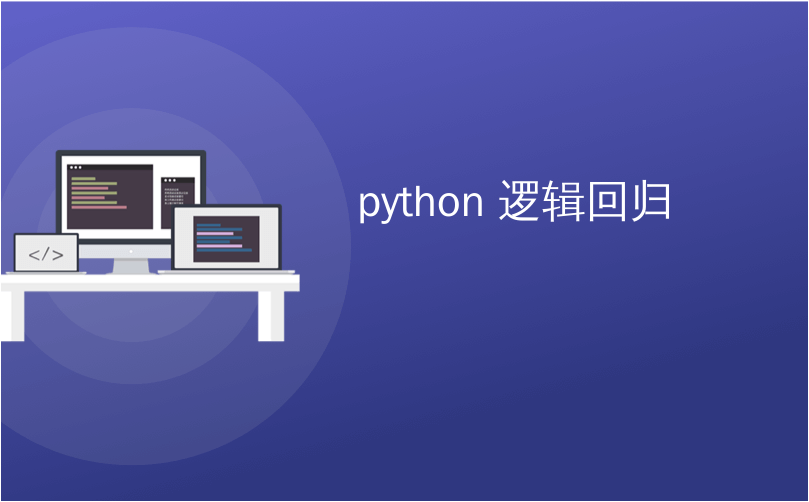
python 逻辑回归
Python中的逻辑回归-快速指南 (Logistic Regression in Python - Quick Guide)
Python中的逻辑回归-简介 (Logistic Regression in Python - Introduction)
Logistic Regression is a statistical method of classification of objects. This chapter will give an introduction to logistic regression with the help of some examples.
Logistic回归是对象分类的一种统计方法。 本章将通过一些示例介绍逻辑回归。
分类 (Classification)
To understand logistic regression, you should know what classification means. Let us consider the following examples to understand this better −
要了解逻辑回归,您应该知道分类的含义。 让我们考虑以下示例以更好地理解这一点-
- A doctor classifies the tumor as malignant or benign. 医生将肿瘤分类为恶性或良性。
- A bank transaction may be fraudulent or genuine. 银行交易可能是欺诈性的或真实的。
For many years, humans have been performing such tasks - albeit they are error-prone. The question is can we train machines to do these tasks for us with a better accuracy?
多年来,人类一直在执行此类任务-尽管它们容易出错。 问题是我们可以训练机器为我们更好地完成这些任务吗?
One such example of machine doing the classification is the email Client on your machine that classifies every incoming mail as “spam” or “not spam” and it does it with a fairly large accuracy. The statistical technique of logistic regression has been successfully applied in email client. In this case, we have trained our machine to solve a classification problem.
这样的机器进行分类的示例是您机器上的电子邮件客户端 ,该电子邮件客户端将所有传入邮件分类为“垃圾邮件”或“非垃圾邮件”,并且做到了相当大的准确性。 Logistic回归统计技术已成功应用于电子邮件客户端。 在这种情况下,我们已经训练了机器以解决分类问题。
Logistic Regression is just one part of machine learning used for solving this kind of binary classification problem. There are several other machine learning techniques that are already developed and are in practice for solving other kinds of problems.
Logistic回归只是用于解决这种二进制分类问题的机器学习的一部分。 还有其他几种机器学习技术已经开发出来,并且正在实践中用于解决其他类型的问题。
If you have noted, in all the above examples, the outcome of the predication has only two values - Yes or No. We call these as classes - so as to say we say that our classifier classifies the objects in two classes. In technical terms, we can say that the outcome or target variable is dichotomous in nature.
如果您已经注意到,在上述所有示例中,谓词的结果只有两个值-是或否。我们将它们称为类-可以说我们说分类器将对象分为两个类。 用技术术语来说,我们可以说结果或目标变量本质上是二分法的。
There are other classification problems in which the output may be classified into more than two classes. For example, given a basket full of fruits, you are asked to separate fruits of different kinds. Now, the basket may contain Oranges, Apples, Mangoes, and so on. So when you separate out the fruits, you separate them out in more than two classes. This is a multivariate classification problem.
