MapPartitionsRDD分析
package org.apache.spark.day02
import org.apache.spark.rdd.{
MapPartitionsRDD, RDD}
import org.apache.spark.{
SparkConf, SparkContext}
object MapPartitionersRddDemo {
def main(args: Array[String]): Unit = {
val conf: SparkConf = new SparkConf().setAppName("data").setMaster("local[*]")
val sc = new SparkContext(conf)
val array = Array(1,2,3, 4,5,6, 7,8,9,10)
val dataRDD: RDD[Int] = sc.parallelize(array, 3)
val mapRDD: RDD[Int] = dataRDD.map(_ * 10)
val func1 = (e: Int) => e * 10
val mapPartitionsRdd: MapPartitionsRDD[Int, Int] =
new MapPartitionsRDD[Int, Int](dataRDD, (_, _, _iterator) => _iterator.map(func1))
println("map(): ", mapRDD.collect().toBuffer)
println("MapPartitionsRDD--map: ", mapPartitionsRdd.collect().toBuffer)
val filterRDD: RDD[Int] = dataRDD.filter(_ % 2 == 0)
val func2 = (e: Int) => e % 2 == 0
val mapPartitionsRDDFilter: MapPartitionsRDD[Int, Int] =
new MapPartitionsRDD[Int, Int](dataRDD, (_, _, _iterator) => _iterator.filter(func2))
println("filterRDD: ", filterRDD.collect().toBuffer)
println("MapPartitionsRDD--filter: ", mapPartitionsRDDFilter.collect().toBuffer)
sc.stop()
}
}
关于Map窄依赖
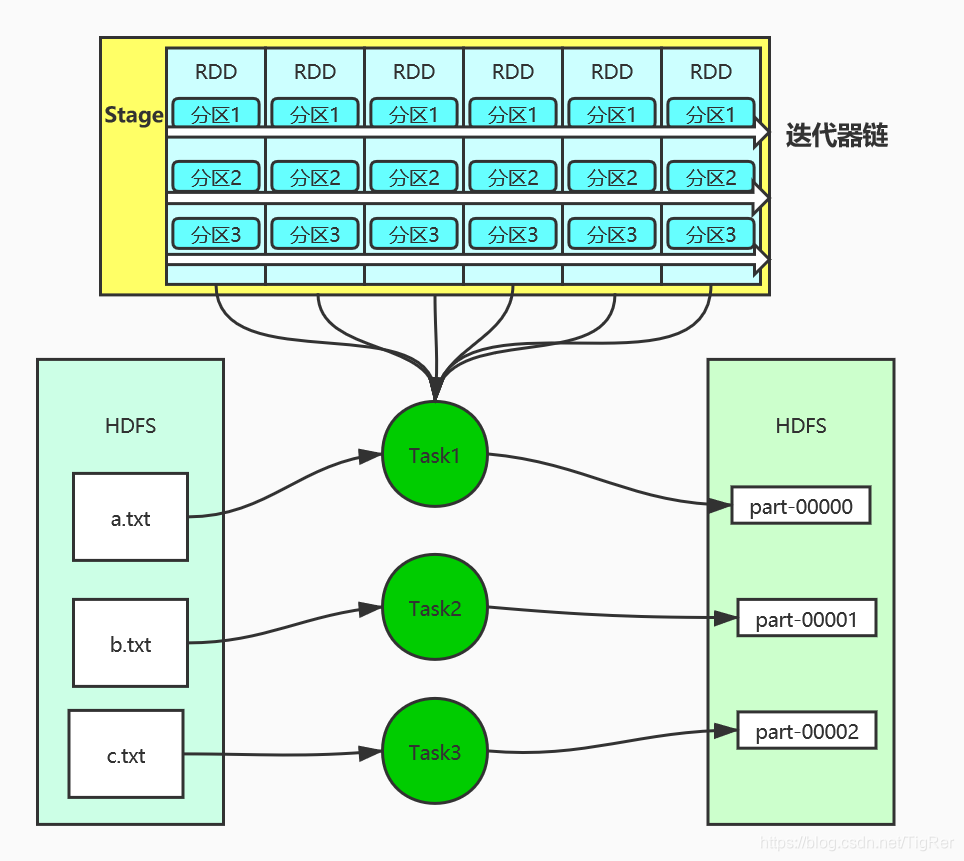
package cn.huq.day02
import org.apache.spark.rdd.RDD
import org.apache.spark.{
SparkConf, SparkContext}
class MapDemo {
def main(args: Array[String]): Unit = {
val sc: SparkContext = new SparkContext(config = new SparkConf().setAppName("map demo").setMaster("local"))
val dataRDD = sc.textFile(args(0))
val rdd1: RDD[Int] = dataRDD.map(_.toInt)
val rdd2: RDD[Int] = rdd1.filter(_ % 2 == 0)
val rdd3: RDD[Int] = rdd2.map(_ * 10)
rdd3.saveAsTextFile(args(1))
sc.stop()
}
}
def textFile(
path: String,
minPartitions: Int = defaultMinPartitions): RDD[String] = withScope {
assertNotStopped()
hadoopFile(path, classOf[TextInputFormat], classOf[LongWritable], classOf[Text],
minPartitions).map(pair => pair._2.toString).setName(path)
}
def map[U: ClassTag](f: T => U): RDD[U] = withScope {
val cleanF = sc.clean(f)
new MapPartitionsRDD[U, T](this, (context, pid, iter) => iter.map(cleanF))
}
def filter(f: T => Boolean): RDD[T] = withScope {
val cleanF = sc.clean(f)
new MapPartitionsRDD[T, T](
this,
(context, pid, iter) => iter.filter(cleanF),
preservesPartitioning = true)
}
def saveAsTextFile(path: String): Unit = withScope {
val nullWritableClassTag = implicitly[ClassTag[NullWritable]]
val textClassTag = implicitly