gt_overlaps的源头来自于如下代码:
def evaluate_recall(self, candidate_boxes=None, thresholds=None,
area='all', limit=None):
"""Evaluate detection proposal recall metrics.
Returns:
results: dictionary of results with keys
'ar': average recall
'recalls': vector recalls at each IoU overlap threshold
'thresholds': vector of IoU overlap thresholds
'gt_overlaps': vector of all ground-truth overlaps
"""
# Record max overlap value for each gt box
# Return vector of overlap values
areas = {'all': 0, 'small': 1, 'medium': 2, 'large': 3,
'96-128': 4, '128-256': 5, '256-512': 6, '512-inf': 7}
area_ranges = [[0 ** 2, 1e5 ** 2], # all
[0 ** 2, 32 ** 2], # small
[32 ** 2, 96 ** 2], # medium
[96 ** 2, 1e5 ** 2], # large
[96 ** 2, 128 ** 2], # 96-128
[128 ** 2, 256 ** 2], # 128-256
[256 ** 2, 512 ** 2], # 256-512
[512 ** 2, 1e5 ** 2], # 512-inf
]
assert area in areas, 'unknown area range: {}'.format(area)
area_range = area_ranges[areas[area]]
gt_overlaps = np.zeros(0)
num_pos = 0
for i in range(self.num_images):
# Checking for max_overlaps == 1 avoids including crowd annotations
# (...pretty hacking :/)
max_gt_overlaps = self.roidb[i]['gt_overlaps'].toarray().max(axis=1)
gt_inds = np.where((self.roidb[i]['gt_classes'] > 0) &
(max_gt_overlaps == 1))[0]
gt_boxes = self.roidb[i]['boxes'][gt_inds, :]
gt_areas = self.roidb[i]['seg_areas'][gt_inds]
valid_gt_inds = np.where((gt_areas >= area_range[0]) &
(gt_areas <= area_range[1]))[0]
gt_boxes = gt_boxes[valid_gt_inds, :]
num_pos += len(valid_gt_inds)
if candidate_boxes is None:
# If candidate_boxes is not supplied, the default is to use the
# non-ground-truth boxes from this roidb
non_gt_inds = np.where(self.roidb[i]['gt_classes'] == 0)[0]
boxes = self.roidb[i]['boxes'][non_gt_inds, :]
else:
