# -*- coding: utf-8 -*-
"""
Created on Sun Jan 14 14:38:00 2018
@author: ainurrofiq
"""
import os
import numpy as np #numpy库数组和矩阵的科学计算库,包含线性代数、傅里叶和随机数的生成等。
import scipy as sp #scipy库可实现最小二乘拟合
from obspy import read #从obspy中导入读取
import scipy.signal as sg
import matplotlib.pyplot as plt
import collections
import scipy.special
from datetime import datetime
class Clustering(object): #创建Clustering聚类归总类,该定义的类会继承于objet类。
def __init__ (self, array, deviation=5):#定义函数;_init为构造方法,该方法在类实例化会自动调用;deviaion误差,array数组
self.array = array
self.deviation = deviation #参数通过__init__()传递到类。
def __call__ (self): #self代表类 的实例,并非类,__call__为函数调用
ValList, IdxList, Cluster, ClusIdx, kepake=[], [], [], [], []
for i in range(len(self.array)):
if self.array[i] not in kepake:
Cluster=[self.array[i]]
ClusIdx=[i]
for j in range(len(self.array)):
if i<j and self.array[j] not in kepake:
if len(Cluster)==1:
if np.std([self.array[i], self.array[j]],0)<= np.abs((self.deviation/100.0)*np.mean([self.array[i], self.array[j]],0)):
#np.std返回数组元素的标准偏差,np.mean返回数组元素的平均值。
Cluster.append(self.array[j])
ClusIdx.append(j)
else:
if np.abs(self.array[j]-np.mean(Cluster,0))<= np.abs((self.deviation/100.0)*np.mean(Cluster,0)):
Cluster.append(self.array[j])
ClusIdx.append(j)
if len(Cluster)>=1:
kepake=Cluster+kepake
ValList.append(np.array(Cluster))
IdxList.append(np.array(ClusIdx))
ClustDict={}
for j in range(len(ValList)):
ClustDict[np.mean(ValList[j])]=[IdxList[j],ValList[j]]
ClustDict=collections.OrderedDict(sorted(ClustDict.items()))
for i in range(len(ClustDict.keys())):
IdxList[i]=ClustDict[ClustDict.keys()[i]][0]
ValList[i]=ClustDict[ClustDict.keys()[i]][1]
return IdxList, ValList
class xcross0(object):
def __init__ (self, xdata, ydata): #_init为构造函数:初始化对象,将需要的状态信息存起来;
self.xdata = xdata
self.ydata = ydata
def __call__ (self):
xnol=[]
for p in range(len(self.ydata)-1):
if (self.ydata[p]<0 and self.ydata[p+1]>0) or (self.ydata[p]>0 and self.ydata[p+1]<0) :
for k in range(len(np.argwhere(self.ydata==self.ydata[p]))): #np.argwhere 为多条件交集或并集
for l in range(len(np.argwhere(self.ydata==self.ydata[p+1]))):
if np.argwhere(self.ydata==self.ydata[p+1])[l][0]-np.argwhere(self.ydata==self.ydata[p])[k][0]==1:
idx1=np.argwhere(self.ydata==self.ydata[p])[k][0]
idx2=np.argwhere(self.ydata==self.ydata[p+1])[l][0]
x1=self.xdata[idx1]
x2=self.xdata[idx2]
y1=self.ydata[idx1]
y2=self.ydata[idx2]
xnol.append((((x2-x1)/(y2-y1))*(-y1))+x1)
return np.array(xnol)
class SPAC(object):
def __init__(self, fout=[8,25], unique='', akhir='.sg2', filtSignal=None, window=3600, CoordinateFile='coordinate10.txt', diststd=5):
"""
Atributes :
unique = part of input name (string) #输入名称部分字符串
