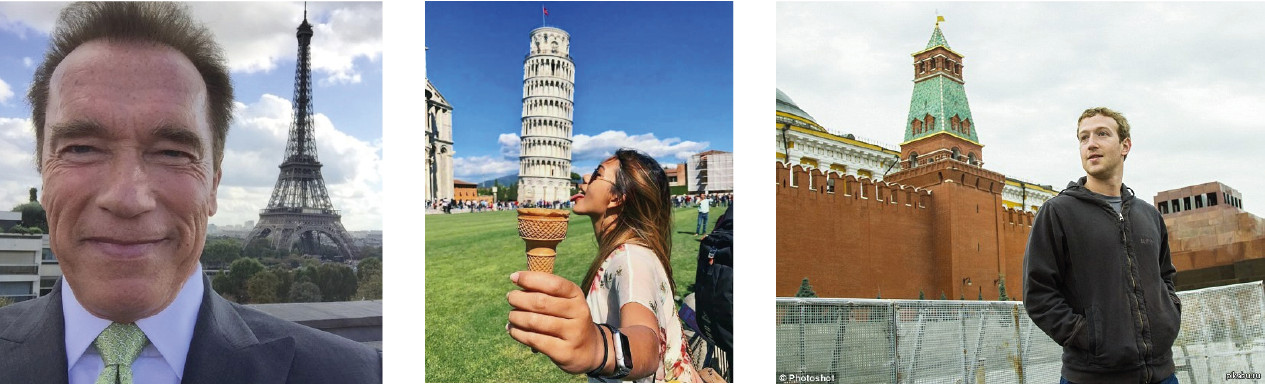
With the advent of mobile phones with high-quality cameras, we started making more and more pictures and videos of bright and memorable moments in our lives. Many of us have photo archives that extend back over decades and comprise thousands of pictures which makes them increasingly difficult to navigate through. Just remember how long it took to find a picture of interest just a few years ago.
随着带有高质量相机的手机的问世,我们开始制作越来越多的照片和视频,这些照片和视频充满了我们生活中令人难忘的瞬间。 我们中许多人的照片档案可以追溯到几十年前,其中包含成千上万张图片,这使它们越来越难以浏览。 只要记住几年前才花了多长时间找到感兴趣的图片。
One of Mail.ru Cloud’s objectives is to provide the handiest means for accessing and searching your own photo and video archives. For this purpose, we at Mail.ru Computer Vision Team have created and implemented systems for smart image processing: search by object, by scene, by face, etc. Another spectacular technology is landmark recognition. Today, I am going to tell you how we made this a reality using Deep Learning.
Mail.ru Cloud的目标之一是提供最方便的方法来访问和搜索您自己的照片和视频档案。 为此,我们Mail.ru计算机视觉团队创建并实施了用于智能图像处理的系统:按对象,按场景,按面部等进行搜索。另一项引人注目的技术是地标识别。 今天,我将告诉您我们如何使用深度学习将其变为现实。
Imagine the situation: you return from your vacation with a load of photos. Talking to your friends, you are asked to show a picture of a place worth seeing, like palace, castle, pyramid, temple, lake, waterfall, mountain, and so on. You rush to scroll your gallery folder trying to find one that is really good. Most likely, it is lost amongst hundreds of images, and you say you will show it later.
想象一下这种情况:您从度假回来时带着大量照片。 与您的朋友交谈,要求您显示一张值得一看的地方的图片,例如宫殿,城堡,金字塔,寺庙,湖泊,瀑布,山脉等。 您急着滚动图库文件夹,试图找到一个确实不错的文件夹。 最有可能的是,它在数百张图像中丢失了,您说稍后再显示。
We solve this problem by grouping user photos in albums. This will let you find pictures you need just in few clicks. Now we have albums compiled by face, by object and by scene, and also by landmark.
我们通过将相册中的用户照片分组来解决此问题。 这使您只需单击几下即可找到所需的图片。 现在,我们有了按面Kong,按对象和按场景以及按地标编译的专辑。
Photos with landmarks are essential because they often capture highlights of our lives (journeys, for example). These can be pictures with some architecture or wilderness in the background. This is why we seek to locate such images and make them readily available to users.
具有地标的照片是必不可少的,因为它们经常捕捉我们生活中的亮点(例如,旅途)。 这些可以是带有某些建筑的图片或背景为荒野的图片。 这就是为什么我们寻求定位此类图像并使它们易于用户使用的原因。
地标识别的特点 (Peculiarities of landmark recognition)
There is a nuance here: one does not merely teach a model and cause it to recognize landmarks right away — there are a number of challenges.
这里有一个细微的差别:不仅要教一个模型并使它立即识别地标,还存在许多挑战。
First, we cannot tell clearly what a “landmark” really is. We cannot tell why a building is a landmark, whereas another one beside it is not. It is not a formalized concept, which makes it more complicated to state the recognition task.
首先,我们不能清楚地分辨出什么是“地标”。 我们无法说出建筑物为何是地标,而建筑物旁却不是。 它不是一个形式化的概念,这使得陈述识别任务更加复杂。
Second, landmarks are incredibly diverse. These can be buildings of historical or cultural value, like a temple, palace or castle. Alternatively, these may be all kinds of monuments. Or natural features: lakes, canyons, waterfalls and so on. Also, there is a single model that should be able to find all those landmarks.
其次,地标的多样性令人难以置信。 这些可以是具有历史或文化价值的建筑物,例如寺庙,宫殿或城堡。 或者,这些可能是各种各样的纪念碑。 或自然特征:湖泊,峡谷,瀑布等。 同样,只有一个模型应该能够找到所有这些地标。
Third, images with landmarks are extremely few. According to our estimations, they account for only 1 to 3 percents of user photos. That is why we can’t afford to make mistakes in recognition because if we show somebody a photograph without a landmark, it will be quite obvious and will cause an adverse reaction. Or, conversely, imagine that you show a picture with a place of interest in New York to a person who has never been to the United States. Thus, the recognition model should have low FPR (false positive rate).
第三,具有地标的图像极少。 根据我们的估计,它们仅占用户照片的1-3%。 这就是为什么我们不能在识别上犯错误,因为如果我们向某人展示没有地标的照片,那将是显而易见的,并且会引起不良React。 相反,或者想象一下,您向从未去过美国的人展示了纽约感兴趣的图片。 因此,识别模型应具有较低的FPR(误报率)。
Fourth, around 50 % of users or even more usually disable geo data saving. We need to take this into account and use only the image itself to identify the location. Today, most of the services able to handle landmarks in some way use geodata from image properties. However, our initial requirements were more stringent.
第四,大约50%甚至更多的用户通常禁用地理数据保存。 我们需要考虑到这一点,仅使用图像本身来标识位置。 如今,大多数能够以某种方式处理地标的服务都使用来自图像属性的地理数据。 但是,我们的最初要求更加严格。
Now let me show you some examples.
现在,让我向您展示一些示例。
Here are three look-alike objects, three Gothic cathedrals in France. On the left is Amiens cathedral, the one in the middle is Reims cathedral, and Notre-Dame de Paris is on the right.
这是三个相似的物件,法国的三个哥特式大教堂。 左边是亚眠大教堂,中间是兰斯大教堂,右边是巴黎圣母院。
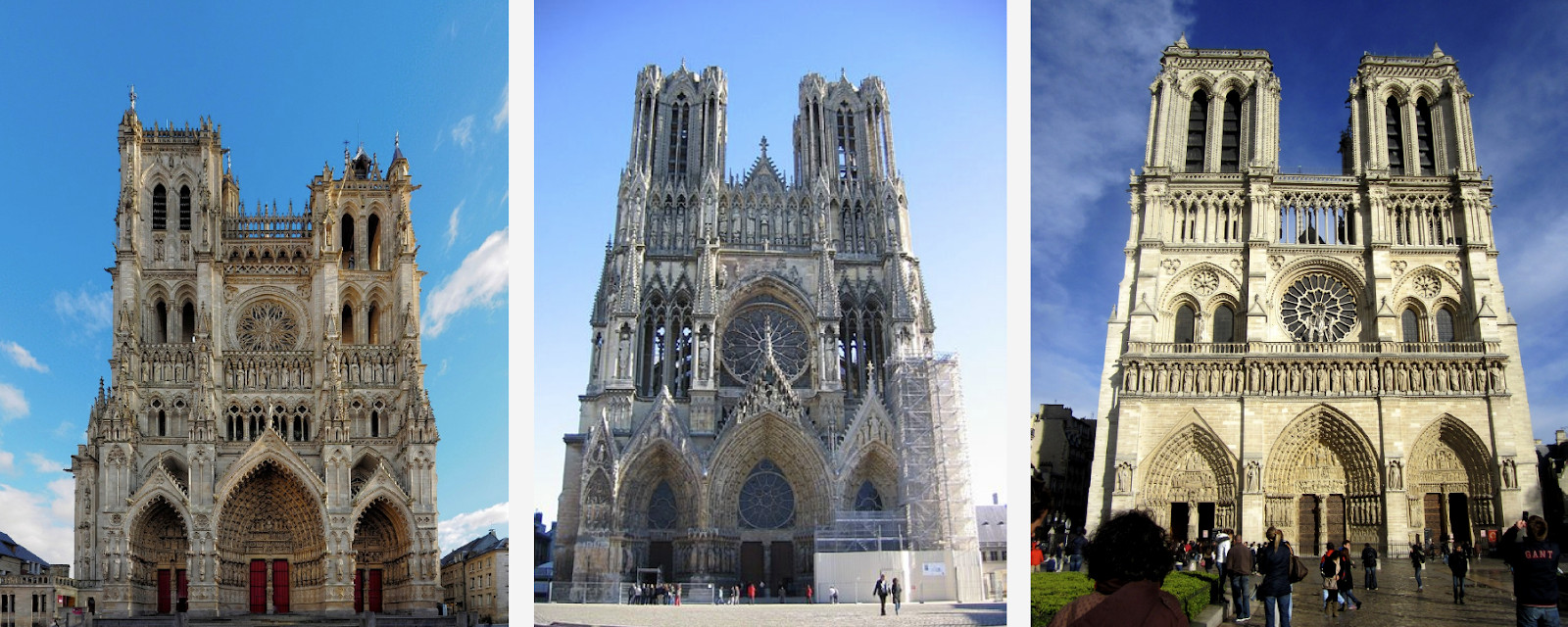
Even a human needs some time to look closely and see that these are different cathedrals, but the engine should be able to do the same, and even faster than a human does.
