactions on Medical Imaging, 2016, 35(5):1252–1261
- Beare et al., Frontiers in Neuroinformatics, 2016, 10:12
- Moeskops et al., PLOS ONE, 2015, 10(7):e0131552
- Moeskops et al., NeuroImage, 2015, 118:628–641
- Cherel et al., In: SPIE Medical Imaging, 2015, 9413:941311
- Wang et al., NeuroImage, 2015, 108:160–172
- Wang et al., In: Medical Computer Vision (MICCAI), 2014, 8848:22–33
- Chita et al., In: SPIE Medical Imaging, 2013, 8669:86693
- Moeskops et al., In: SPIE Medical Imaging, 2013, 8670:867011
其他
1.Nie D, Wang L, Adeli E, et al. 3-D fully convolutional networks for multimodal isointense infant brain image segmentation[J]. IEEE Transactions on Cybernetics, 2018. 1区
2.Bernal J, Kushibar K, Cabezas M, et al. Quantitative analysis of patch-based fully convolutional neural networks for tissue segmentation on brain magnetic resonance imaging[J]. arXiv preprint arXiv:1801.06457, 2018.
3.Chen J, Zhang H, Nie D, et al. Automatic accurate infant cerebellar tissue segmentation with densely connected convolutional network[C]//International Workshop on Machine Learning in Medical Imaging. Springer, Cham, 2018: 233-240.
MICCAI挑战赛底部文章:
[1]. Li Wang, Yaozong Gao, Feng Shi, Gang Li, John H. Gilmore, Weili Lin, Dinggang Shen. LINKS: Learning-based multi-source IntegratioN frameworK for Segmentation of infant brain images, Neuroimage, 108, 160-172, 2015.
[2]. Li Wang, Feng Shi, Yaozong Gao, Gang Li, John H. Gilmore, Weili Lin, Dinggang Shen. Integration of Sparse Multi-modality Representation and Anatomical Constraint for Isointense Infant Brain MR Image Segmentation, Neuroimage, 89, 152-164, 2014.
[3]. Wenlu Zhang, Rongjian Li, Houtao Deng, Li Wang, Weili Lin, Shuiwang Ji, Dinggang Shen. Deep Convolutional Neural Networks for Multi-Modality Isointense Infant Brain Image Segmentation, Neuroimage, 108, 214–224, 2015.
综述文章:
Li G, Wang L, Yap P T, et al. Computational neuroanatomy of baby brains: A review[J]. Neuroimage, 2018.
---引用9 综述文章
A review on automatic fetal and neonatal brain MRI segmentation
---综述文章
Convolutional Neural Networks for Rapid and Simultaneous Brain Extraction and Tissue Segmentation 书籍
吃鸡加我:360258904