akhir = format file(string) #格式文件字符
fout = output frequency in output file ([fmin, fmax])
format = input's format (string)
filtSignal = apply bandpass filter to all signal
None - No filter appy
[freqmin, freqmax] - filter from freqmin to freqmax
window = length window in seconds
CoordinateFile = file coordinate (name, x, y, z)(string)
"""
self.unique = unique
self.akhir = akhir
self.window = window
self.CoordinateFile = CoordinateFile
self.fout = fout
self.diststd = diststd
self.filtSignal = filtSignal
def __call__(self):
start_time = datetime.now()
#=============== Zero Order Bessel Function of the First Kind ==================第一类零阶贝塞尔函数
xo = np.linspace(0,50,500)
Jo = scipy.special.jv(0,xo)
x0 = xcross0(xo, Jo)()
x0 = x0[0:12]
if not os.path.exists(os.getcwd()+'\\TIME AVERAGE'):
os.makedirs(os.getcwd()+'\\TIME AVERAGE')
if not os.path.exists(os.getcwd()+'\\SPATIAL_AVERAGE'):
os.makedirs(os.getcwd()+'\\SPATIAL_AVERAGE')
#=============== List of Input Record Files ==================输入记录文件列表
RecordFiles=[a for a in os.listdir(os.getcwd()) if self.unique in a and a.endswith(self.akhir)]
#for 。。。in语句遍历列表集合元组,if a in b and c:判断a是否在b与c中
#os.listdir()方法用于返回指定文件夹包含的文件或文件夹的名字的列表;os.getcwd为获得当前的路径,该函数不需要传递参数,它返回当前的目录。
#endswith()函数:判断字符串是否以指定字符或字符串结尾
#=============== Identify Station Coordinate ==================识别站坐标
floc=open((self.CoordinateFile),'r') #读取坐标文件
fline=floc.readlines()
LocDict={}
for i in range(len(fline)):
fline[i]=fline[i].split(' ')
LocDict[fline[i][0]]=[float(fline[i][1]),float(fline[i][2]),float(fline[i][3])]
#=============== Read Input Files ==================
st=read(RecordFiles[0])
for i in range(len(RecordFiles)-1):
st+=read(RecordFiles[i+1])
st.sort(keys=['station'])
if self.filtSignal != None:
st.filter('bandpass', freqmin=self.filtSignal[0], freqmax=self.filtSignal[1])
#=============== Apply Coordinate to Station ==================将坐标应用到台站
for i in range(len(st)):
if LocDict.has_key(st[i].stats.station)==True:
st[i].stats.location=LocDict[st[i].stats.station]
#========================= Logfile ===========================读文件
logfile=''
logfile=logfile+'=======================================\n'
logfile=logfile+'Start Time = '+str(start_time)+'\n'
logfile=logfile+'=======================================\n'
logfile=logfile+'=======================================\n'
logfile=logfile+'Length Window = '+'{:>8.2f}'.format(self.window)+' seconds\n'
logfile=logfile+('Output Frequency = '+'{:5.2f}'.format(self.fout[0])+
' Hz to '+'{:5.2f}'.format(self.fout[1])+' Hz\n')
if self.filtSignal != None:
logfile=logfile+('Filter ( Bandpass ) = '+'{:5.2f}'.format(self.filtSignal[0])+