即使是人类,也需要一些时间仔细观察,才能发现它们是不同的大教堂,但是引擎应该能够做到相同,甚至比人类更快。
Here is another challenge: all the three photos here feature Notre-Dame de Paris shot from different angles. The photos are quite different, but they still need to be recognized and retrieved.
这是另一个挑战:这里的三张照片都以巴黎圣母院的不同角度拍摄。 这些照片有很大的不同,但是仍然需要对其进行识别和检索。
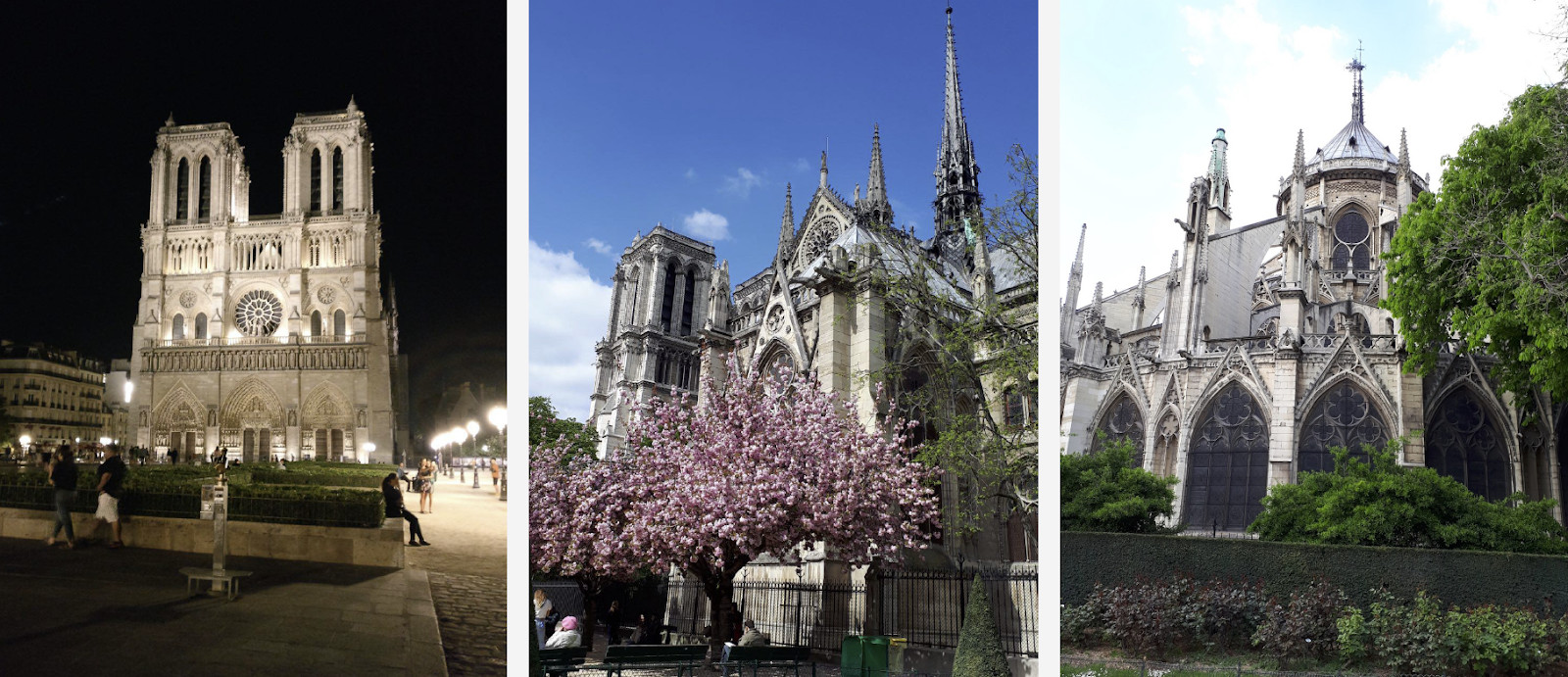
Natural features are entirely different from architecture. On the left is Caesarea in Israel, on the right is Englischer Garten in Munich.
自然特征与建筑完全不同。 左边是以色列的凯撒利亚,右边是慕尼黑的Englischer Garten。
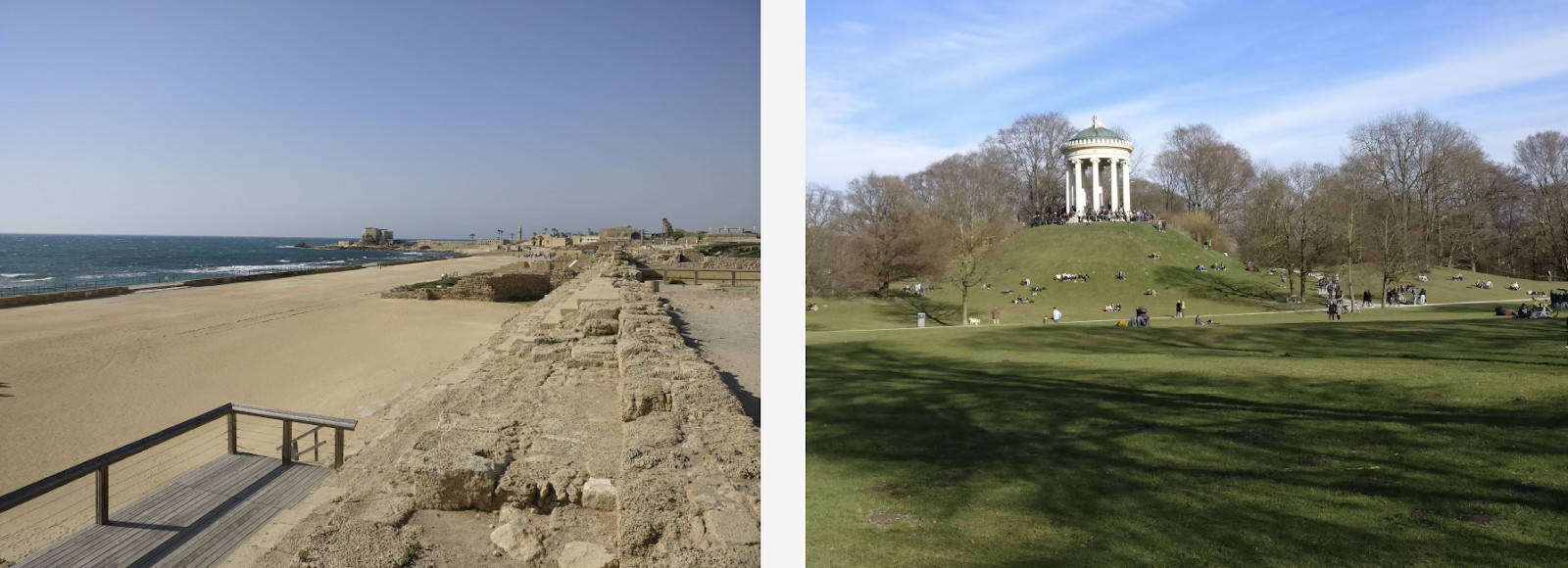
These photos give the model very few clues to guess.
这些照片很少给模型提供线索。
我们的方法 (Our method)
Our method is based completely on deep convolutional neural networks. The training strategy we chose was so-called curriculum learning which means learning in several steps. To achieve greater efficiency both with and without geo data available, we made a specific inference. Let me tell you about each step in more detail.
我们的方法完全基于深度卷积神经网络。 我们选择的培训策略是所谓的课程学习,即分几步学习。 为了在有或没有可用地理数据的情况下均能达到更高的效率,我们做了一个具体的推断。 让我详细介绍每个步骤。
资料集 (Data set)
Data is the fuel of machine learning. First of all, we had to get together data set to teach the model.
数据是机器学习的动力。 首先,我们必须汇总数据集来教授该模型。
We divided the world into 4 regions, each being used at a specific step in the learning process. Then, we selected countries in each region, picked a list of cities for each country, and collected a bank of photos. Below are some examples.
我们将世界分为4个区域,每个区域都用于学习过程中的特定步骤。 然后,我们选择了每个地区的国家/地区,为每个国家/地区选择了一个城市列表,并收集了大量照片。 以下是一些示例。
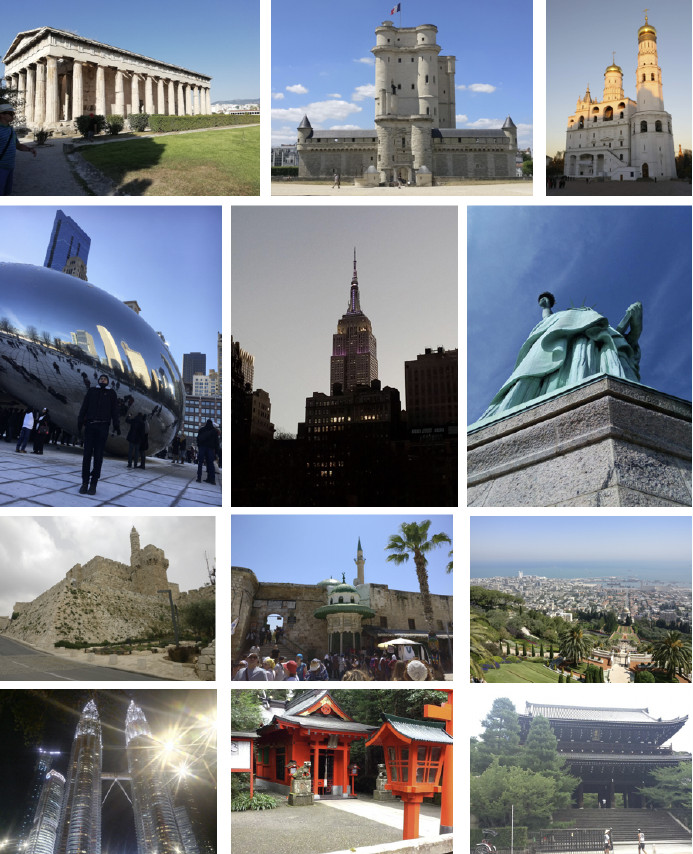
First, we attempted to make our model learn from the database obtained. The results were poor. Our analysis showed that the data was dirty. There was too much noise interfering with recognition of each landmark. What were we to do? It would be expensive, cumbersome, and not too wise to review all the bulk of data manually. So, we devised a process for automatic database cleaning where manual handling is used only at one step: we handpicked 3 to 5 reference photographs for each landmark which definitely showed the desired object at a more or less suitable angle. It works fast enough because the amount of such reference data is small as compared to the whole database. Then automatic cleaning based on deep convolutional neural networks is performed.
