1. np.squeeze
一,np.squeeze
"""
np.squeeze 删除单维度的条 对多维度无效
"""
import numpy as np
a=np.array([[1,2],[3,4],[4,5]])
print(a)
print(a.shape)
b=np.squeeze(a)
print(b)
c=a.reshape(1,6,1)
print(c)
print(np.squeeze(c))
print(np.squeeze(c).shape)
print(np.squeeze(c,axis=0))
print(np.squeeze(c,axis=0).shape)
print(np.squeeze(c,axis=1))
print(np.squeeze(c,axis=1).shape)
维度不为1,报错。
print(np.squeeze(c,axis=2))
print(np.squeeze(c,axis=2).shape)
2.np.newaxis增加维度
import numpy as np
a=np.arange(1,5)
print(a)
print(a.reshape([-1,1]))
b=a[:,np.newaxis]
print(b)
print(b.shape)
3. np.random
import numpy as np
"""
0~1之间产生随机值
"""
a=np.random.rand(3,2)
print(a)
"""
产生标准正态分布的值
"""
a=np.random.randn(3,2)
print(a)
"""
产生随机整数1~3之间
"""
a=np.random.randint(low=1,high=3,size=(3,2))
print(a)
"""
产生高斯分布:均值 方差
"""
a=np.random.normal(loc=0,scale=1,size=(3,2))
print(a)
numpy.random.choice(a, size=None, replace=True, p=None)
从给定的一维数组中生成一个随机样本;
参数 | 参数意义 |
---|---|
a | 为一维数组或者int数据; |
size | 为生成的数组维度; |
replace | 是否原地替换; |
p | 为样本出现的概率; |
np.random.choice(5,3) # 等价于np.random.randint(0,5,3)
replace为true会重复,为false不会重复
a = ['bird', 'meh', 'sad', 'd', '123']
print(np.random.choice(a, 5)) # replace默认为True
print(np.random.choice(a, 5, replace=False))
4. np.logspace
import numpy as np
#等比数列 9/(5-1)=2.25 10^0 10^2.25 10^5.5 10^7.75 10^9
a=np.logspace(0,9,5)
print(a)
5. 等差数列
a=np.linspace(2.0, 3.0, num=5)
print(a)
b=np.linspace(2.0, 3.0, num=5, endpoint=False)
print(b)
c=np.linspace(2.0, 3.0, num=5, retstep=True)
print(c)
6. np.argmax,np.sum
np.max操作也满足记住, 0列1行, 0从上往下看,1从左往右看
#axis=0 对列操作 axis=1 对行操作 axis=2 对最后一根轴操作
a = np.array([[1, 2,3],
[3, 4,5],
[4, 5,6],
[6, 7,8]])
print(np.mean(a,axis=0))
print(np.sum(a,axis=0))
print(np.argmax(a,axis=0))
print(np.all(a==2,axis=0))
#axis = 2对最后一根轴操作
a=a.reshape((2,2,3))
print(a)
print()
print(np.mean(a,axis=0))
print()
print(np.mean(a, axis=1))
print()
print(np.mean(a, axis=-1))
a=np.array([[1,0,0],[0,1,0],[0,0,1],[0,1,0]])
print(a)
print(np.argmax(a,1))#对行找最大值索引
b=np.array([[1,0,0],[0,1,0],[1,0,0],[0,0,1]])
print(b)
print(np.argmax(b,1))
print(np.argmax(a,1)==np.argmax(b,1))
print(np.sum(np.argmax(a,1)==np.argmax(b,1)))
img=np.array([[[1,2,3],[6,4,5]]])
print(img)
pre=np.argmax(img,axis=2)
print(pre)
pre=np.expand_dims(pre,axis=-1)
print(pre)
找出每个channel的最值索引,然后进行分割。
np.sum
import numpy as np
a=np.array([[[[1,2],[1,3],[1,4]],
[[1,7],[1,6],[1,5]],
[[1,2],[1,3],[1,4]]]])
print(a.shape)
print(np.sum(a,axis=3))
print(np.sum(a,axis=3).shape)
print(np.sum(a,axis=3,keepdims=True))
print(np.sum(a,axis=3,keepdims=True).shape)
保持维度故变为(1,3,3,1)
对于三维的话 比如(m,32,32,17)是由每一个channel贡献loss
a=np.array([[[[1,2,3]],
[[2,3,4]]],
[[[1, 2, 3]],
[[2, 3, 4]]]]
)
print(a)
print(a.shape)
print(np.sum(a, axis=0))
print(np.sum(a,axis=1))
# print(np.sum(a, axis=2))
# print(np.sum(a, axis=3))
print(np.mean(np.sum(a,axis=1)))
xe = -tf.reduce_sum(
tf.multiply(labels_ * tf.log(logits + epsilon), cb),
reduction_indices=[1])
loss_total = tf.reduce_mean(xe)+m_IOU_loss
np.all(),和sum一样axis=1,是行操作。沿着轴的方向都为true,则返回为true。
a=np.all([[True, False],
[True, True]])
print(a)