' Hz to '+'{:5.2f}'.format(self.filtSignal[1])+' Hz\n')
else:
logfile=logfile+'Filter ( Bandpass ) = No Filter\n'
logfile=logfile+'\n----------- Station List -----------\n'
logfile=logfile+'Name \t Easting (m) \t Northing (m) \t Elevation(m)\n'
for i in range(len(st)):
logfile=(logfile+
'{:8.6}'.format(str(st[i].stats.station))+
'{:12.4f}'.format(st[i].stats.location[0])+
'{:17.4f}'.format(st[i].stats.location[1])+
'{:14.4f}'.format(st[i].stats.location[2])+'\n')
logfile=logfile+'--------------------------------------\n\n'
with open(os.getcwd()+'\\SPATIAL_AVERAGE\\LOGFILE.txt','w') as f:
f.write(logfile)
f.close()
#=========== Smoothing Constant ( Hanning Window ) =============
smooth=(np.hanning(int(0.0005*self.window*st[0].stats.sampling_rate))/
sum(np.hanning(int(0.0005*self.window*st[0].stats.sampling_rate))))
#=== Iteration to make every station as a center of irregular array ===
for sig in range(len(st)):
#========================= Logfile ===========================
log=open(os.getcwd()+'\\SPATIAL_AVERAGE\\LOGFILE.txt','a')
log.write('{:8.6}'.format(str(st[sig].stats.station))+'( Center of Array )'+'\n')
# Amplitude and sampling frequency of centre in time domain 时域中心的振幅和采样率
StaPusat=st[sig]
Fs=StaPusat.stats.sampling_rate
# ================= FFT Parameter ===================快速傅里叶变换参数
wd=self.window*StaPusat.stats.sampling_rate
nft=self.window*StaPusat.stats.sampling_rate
# ================= Do FFT at center ================ 在中心做快速傅里叶变换
freq_pusat, time_pusat, Sxx_pusat_ = sg.spectrogram(StaPusat.data,
nperseg=wd,
noverlap=0,
nfft=nft,
fs=Fs,
scaling='spectrum',
mode='complex')
# ================= Find Data in range output frequency =================在输出频率范围内查找数据
idx = np.where((freq_pusat >= self.fout[0]) & (freq_pusat <= self.fout[1]))
freq_pusat=freq_pusat[idx]
Sxx_pusat_=Sxx_pusat_[idx]
# Make directory for 'TIME AVERAGE' and 'SPATIAL AVERAGE' output 为时间平均和空间平均的输出创建目录
if not os.path.exists(os.getcwd()+'\\TIME AVERAGE\\'+StaPusat.stats.station):
os.makedirs(os.getcwd()+'\\TIME AVERAGE\\'+StaPusat.stats.station)
if not os.path.exists(os.getcwd()+'\\SPATIAL_AVERAGE\\'+StaPusat.stats.station):
os.makedirs(os.getcwd()+'\\SPATIAL_AVERAGE\\'+StaPusat.stats.station)
# ================= List of non Center Station =================非中心站名单
irover=[x for x in range(len(st)) if x!=sig]
autocorr=np.zeros((len(Sxx_pusat_[:,0]),len(irover)), dtype=np.complex)
xnolList=[]
distList=[]
ListDist=[]
# ================= Iteration of non Center Station =================非中心站的迭代
for c in range(len(irover)):
# ================= Non center Station Index =================
Atr=st[irover[c]]
