Datawhale干货
作者:皮钱超,厦门大学,Datawhale成员
深度学习框架Keras入门项目
本文介绍3个案例来帮助读者认识和入门深度学习框架Keras。3个案例解决3个问题:回归、二分类、多分类.
本文审稿人:牧小熊,Datawhale成员
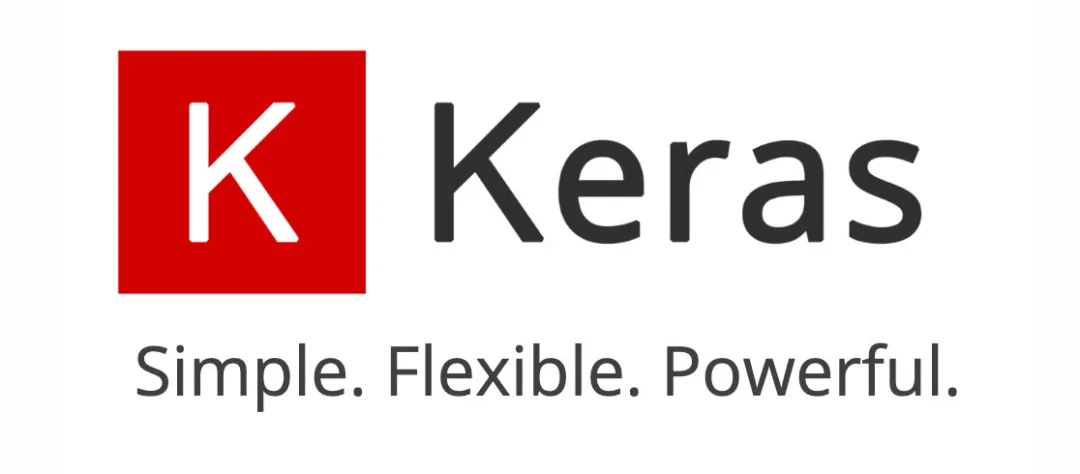
为什么选择Keras
相信很多小伙伴在入门深度学习时候首选框架应该是TensorFlow或者Pytorch。在如今无数深度学习框架中,为什么要使用 Keras 而非其他?整理自Keras中文官网:
Keras 优先考虑开发人员的经验
Keras 被工业界和学术界广泛采用
Keras 可以轻松将模型转化为产品
Keras 支持多个后端引擎
Keras 拥有强大的多 GPU 和分布式训练支持
Keras 的发展得到关键公司的支持,比如:谷歌、微软等
详细信息见中文官网:https://keras.io/zh/why-use-keras/
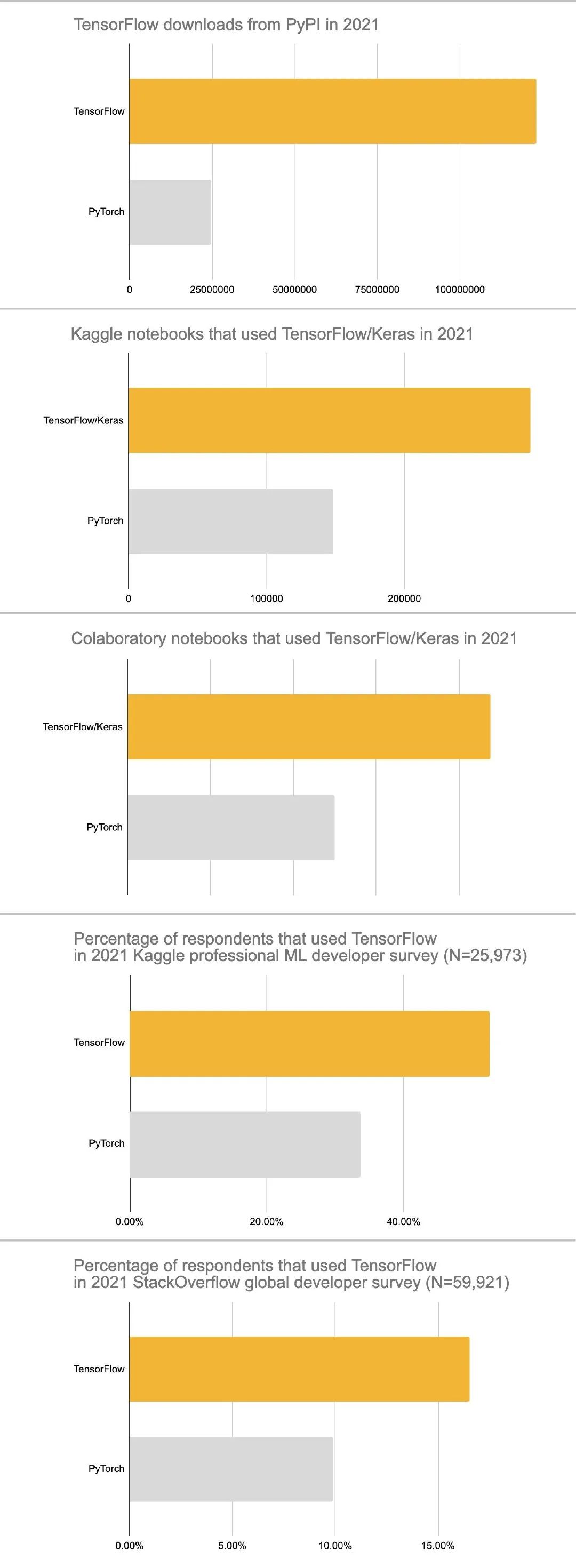
主要步骤
使用Keras解决机器学习/深度学习问题的主要步骤:
特征工程+数据划分
搭建神经网络模型add
查看网络架构summary
编译网络模型compile
训练网络fit
保存模型save
评估模型evaluate
评价指标可视化visualize
导入库
import pandas as pd
import numpy as np
import matplotlib.pyplot as plt
%matplotlib inline
from sklearn import datasets
from sklearn.preprocessing import MinMaxScaler
from sklearn.preprocessing import StandardScaler
from sklearn.model_selection import train_test_split
import tensorflow as tf
from keras import models
from keras import layers
from keras.models import load_model
np.random.seed(1234)
回归案例
回归案例中使用的是Keras自带的波士顿房价数据集。
导入数据
In [2]:
from keras.datasets import boston_housing
(train_X, train_y), (test_X, test_y) = boston_housing.load_data()
In [3]:
train_X.shape # 数据形状
Out[3]:
(404, 13)
In [4]:
train_X[:3] # 特征向量值