首先,我们试图使我们的模型从获得的数据库中学习。 结果很差。 我们的分析表明,数据很脏。 太多的噪音干扰了每个地标的识别。 我们该怎么办? 手动查看所有大批量数据将非常昂贵,麻烦且不明智。 因此,我们设计了一种自动清理数据库的过程,该过程只在一个步骤中使用了手动处理:我们为每个地标选择了3至5张参考照片,这些照片肯定以或多或少合适的角度显示了所需的对象。 它之所以足够快,是因为此类参考数据的数量比整个数据库少。 然后基于深度卷积神经网络进行自动清洗。
Further on, I am going to use the term “embedding” by which I mean the following. We have a convolutional neural network. We trained it to classify objects, then we cut off the last classifying layer, picked some images, had them analyzed by the network, and obtained a numeric vector at the output. This is what I will call embedding.
进一步,我将使用术语“嵌入”,其含义如下。 我们有一个卷积神经网络。 我们训练了它以对对象进行分类,然后我们切断了最后一个分类层,选择了一些图像,通过网络对其进行了分析,并在输出处获得了数值矢量。 这就是我所说的嵌入。
As I said before, we arranged our learning process in several steps corresponding to parts of our database. So, first, we take either neural network from the preceding step or the initializing network.
正如我之前所说,我们将学习过程分为几个步骤,与数据库的各个部分相对应。 因此,首先,我们采用上一步的神经网络或初始化网络。
We have reference photos of a landmark, process them by the network and obtain several embeddings. Now we can proceed to data cleaning. We take all pictures from data set for the landmark and have each one processed by the network as well. We obtain some embeddings and determine the distance to reference embeddings for each one. Then, we determine the average distance and, if it exceeds some threshold which is a parameter of the algorithm, treat the object as non-landmark. If the average distance is less than the threshold, we keep the photograph.
我们具有地标的参考照片,通过网络对其进行处理并获得多个嵌入。 现在我们可以进行数据清理了。 我们从地标数据集中获取所有图片,并由网络进行处理。 我们获得一些嵌入,并确定每个嵌入到参考嵌入的距离。 然后,我们确定平均距离,如果平均距离超过算法的某个阈值,则将该对象视为非地标。 如果平均距离小于阈值,则保留照片。
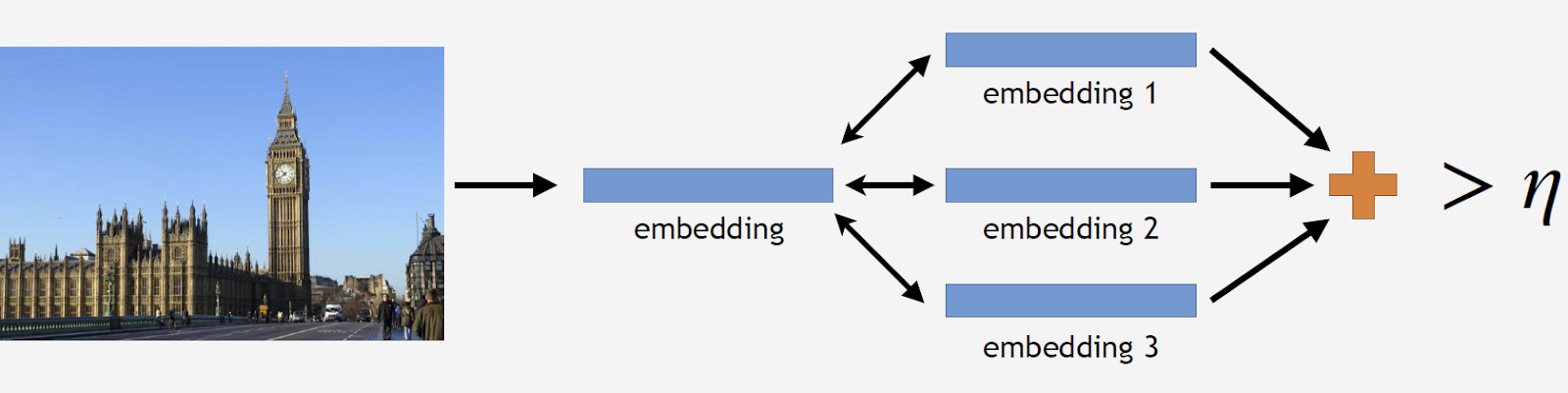
As a result, we had a database which contained over 11 thousand landmarks from over 500 cities in 70 countries, more than 2.3 million photos. Remember that the major part of photographs has no landmarks at all. We need to tell it to our models somehow. For this reason, we added 900 thousand photos without landmarks to our database and trained our model with the resulting data set.
结果,我们有了一个数据库,其中包含来自70个国家/地区的500多个城市的11000多个地标,超过230万张照片。 请记住,照片的主要部分根本没有地标。 我们需要以某种方式将其告知我们的模型。 因此,我们向数据库中添加了90万张没有地标的照片,并使用结果数据集训练了模型。
We introduced an offline test to measure learning quality. Given that landmarks occur only in 1 to 3 % of all photos, we manually compiled a set of 290 pictures which did show a landmark. Those photos were quite diverse and complex, with a large number of objects shot from different angles to make the test as difficult as possible for the model. Following the same pattern, we picked 11 thousand photographs without landmarks, rather complicated as well, and we tried to find objects that looked much like the landmarks in our database.
我们引入了离线测试来衡量学习质量。 鉴于地标仅出现在所有照片的1-3%中,我们手动编辑了一组290张确实显示地标的照片。 这些照片非常多样且复杂,具有从不同角度拍摄的大量物体,从而使模型的测试尽可能困难。 按照相同的模式,我们选择了11千张没有地标的照片,而且也很复杂,并且我们尝试在数据库中找到看起来很像地标的对象。
To evaluate learning quality, we measure our model’s accuracy using photos both with and without landmarks. These are our two main metrics.
为了评估学习质量,我们使用带有和不带有界标的照片来衡量模型的准确性。 这是我们的两个主要指标。
现有方法 (Existing approaches)
There is relatively few information about landmark recognition in the literature. Most solutions are based on local features. The main idea is that we have some query picture and a picture from the database. Local features — key points — are found and then matched. If the number of matches is large enough, we conclude that we have found a landmark.
文献中关于地标识别的信息相对较少。 大多数解决方案基于本地功能。 主要思想是我们有一些查询图片和来自数据库的图片。 找到并匹配局部特征(关键点)。 如果匹配数目足够大,则可以得出结论,我们找到了一个里程碑。
Currently, the best method is DELF (deep local features) offered by Google, which combines local features matching with deep learning. By having an input image processed by the convolutional network, we obtain some DELF features.
当前,最好的方法是Google提供的DELF(深度本地特征),它将本地特征与深度学习相结合。 通过卷积网络处理输入图像,我们获得了一些DELF特征。
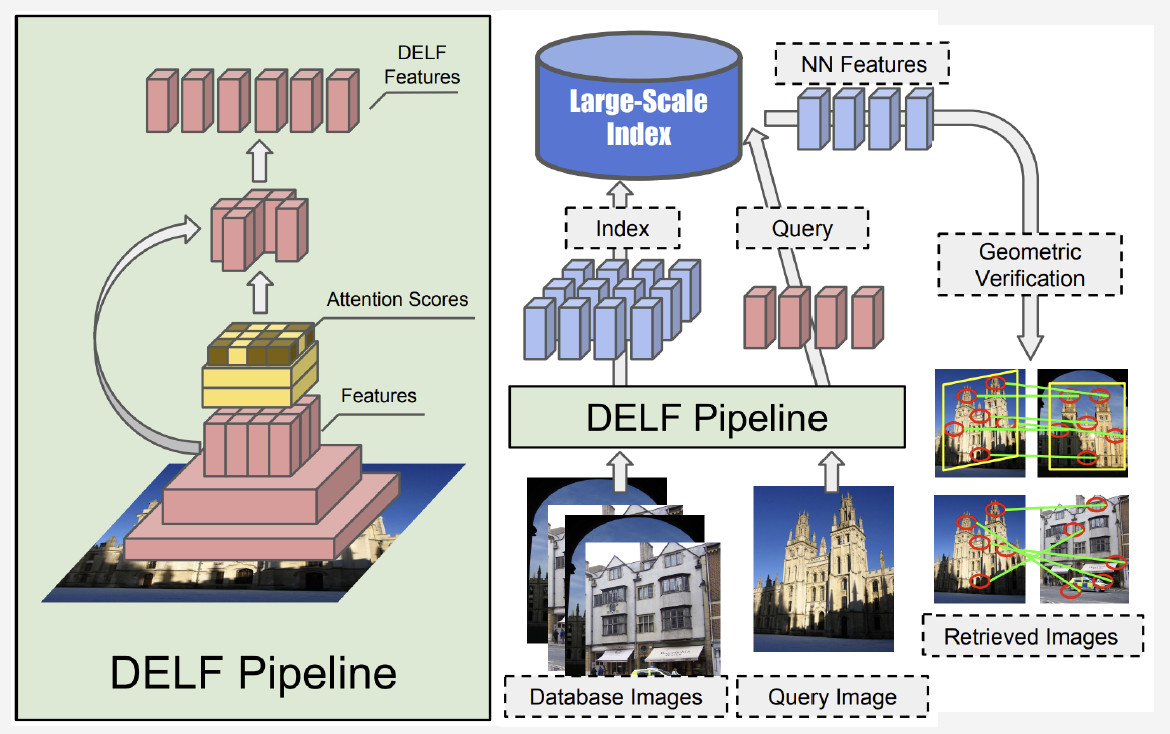
How does landmark recognition work? We have a bank of photos and an input image, and we want to know whether it shows a landmark or not. By running DELF network of all photos, corresponding features for the database and the input image can be obtained. Then we perform a search by the nearest-neighbor method and obtain candidate images with features at the output. We use geometrical verification to match the features: if successful, we conclude that the picture shows a landmark.