# ================= Do FFT at non center =================
freq_rover, time_rover, Sxx_rover_ = sg.spectrogram(Atr.data ,
nperseg=wd,
noverlap=0,
nfft=nft,
fs=Fs,
scaling='spectrum',
mode='complex')
# ======== Find Data in range output frequency ===========
Sxx_rover_=Sxx_rover_[idx]
# ================= Measure AutoCorrelation Ratio ================计算自相关系数
rat_autocorr=np.zeros((len(Sxx_pusat_[:,0]),len(Sxx_pusat_[0,:])), dtype=np.complex)
for nwd in range(len(Sxx_pusat_[0,:])):
Abs_Sxx_pusat=(abs(Sxx_pusat_[:,nwd]))
Smooth_Abs_Sxx_pusat=sg.convolve(Abs_Sxx_pusat,smooth,mode='same')
Abs_Sxx_rover=(abs(Sxx_rover_[:,nwd]))
Smooth_Abs_Sxx_rover=sg.convolve(Abs_Sxx_rover,smooth,mode='same')
rat_autocorr[:,nwd]=((Sxx_pusat_[:,nwd]*np.conj(Sxx_rover_[:,nwd]))/
(Smooth_Abs_Sxx_pusat*Smooth_Abs_Sxx_rover))
time_autocorr=np.mean(rat_autocorr,axis=1)
autocorr[:,c]=time_autocorr
# ======== Save result every pair AutoCorrelation Ratio ========
with open(os.getcwd()+'\\TIME AVERAGE\\'+StaPusat.stats.station+'\\SPAC_'+
StaPusat.stats.station+'-'+Atr.stats.station+'.txt','w') as f:
datas=np.array([freq_pusat,autocorr[:,c].real])
np.savetxt(f,datas.T,fmt='%8.5f')
f.close()
# ======== measure distance every possible station pair ========
distance=((Atr.stats.location[0]-StaPusat.stats.location[0])**2+
(Atr.stats.location[1]-StaPusat.stats.location[1])**2+
(Atr.stats.location[2]-StaPusat.stats.location[2])**2)**0.5
ListDist.append(distance)
# ======== CLusterting pair based on its distance ======== 基于距离的聚类对
ListDist=np.array(ListDist)
[IdxList,AllList]=Clustering(ListDist, self.diststd)() #该处调用了聚类归总类
StaList, IdxListNew = [], []
for k in range(len(IdxList)):
StaList_, IdxListNew_ = '', []
for l in range(len(IdxList[k])):
if IdxList[k][l]>= sig :
IdxListNew_.append(IdxList[k][l]+1)
if l==0:
StaList_+=str(st[IdxList[k][l]+1].stats.station)
else:
StaList_+=' + '+str(st[IdxList[k][l]+1].stats.station)
else:
IdxListNew_.append(IdxList[k][l])
if l==0:
StaList_+=str(st[IdxList[k][l]].stats.station)
else:
StaList_+=' + '+str(st[IdxList[k][l]].stats.station)
StaList.append(StaList_)
IdxListNew.append(np.array(IdxListNew_))
dispersion, labelplot = [], []
# ========================= smoothing ==========================
for j in range(len(AllList)):
time_mean=np.mean(autocorr[:,IdxList[j]].real,axis=1)
#========================= Logfile ===========================
logfile=('\t'+StaList[j]+'\n\taverage radius '+'{:10.4f}'.format(np.mean(AllList[j]))+
' Standart Deviation '+'{:6.2f}'.format(np.std(AllList[j]))+
' ( '+'{:4.2f}'.format(100*(np.std(AllList[j])/np.mean(AllList[j])))+
' % from average radius )\n')
log.write(logfile)