Out[4]:
array([[1.23247e+00, 0.00000e+00, 8.14000e+00, 0.00000e+00, 5.38000e-01,
6.14200e+00, 9.17000e+01, 3.97690e+00, 4.00000e+00, 3.07000e+02,
2.10000e+01, 3.96900e+02, 1.87200e+01],
[2.17700e-02, 8.25000e+01, 2.03000e+00, 0.00000e+00, 4.15000e-01,
7.61000e+00, 1.57000e+01, 6.27000e+00, 2.00000e+00, 3.48000e+02,
1.47000e+01, 3.95380e+02, 3.11000e+00],
[4.89822e+00, 0.00000e+00, 1.81000e+01, 0.00000e+00, 6.31000e-01,
4.97000e+00, 1.00000e+02, 1.33250e+00, 2.40000e+01, 6.66000e+02,
2.02000e+01, 3.75520e+02, 3.26000e+00]])
In [5]:
train_y[:3] # 标签值
Out[5]:
array([15.2, 42.3, 50. ])
数据标准化
神经网络中一般输入的都是较小数值的数据,数据之间的差异不能过大。现将特征变量的数据进行标准化处理
In [6]:
train_X[:3] # 处理前
Out[6]:
array([[1.23247e+00, 0.00000e+00, 8.14000e+00, 0.00000e+00, 5.38000e-01,
6.14200e+00, 9.17000e+01, 3.97690e+00, 4.00000e+00, 3.07000e+02,
2.10000e+01, 3.96900e+02, 1.87200e+01],
[2.17700e-02, 8.25000e+01, 2.03000e+00, 0.00000e+00, 4.15000e-01,
7.61000e+00, 1.57000e+01, 6.27000e+00, 2.00000e+00, 3.48000e+02,
1.47000e+01, 3.95380e+02, 3.11000e+00],
[4.89822e+00, 0.00000e+00, 1.81000e+01, 0.00000e+00, 6.31000e-01,
4.97000e+00, 1.00000e+02, 1.33250e+00, 2.40000e+01, 6.66000e+02,
2.02000e+01, 3.75520e+02, 3.26000e+00]])
针对训练集的数据做标准化处理:减掉均值再除以标准差
In [7]:
mean