The notes when study the Coursera class by Mr. Andrew Ng "Neural Networks & Deep Learning", section 3.6 "Activation functions". It shows different types of activation functions for NN and the rule of thumb for choosing the activation functions in practice. Share it with you and hope it helps!
————————————————
When build a NN, one of the choices to make is what activation functions to use in the hidden layers as well as the output layer. Besides the sigmoid activation function, sometimes other choices can work much better.
- sigmoid function
It's defined as:
Its plot looks like:
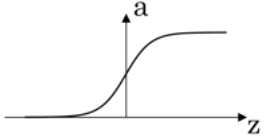
- tanh function
Also called hyperbolic function. And defined as:
Its plot looks like:
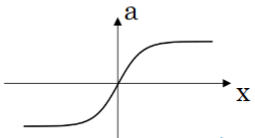
It's actually a shifted version of sigmoid function and crosses point and goes between
.
It turns out that if you replace the sigmoid function of hidden layer with tanh function, it almost always works better. This is because the mean of activations that come out of your hidden layer is closer or equal to 0. Sometimes, when you train a learning algorithm, you might center the data and let your data have zero mean. Using a tanh instead of a sigmoid function kind of has such effect. This makes the learning for the next layer a little bit easier. One exception is for output layer where we still use sigmoid function. The reason is , it makes sense for
- ReLU
One downside for both sigmoid function and tanh function is that when z is very large or small, then the gradient or slope of this function will be close to 0. This can slow down gradient descent.
ReLU (Rectified Linear Unit) is one of popular choises of activation that mitigated this issue, and it's defined as below:
Its plot looks like this:
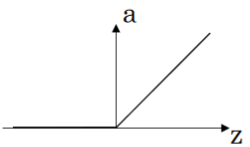
Note that for half of the range of z, the slope for ReLu is 0. But in practice, enough of the hidden units will have z greater than 0, so learning can still be quite fast.
- Leaky ReLu
One disadvantage of ReLu is the derivative is equal to 0 when z is negative. One activation function is called Leaky ReLU. It usually works better than ReLU although it's just not used as much in practice.
Here's the definition:
The plot looks like:
Rules of thumb for choosing activation functions:
- For binary classification, the sigmoid function is very natural for the output layer.
- For all other units of hidden layers, ReLU is increasingly the default choice of activation function
- If you're not sure which activation functions work best, try them all and evaluate them on validation set
<end>