# ==========================================================
# ============== smoothing Correlation Curve ===============
# NEED TO BE FIXED!!!!!
# MUST KNOW RELATION BETWEEN FFT LENGTH WINDOW, RADII,
# FREQUENCY (FIRST CROSS) AND SMOOTHING CONSTANT
#
# NOT A GOOD CHOICE FOR LONG DISTANCE PAIR
# SO, MANUAL !!!!
# ==========================================================
delta=[]
if (np.mean(AllList[j]))<=1000:
wind=np.arange(2001, 10001, 100)
elif (np.mean(AllList[j]))>1000 and (np.mean(AllList[j]))<=4000 :
wind=np.arange(401, 2001, 50)
elif (np.mean(AllList[j]))>4000 and (np.mean(AllList[j]))<=8000 :
wind=np.arange(201, 801, 20)
elif (np.mean(AllList[j]))>8000 and (np.mean(AllList[j]))<=16000 :
wind=np.arange(101, 401, 10)
elif (np.mean(AllList[j]))>16000:
wind=np.arange(5, 201, 2)
for i in range(len(wind)):
ydata2 = sp.signal.savgol_filter(time_mean,wind[i],3)
delta.append(np.mean(abs(time_mean-ydata2))**2)
gradient=np.gradient(delta)
if np.argwhere(gradient<0).size!=0:
locgradient=np.argwhere(gradient<0)
window=wind[min(locgradient)[0]]
if np.argwhere(gradient<0).size==0:
locgradient=np.argmin(gradient)
window=wind[locgradient]
ydata = sp.signal.savgol_filter(time_mean,window,3)
#---------------------------------------------------------------------
#-----------------------cross at y-axes = 0 --------------------------
#---------------------------------------------------------------------
xnol=xcross0(freq_pusat, ydata)()
if np.gradient(ydata)[max(np.argwhere(freq_pusat<=xnol[0]))]>0:
xnol=xnol[1:len(xnol)]
else:
xnol=xnol[0:len(xnol)]
#--------------------------------------------------------------------------
#----------------------------Dispersion Curve----------------------
#--------------------------------------------------------------------------
vphase = []
if len(xnol)<len(x0):
for dc in range(len(xnol)):
if ((2*(22/7)*np.mean(AllList[j])*xnol[dc])/x0[dc])<4000:
vphase.append((2*(22/7)*np.mean(AllList[j])*xnol[dc])/x0[dc])
elif len(xnol)>len(x0):
for dc in range(len(x0)):
if ((2*(22/7)*np.mean(AllList[j])*xnol[dc])/x0[dc])<4000:
vphase.append((2*(22/7)*np.mean(AllList[j])*xnol[dc])/x0[dc])
vphase=np.array(vphase)
dispersion.append([xnol[0:len(vphase)],vphase])
labelplot.append('{:.2f}'.format(np.mean(AllList[j])))
#print len(vphase),len(xnol[0:len(vphase)])
#--------------------------------------------------------------------------
#--Clustering for crossing y=0 (Avoid double Crossing / x-value to close)--
#--------------------------------------------------------------------------
[IdxList1,AllList1]=Clustering(xnol, 0.05)()
xnol_rev=[]
for s in range((len(AllList1))):
xnol_rev.append(np.mean(AllList1[s]))
xnol_rev=np.array(xnol_rev)
#---------------------------------------------------------------------
# -------------------------Plot Figure--------------------------------
#---------------------------------------------------------------------
fig1=plt.figure()
plt.plot(freq_pusat,time_mean)
plt.plot(freq_pusat, ydata,'r',lw=3)
plt.xlabel('frequency [Hz]')
plt.ylabel('AutoCorrelation')
plt.ylim(-1,1)
plt.title(StaPusat.stats.station+' - '+str(StaList[j])+' - '+
str(np.mean(AllList[j])))
plt.grid()
if len(xnol_rev)>=25:
plt.xlim(0,xnol_rev[24])
fig2=plt.figure()
plt.plot(freq_pusat, ydata,'r')
plt.plot(xnol_rev,np.linspace(0,0,len(xnol_rev)),'bo',ms=4)
plt.xlabel('frequency [Hz]')
plt.ylabel('AutoCorrelation')
plt.ylim(-0.5,0.5)
plt.title(StaPusat.stats.station+' - '+str(StaList[j])+' - '+
str(np.mean(AllList[j])))
plt.grid()
if len(xnol_rev)>=25:
plt.xlim(0,xnol_rev[24])
#-------------------------------------------------------------