地标识别如何工作? 我们有一堆照片和一个输入图像,我们想知道它是否显示地标。 通过运行所有照片的DELF网络,可以获得数据库和输入图像的相应功能。 然后,我们通过最邻近方法执行搜索,并在输出处获得具有特征的候选图像。 我们使用几何验证来匹配特征:如果成功,我们得出的结论是图片显示了地标。
卷积神经网络 (Convolutional neural network)
Pre-training is crucial for Deep Learning. So we used a database of scenes to pre-train our neural network. Why this way? A scene is a multiple object which comprises a large number of other objects. Landmark is an instance of a scene. By pre-training the model with such a database, we can give it an idea of some low-level features which can be then generalized for successful landmark recognition.
预培训对于深度学习至关重要。 因此,我们使用了一个场景数据库来预先训练我们的神经网络。 为什么这样呢? 场景是包含大量其他对象的多个对象。 地标是场景的一个实例。 通过使用这样的数据库对模型进行预训练,我们可以为它提供一些低级功能的概念,然后将其概括以成功实现地标识别。
We used a neural network from the Residual network family as the model. The critical difference of such networks is that they use a residual block that includes skip connection which allows a signal to jump over layers with weights and pass freely. Such architecture makes it possible to train deep networks with a high level of quality and control vanishing gradient effect, which is essential for training.
我们使用残差网络家族的神经网络作为模型。 这种网络的关键区别在于,它们使用的剩余块包括跳过连接,该跳过连接允许信号以权重跳过层并自由通过。 这样的体系结构使得可以训练高质量的深度网络并控制消失的梯度效果,这对于训练至关重要。
Our model is Wide ResNet-50-2, a version of ResNet-50 where the number of convolutions in the internal bottleneck block is doubled.
我们的模型是Wide ResNet-50-2,这是ResNet-50的版本,内部瓶颈块中的卷积数量翻了一番。
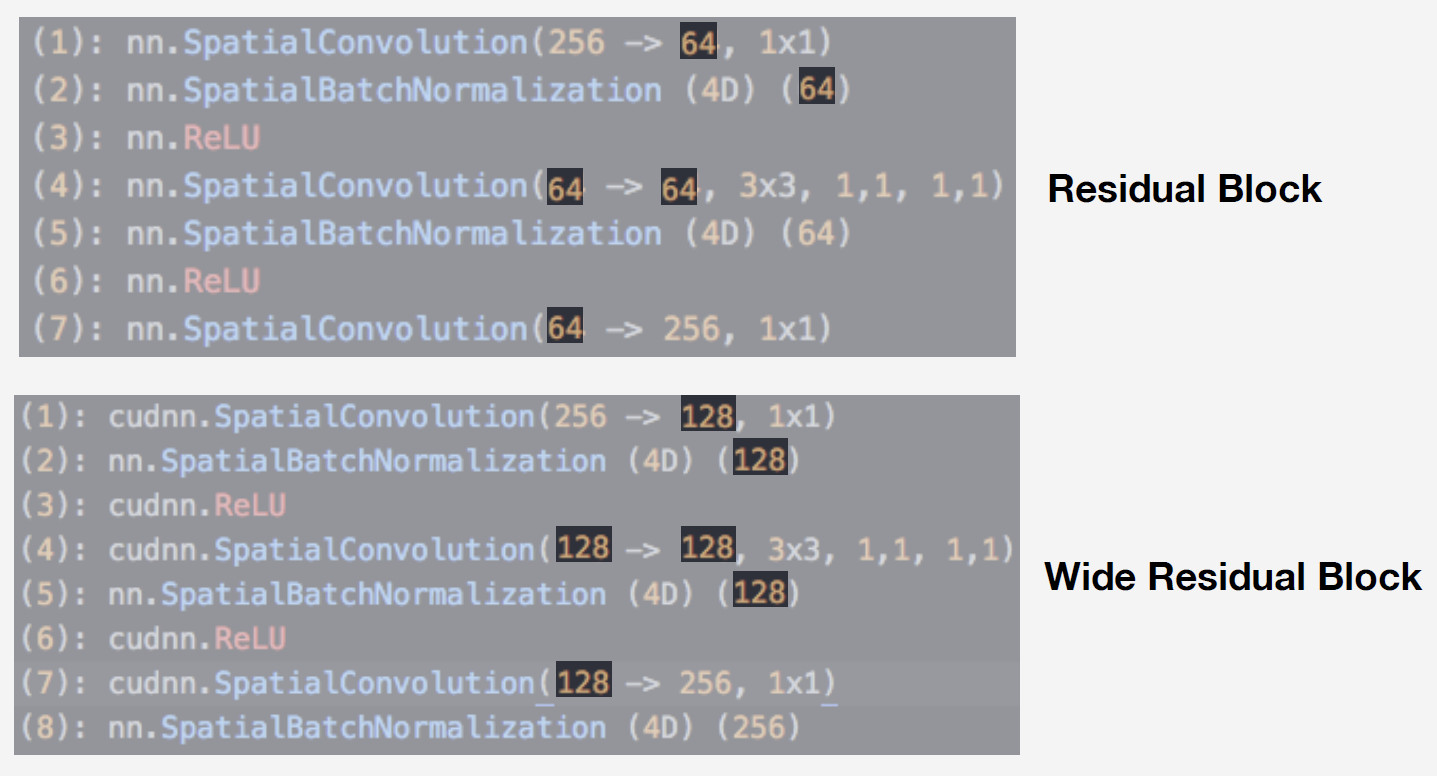
The network performs very well. We tested it with our scene database, and here are the results:
网络性能很好。 我们使用场景数据库对其进行了测试,结果如下:
Model | Top 1 err | Top 5 err |
---|---|---|
ResNet-50 | 46.1 % | 15.7 % |
ResNet-200 | 42.6 % | 12.9 % |
SE-ResNext-101 | 42 % | 12.1 % |
WRN-50-2 (fast!) | 41.8 % | 11.8 % |
模型 | 前1错误 | 前5错误 |
---|---|---|
ResNet-50 | 46.1% | 15.7% |
ResNet-200 | 42.6% | 12.9% |
SE-ResNext-101 | 42% | 12.1% |
WRN-50-2(快速!) | 41.8% | 11.8% |
Wide ResNet worked nearly twice as fast as ResNet-200. After all, it is running speed that is crucial for production. Given all these considerations, we chose Wide ResNet-50-2 as our main neural network.
Wide ResNet的运行速度几乎是ResNet-200的两倍。 毕竟,运行速度对于生产至关重要。 考虑到所有这些因素,我们选择Wide ResNet-50-2作为我们的主要神经网络。
训练 (Training )
We need a loss function to train our network. We decided to use metric learning approach to pick it: a neural network is trained so that items of the same class flock to one cluster, while clusters for different classes are to be spaced apart as much as possible. For landmarks, we used Center loss which pulls elements of one class towards some center. An important feature of this approach is that it does not require negative sampling, which becomes a rather difficult thing to do at later epochs.