a = np.all([[True, False],
[True, True]],axis=1)
print(a)
a = np.all([[1, 0],
[1, 1]], axis=1)
print(a)
可用来清掉,全0的元素。
x=np.array([[0,1,0,1],
[0,200,1,0],
[34,40,0,3],
[35,0,3,4],
[0,0,0,0]])
print(np.all(x == 0, axis=1))
y=x[~np.all(x == 0, axis=1)]
print(x)
print(y)
7. np.stack,np.hstack,np.vstack
np.stack二维情况
import numpy as np
a=[[1,2,3],
[4,5,6]]
print("列表a如下:")
print(a)
print("增加一维,新维度的下标为0")
c=np.stack(a,axis=0)
print(c)
print("增加一维,新维度的下标为1")
c=np.stack(a,axis=1)
print(c)
a=[[1,2,3],
[4,5,6]]
b=[[1,2,3],
[4,5,6]]
c=[[1,2,3],
[4,5,6]]
print("a=",a)
print("b=",b)
print("c=",c)
print("增加一维,新维度的下标为0")
d=np.stack((a,b,c),axis=0)
print(d)
print(d.shape)
print("增加一维,新维度的下标为1")
d=np.stack((a,b,c),axis=1)
print(d)
print(d.shape)
print("增加一维,新维度的下标为2")
d=np.stack((a,b,c),axis=2)
print(d)
print(d.shape)
np.hstack按照水平方向连接
import numpy as np
a=[[1],[2],[3]]
b=[[1],[2],[3]]
c=[[1],[2],[3]]
d=[[1],[2],[3]]
print(np.hstack((a,b,c,d)))
np.vstack按垂直方向连接
import numpy as np
a=[[1],[2],[3]]
b=[[1],[2],[3]]
c=[[1],[2],[3]]
d=[[1],[2],[3]]
print(np.vstack((a,b,c,d)))
把sober算子变成两个通道的sober算子,其中生成的2用作刚好是输入的channel
import tensorflow as tf
import numpy as np
fx = np.array([[-1, 0, 1], [-2, 0, 2], [-1, 0, 1]]).astype(np.float32)
fy = np.array([[-1, -2, -1], [0, 0, 0], [1, 2, 1]]).astype(np.float32)
fx = np.stack((fx, fx), axis=2)
print(fx)
# fy = np.stack((fy, fy), axis=2)
# print(fy)
fx = np.reshape(fx, (3, 3, 2, 1))
print(fx)
# fy = np.reshape(fy, (3, 3, 2, 1))
tf_fx = tf.Variable(tf.constant(fx))
8. np.array
>>> a
array([0, 2, 1])
>>> scores
array([[1, 2, 3],
[2, 3, 4],
[4, 5, 6]])
>>> scores[a]
array([[1, 2, 3],
[4, 5, 6],
[2, 3, 4]])
一个样本对应一列,下面的代码可以用来寻找每个样本标签量对应的数值。
9. np.concatenate
import numpy as np
a = np.array([[1, 2], [3, 4]])
b=np.array([[5,6]])
c=np.concatenate((a, b), axis=0)
print(c)
c=np.concatenate((a, b.T), axis=1)
print(c)
c=np.concatenate((a, b.T), axis=None)
print(c)
对于列表也可以
a=np.concatenate([[[1,2]],[[3,4]]],axis=0) print(a)
10. np.transpose()
import numpy as np
a=np.array([[1],[2],[3],[4],[5],[6]])
print(a)
b=a[:2,:].transpose()
c=a[2:4,:].transpose()
d=a[4:,:].transpose()
print(b,c,d)
e=np.append(b,c,axis=0)
a=np.append(e,d,axis=0)
print(a)
11. one_hot
import numpy as np
#转换one-hot
def convert_to_one_hot(label):
n_classes=max(label)+1
label = np.eye(n_classes)[label.reshape(-1)]
return label
label=[1,0,2,3,0]
Y=convert_to_one_hot(np.array(label))
print(Y)
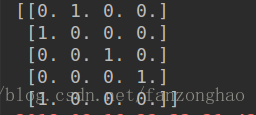
12. np广播机制
13. np.split
方便对生成的box坐标进切分
import numpy as np
A = np.arange(16).reshape(4,4)
print('A:',A)
print(np.split(A,2,axis = 1))
c,d=np.split(A,2,axis = 1)
print(c)
print(d)
import numpy as np
A = np.arange(16).reshape(4,4)
print(A)
print(np.split(A,2,axis = 0))
c,d=np.split(A,2,axis = 0)
print(c)
print(d)
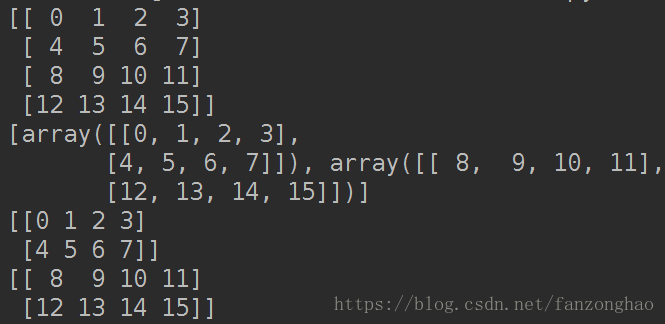
14. np.rot90,图片旋转90度
path='./data/2.png'
img=cv2.imread(path)
# 逆时针90度
img=np.rot90(img)
cv2.imwrite('./data/img_size_out_1.jpg',img)
15. np.unravel_index,找最值的索引值
a = np.array([[1, 2, 3],
[4, 5, 6]])
index = np.unravel_index(np.argmax(a), a.shape)
print(index)
16. np.where
