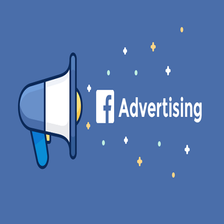
推荐系统论文进阶
文章平均质量分 94
推荐系统及计算广告相关论文阅读、记录、总结、分享
dby_freedom
Everythin has a good ending, if it's not, then it's not the end.
展开
-
CTR预估 论文精读(十七)--ESMM: Entire Space Multi-Task Model
ESMM: Entire Space Multi-Task Model: An Effective Approach for Estimating Post-Click Conversion Rate 论文总结本文介绍 阿里妈妈团队 发表在 SIGIR’2018 的论文《Entire Space Multi-Task Model: An Effective Approach for Estimating Post-Click Conversion Rate》。文章基于 Multi-Task Learning原创 2021-01-11 11:29:22 · 1595 阅读 · 0 评论 -
CTR预估 论文精读(十六)--Youtube 排序系统:Recommending What Video to Watch Next
1. 摘要In this paper, we introduce a large scale multi-objective ranking system for recommending what video to watch next on an industrial video sharing platform. The system faces many real-world challenges, including the presence of multiple competing rank原创 2021-01-04 20:41:39 · 897 阅读 · 0 评论 -
CTR预估 论文精读(十五)--MMOE: Multi-gate Mixture-of-Experts
MMOE: Modeling Task Relationships in Multi-task Learning with Multi-gate Mixture-of-Experts0. 论文摘要Neural-based multi-task learning has been successfully used in many real-world large-scale applications such as recommendation systems. For example, in movi原创 2021-01-03 20:42:24 · 2682 阅读 · 0 评论 -
CTR预估 论文精读(十四)--Deep Rerank: Personalized Re-ranking for Recommendation(PRM)
Deep Rerank: Personalized Re-ranking for Recommendation 论文解读0. 论文摘要Ranking is a core task in recommender systems, which aims at providing an ordered list of items to users. Typically, a ranking function is learned from the labeled dataset to optimize the原创 2020-12-25 17:06:30 · 2199 阅读 · 2 评论 -
CTR预估 论文精读(十三)--Behavior Sequence Transformer for E-commerce Recommendation in Alibaba(BST)
Behavior Sequence Transformer for E-commerce Recommendation in Alibaba 论文解读0. 摘要In this paper, we propose to use the powerful Transformer model to capture the sequential signals underlying users’ behavior sequences for recommendation in Alibaba.1. 创新点其原创 2020-11-07 17:58:52 · 1668 阅读 · 1 评论 -
CTR预估 论文精读(十二)--Deep Session Interest Network(DSIN)
We observe that user behaviors are highly homogeneous in each session, and heterogeneous cross sessions. Based on this observation, we propose a novel CTR model named Deep Session Interest Network (DSIN) that leverages users’ multiple historical sessions i原创 2020-11-06 19:16:02 · 505 阅读 · 0 评论 -
CTR预估 论文精读(十一)--Deep Interest Evolution Network(DIEN)
Deep Interest Evolution Network(DIEN) 论文解读0. 摘要Deep Interest Evolution Network (DIEN) uses interest extractor layer to capture temporal interests from history behavior sequence. At this layer, an auxiliary loss is proposed to supervise interest extracti原创 2020-11-03 20:30:06 · 544 阅读 · 0 评论 -
CTR预估 论文精读(十)--xDeepFM: Combining Explicit and Implicit Feature Interactions for Recommender Systems
以xDeepFM为引,整理一下CTR深度学习模型的发展脉络,并对xDeepFM做具体解读。原创 2019-12-05 10:04:26 · 1341 阅读 · 5 评论 -