我们需要损失函数来训练我们的网络。 我们决定使用度量学习方法进行选择:训练一个神经网络,使同一类别的项目聚集到一个群集中,而将不同类别的群集尽可能地隔开。 对于地标,我们使用中心损失将一类元素拉向某个中心。 这种方法的一个重要特征是它不需要负采样,这在以后的时代变得相当困难。
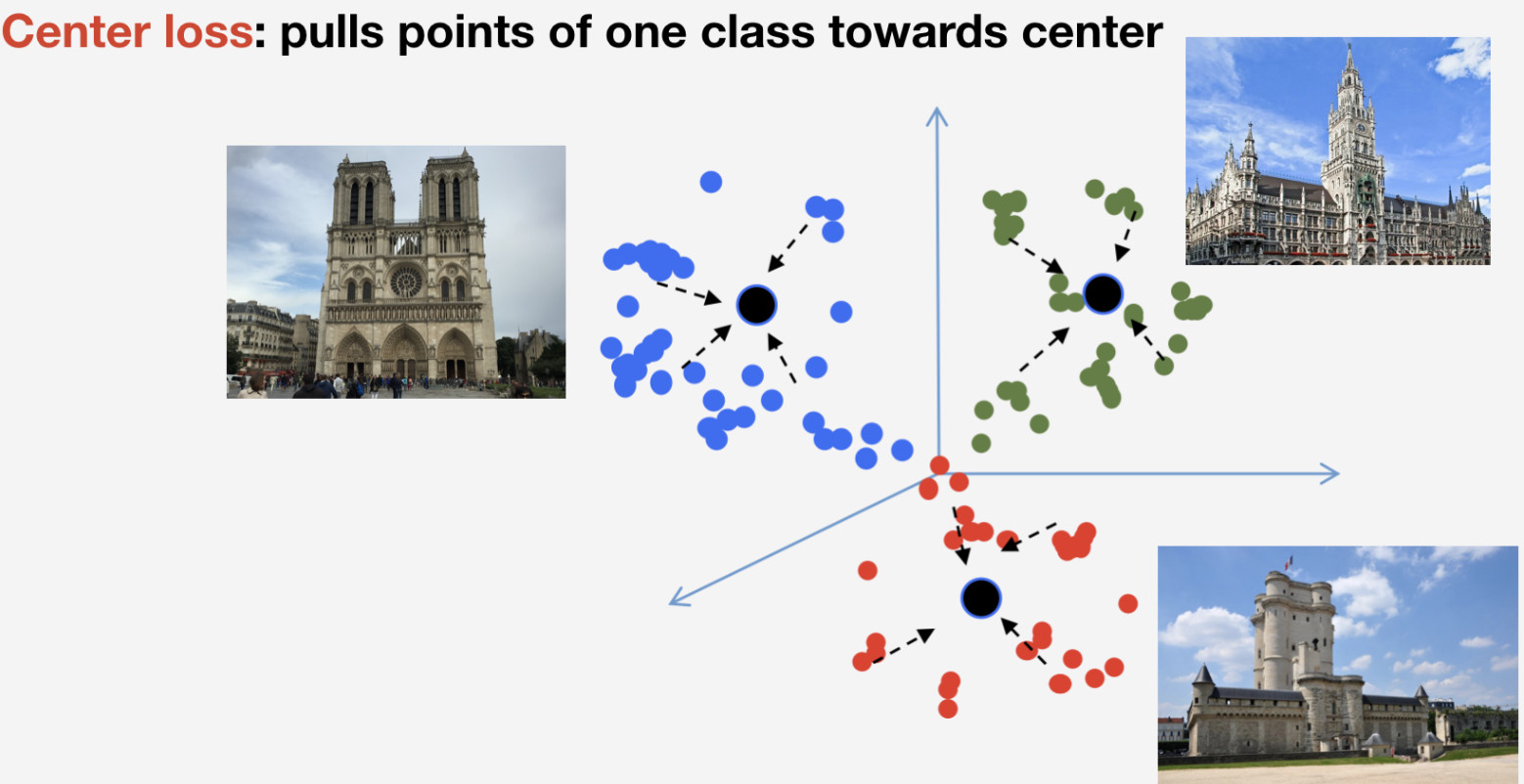
Remember that we have n classes of landmarks and one more “non-landmark” class for which Center loss is not used. We imply that a landmark is one and the same object, and it has structure, so it makes sense to determine its center. As to non-landmark, it can refer to whatever, so it makes no sense to determine center for it.
请记住,我们有n个地标类和另外一个不使用中心损失的“非地标”类。 我们暗示地标是一个相同的对象,并且具有结构,因此确定其中心是有意义的。 至于非地标,它可以指代任何东西,因此确定其中心毫无意义。
We then put all this together, and there is our model for training. It comprises three major parts:
然后,我们将所有这些放在一起,就有了我们的培训模型。 它包括三个主要部分:
- Wide ResNet 50-2 convolutional neural network pre-trained with a database of scenes; 使用场景数据库预先训练的Wide ResNet 50-2卷积神经网络;
- Embedding part comprising a fully connected layer and Batch norm layer; 嵌入部分包括完全连接的层和批处理规范层;
- Classifier which is a fully connected layer, followed by a pair comprised of Softmax loss and Centre loss. 分类器是一个完全连接的层,后面是由Softmax损耗和Center损耗组成的一对。

As you remember, our database is split into 4 parts by region. We use these 4 parts in a curriculum learning paradigm. We have a current dataset, and at each stage of learning, we add another part of the world to obtain a new dataset for training.
您还记得,我们的数据库按地区分为4个部分。 我们在课程学习范例中使用这4个部分。 我们拥有一个当前的数据集,并且在学习的每个阶段,我们都会增加世界的另一部分,以获得用于训练的新数据集。
The model comprises three parts, and we use a specific learning rate for each one in the training process. This is required for the network to be able both to learn landmarks from a new dataset part we have added and to remember data already learned. Many experiments proved this approach to be the most efficient.
该模型包括三个部分,我们在训练过程中对每个部分使用特定的学习率。 网络必须具备此功能,才能从我们添加的新数据集部分中学习地标并记住已经学习的数据。 许多实验证明这种方法是最有效的。
So, we have trained our model. Now we need to realize how it works. Let us use class activation map to find the part of the image to which our neural network reacts most readily. The picture below shows input images in the first row, and the same images overlaid with class activation map from the network we have trained at the previous step are shown in the second row.
因此,我们已经训练了模型。 现在我们需要意识到它是如何工作的。 让我们使用类激活图来查找神经网络最容易React的图像部分。 下图显示了第一行中的输入图像,第二行中显示了我们在上一步中训练的网络中覆盖有课程激活图的相同图像。
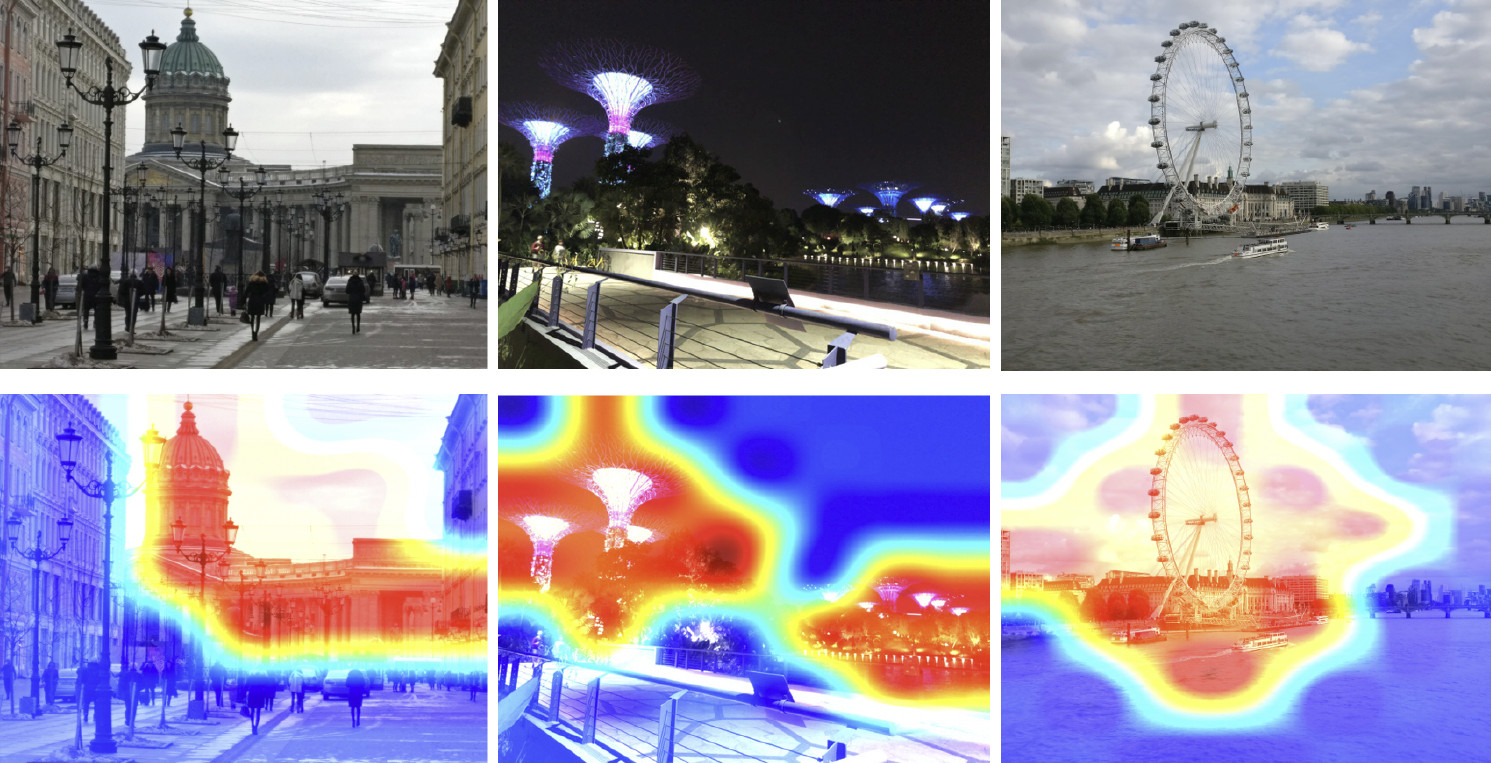
Heat map shows which parts of the image are attended more by the network. As shown by class activation map, our neural network has learned the concept of landmark successfully.
热图显示网络更多地关注图像的哪些部分。 如类激活图所示,我们的神经网络已成功学习了地标的概念。
推理 (Inference)
Now we need to use this knowledge somehow to get things done. Since we have used Center loss for training, in the case of inference, it appears to be quite logical to determine centroids for landmarks too.
现在,我们需要以某种方式使用这些知识来完成任务。 由于我们已使用中心损失进行训练,因此在推断的情况下,确定地标的质心似乎也很合逻辑。
To do this, we take a part of images from the training set for some landmark, say, the Bronze Horseman in Saint Petersburg. Then we have them processed by the network, obtain embeddings, average out, and derive a centroid.