np.where两种用法
where(condition, x=None, y=None)
如果x,y为空,返回condition中值为True的位置的ndarray
对于三维
#三维情况
import numpy as np
b = np.where([[0, 1],
[1, 0]])
print('==b:', b)
x = np.arange(12).reshape(2, 3, 2)
print('==x:', x)
index = np.where(x > 3)
print('==index:', index)
print('==x[index]:', x[index])
对于二维
import numpy as np
b = np.where([[0, 1],
[1, 0]])
print('==b:', b)
x = np.arange(9.).reshape(3, 3)
print('==x:', x)
index = np.where(x > 3)
print('==index:', index)
print('==x[index]:', x[index])
index_y, index_x = index
print('==index_y, index_x:', index_y, index_x)
need_value = []
for i in range(len(index_y)):
need_value.append(x[index_y[i]][index_x[i]])
print('==need_value:', need_value)
# 同时满足两个条件的
index = np.where((x[:, 0] > 3) & (x[:, 1] < 8))
print('==index', index)
print('x[index]:', x[index])
对于一维
class_ids=np.array([1,2,3])
index = np.where(class_ids == 1)
print('==index:', index)
print('==class_ids[index]:', class_ids[index])
如果x,y不为空,返回值和x、y有相同的形状,如果condition对应位置值为True那么返回ndarrayr对应位置为x的值,否则为y的值
a=np.where([[True, False],
[True, True]],
[[1, 2],
[3, 4]],
[[9, 8],
[7, 6]])
print(a)
可以用来找出某个点的索引
a = np.array([[255, 0, 0],
[0,0,255],
[0,255,0]])
# 找出255的索引号
b=np.where(a[...]==255)
print(b)
y,x=b#是索引号,故行对应y,列对应x
print(y)
print(x)
point=np.stack((x,y),axis=-1)
plt.plot(point[:,0],point[:,1],'o')
plt.show()
同时要满足两个np.where
index_x = np.where(abs(points[:, 0] - points[0][-2]) < x_dis)[0]
print('index_x:',index_x)
# if len(index_x):
# #y1-y1
index_y = np.where(abs(points[:, 1] - points[0][1]) < y_dis)[0]
print('index_y: ',index_y)
common_index=list(set(index_x)&set(index_y))
print('common_index:',common_index)
更简单写法:
kpts_2d = np.array([[680, 0],
[10, 10]], dtype=np.float32)
print((kpts_2d[:, 0] < 640)*(kpts_2d[:, 0] >= 0))
kpts_2d = kpts_2d[(kpts_2d[:, 0] < 640)*(kpts_2d[:, 0] >= 0)]
print(kpts_2d)
例如centernet中用于后处理找到每一类对应的类别,长宽与偏移量
import numpy as np
# (c, h, w)
cls = np.array([[[0.5, 0.2],
[0.9, 0.8],
[0.8, 0.1]],
[[0.6, 0.7],
[0.8, 0.6],
[0.9, 0.8]]])
# (2, h, w)
wh = np.array([[[5, 2],
[9, 8],
[8, 1]],
[[6, 7],
[8, 9],
[9, 1]]])
# (2, h, w)
reg = np.random.rand(2, 3, 3)
print('==reg:', reg)
print('==cls.shape:', cls.shape)
index = np.where(cls >= 0.8)
print('==index:', index)
score = np.array(cls[index])
print('==score:', score)
cat = np.array(index[0])
print('===cat:', cat)
ctx, cty = index[-1], index[-2]
print('==before ctx, cty', ctx, cty)
w, h = wh[0, cty, ctx], wh[1, cty, ctx] # 预测的长宽
print('==w, h:', w, h)
off_x, off_y = reg[0, cty, ctx], reg[1, cty, ctx] # 预测的中心点偏移量
print('==off_x, off_y:', off_x, off_y)
ctx = np.array(ctx) + np.array(off_x) # 中心点x
cty = np.array(cty) + np.array(off_y) # 中心点y
print('==after ctx, cty', ctx, cty)
x1, x2 = ctx - np.array(w) / 2, ctx + np.array(w) / 2
y1, y2 = cty - np.array(h) / 2, cty + np.array(h) / 2
bbox = np.stack((cat, score, x1, y1, x2, y2), axis=1).tolist()
bbox = sorted(bbox, key=lambda x: x[1], reverse=True)
print('==bbox:', bbox)
17. argsort,可用来寻找score的最大值
scores=np.array([0.5,0.7,0.3,0.2])
#得到从小到大的索引值
print(scores.argsort())
# 得到从大到小的索引值
print(scores.argsort()[::-1])
