网上学习资料一大堆,但如果学到的知识不成体系,遇到问题时只是浅尝辄止,不再深入研究,那么很难做到真正的技术提升。
一个人可以走的很快,但一群人才能走的更远!不论你是正从事IT行业的老鸟或是对IT行业感兴趣的新人,都欢迎加入我们的的圈子(技术交流、学习资源、职场吐槽、大厂内推、面试辅导),让我们一起学习成长!
LogisticRegressionTrainingSummary提供LogisticRegressionModel的一些训练指标摘要。 在二进制分类的情况下例如, ROC曲线。
继续前面的示例:
# -\*- coding: utf-8 -\*-
from __future__ import print_function
from pyspark.ml.classification import LogisticRegression
from pyspark.sql import SparkSession
if __name__ == "\_\_main\_\_":
spark = SparkSession \
.builder \
.appName("LogisticRegressionSummary") \
.getOrCreate()
training = spark.read.format("libsvm").load("../data/mllib/sample\_libsvm\_data.txt")
lr = LogisticRegression(maxIter=10, regParam=0.3, elasticNetParam=0.8)
lrModel = lr.fit(training)
trainingSummary = lrModel.summary
# 获得每次迭代的优化目标(损失 + 惩罚项)
objectiveHistory = trainingSummary.objectiveHistory
print("objectiveHistory:")
for objective in objectiveHistory:
print(objective)
# Obtain the receiver-operating characteristic as a dataframe and areaUnderROC.
trainingSummary.roc.show()
print("areaUnderROC: " + str(trainingSummary.areaUnderROC))
# 设置模型阈值,使得最大化F度量值
fMeasure = trainingSummary.fMeasureByThreshold
maxFMeasure = fMeasure.groupBy().max('F-Measure').select('max(F-Measure)').head()
bestThreshold = fMeasure.where(fMeasure['F-Measure'] == maxFMeasure['max(F-Measure)']) \
.select('threshold').head()['threshold']
lr.setThreshold(bestThreshold)
spark.stop()
日志信息:
objectiveHistory:
0.683314913574
...
+---+--------------------+
|FPR| TPR|
+---+--------------------+
|0.0| 0.0|
|0.0|0.017543859649122806|
|0.0| 0.03508771929824561|
|0.0| 0.05263157894736842|
|0.0| 0.07017543859649122|
|0.0| 0.08771929824561403|
|0.0| 0.10526315789473684|
|0.0| 0.12280701754385964|
|0.0| 0.14035087719298245|
|0.0| 0.15789473684210525|
|0.0| 0.17543859649122806|
|0.0| 0.19298245614035087|
|0.0| 0.21052631578947367|
|0.0| 0.22807017543859648|
|0.0| 0.24561403508771928|
|0.0| 0.2631578947368421|
|0.0| 0.2807017543859649|
|0.0| 0.2982456140350877|
|0.0| 0.3157894736842105|
|0.0| 0.3333333333333333|
+---+--------------------+
only showing top 20 rows
areaUnderROC: 1.0
1.2 多分类LR
示例代码如下:
# -\*- coding: utf-8 -\*-
from __future__ import print_function
from pyspark.ml.classification import LogisticRegression
from pyspark.sql import SparkSession
if __name__ == "\_\_main\_\_":
spark = SparkSession \
.builder \
.appName("MulticlassLogisticRegressionWithElasticNet") \
.getOrCreate()
training = spark \
.read \
.format("libsvm") \
.load("../data/mllib/sample\_multiclass\_classification\_data.txt")
lr = LogisticRegression(maxIter=10, regParam=0.3, elasticNetParam=0.8)
lrModel = lr.fit(training)
# 系数和截距项
print("Coefficients: \n" + str(lrModel.coefficientMatrix))
print("Intercept: " + str(lrModel.interceptVector))
trainingSummary = lrModel.summary
# 获得每次迭代的优化目标(损失 + 惩罚项)
objectiveHistory = trainingSummary.objectiveHistory
print("objectiveHistory:")
for objective in objectiveHistory:
print(objective)
# 可以查看每个类的FPR & TPR
print("False positive rate by label:")
for i, rate in enumerate(trainingSummary.falsePositiveRateByLabel):
print("label %d: %s" % (i, rate))
print("True positive rate by label:")
for i, rate in enumerate(trainingSummary.truePositiveRateByLabel):
print("label %d: %s" % (i, rate))
print("Precision by label:")
for i, prec in enumerate(trainingSummary.precisionByLabel):
print("label %d: %s" % (i, prec))
print("Recall by label:")
for i, rec in enumerate(trainingSummary.recallByLabel):
print("label %d: %s" % (i, rec))
print("F-measure by label:")
for i, f in enumerate(trainingSummary.fMeasureByLabel()):
print("label %d: %s" % (i, f))
accuracy = trainingSummary.accuracy
falsePositiveRate = trainingSummary.weightedFalsePositiveRate
truePositiveRate = trainingSummary.weightedTruePositiveRate
fMeasure = trainingSummary.weightedFMeasure()
precision = trainingSummary.weightedPrecision
recall = trainingSummary.weightedRecall
print("Accuracy: %s\nFPR: %s\nTPR: %s\nF-measure: %s\nPrecision: %s\nRecall: %s"
% (accuracy, falsePositiveRate, truePositiveRate, fMeasure, precision, recall))
spark.stop()
结果如下:
Coefficients:
3 X 4 CSRMatrix
(0,3) 0.3176
(1,2) -0.7804
(1,3) -0.377
Intercept: [0.05165231659832854,-0.12391224990853622,0.07225993331020768]
objectiveHistory:
1.09861228867
...