为此,我们从训练集中获取了一些具有里程碑意义的图像,例如圣彼得堡的青铜骑士。 然后我们对它们进行网络处理,获得嵌入,取平均值,并得出质心。
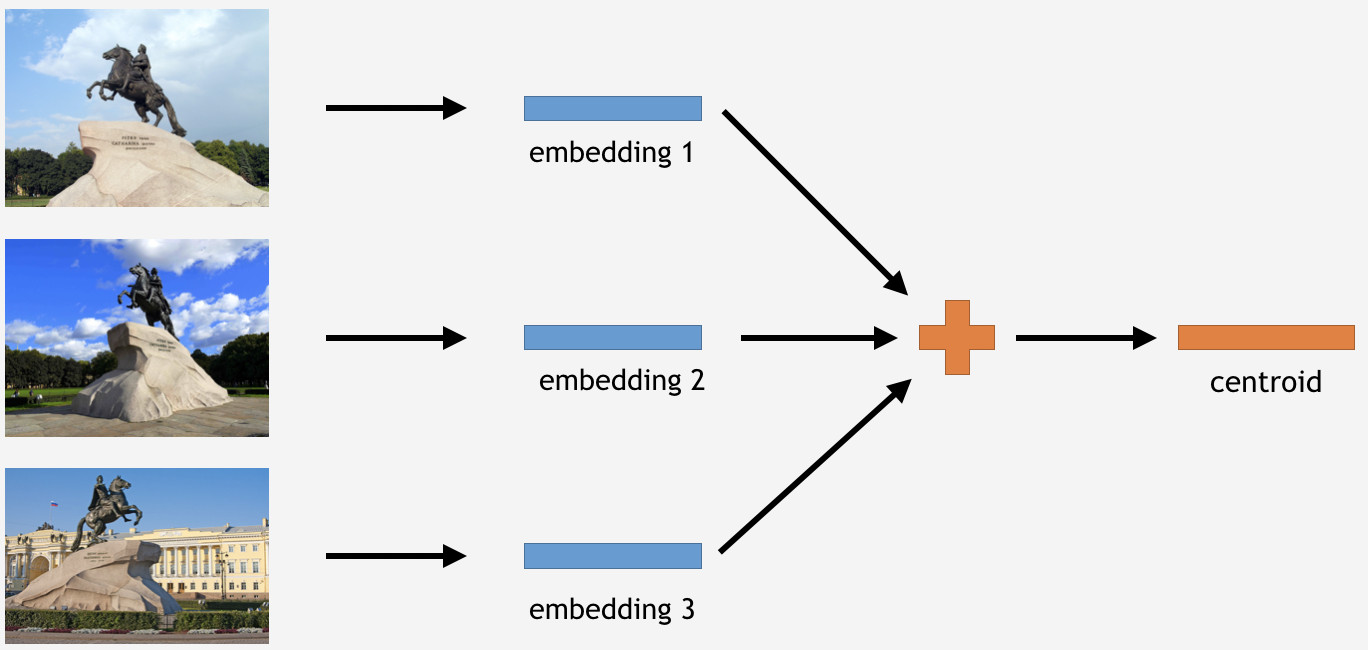
However, here is a question: how many centroids per landmark it makes sense to derive? Initially, it appeared to be clear and logical to say: one centroid. Not exactly, as it turned out. We initially decided to make a single centroid too, and the result was not bad. So why several centroids?
但是,这里有一个问题:每个地标派生多少个质心才有意义? 最初,似乎很清楚且合乎逻辑地说:一个质心。 事实并非如此。 我们最初也决定制作一个质心,结果还不错。 那为什么要几个质心呢?
First, the data we have is not so clean. Though we have cleaned the dataset, we removed only obvious waste data. However, there still could be images not obviously waste but adversely affecting the result.
首先,我们拥有的数据不是那么干净。 尽管我们已经清理了数据集,但仅删除了明显的浪费数据。 但是,仍然可能存在图像不明显浪费但对结果产生不利影响的情况。
For example, I have a landmark class Winter Palace in Saint Petersburg. I want to derive a centroid for it. However, its dataset includes some photos with Palace Square and General Headquarters arch, because these objects are close to each other. If the centroid is to be determined for all images, the result will be not so stable. What we need to do is to cluster somehow their embeddings derived from the neural network, take only the centroid that deals with the Winter Palace, and average out using the resulting data.
例如,我在圣彼得堡有一个地标类的冬宫。 我想为此得出一个质心。 但是,其数据集包含一些带有宫殿广场和总部大楼拱门的照片,因为这些对象彼此靠近。 如果要为所有图像确定质心,则结果将不太稳定。 我们需要做的是以某种方式对它们从神经网络中得到的嵌入进行聚类,仅获取处理冬宫的质心,然后使用所得数据求平均值。
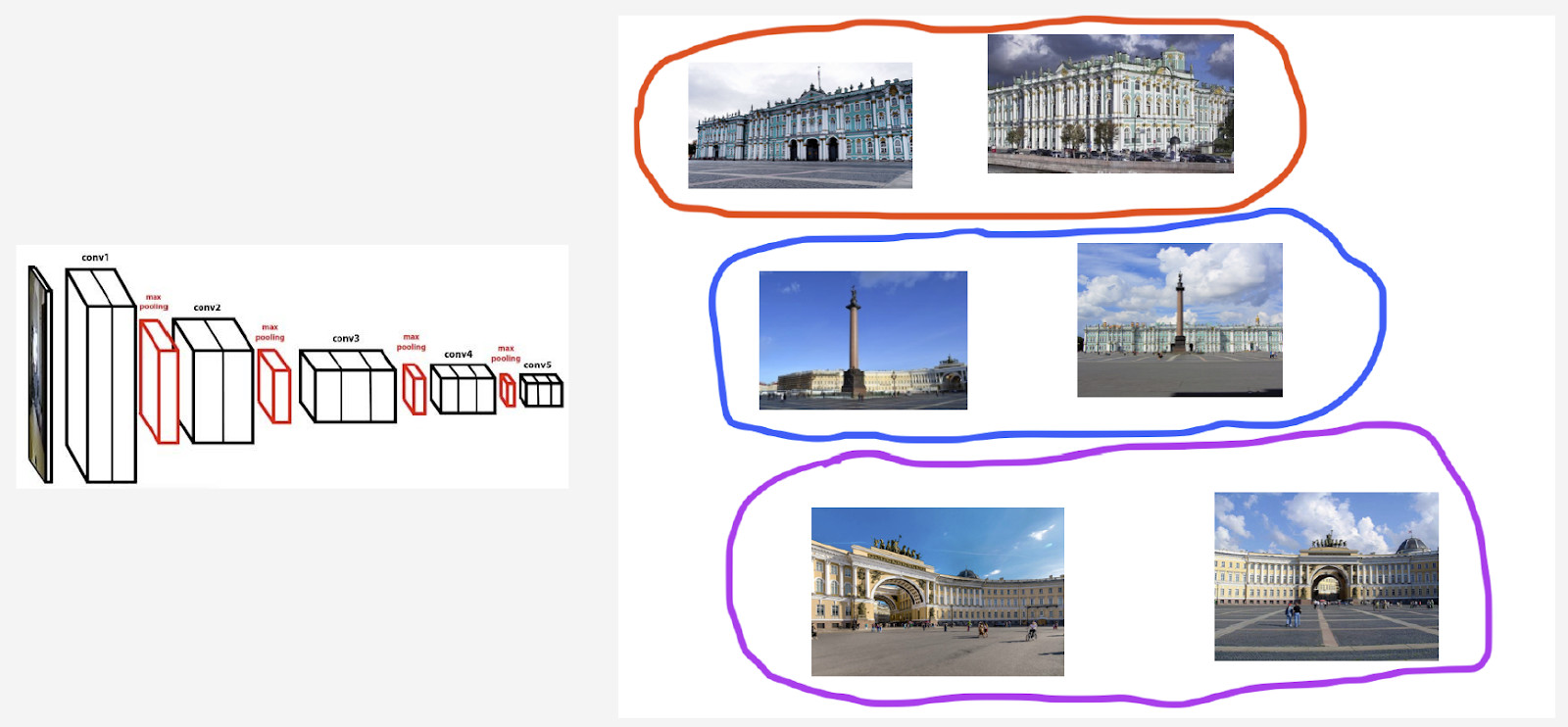
Second, photographs might have been taken from different angles.
其次,照片可能是从不同角度拍摄的。
Here is an example of such behavior illustrated with the Belfry of Bruges. Two centroids have been derived for it. In the top row in the image, there are those photos which are closer to the first centroid, and in the second row — the ones that are closer to the second centroid.
这是布鲁日钟楼所举例说明的这种行为。 为此已经导出了两个质心。 在图像的第一行中,有一些照片更靠近第一个质心,而在第二行中有一些照片更靠近第二个质心。
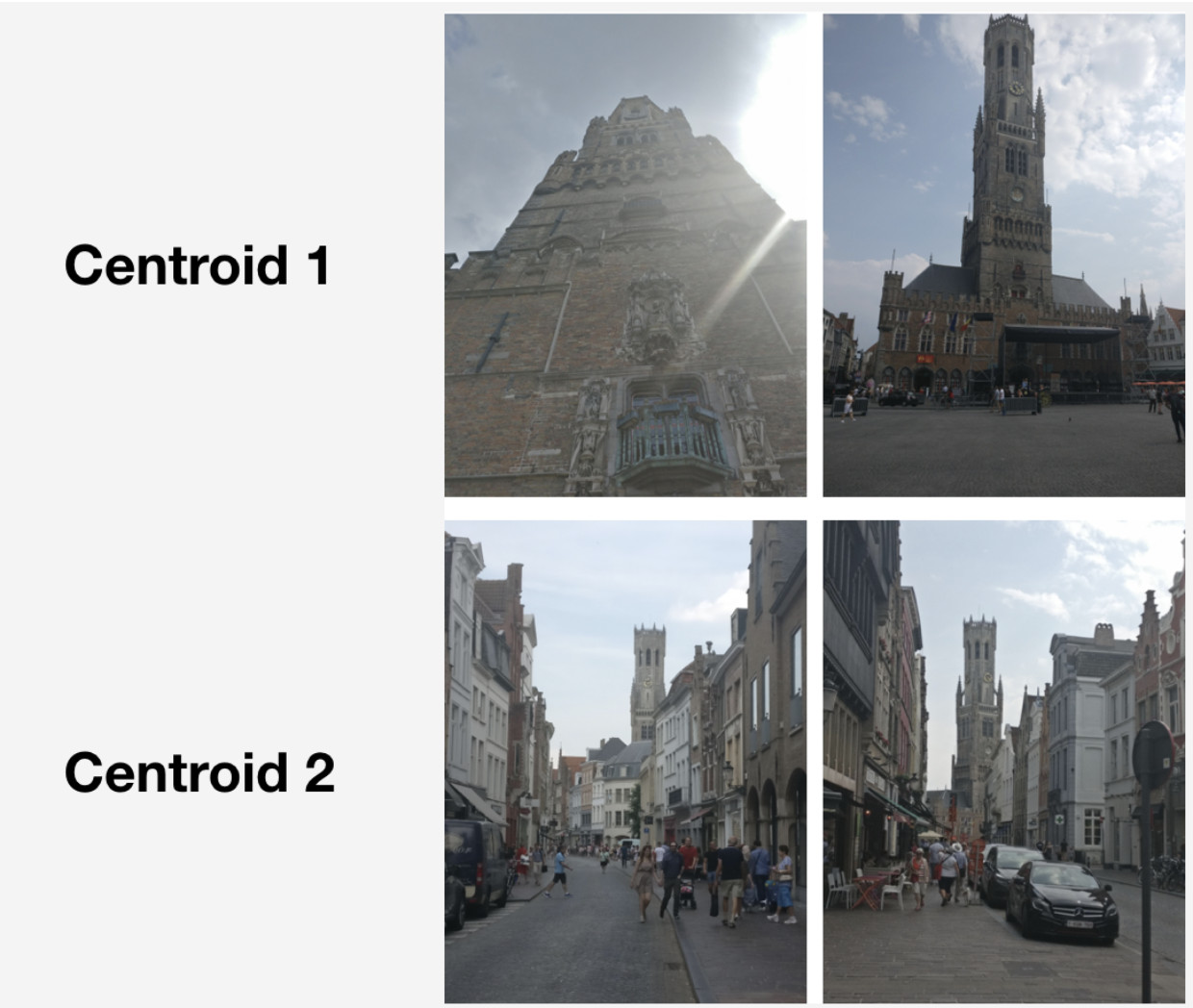
The first centroid deals with more “grand” photographs which were taken at the marketplace in Bruges at short range. The second centroid deals with photographs taken from a distance in particular streets.
