你好,我是郭震
今天我们研究「AI大模型第三篇」:词维度预测,很多读者听过词嵌入,这篇文章解答下面问题:
词嵌入是什么意思?
怎么做到的?原理是什么?
从零实现一个专属你数据集的词嵌入
我们完整从零走一遍,根基的东西要理解透,这样才能发明出更好的东西。
1 skip-gram模型
Skip-gram模型是一种广泛使用的词嵌入(Word Embedding)方法,由Mikolov等人在2013年提出。它是Word2Vec模型的一种形式,主要用于从大量文本中学习词汇的高质量向量表示。
Skip-gram模型的目标是通过给定的目标词来预测其上下文中的词汇,从而在这个过程中学习词的嵌入表示。
因此,Skip-gram模型通过给定词预测上下文,来最终学习到每个单词的词嵌入表示。
★有些同学可能不理解,通过给定词预测上下文,是什么意思?为什么要这么做?
因为,某个单词的上下文是有规律可寻的,比如 am
单词的上下文,一般就是 I
,teacher
,或tired
,am
后面一定不会出现:eat
或walk
,因为两个动词不可能出现在一起。
正是利用这个规律,也就是已知条件,我们学习到另一些好的特性,比如在这里,我们学习到每一个单词的数学向量表示,计算机只认得数字,它不认识我们认识的单词。
2 求解问题
假设我们有一个简单的句子:"the quick brown fox jumps over the lazy dog",并且我们选择Skip-gram模型进行词向量的训练。
我们可以挑选“fox”作为输入词,上下文窗口大小为2:
输入:"fox"
预测的上下文:"quick"、"brown"、"jumps"、"over"
3 求解思路:
1 对“fox”进行独热编码。
2 使用Word2Vec模型预测“fox”的上下文词。
3 通过调整模型权重来最小化预测误差,使得模型可以更准确地预测到“fox”的正确上下文。
4 训练模型
用到的包
import torch
import torch.nn as nn
import torch.optim as optim
from torch.utils.data import DataLoader, Dataset
词汇表和单词索引
sentence = "the quick brown fox jumps over the lazy dog"
words = sentence.split()
word_to_ix = {word: i for i, word in enumerate(set(words))}
创建你的数据集
context_size = 2
data = []
for i in range(context_size, len(words) - context_size):
target = words[i]
context = [words[i - j - 1] for j in range(context_size)] + [words[i + j + 1] for j in range(context_size)]
data.append((target, context))
class SkipGramDataset(Dataset):
def __init__(self, data, word_to_ix):
self.data = data
self.word_to_ix = word_to_ix
def __len__(self):
return len(self.data)
def __getitem__(self, idx):
target, context = self.data[idx]
target_idx = self.word_to_ix[target]
context_idx = torch.tensor([self.word_to_ix[w] for w in context], dtype=torch.long)
return target_idx, context_idx
创建模型SkipGramModel
class SkipGramModel(nn.Module):
def __init__(self, vocab_size, embedding_dim):
super(SkipGramModel, self).__init__()
self.embeddings = nn.Embedding(vocab_size, embedding_dim)
self.predictions = nn.Linear(embedding_dim, vocab_size)
def forward(self, input_words):
embeds = self.embeddings(input_words)
scores = self.predictions(embeds)
log_probs = torch.log_softmax(scores, dim=1)
return log_probs
训练模型SkipGramModel
# 初始化模型和优化器
embedding_dim = 10
vocab_size = len(word_to_ix)
model = SkipGramModel(vocab_size, embedding_dim)
loss_function = nn.NLLLoss()
optimizer = optim.SGD(model.parameters(), lr=0.01)
# 数据加载器
dataset = SkipGramDataset(data, word_to_ix)
dataloader = DataLoader(dataset, batch_size=1, shuffle=True)
for epoch in range(50):
total_loss = 0
for target_idx, context_idx in dataloader:
model.zero_grad()
# 得到模型的预测对数概率输出
log_probs = model(target_idx)
# 循环计算每个上下文词的损失并累加
loss = 0
for context_word_idx in context_idx.view(-1):
loss += loss_function(log_probs, context_word_idx.unsqueeze(0))
# 反向传播和优化
loss.backward()
optimizer.step()
total_loss += loss.item()
print(f'Epoch {epoch + 1}, Loss: {total_loss}')
5 使用模型预测
使用模型SkipGramModel
def predict_context(model, input_word, word_to_ix, ix_to_word, top_n=3):
# Check if the word is in the dictionary
if input_word not in word_to_ix:
return f"Word '{input_word}' not in vocabulary."