False positive rate by label:
label 0: 0.22
label 1: 0.05
label 2: 0.0
True positive rate by label:
label 0: 1.0
label 1: 1.0
label 2: 0.46
Precision by label:
label 0: 0.694444444444
label 1: 0.909090909091
label 2: 1.0
Recall by label:
label 0: 1.0
label 1: 1.0
label 2: 0.46
F-measure by label:
label 0: 0.819672131148
label 1: 0.952380952381
label 2: 0.630136986301
Accuracy: 0.82
FPR: 0.09
TPR: 0.82
F-measure: 0.800730023277
Precision: 0.867845117845
Recall: 0.82
2. 决策树分类器
举例
以LibSVM格式加载数据集,将其拆分为训练集和测试集,在第一个数据集上训练,然后在保留的测试集上进行评估。 我们使用两个特征变换器(transformers)来准备数据; 这些帮助标记和分类特征的索引类别,向决策树算法可识别的DataFrame添加元数据。
# -\*- coding: utf-8 -\*-
from __future__ import print_function
from pyspark.ml import Pipeline
from pyspark.ml.classification import DecisionTreeClassifier
from pyspark.ml.feature import StringIndexer, VectorIndexer
from pyspark.ml.evaluation import MulticlassClassificationEvaluator
from pyspark.sql import SparkSession
if __name__ == "\_\_main\_\_":
spark = SparkSession\
.builder\
.appName("DecisionTreeClassificationExample")\
.getOrCreate()
data = spark.read.format("libsvm").load("../data/mllib/sample\_libsvm\_data.txt")
# 对于整个数据集,将label转换为索引
labelIndexer = StringIndexer(inputCol="label", outputCol="indexedLabel").fit(data)
# 自动识别数据集中的分类特征,并且进行矢量化处理;设定maxCategories,以便将具有> 4个不同值的特性视为连续的。
featureIndexer = VectorIndexer(inputCol="features", outputCol="indexedFeatures", maxCategories=4).fit(data)
# 切分训练集和测试集
(trainingData, testData) = data.randomSplit([0.7, 0.3])
# 训练一颗决策树
dt = DecisionTreeClassifier(labelCol="indexedLabel", featuresCol="indexedFeatures")
# 连接indexers和决策树
pipeline = Pipeline(stages=[labelIndexer, featureIndexer, dt])
model = pipeline.fit(trainingData)
# 进行预测
predictions = model.transform(testData)
predictions.select("prediction", "indexedLabel", "features").show(5)
# 计算测试误差
evaluator = MulticlassClassificationEvaluator(
labelCol="indexedLabel", predictionCol="prediction", metricName="accuracy")
accuracy = evaluator.evaluate(predictions)
print("Test Error = %g " % (1.0 - accuracy))
treeModel = model.stages[2]
print(treeModel)
spark.stop()
结果如下:
+----------+------------+--------------------+
|prediction|indexedLabel| features|
+----------+------------+--------------------+
| 1.0| 1.0|(692,[98,99,100,1...|
| 1.0| 1.0|(692,[121,122,123...|
| 1.0| 1.0|(692,[122,123,148...|
| 1.0| 1.0|(692,[124,125,126...|
| 1.0| 1.0|(692,[126,127,128...|
+----------+------------+--------------------+
only showing top 5 rows
Test Error = 0.0357143
DecisionTreeClassificationModel (uid=DecisionTreeClassifier_4f508c37c4be93461970) of depth 1 with 3 nodes
3. 随机森林分类器
与DT类似的,只不过选择RF来进行训练,示例代码如下:
# -\*- coding: utf-8 -\*-
from __future__ import print_function