第一个质心涉及更多在布鲁日市场上短距离拍摄的“大”照片。 第二个质心处理从特定街道远距离拍摄的照片。
As it turns out, by deriving several centroids per landmark class, we can reflect on inference different camera angles for that landmark.
事实证明,通过为每个地标类推导几个质心,我们可以反思推断出该地标的不同相机角度。
So, how do we obtain those sets for deriving centroids? We apply hierarchical clustering (complete link) to datasets for each landmark. We use it to find valid clusters from which centroids are to be derived. By valid clusters we mean those comprising at least 50 photographs as a result of clustering. The other clusters are rejected. As a result, we obtained around 20 % of landmarks with more than one centroid.
那么,我们如何获得推导质心的那些集合? 我们将分层聚类(完整链接)应用于每个地标的数据集。 我们使用它来找到要从其派生质心的有效簇。 有效聚类是指由于聚类而至少包含50张照片的聚类。 其他集群被拒绝。 结果,我们获得了大约20%具有多个质心的地标。
Now to inference. It is obtained in two steps: firstly, we feed the input image to our convolutional neural network and obtain embedding, and then match the embedding with centroids using dot product. If images have geo data, we restrict the search to centroids, which refer to landmarks located within a 1x1 km square from the image location. This enables a more accurate search and a lower threshold for subsequent matching. If the resulting distance exceeds the threshold which is a parameter of the algorithm, then we conclude that a photo has a landmark with the maximum dot product value. If it is less, then it is a non-landmark photo.
现在推断。 它是通过两个步骤获得的:首先,将输入图像输入到卷积神经网络并获得嵌入,然后使用点积将嵌入与质心进行匹配。 如果图像具有地理数据,则将搜索限制为质心,质心是指距图像位置1x1 km平方内的地标。 这样可以进行更准确的搜索,并降低后续匹配的阈值。 如果得出的距离超过作为算法参数的阈值,那么我们得出结论,一张照片的地标具有最大的点积值。 如果较小,则为非地标照片。
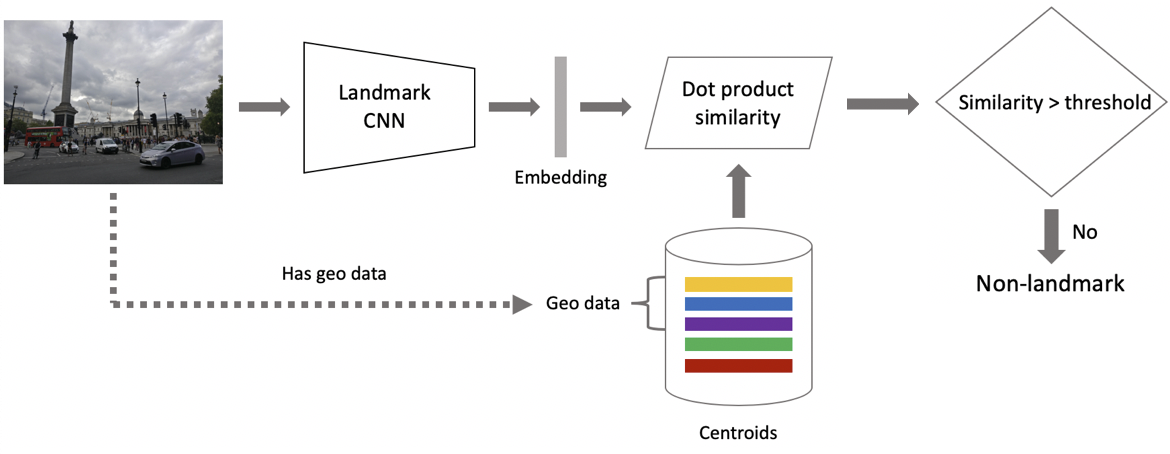
Suppose that a photo has a landmark. If we have geo data, then we use it and derive an answer. If geo data is unavailable, then we run an additional check. When we were cleaning the dataset, we made a set of reference images for each class. We can determine embeddings for them, and then obtain average distance from them to the embedding of the query image. If it exceeds some threshold, then verification is passed, and we bring in metadata and derive a result. It is important to note that we can run this procedure for several landmarks which have been found in an image.
假设一张照片具有地标性。 如果我们有地理数据,则可以使用它并得出答案。 如果地理数据不可用,那么我们将进行其他检查。 清理数据集时,我们为每个类制作了一组参考图像。 我们可以确定它们的嵌入,然后获得它们到查询图像嵌入的平均距离。 如果超过某个阈值,那么将通过验证,然后我们将引入元数据并得出结果。 重要的是要注意,我们可以对图像中找到的几个地标运行此过程。
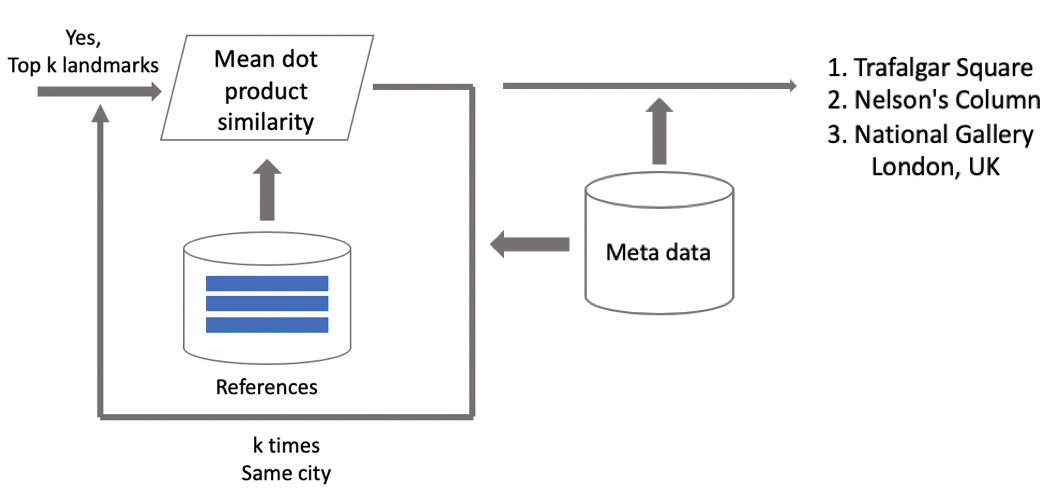
测试结果 (Results of tests)
We compared our model with DELF, for which we took parameters with which it would show the best performance in our test. The results are nearly identical.
我们将模型与DELF进行了比较,为此我们采用了可以在测试中显示最佳性能的参数。 结果几乎相同。
Model | Landmark | Non-landmark |
---|---|---|
Our model | 80 % | 99 % |
DELF | 80.1 % | 99 % |
模型 | 地标 | 非地标 |
---|---|---|
我们的模型 | 80% | 99% |
定义 | 80.1% | 99% |
Then we classified landmarks into two types: frequent (over 100 photographs in the database), which accounted for 87 % of all landmarks in the test, and rare. Our model works well with the frequent ones: 85.3 % precision. With rare landmarks, we had 46 % which was also not bad at all, meaning that our approach worked fairly well even with few data.
然后,我们将地标分为两种类型:频繁(数据库中有100多张照片),占测试中所有地标的87%;稀有。 我们的模型可以很好地处理频繁出现的问题:85.3%的精度。 在地标稀少的情况下,我们有46%的数据还算不错,这意味着即使数据很少,我们的方法也能很好地工作。
Type | Precision | Share of the total number |
---|---|---|
Frequent | 85.3 % | 87 % |
Rare | 46 % | 13 % |
类型 | 精确 | 占总数 |
---|---|---|
频繁的 | 85.3% | 87% |
罕见 | 46% | 13% |
Then we ran A/B test with user photos. As a result, cloud space purchase conversion rate grew by 10 %, mobile app uninstall conversion rate reduced by 3 %, and the number of album views increased by 13 %.
然后,我们使用用户照片进行A / B测试。 结果,云空间购买转换率增加了10%,移动应用程序卸载转换率减少了3%,相册浏览量增加了13%。
Let us compare our speed to DELF’s. With GPU, DELF requires 7 network runs because it uses 7 image scales, while our approach uses only 1. With CPU, DELF uses a longer search by the nearest-neighbor method and a very long geometrical verification. In the end, our method was 15 times faster with CPU. Our approach shows higher speed in both cases, which is crucial for production.
