https://mp.weixin.qq.com/s/s6Hl8g4mCq-AiMxR03Y6KQ
Focal loss用于解决一阶段目标检测场景中前后背景不平衡的问题。使用此损失让网络学习过程中更加专注于难例。
down-weight easy examples and thus focus training on hard negatives
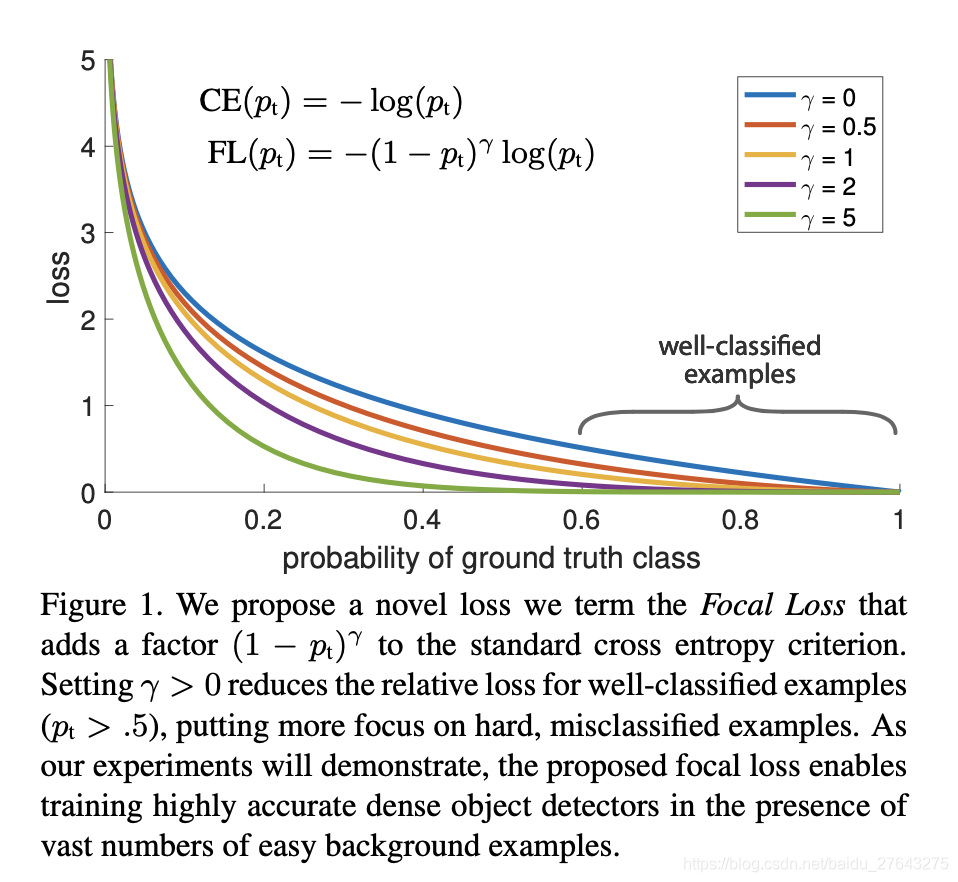
easily classified (pt ≫ 0.5)
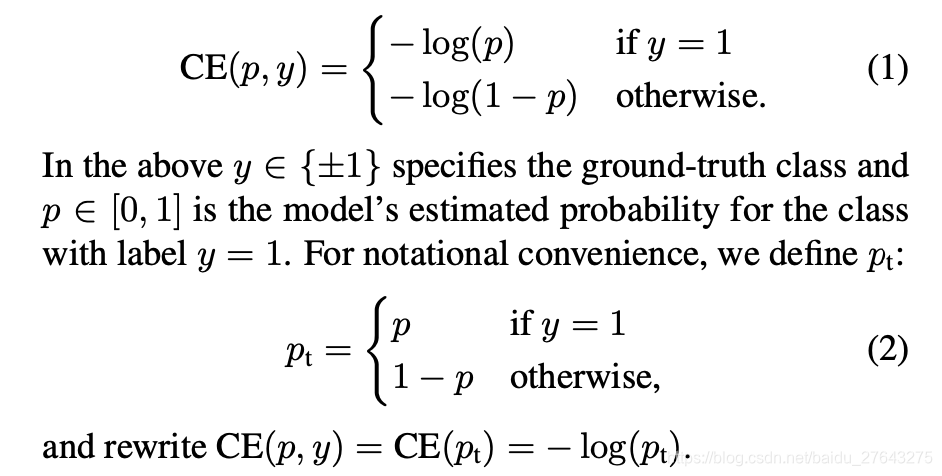
Focal loss
F L ( p t ) = − α t ( 1 − p t ) γ log ( p t ) \mathrm{FL}\left(p_{\mathrm{t}}\right)=-\alpha_{\mathrm{t}}\left(1-p_{\mathrm{t}}\right)^{\gamma} \log \left(p_{\mathrm{t}}\right) FL(pt)=−αt(1−pt)γlog(pt)
p t = { p if y = 1 1 − p otherwise p_{\mathrm{t}}=\left\{\begin{array}{ll}{p} & {\text { if } y=1} \\ {1-p} & {\text { otherwise }}\end{array}\right. pt={p1−p if y=1 otherwise
As pt → 1
, the factor goes to 0 and the loss for well-classified examples
is down-weighted.
tensorflow实现
import tensorflow as tf
def focal_loss_sigmoid(labels, logits, gamma=2, alpha=0.25):
"""
Computer focal loss for binary classification
Args:
labels: A int32 tensor of shape [batch_size].
logits: A float32 tensor of shape [batch_size].
alpha: class weights
gamma: A scalar for focal loss gamma hyper-parameter.
Returns:
A tensor of the same shape as `lables`
"""
logits = tf.nn.sigmoid(logits)
focal_loss = -labels * (1 - alpha) * tf.pow(1 - logits, gamma) * tf.log(logits) \
- (1 - labels) * (alpha) * tf.pow(logits, gamma) * tf.log(1 - logits)
return focal_loss
参考:focal_loss