for i in scores.argsort()[::-1]:
print(scores[i])
a=np.array([1,3,0,2])
b=np.argsort(a)
print(b)
a = np.array([[1, 5, 0, 8],
[ 3, 4, 7, 3]])
ind = np.unravel_index(np.argsort(a, axis=None), a.shape)
print(ind)
print(ind[0][-1],ind[1][-1])
print(a[ind[0][-1],ind[1][-1]])
print(a[ind[0][-2], ind[1][-2]])
18. np.delete
import numpy as np
a = np.array(np.arange(12).reshape(3, 4))
print(a)
#沿着行删除,删除第一行
print(np.delete(a,obj=1,axis=0))
# 沿着行删除,删除第0行和第一行
print(np.delete(a, obj=[0,1], axis=0))
# 沿着列删除,删除第三列
print(np.delete(a, obj=3, axis=1))
#删除多个行
print(np.delete(a, obj=[0,1], axis=0))
19. np.tile,沿着行和列复制
沿着列复制
mat = np.array([[1,2],
[3, 4]])
mat=np.tile(mat,(1,3))
print(mat)
沿着行复制
mat = np.array([[1,2],
[3, 4]])
mat=np.tile(mat,(3,1))
print(mat)
20. np.prod连乘
a=np.array([[1,2],[3,4]])
print(np.prod(a))
print(np.prod(a,axis=0))
print(np.prod(a, axis=1))
21.np.reshape,flatten()
a=np.array([[1,2]],dtype=np.float32)
print(a.reshape(-1))
print(a.flatten().astype(np.int32))
得到的每一列代表每一个channel
a=np.array([[[1,0,1],
[1,1,0]],
[[0,1,1],
[0,1,0]]])
print(a)
print(a.shape)
b=a.reshape(-1, a.shape[-1])
print(b)
print('============')
"""转置方便求交集"""
print(b.T)
制作阈值大于0.5预测的mask.
masks1=np.array([[0.6,1,0],
[0.2,0,1],
[0.3,1,0],
[0.6,0,1]])
print(masks1.shape[-1])
masks1 = np.reshape(masks1 > .5, (-1, masks1.shape[-1]))
print(masks1)
总的计算多个channel的IOU
def compute_overlaps_masks(masks1, masks2):
"""Computes IoU overlaps between two sets of masks.
masks1, masks2: [Height, Width, instances]
"""
# If either set of masks is empty return empty result
if masks1.shape[-1] == 0 or masks2.shape[-1] == 0:
return np.zeros((masks1.shape[-1], masks2.shape[-1]))
# flatten masks and compute their areas
masks1 = np.reshape(masks1 > .5, (-1, masks1.shape[-1])).astype(np.float32)
masks2 = np.reshape(masks2 > .5, (-1, masks2.shape[-1])).astype(np.float32)
area1 = np.sum(masks1, axis=0)
area2 = np.sum(masks2, axis=0)
# intersections and union
intersections = np.dot(masks1.T, masks2)
union = area1[:, None] + area2[None, :] - intersections
overlaps = intersections / union
return overlaps
22. np 切片
a= np.array([[1, 2, 3],
[4, 5, 6],
[7, 8, 9],
[10, 11, 12]])
b = np.array([0, 2, 0, 1])
print(a[np.arange(4),b])
a[np.arange(4), b]+=10
print('a=',a)
#所有大于5的替换为5
MAX = 5
nums = np.array([1, 4, 10, -1, 15, 0, 5])
print(nums > MAX)
nums[nums > MAX] = MAX
print(nums)
#直接根据索引找到一行向量的值
nums = np.array([1, 4, 10, -1, 15, 0, 5])
print(nums[[1, 2, 3, 1, 0]])
23. np.flatnonzero与np.nonzero(x)
(1)np.flatnonzero
该函数输入一个矩阵,返回拉伸后矩阵中非零元素的索引
x = np.arange(-3, 5)
print('x=',x)
print(np.flatnonzero(x))
print(np.flatnonzero(x==-3))
(2)np.nonzero(x), 返回非零元素的索引
x = np.array([[3, 0, 0], [0, 4, 0], [5, 6, 0]])
print(x)
res = np.nonzero(x)
print('res:', res)
res_ = x[np.nonzero(x)]
print('res_:', res_)
24. np.bincount统计元素出现的次数
x = np.array([0, 1, 2, 2, 1, 1])
print(np.bincount(x))
25. 求交集np.intersect1d
print(np.intersect1d([1, 3, 4, 2], [3, 1, 2, 1]))
26. np.arctan2与np.arctan
x = np.array([-1, +1, +1, -1])
y = np.array([-1, -1, +1, +1])
#-180~180
print(np.arctan2(y, x) * 180 / np.pi)
#-90~90