CTR预估 论文精读(九)--Deep & Cross Network for Ad Click Predictions
摘要Feature engineering has been the key to the success of many prediction models. However, the process is nontrivial and often requires manual feature engineering or exhaustive searching. DNNs are abl...原创 2019-01-16 09:54:44 · 7543 阅读 · 8 评论 -
CTR预估 论文精读(八)--Deep Interest Network for Click-Through Rate Prediction
文章目录摘要1. 背景1.1 名词解释1.2 相关工作2. 系统总览2.1 电商CTR数据特点2.2 特征处理(User Behavior Features)2.3 **BaseModel**2.4 **Deep Interest Network**3. 训练技术3.1 Mini-batch Aware Regularization3.2 Dice: Data Dependent Activati...原创 2019-01-06 17:00:21 · 4662 阅读 · 2 评论 -
CTR预估 论文精读(七)--DeepFM: A Factorization-Machine based Neural Network for CTR Prediction
AbstractLearning sophisticated feature interactions behinduser behaviors is critical in maximizing CTR forrecommender systems. Despite great progress, existing methods seem to have a strong bias to...原创 2018-12-26 15:58:43 · 4368 阅读 · 4 评论 -
CTR预估 论文精读(六)--Wide & Deep Learning for Recommender Systems
Wide & Deep Learning for Recommender Systems 论文阅读总结文章目录Wide & Deep Learning for Recommender Systems 论文阅读总结Abstract1. Introduction1.1 Memorization & Generalizat原创 2018-12-19 14:40:03 · 2691 阅读 · 0 评论 -
CTR预估 论文精读(五)--Practical Lessons from Predicting Clicks on Ads at Facebook
AbstractOnline advertising allows advertisers to only bid and pay for measurable user responses, such as clicks on ads. As a consequence, click prediction systems are central to most online advertisi...原创 2018-12-12 15:53:55 · 5375 阅读 · 3 评论 -
CTR预估 论文精读(四)--Field-aware Factorization Machines for CTR Prediction
Field-aware Factorization Machines for CTR Prediction1. FMFM的具体详情可以参考之前的论文总结Factorization Machines 论文阅读总结1.1 FM是什么将矩阵 W=wi,jW={w_{i,j}}W=wi,j 矩阵(这是一个对称方阵)分解成 W=VTVW=V^TVW=VTV 的形式,其中 V=(v1,v2,⋯,vd...原创 2018-12-08 21:13:32 · 2009 阅读 · 3 评论 -
CTR预估 论文精读(三)--Factorization Machines
AbstractIn this paper, we introduce Factorization Machines (FM) which are a new model class that combines the advantages of Support Vector Machines (SVM) with factorization models. Like SVMs, FMs are...原创 2018-12-04 09:33:04 · 1992 阅读 · 6 评论 -
CTR预估 论文精读(二)--LightGBM
1. Abstract在之前我介绍过XGBoost,这次想跟大家分享一下LightGBM,它是一款常用的GBDT工具包,由微软亚洲研究院(MSRA)进行开发。LightGBM论文的标题为A Highly Efficient Gradient Boosting Decision Tree。这说明LightGBM它是对于XGB提升性能的版本。而LightGBM相对于其他GBM来说具有相近的准确率而且...原创 2019-02-26 08:16:30 · 3247 阅读 · 1 评论 -
CTR预估 论文精读(一)--XGBoost
XGBoost 论文阅读总结1. 介绍XGBoost的可扩展性(scalability)归因于一些重要的系统优化和算法优化。这些优化包括:一种新的tree-learning算法(a novel tree learning algorithm):用于处理稀疏数据(sparse data)一种理论正确的加权分位数略图过程(a theoretically justified weighted ...原创 2018-11-20 14:27:24 · 3812 阅读 · 4 评论 -
计算广告学习资料汇总
首先感谢以下资料来源的作者papers计算广告论文、学习资料、业界分享 - 王喆Paper Collection of Real-Time Bidding - Weinan Zhang计算广告干货整理 - 雪伦在线课程Introduction to Computational Advertising - Stanford计算广告学 - 刘鹏计算广告2.0 - 刘鹏计算广告学...原创 2018-11-11 13:14:19 · 1902 阅读 · 0 评论