# Prepare the model for evaluation
model.eval()
# Convert word to index and wrap in tensor
word_idx = torch.tensor([word_to_ix[input_word]], dtype=torch.long)
# Forward pass to get log probabilities
with torch.no_grad():
log_probs = model(word_idx)
# Convert log probabilities to actual probabilities
probs = torch.exp(log_probs).squeeze(0) # Remove batch dimension
# Get the indices of the top N probabilities
top_indices = torch.topk(probs, top_n, dim=0)[1].tolist()
# Convert indices back to words
top_words = [ix_to_word[idx] for idx in top_indices]
return top_words
# Create a reverse dictionary to map indices back to words
ix_to_word = {index: word for word, index in word_to_ix.items()}
# Example usage: predict context words for 'fox'
predicted_context_words = predict_context(model, 'fox', word_to_ix, ix_to_word, top_n=4)
print(f"Context words for 'fox': {predicted_context_words}")
6 结果分析
以上代码完整可运行,我们打印预测结果,看到预测fox
的上下文是准确的:
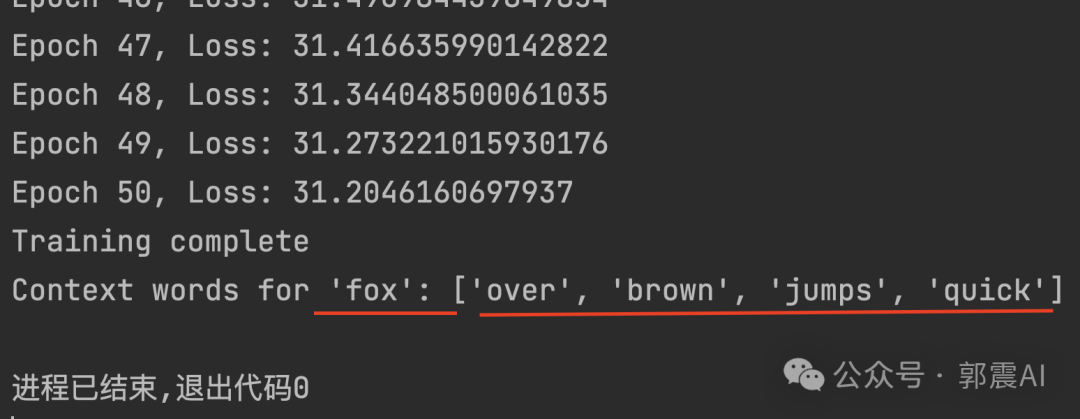
最后打印我们得到的fox
单词的嵌入词向量:
# 确保'fox'在词汇表中
if 'fox' in word_to_ix:
# 获取'fox'的索引
fox_index = word_to_ix['fox']
# 获取嵌入层
embeddings = model.embeddings
# 提取'fox'的嵌入向量
fox_vector = embeddings(torch.tensor([fox_index], dtype=torch.long))
# 打印向量
print("Embedding vector for 'fox':")
print(fox_vector)
else:
print("Word 'fox' not found in the vocabulary.")
我们这里嵌入词向量长度为10,见代码,看到打印结果长度也是10,这是正确的:
我的课程
我打造了一个《Python从零到高薪就业全栈视频课》,目前上线700节课程,每节课15分钟,总共超180个小时。包括:《从零学Python》、《Python进阶》、《爬虫》、《NumPy数值分析》、《Pandas数据分析》、《Matplotlib和Pyecharts绘图》、《PyQt软件开发》、《接单项目串讲》、《Python办公自动化》、《多线程和多进程》、《unittest和pytest自动化测试》、《Flask和Django网站开发》、《基础算法》、《人工智能入门》、《机器学习》、《深度学习》、《Pytorch实战》,将我过去工作8年以及现在科研的经历都融入到课程中,里面有很多实际项目,是一个全栈技术课。
如果你想掌握全栈开发技术,提升你自己,提升就业能力,多学技能做副业项目赚钱等,可以报名,课程带有我的答疑。价格现在比较优惠,推荐现在加入。长按下方二维码查看,报名后加我微信:gz113097485