import input_data
import tensorflow as tf
import numpy as np
import matplotlib.pyplot as plt
mnist = input_data.read_data_sets('data/', one_hot=True)
#设置训练超参
learning_rate = 0.01
training_epochs = 20
batch_size = 256
display_step = 1
examples_to_show = 10
#网络参数
n_hidden_1 = 256
n_hidden_2 = 128
n_input = 784
X = tf.placeholder("float", [None, n_input])
weights = {
'encoder_h1': tf.Variable(tf.random_normal([n_input, n_hidden_1])),
'encoder_h2': tf.Variable(tf.random_normal([n_hidden_1, n_hidden_2])),
'decoder_h1': tf.Variable(tf.random_normal([n_hidden_2, n_hidden_1])),
'decoder_h2': tf.Variable(tf.random_normal([n_hidden_1, n_input])),
}
biases = {
'encoder_b1': tf.Variable(tf.random_normal([n_hidden_1])),
'encoder_b2': tf.Variable(tf.random_normal([n_hidden_2])),
'decoder_b1': tf.V
TensorFlow(六)——MNIST分类之自动编码器
最新推荐文章于 2023-11-15 10:45:05 发布
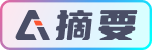