#----------------------- Save Figure -------------------------
#-------------------------------------------------------------
fig1.savefig(os.getcwd()+'\\SPATIAL_AVERAGE\\'+StaPusat.stats.station+
'\\'+StaPusat.stats.station+'_raw - '+str(np.mean(AllList[j]))+
'.png',dpi=fig1.dpi)
fig2.savefig(os.getcwd()+'\\SPATIAL_AVERAGE\\'+StaPusat.stats.station+
'\\'+StaPusat.stats.station+' - '+str(np.mean(AllList[j]))+
'.png',dpi=fig1.dpi)
plt.close(fig1)
plt.close(fig2)
xnolList.append(xnol_rev)
distList.append(np.mean(AllList[j]))
#---------------------------------------------------------------------
# ------- Save frequency, Spatial average (raw and smoothing) --------
#---------------------------------------------------------------------
with open(os.getcwd()+'\\SPATIAL_AVERAGE\\'+StaPusat.stats.station+
'\\SPAC_'+str(round(np.mean(AllList[j]/1000),2))+'_RADIUS_'+
str(len(IdxList[j]))+'-STATION(s).txt','w') as f:
datas=np.array([freq_pusat,time_mean, ydata])
np.savetxt(f,datas.T,fmt='%8.5f')
f.close()
#--------------------------------------------------------------
#-------------- Dispersion Curve for all pairs ----------------
#--------------------------------------------------------------
freqCurve, dispCurve=[], []
for pi in range(len(dispersion)):
for qi in range(len(dispersion[pi][0])):
freqCurve.append(dispersion[pi][0][qi])
dispCurve.append(dispersion[pi][1][qi])
IdxFreq=np.argsort(freqCurve)
dispCurveSort=[]
freqCurveSort=[]
for si in IdxFreq:
freqCurveSort.append(freqCurve[si])
dispCurveSort.append(dispCurve[si])
fig4=plt.figure()
plt.semilogx(freqCurveSort,dispCurveSort,'k')
plt.semilogx(freqCurveSort,dispCurveSort,'ro')
plt.ylim(ymin=0)
plt.title('Dispersion ('+StaPusat.stats.station+')')
plt.xlabel('Frequency (Hz)')
plt.ylabel('Phase Velocity (m/s)')
plt.grid()
fig4.savefig(os.getcwd()+'\\SPATIAL_AVERAGE\\'+StaPusat.stats.station+
'\\CurvDisp_'+StaPusat.stats.station+'.png',
bbox_inches="tight",dpi=fig4.dpi)
fig3=plt.figure()
for di in range(len(dispersion)):
plt.semilogx(dispersion[di][0],dispersion[di][1],'--',color='gray')
plt.semilogx(dispersion[di][0],dispersion[di][1],'o', label='Ring = '+labelplot[di])
plt.hold(True)
plt.legend(loc='upper left', prop={'size':10}, bbox_to_anchor=(1,1))
plt.ylim(ymin=0)
plt.title('Dispersion ('+StaPusat.stats.station+')')
plt.xlabel('Frequency (Hz)')
plt.ylabel('Phase Velocity (m/s)')
plt.grid()
fig3.savefig(os.getcwd()+'\\SPATIAL_AVERAGE\\'+StaPusat.stats.station+
'\\Dispersion_'+StaPusat.stats.station+'.png',
bbox_inches="tight",dpi=fig3.dpi)
plt.close(fig3)
plt.close(fig4)
#---------------------------------------------------------------------
# -------------------- Save Location Y=0 -----------------------------
#---------------------------------------------------------------------
nxnol=25
xnolall=np.empty((len(xnolList),nxnol+1),dtype=float)
xnolall[:]=np.nan
for i in range(len(xnolList)+1):
if i==0:
xnolall[:,i]=np.array(distList)
else:
if len(xnolList[i-1])>=nxnol:
xnolall[i-1,1:nxnol+1]=xnolList[i-1][0:nxnol]
else:
xnolall[i-1,1:len(xnolList[i-1])+1]=xnolList[i-1]
with open(os.getcwd()+'\\SPATIAL_AVERAGE\\'+StaPusat.stats.station+'\\Xnol.txt','w') as f:
np.savetxt(f,xnolall.T,fmt='%12.5f')
f.close()
#========================= Logfile ===========================
end_time = datetime.now()
logfile='------------------------------------------------\n'
logfile=logfile+'End Time = '+str(end_time)+'\n\n'
logfile=logfile+'Duration = '+str(end_time-start_time) #duration:持续时间
log.write(logfile)
log.close()
return
Python求助攻,哥哥姐姐们帮忙一下,网上下载的这个程序需要什么数据参数读入吗?帮忙写个主程序调用一下SPAC出结果,必有重谢
最新推荐文章于 2024-09-22 17:12:26 发布
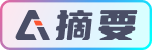