print(np.arctan(y/x) * 180 / np.pi)
27. np.transpose
path='./data/raw.jpg'
img=cv2.imread(path)
img=np.transpose(img,(1,0,2))
cv2.imwrite('./data/raw_1.jpg',img)
实现图片转90度
变为
上下翻转 左右翻转 BGR变为RGB
img=img[::-1,...] img=img[:,::-1,:] img=img[:,:,::-1]
28. np.zeros
a=np.zeros((1,2,3))
print(a)
a[0,1,:]=255
print(a)
29. 返回上三角的索引,可用来制作显示下三角的相关系数矩阵。np.triu_indices_from()
import numpy as np
mask=np.array([[1,2,3],
[4,5,6],
[5,6,7]])
print(mask)
print(np.triu_indices_from(mask))
mask[np.triu_indices_from(mask)] = True
print(mask)
30. random.getrandbits(1)用来产生0,1其中之一的随机数,用来做数据增强
import random
for i in range(10):
a = random.getrandbits(1)
print(a)
if a:
print(True)
31. np.fliplr,np.flipud实现左右 上下翻转
32. np.random.shuffle
import numpy as np
X=np.array([[1,2,3],
[3,4,5],
[5,6,7],
[7,8,9]])
print(X)
idx_random = np.arange(X.shape[0])
print(idx_random)
np.random.shuffle(idx_random)
print(idx_random)
X= X[idx_random]
print('==========after shuffle=============')
print(X)
33.np.clip
将小于3的和大于8的强制为3和8
x = np.array([[1, 2, 3, 5],
[6, 7, 8, 9]])
print('x=', x)
y = np.clip(x, 3, 8)
print('y=', y)
#等价于
x[x>=8]=8
x[x<=3]=3
print('x=', x)
34.np.percentile求百分位数,可用来确定卫星图像的RGB三个波段的阈值,方便进行0~255归一化处理,其中取值为50时相当于求中位数。
a=np.array([[1,2,3,4],
[0,1,2,0]])
print(a[a>0])
print(np.percentile(a[a>0],50))
print(np.percentile(a, 50))
35. np.ploy1d 多项式子
print(np.poly1d(3))
print('=============')
print(np.poly1d([1,2,3,4]))
36. np.exp,注意数值不稳定问题
f = np.array([123, 456, 789]) # 例子中有3个分类,每个评分的数值都很大
p = np.exp(f) / np.sum(np.exp(f)) # 不妙:数值问题,可能导致数值爆炸
print('p=',p)
# 那么将f中的值平移到最大值为0:
f -= np.max(f) # f becomes [-666, -333, 0]
p = np.exp(f) / np.sum(np.exp(f)) # 现在OK了,将给出正确结果
print('p=', p)
37. np.r_,np.c_,类似np.vstack.np.hstack
import numpy as np
a = np.array([[1, 2],
[3,4]])
b = np.array([[4, 5],
[6,7]])
c = np.c_[a,b]
print(np.r_[a,b])
print(np.c_[a,b])
print(np.c_[c,a])
38. 实现max(0, Sjc - Sjyj + 1)
第一种解法 :
x1 = np.array(np.arange(6)).reshape(3, 2)
x2 = np.array([2, 3, 3]).reshape(-1, 1)
print('x1=')
print(x1)
print('x2=')
print(x2)
print('x1-x2+1=')
print(x1-x2+1)
mask=x1-x2+1>0
print('mask=')
print(mask)
scores=(x1-x2+1)*mask
print('scores=')
print(scores)
第二种解法:
x1 = np.array(np.arange(6)).reshape(3, 2)
x2 = np.array([2, 3, 3]).reshape(-1, 1)
for i in range(x1.shape[0]):
for j in range(x1.shape[1]):
x1[i][j] = max(0, x1[i][j] - x2[i][0] + 1)
print(x1)
39. label smoothing,制作平滑的标签
new_labels = (1.0 - label_smoothing) * one_hot_labels + label_smoothing / (num_classes-1)
label_smoothing是一个很小的常数
40. np.unique 去重(可以对坐标)
a=np.unique([1, 1, 2, 2, 3, 3])
print(a)
a = np.array([[1, 1], [2, 3]])
print(np.unique(a))
a = np.array([[1, 0, 0], [1, 0, 0], [2, 3, 4]])
print(np.unique(a, axis=0))
41. np.pad
用来补黑边,变成1024×1024
_, ax = plt.subplots(1, figsize=(16, 16))
ax.axis('off')
image=cv2.imread('1.jpg')
print(image.shape)
h, w = image.shape[:2]
max_dim=1024
top_pad = (max_dim - h) // 2
bottom_pad = max_dim - h - top_pad
left_pad = (max_dim - w) // 2
right_pad = max_dim - w - left_pad
padding = [(top_pad, bottom_pad), (left_pad, right_pad), (0, 0)]
image = np.pad(image, padding, mode='constant', constant_values=0)