from pyspark.ml import Pipeline
from pyspark.ml.classification import RandomForestClassifier
from pyspark.ml.feature import IndexToString, StringIndexer, VectorIndexer
from pyspark.ml.evaluation import MulticlassClassificationEvaluator
from pyspark.sql import SparkSession
if __name__ == "\_\_main\_\_":
spark = SparkSession\
.builder\
.appName("RandomForestClassifierExample")\
.getOrCreate()
# 处理方式如DT类似
data = spark.read.format("libsvm").load("../data/mllib/sample\_libsvm\_data.txt")
labelIndexer = StringIndexer(inputCol="label", outputCol="indexedLabel").fit(data)
featureIndexer = VectorIndexer(inputCol="features", outputCol="indexedFeatures", maxCategories=4).fit(data)
(trainingData, testData) = data.randomSplit([0.7, 0.3])
# TRAIN RF
rf = RandomForestClassifier(labelCol="indexedLabel", featuresCol="indexedFeatures", numTrees=10)
# 将标签的索引转换为原始标签
labelConverter = IndexToString(inputCol="prediction", outputCol="predictedLabel", labels=labelIndexer.labels)
# 在Pipeline中进行整个训练流程
pipeline = Pipeline(stages=[labelIndexer, featureIndexer, rf, labelConverter])
model = pipeline.fit(trainingData)
predictions = model.transform(testData)
predictions.select("predictedLabel", "label", "features").show(5)
evaluator = MulticlassClassificationEvaluator(labelCol="indexedLabel", predictionCol="prediction",
metricName="accuracy")
accuracy = evaluator.evaluate(predictions)
print("Test Error = %g" % (1.0 - accuracy))
rfModel = model.stages[2]
print(rfModel)
spark.stop()
结果如下:
+--------------+-----+--------------------+
|predictedLabel|label| features|
+--------------+-----+--------------------+
| 0.0| 0.0|(692,[98,99,100,1...|
| 0.0| 0.0|(692,[122,123,148...|
| 0.0| 0.0|(692,[124,125,126...|
| 0.0| 0.0|(692,[124,125,126...|
| 0.0| 0.0|(692,[124,125,126...|
+--------------+-----+--------------------+
only showing top 5 rows
Test Error = 0.0294118
RandomForestClassificationModel (uid=RandomForestClassifier_421b9fdfb8d0ee9acde3) with 10 trees
4. 梯度提升树分类器
如前文类似,选用梯度提升树(Gradient-boosted trees, GBTs)来进行训练,示例代码如下:
# -\*- coding: utf-8 -\*-
from __future__ import print_function
from pyspark.ml import Pipeline
from pyspark.ml.classification import GBTClassifier
from pyspark.ml.feature import StringIndexer, VectorIndexer
from pyspark.ml.evaluation import MulticlassClassificationEvaluator
from pyspark.sql import SparkSession
if __name__ == "\_\_main\_\_":
spark = SparkSession\
.builder\
.appName("GradientBoostedTreeClassifierExample")\
.getOrCreate()
data = spark.read.format("libsvm").load("../data/mllib/sample\_libsvm\_data.txt")
labelIndex = StringIndexer(inputCol="label", outputCol="indexedLabel").fit(data)