让我们将我们的速度与DELF的速度进行比较。 使用GPU,DELF需要7个网络运行,因为它使用7个图像比例,而我们的方法仅使用1。对于CPU,DELF使用最近邻居方法进行更长的搜索,并且进行非常长的几何验证。 最终,我们的方法使用CPU的速度提高了15倍。 我们的方法在两种情况下均显示出较高的速度,这对于生产至关重要。
结果:假期的回忆 (Results: memories from vacation)
At the beginning of this article, I mentioned a solution for scrolling and finding desired landmark pictures. Here it is.
在本文的开头,我提到了一种滚动和查找所需地标图片的解决方案。 这里是。
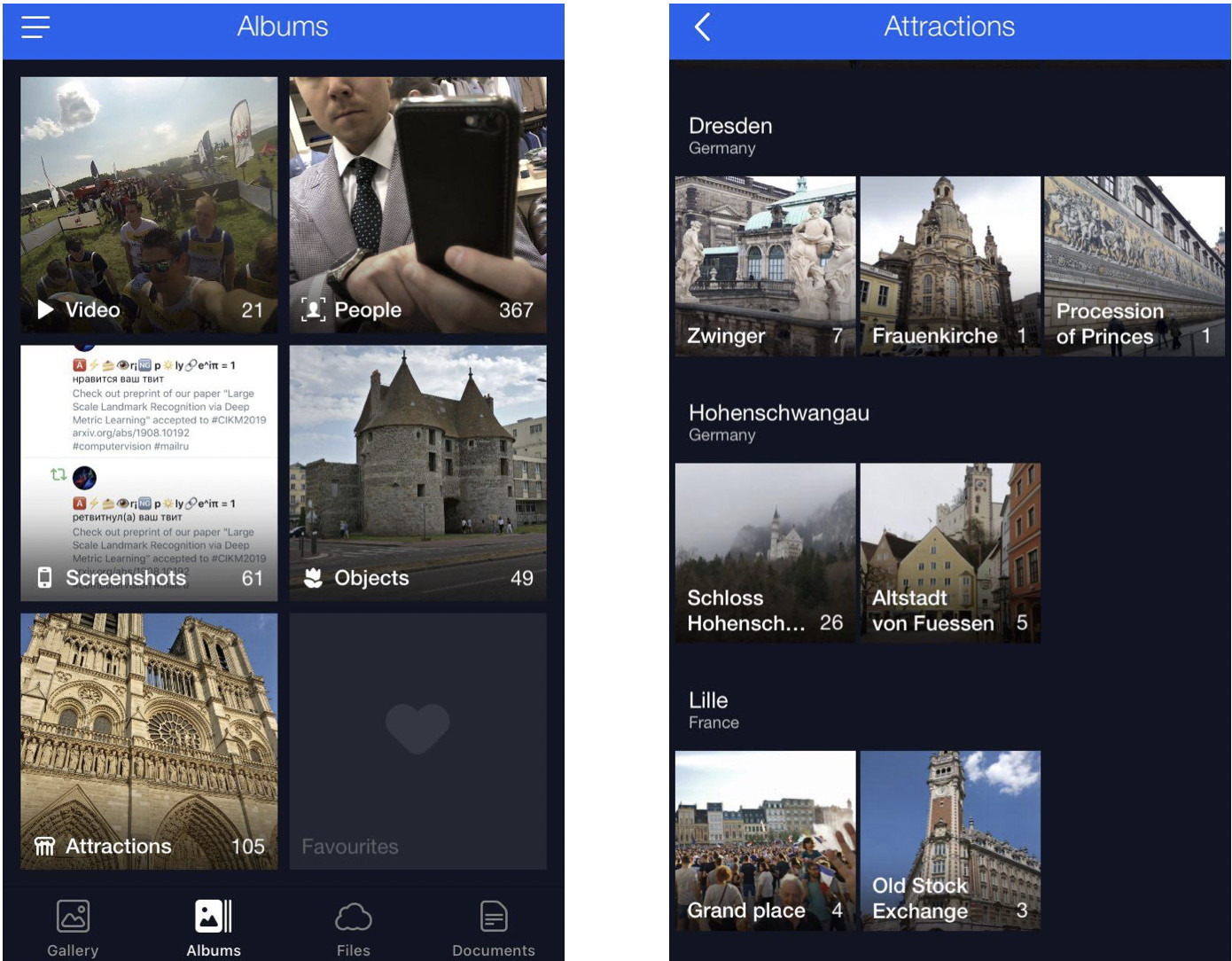
This is my cloud where all photos are classified into albums. There are albums “People”, “Objects”, and “Attractions”. In Attractions album, the landmarks are classified into albums that are grouped by city. A click on Dresdner Zwinger opens an album with photos of this landmark only.
这是我的云,所有照片都分类为相册。 有专辑“ People”,“ Objects”和“ Attractions”。 在“景点”相册中,地标被分类为按城市分组的相册。 单击Dresdner Zwinger可以打开仅包含该地标照片的相册。
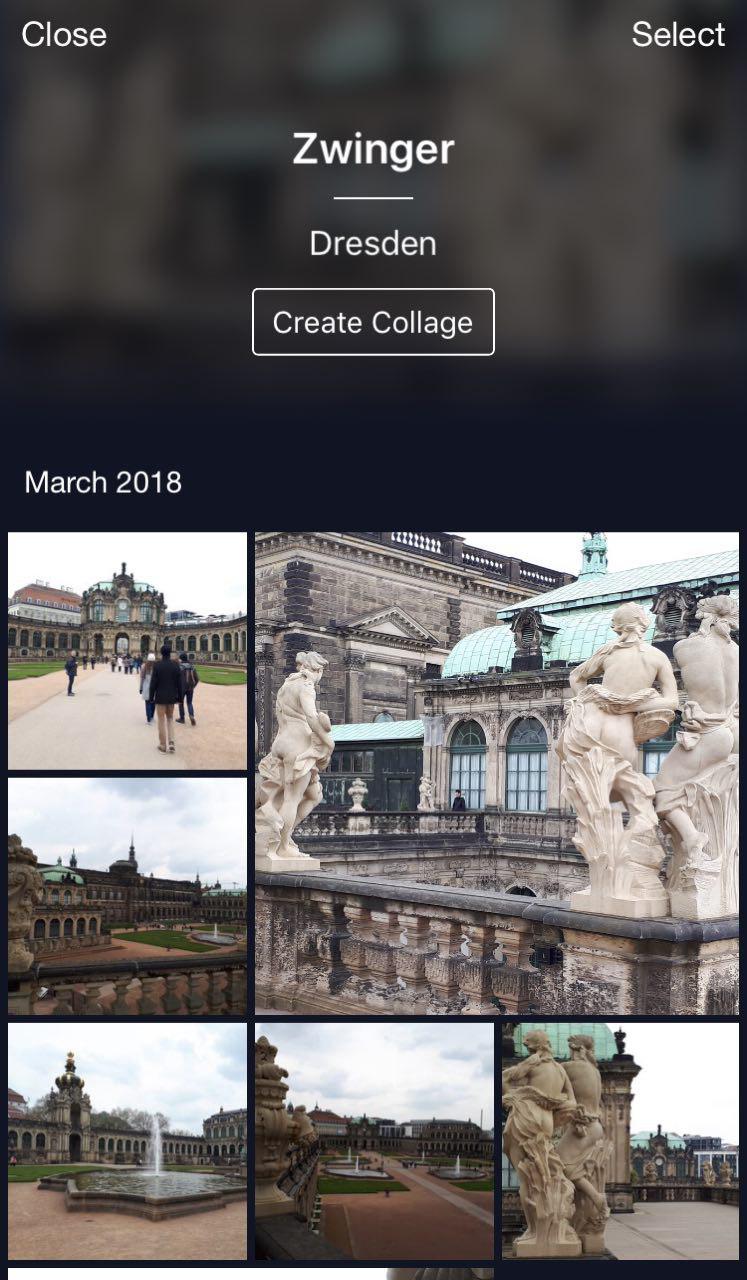
A handy feature: you can go on vacation, take some photos and store them in your cloud. Later, when you wish to upload them to Instagram or share with friends and family, you won’t have to search and pick too long — the desired photos will be available in just a few clicks.
方便的功能:您可以休假,拍摄一些照片并将其存储在云中。 以后,当您希望将它们上传到Instagram或与朋友和家人共享时,您无需搜索和挑选时间太长-只需单击几下即可获得所需的照片。
结论 (Conclusions)
Let me remind you of the key features of our solution.
让我提醒您我们解决方案的主要功能。
- Semi-automatic database cleaning. A bit of manual work is required for initial mapping, and then the neural network will do the rest. This allows for cleaning new data quickly and using it to retrain the model. 半自动数据库清理。 初始映射需要一些手工工作,然后神经网络将完成其余工作。 这样可以快速清除新数据并使用它来重新训练模型。
- We use deep convolutional neural networks and deep metric learning which allows us to learn structure in classes efficiently. 我们使用深度卷积神经网络和深度度量学习,这使我们能够有效地学习课堂结构。
- We have used curriculum learning, i.e. training in parts, as training paradigm. This approach has been very helpful to us. We use several centroids on inference, which allow using cleaner data and find different views of landmarks. 我们将课程学习(即部分培训)用作培训范式。 这种方法对我们非常有帮助。 我们在推论上使用多个质心,从而可以使用更清洁的数据并找到地标的不同视图。
It might seem that object recognition is a trivial task. However, exploring real-life user needs, we find new challenges like landmark recognition. This technique makes it possible to tell people something new about the world using neural networks. It is very encouraging and motivating!
似乎对象识别是一项琐碎的任务。 但是,在探索现实生活中的用户需求时,我们发现了新的挑战,例如地标识别。 这项技术可以使用神经网络告诉人们有关世界的新知识。 这是非常令人鼓舞和激励的!