cv2.imwrite('2.jpg',image)
# ax.imshow(image)
# plt.show()
42. np.cumsum累加
stage_epochs=[50,50,50]
a=np.cumsum(stage_epochs)[:-1]
print(a)
43. 交换二维数据的列向量
print(coord_Y)
coord_Y[:, [0, 1]] = coord_Y[:, [1, 0]]
print(coord_Y)
44. 将一个二维数组按照X先排序,Y在排序,np.lexsort
def coord_sort(x,y):
#按照x,y来排序
ind = np.lexsort((y.tolist(), x.tolist())) # Sort by x, then by y
coord = [(x.tolist()[i], y.tolist()[i]) for i in ind]
coord = np.array(coord).reshape(-1, 2)
return coord
coord = np.array([[10, 60],
[10, 20],
[20, 20],
[40, 40],
[40, 60],
[20, 40]])
#按照先X后Y排的序
coord_X=coord_sort(coord[:,0],coord[:,1])
print(coord_X)
四个点的
import numpy as np
def coord_sort(points):
# 按照x,y来排序
ind = np.lexsort((points[:,1].tolist(), points[:,0].tolist())) # Sort by x, then by y
return points[ind]
coord = np.array([[10, 60, 10,20],
[11, 70, 20, 20],
[11, 40,40,60],
[30, 20,200,100],
[30, 30,40,50]])
# 按照先X后Y排的序
coord_X = coord_sort(coord)
print(coord_X)
45. 删除副黑色图片中过短的白线
import numpy as np
def delete_short_line(img,line_length):
sum_axis = np.sum(img == 255,axis=0,keepdims=True)
print('sum_axis:',sum_axis)
print('sum_axis>line_length:',sum_axis>line_length)
img = img*(sum_axis>line_length)
return img
if __name__ == '__main__':
img=np.array([[255,0,0],
[255,0,255],
[255,255,0]])
img=delete_short_line(img,line_length=1)
print('==img==')
print(img)
46.对竖值方向的一系列点做聚类
import numpy as np
def get_cluster(mode,x_dis,y_dis):
points=np.array([[3,2],
[10,2],
[1,4],
[2,5],
[1,3],
[12,3],
[15,4],
[10,5],
[20,1]])
if mode == 'veri':
points = points[points[:,0].argsort()]
else:
points = points[points[:,1].argsort()]
# print(points)
# print(points.shape[0])
cluster = []
while points.shape[0] > 2:
# print('points:',points)
if mode=='veri':
index = np.where(abs(points[:, 0] - points[0][0]) < x_dis)[0]
else:
index = np.where(abs(points[:, 1] - points[0][1]) < y_dis)[0]
index = sorted(index)
cluster.append(points[index])
points=points[index[-1]+1:]
print('cluster:',cluster)
return cluster
if __name__ == '__main__':
get_cluster(mode='veri',x_dis=5,y_dis=5)
47. np.max,np.maximum
np.max是计算最大值,而np.maximum是计算相比较的值
a=np.array([1,2,3])
print(np.max(a))
print(np.maximum(a,2))
48. np.setdiff1d,可以得到一个ndarray中有,一个无
a = np.array([1, 2, 3, 4, 5, 6, 7, 8, 9])
b = np.array([3, 4, 7, 6, 7, 8, 11, 12, 14])
c = np.setdiff1d(a, b)
print('a中有,b中无')
print('c:', c)
49. np.count_nonzero得到非零元素个数
a=np.count_nonzero([[0, 1, 7, 0, 0],
[3, 0, 0, 2, 19]], axis=0)
print('a:', a)
50.np.nonzero得到非零元素索引
x = np.array([[3, 0, 0],
[0, 4, 0],
[5, 6, 0]])
a = np.nonzero(x)
print('a:', a)
51.np.diff
矩阵中后一个元素减去前一个元素的差值,此处用于找到polygon的四个点(局限于水平框)
import numpy as np
rect = np.zeros((4,2))
# pts = np.array([[0, 0],
# [0, 2],
# [2, 2],
# [2, 0]])
pts = np.array([933,255,954,255,956,277,936,277])
pts = pts.reshape(-1,2)
print('===pts', pts)
d = np.diff(pts, axis=1)
print('===d:', d)
s = np.sum(pts, axis=1)
rect[0] = pts[np.argmin(s)]
rect[2] = pts[np.argmax(s)]
# the top-right point will have the smallest difference,
# whereas the bottom-left will have the largest difference
d = np.diff(pts, axis=1)