featureIndexer = VectorIndexer(inputCol="features", outputCol="indexedFeatures", maxCategories=4).fit(data)
(trainingData, testData) = data.randomSplit([0.7, 0.3])
# train
gbt = GBTClassifier(labelCol="indexedLabel", featuresCol="indexedFeatures", maxDepth=10)
# 在管道中进行整个训练流程
pipeline = Pipeline(stages=[labelIndex, featureIndexer, gbt])
model = pipeline.fit(trainingData)
# 预测
predictions = model.transform(testData)
predictions.select("prediction", "indexedLabel", "features").show(5)
# 计算测试误差
evaluator = MulticlassClassificationEvaluator(labelCol="indexedLabel", predictionCol="prediction",
metricName="accuracy")
accuracy = evaluator.evaluate(predictions)
print("Test Error = %g" % (1.0 - accuracy))
gbtModel = model.stages[2]
print(gbtModel)
spark.stop()
结果如下:
+----------+------------+--------------------+
|prediction|indexedLabel| features|
+----------+------------+--------------------+
| 1.0| 1.0|(692,[95,96,97,12...|
| 1.0| 1.0|(692,[100,101,102...|
| 1.0| 1.0|(692,[122,123,148...|
| 1.0| 1.0|(692,[123,124,125...|
| 1.0| 1.0|(692,[124,125,126...|
+----------+------------+--------------------+
only showing top 5 rows
Test Error = 0
GBTClassificationModel (uid=GBTClassifier_4a1fa549ada75fa70795) with 20 trees
5. 多层感知器分类器
多层感知器分类器(Multilayer perceptron classifier, MLPC)是基于前馈人工神经网络的分类器。 MLPC由多层节点组成。 每层完全连接到网络中的下一层。 输入层中的节点表示输入数据。 所有其他节点通过输入与节点权重www和偏差bbb的线性组合将输入映射到输出,并应用激活函数。 这可以用矩阵形式写入MLPC,K+1层如下:
中间层中的节点使用sigmoid函数:
输出层中的节点使用softmax函数:
输出层中的节点数N对应于类的数量。
MLPC采用反向传播来学习模型。 我们使用逻辑损失函数进行优化,使用L-BFGS作为优化过程。
示例代码如下:
# -\*- coding: utf-8 -\*-
from __future__ import print_function
from pyspark.ml.classification import MultilayerPerceptronClassifier
from pyspark.ml.evaluation import MulticlassClassificationEvaluator
from pyspark.sql import SparkSession
if __name__ == "\_\_main\_\_":
spark = SparkSession.builder.appName("multilayer\_perceptron\_classification\_example").getOrCreate()
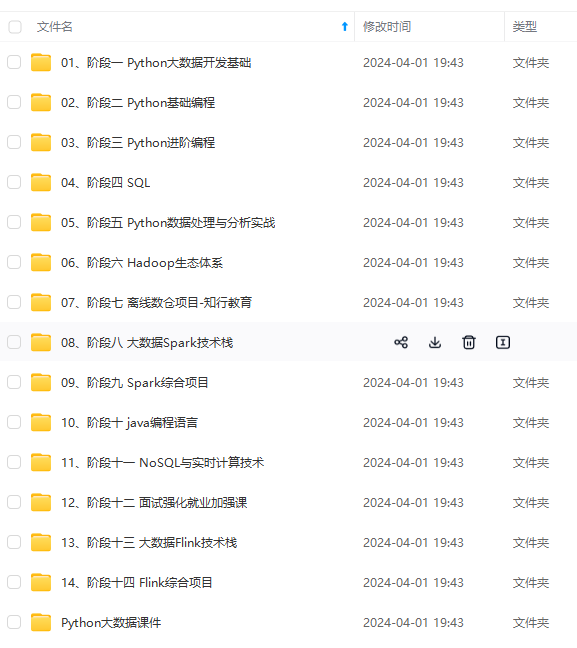
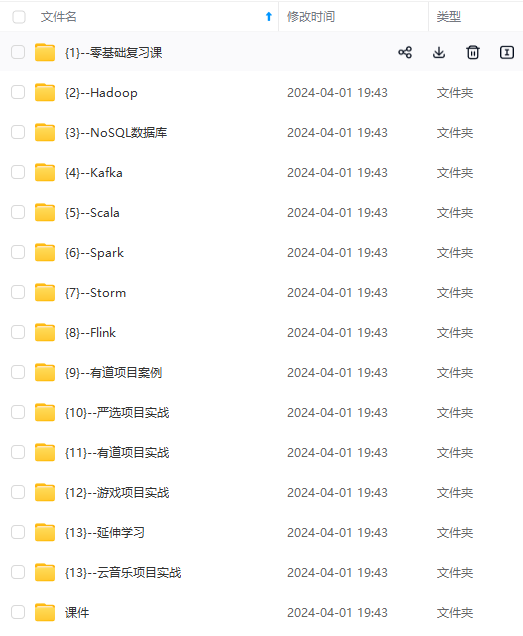
**网上学习资料一大堆,但如果学到的知识不成体系,遇到问题时只是浅尝辄止,不再深入研究,那么很难做到真正的技术提升。**
**[需要这份系统化资料的朋友,可以戳这里获取](https://bbs.csdn.net/topics/618545628)**
**一个人可以走的很快,但一群人才能走的更远!不论你是正从事IT行业的老鸟或是对IT行业感兴趣的新人,都欢迎加入我们的的圈子(技术交流、学习资源、职场吐槽、大厂内推、面试辅导),让我们一起学习成长!**
ark.sql import SparkSession
if __name__ == "\_\_main\_\_":
spark = SparkSession.builder.appName("multilayer\_perceptron\_classification\_example").getOrCreate()
[外链图片转存中...(img-Q8meKHSX-1714914002062)]
[外链图片转存中...(img-4LNVonrQ-1714914002063)]
**网上学习资料一大堆,但如果学到的知识不成体系,遇到问题时只是浅尝辄止,不再深入研究,那么很难做到真正的技术提升。**
**[需要这份系统化资料的朋友,可以戳这里获取](https://bbs.csdn.net/topics/618545628)**
**一个人可以走的很快,但一群人才能走的更远!不论你是正从事IT行业的老鸟或是对IT行业感兴趣的新人,都欢迎加入我们的的圈子(技术交流、学习资源、职场吐槽、大厂内推、面试辅导),让我们一起学习成长!**