Structured Fusion Networks for Dialog
似乎跨领域确实是个问题
overwhelming implicit language model
Using reinforcement learning to fine-tune a decoder, will likely place a strong emphasis on improving the decoder’s policy and un-learn the im- plicit language model of the decoder.就是decoder的生成能力变差。查看rethinking的原文解释。
fusion:pretrained language model与(seq2seq的)decoder融合
1.Zero-Shot Naive Fusion
不明白为什么加上zero-shot,没什么新意,就是说可以backprop,
Since the forward propagation described in Equa- tions 5 and 6 is continuous and there is no sampling procedure until the response is generated。
2.multitask fusion
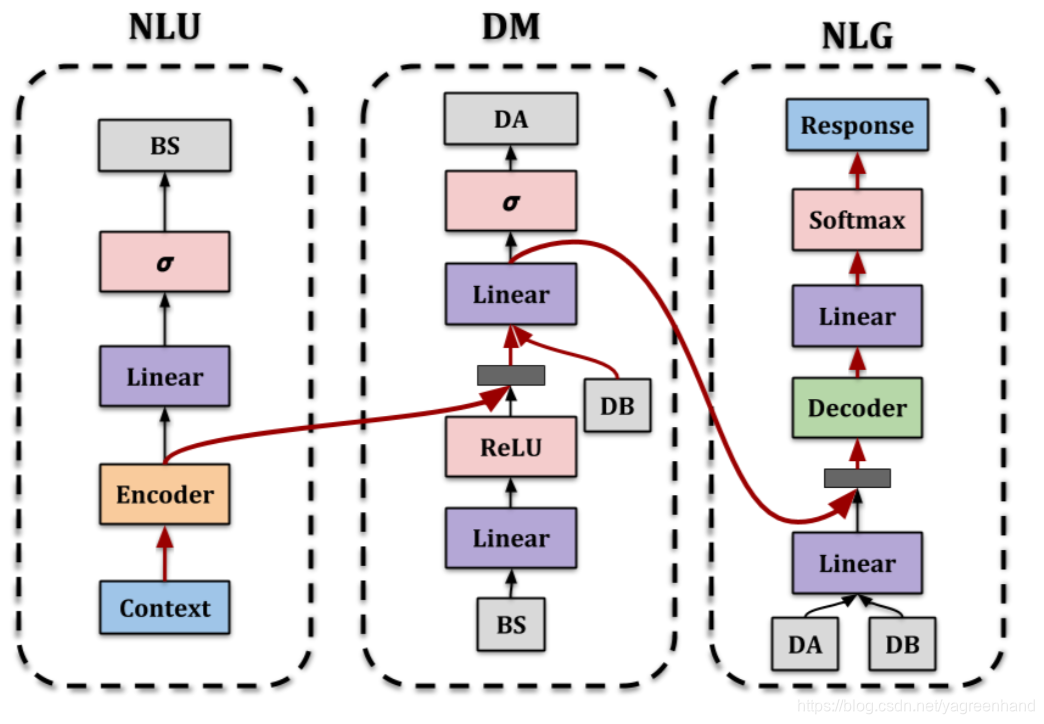
the individual neural modules are learned simulta- neously with the end-to-end task of dialog genera- tion.应该是loss 相加。encoder能学到怎么生成belief state,还能学到DM过程中需要提供的知识。
3. Structured Fusion Networks
就是增加输入的约束,没什么厉害的