rect[1] = pts[np.argmin(d)]
rect[3] = pts[np.argmax(d)]
print('===rect:', rect)
利用np.argmin找到框的最小点,再用np.roll滚动获取最小点开始的点(局限于框是有顺序的,不是乱序)
#得到左上右下
def cal_stand_points(points):
s = np.sum(points, axis=1)
left_top_index = np.argmin(s)
right_bottom_index = np.argmax(s)
rect = np.roll(points, 4-left_top_index, axis=0)
return rect
pts = np.array([933, 255, 954, 255, 956, 277, 936, 277])
pts = pts.reshape(-1, 2)
new_pts = cal_stand_points(pts)
print('==new_pts:', new_pts)
52.np.meshgrid生成网格点
import numpy as np
import matplotlib.pyplot as plt
x = np.array([0, 1, 2])
y = np.array([0, 1])
X, Y = np.meshgrid(x, y)
print('==X:', X)
print('==Y:', Y)
plt.plot(X, Y,
color='red', # 全部点设置为红色
marker='.', # 点的形状为圆点
linestyle='') # 线型为空,也即点与点之间不用线连接
plt.xlim(-1, 3)
plt.ylim(-1, 2)
plt.grid(True)
plt.show()
其中X代表横坐标 Y代表纵坐标
x = np.array([0, 1, 2])
y = np.array([0, 1])
X, Y = np.meshgrid(x, y)
print('==X:', X)
print('==Y:', Y)
#得到沿x方向走的竖直y方向坐标
points = np.stack(np.meshgrid(x, y)).T
print(points)
#
print('points[0, :]', points[0, :])
print('points[1, :]', points[1, :])
print('points[2, :]', points[2, :])
53.np.linalg.norm计算范数(默认计算2范数)
1.得到两个向量角度
import math
feature0 = np.array([1, 1])
feature1 = np.array([2, 2])
x0 = feature0 / np.linalg.norm(feature0)
print('==x0:', x0)
x1 = feature1 / np.linalg.norm(feature1)
print('==x1:', x1)
cosine = np.dot(x0, x1)
print('==cosine:', cosine)
cosine = np.clip(cosine, -1.0, 1.0)
theta = math.acos(cosine)
theta = theta * 180 / math.pi
print('===theta:', theta)
2.得到box长宽
box = np.array([[0, 0],
[2, 0],
[2, 1],
[0, 1]])
w, h = np.linalg.norm(box[0] - box[1]), np.linalg.norm(box[1] - box[2])
print('宽度:{},长度:{}'.format(w, h))
54.np.roll滚动(默认axis是1)
x = np.arange(10)
print('x:',x)
y = np.roll(x, 2)
print('==y:', y)
x2 = np.reshape(x, (2,5))
print('==x2:', x2)
y2 = np.roll(x2, 1)
print('==y2:', y2)
y3 = np.roll(x2, 1, axis=0)
print('===y3:', y3)
y4 = np.roll(x2, 2, axis=1)
print('====y4:', y4)
55:计算矩形的相邻边长度与周长面积
import Polygon as plg
import cv2
def dist(a, b):
return np.sqrt(np.sum((a - b) ** 2))
bbox = np.array([[0, 0],
[2, 0],
[2, 1],
[0, 1]])
compute_1_area = plg.Polygon(bbox).area()
compute_2_area = cv2.contourArea(bbox)
#方式3
compute_3_peri = cv2.arcLength(bbox, True)
compute_1_peri, compute_2_peri = 0, 0
for i in range(bbox.shape[0]):
print('==bbox[i], bbox[i + 1]:', bbox[i], bbox[(i + 1) % bbox.shape[0]])
# 方式1
compute_1_dis = dist(bbox[i], bbox[(i + 1) % bbox.shape[0]])
print('===compute_1_dis:', compute_1_dis)
compute_1_peri += compute_1_dis
# 方式2
compute_2_dis = np.linalg.norm(bbox[i] - bbox[(i + 1) % bbox.shape[0]])
compute_2_peri += compute_2_dis
print('===compute_2_dis:', compute_2_dis)
print('方式1计算面积为:{},方式2计算面积为:{}'.format(compute_1_area, compute_2_area))
print('方式1计算周长:{},方式2计算周长:{},方式3计算周长:{}'.format(compute_1_peri, compute_2_peri, compute_3_peri))
56.np.linalg.eig计算特征值和特征向量
x = np.diag((1, 2, 3))
print('x:\n', x)
w, v = np.linalg.eig(x)
print('w:\n', w)
print('v:\n', v)
57.奇异值分解np.linalg.svd
# 创建一个矩阵A: A = u s vt
# A = np.array([[0, 1],
# [1, 1],
# [1, 0]])
A = np.array([[4, 1, 3],
[2, 2, 4]])