还有其他分类问题,其中输出可能被分类为两个以上的类。 例如,给定装满水果的篮子,要求您分离不同种类的水果。 现在,购物篮中可能装有橘子,苹果,芒果等。 因此,当您分离出水果时,会将它们分成两个以上的类。 这是一个多元分类问题。
Python中的逻辑回归-案例研究 (Logistic Regression in Python - Case Study)
Consider that a bank approaches you to develop a machine learning application that will help them in identifying the potential clients who would open a Term Deposit (also called Fixed Deposit by some banks) with them. The bank regularly conducts a survey by means of telephonic calls or web forms to collect information about the potential clients. The survey is general in nature and is conducted over a very large audience out of which many may not be interested in dealing with this bank itself. Out of the rest, only a few may be interested in opening a Term Deposit. Others may be interested in other facilities offered by the bank. So the survey is not necessarily conducted for identifying the customers opening TDs. Your task is to identify all those customers with high probability of opening TD from the humongous survey data that the bank is going to share with you.
考虑到一家银行会与您联系,开发一种机器学习应用程序,这将帮助他们确定可能与他们一起开立定期存款(某些银行也称为定期存款)的潜在客户。 银行定期通过电话或网络表格进行调查,以收集有关潜在客户的信息。 该调查本质上是一般性的,针对的受众非常广泛,其中许多人可能不愿与该银行本身打交道。 在其余的帐户中,只有少数几个有兴趣开设定期存款。 其他人可能会对银行提供的其他服务感兴趣。 因此,不一定需要进行调查来识别开通TD的客户。 您的任务是从银行将与您共享的庞大调查数据中识别出所有可能开通TD的客户。
Fortunately, one such kind of data is publicly available for those aspiring to develop machine learning models. This data was prepared by some students at UC Irvine with external funding. The database is available as a part of UCI Machine Learning Repository and is widely used by students, educators, and researchers all over the world. The data can be downloaded from here.
幸运的是,有这样一种数据可供有志开发机器学习模型的人使用。 该数据是由加州大学欧文分校的一些学生在外部资助下准备的。 该数据库可作为UCI机器学习存储库的一部分获得,并被全世界的学生,教育者和研究人员广泛使用。 数据可以从这里下载。
In the next chapters, let us now perform the application development using the same data.
在下一章中,让我们现在使用相同的数据执行应用程序开发。
建立一个项目 (Setting Up a Project)
In this chapter, we will understand the process involved in setting up a project to perform logistic regression in Python, in detail.
在本章中,我们将详细了解设置项目以在Python中执行逻辑回归的过程。
安装Jupyter (Installing Jupyter)
We will be using Jupyter - one of the most widely used platforms for machine learning. If you do not have Jupyter installed on your machine, download it from here. For installation, you can follow the instructions on their site to install the platform. As the site suggests, you may prefer to use Anaconda Distribution which comes along with Python and many commonly used Python packages for scientific computing and data science. This will alleviate the need for installing these packages individually.
我们将使用Jupyter-机器学习最广泛使用的平台之一。 如果您的计算机上未安装Jupyter,请从此处下载。 对于安装,您可以按照其网站上的说明安装平台。 正如该站点所建议的那样,您可能更喜欢使用Python附带的Anaconda Distribution和用于科学计算和数据科学的许多常用Python软件包。 这将减少单独安装这些软件包的需要。
After the successful installation of Jupyter, start a new project, your screen at this stage would look like the following ready to accept your code.
成功安装Jupyter后,开始一个新项目,此阶段的屏幕如下所示,可以接受您的代码。
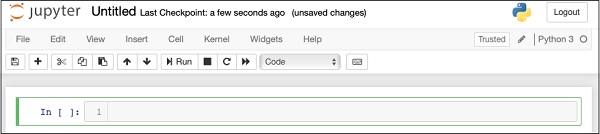
Now, change the name of the project from Untitled1 to “Logistic Regression” by clicking the title name and editing it.
现在,通过单击标题名称并对其进行编辑,将项目名称从Untitled1更改为“ Logistic Regression” 。
First, we will be importing several Python packages that we will need in our code.
首先,我们将导入代码中需要的几个Python软件包。
导入Python包 (Importing Python Packages)
For this purpose, type or cut-and-paste the following code in the code editor −
为此,请在代码编辑器中键入或剪切以下代码-
In [1]: # import statements
import pandas as pd
import numpy as np
import matplotlib.pyplot as plt
from sklearn import preprocessing
fr