boxes = candidate_boxes[i]
if boxes.shape[0] == 0:
continue
if limit is not None and boxes.shape[0] > limit:
boxes = boxes[:limit, :]
overlaps = bbox_overlaps(boxes.astype(np.float),
gt_boxes.astype(np.float))
_gt_overlaps = np.zeros((gt_boxes.shape[0]))
for j in range(gt_boxes.shape[0]):
# find which proposal box maximally covers each gt box
argmax_overlaps = overlaps.argmax(axis=0)
# and get the iou amount of coverage for each gt box
max_overlaps = overlaps.max(axis=0)
# find which gt box is 'best' covered (i.e. 'best' = most iou)
gt_ind = max_overlaps.argmax()
gt_ovr = max_overlaps.max()
assert (gt_ovr >= 0)
# find the proposal box that covers the best covered gt box
box_ind = argmax_overlaps[gt_ind]
# record the iou coverage of this gt box
_gt_overlaps[j] = overlaps[box_ind, gt_ind]
assert (_gt_overlaps[j] == gt_ovr)
# mark the proposal box and the gt box as used
overlaps[box_ind, :] = -1
overlaps[:, gt_ind] = -1
# append recorded iou coverage level
gt_overlaps = np.hstack((gt_overlaps, _gt_overlaps))
gt_overlaps = np.sort(gt_overlaps)
if thresholds is None:
step = 0.05
thresholds = np.arange(0.5, 0.95 + 1e-5, step)
recalls = np.zeros_like(thresholds)
# compute recall for each iou threshold
for i, t in enumerate(thresholds):
recalls[i] = (gt_overlaps >= t).sum() / float(num_pos)
# ar = 2 * np.trapz(recalls, thresholds)
ar = recalls.mean()
return {'ar': ar, 'recalls': recalls, 'thresholds': thresholds,
'gt_overlaps': gt_overlaps}
从改代码中可以看出:gt_overlaps记录的是candidate_boxes(假定数量为M)与gt_boxes(假定数量为N)之间的IoU的值,这些值构成的是一个MxN的矩阵。上述代码的作用就在M个candidate框当中取出与每个gt_boxes的IoU最大的那个框,这时有两种情况,如下图所示:
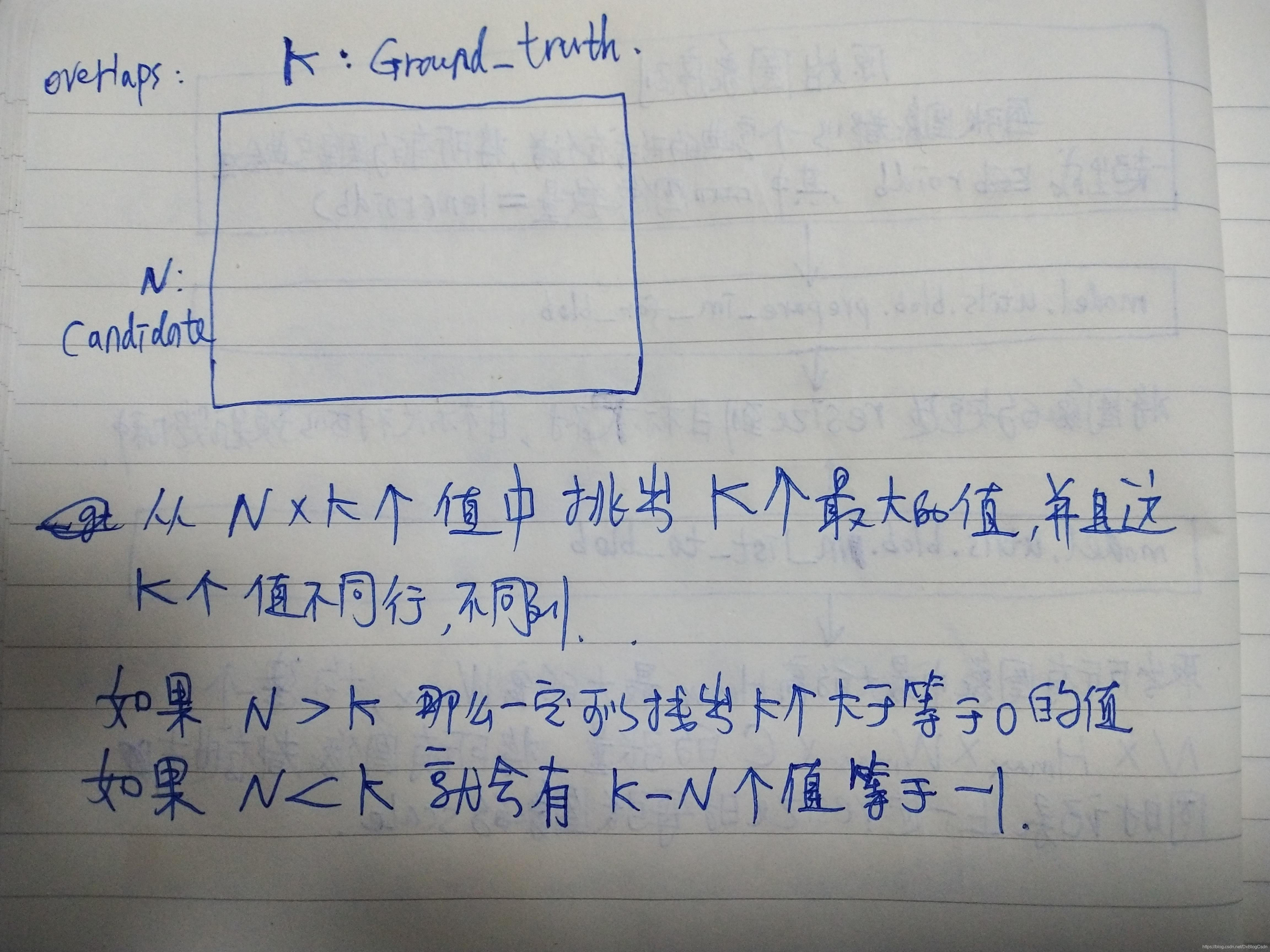
从上图的分析中可以看到,存在gt_overlaps=-1的请框,这就意味着candidate box的数量大于gt_boxes的数量,这也就是is_crowded这个标记的来源。