print('A:\n', A)
# 对其进行SVD分解 s是对角矩阵的值
u, s, vt = np.linalg.svd(A, full_matrices=True)
print('u.shape, s.shape, vt.shape:', u.shape, s.shape, vt.shape)
res = np.dot(u*s, vt[:2, :])
print('res:', res)
#U UT=I
print('np.dot(u, u.T):\n', np.dot(u, u.T))
#v vT=I
print('np.dot(vt, vt.T):\n', np.dot(vt, vt.T))
58. np实现avg_pool和max_pool
import numpy as np
def pooling(inputMap, k = 3, s=1, pad=False, mode='max'):
"""
:param inputMap:
:param k:
:param s:
:param pad:
:return:
"""
# inputMap sizes
h, w = np.shape(inputMap)
if pad:
new_h, new_w = h, w
else:
new_h, new_w = (h - k)//s + 1, (w - k)//s + 1
outputMap = np.zeros((new_h, new_w))
# padding
if pad:
padding = [(0, (h*s - s + k - h)//2 + 1), (0, (h*s - s + k - h)//2+1)]
temp_map = np.pad(inputMap, padding, mode='constant', constant_values=0)
else:
temp_map = inputMap
print('===temp_map:', temp_map)
# # max pooling
for i in range(0, new_h):
for j in range(0, new_w):
start_y = i * s
start_x = j * s
pool_region = temp_map[start_y:start_y+k, start_x:start_x+k]
# print('===pool_region:', pool_region)
if mode == 'max':
value = np.max(pool_region)
else:
value = np.mean(pool_region)
outputMap[i, j] = value
print('==outputMap:', outputMap)
return outputMap
# 测试实例
# test = np.array([[1, 2, 3, 4],
# [5, 6, 7, 8],
# [9, 10, 11, 12],
# [10, 11, 13, 14]])
test = np.array([[1, 2, 3, 4, 5],
[5, 6, 7, 8, 9],
[9, 10, 11, 12, 13],
[10, 11, 13, 14, 15],
[10, 11, 13, 14, 15]])
test_result = pooling(test, k=3, s=1, pad=False, mode='max')
print('==test_result:', test_result)
59.实现高斯分布
import numpy as np
def get_gaussian_kernel(k=3, mu=0, sigma=1, normalize=True):
# compute 1 dimension gaussian
gaussian_1D = np.linspace(-1, 1, k)
print('===gaussian_1D:', gaussian_1D)
# compute a grid distance from center
x, y = np.meshgrid(gaussian_1D, gaussian_1D)
print('===x:', x)
print('===y:', y)
distance = (x ** 2 + y ** 2) ** 0.5
print('==distance:', distance)
# compute the 2 dimension gaussian
gaussian_2D = np.exp(-(distance - mu) ** 2 / (2 * sigma ** 2))
gaussian_2D = gaussian_2D / (2 * np.pi *sigma **2)
print('==gaussian_2D:\n', gaussian_2D)
# normalize part (mathematically)
if normalize:
gaussian_2D = gaussian_2D / np.sum(gaussian_2D)
return gaussian_2D
get_gaussian_kernel()
60.二维图片(图像索引)变三维(颜色)
常用于分割
seg = np.array([[0, 2, 3],
[0, 1, 4]])
color_seg = np.zeros((seg.shape[0], seg.shape[1], 3), dtype=np.uint8)
palette = [[0, 0, 0], [0, 0, 255], [0, 255, 0], [255, 0, 0], [255, 255, 0]]
for label, color in enumerate(palette):
color_seg[seg == label, :] = color
print('==color_seg:', color_seg)
61.求解多元一次方程组
def test_np_slove():
a = np.array([[1, 2, 3],
[5, 23, 5],
[4, 7, 9]])
b = np.array([10,
20,
30])
x = np.linalg.solve(a, b)
print(x)
print(np.sum(np.dot(a, x.reshape(3, 1)), axis=1))
62.利用数组索引获取每个值
target = np.array([[1, 2, 3],
[4, 5, 6]])
idx = np.array([[0, 1],
[1, 0],
[0, 2],
[0, 0],
[1, 1],
[1, 2]])
print('target[idx[:, 0], idx[:, 1]]', target[idx[:, 0], idx[:, 1]])
63.softmax
def softmax(f, axis=1):
f -= np.max(f, axis=axis, keepdims=True)
return np.exp(f) / np.sum(np.exp(f), axis=axis, keepdims=True)