J. Zheng, L. Li, Q. Lin and Z. Ming, “An Improved Weighted Optimization-based Framework for Large-scale MOPs,” 2021 IEEE Congress on Evolutionary Computation (CEC), 2021, pp. 2156-2163, doi: 10.1109/CEC45853.2021.9504854.
摘要
针对大规模多目标优化问题,提出了一种改进的加权优化框架。
1、引言
More recently, large-scale MOPs (LSMOPs)have become a hot topic in multiobjective optimization, which indicates the optimization problems that involve a large number of decision variables. Compared to the normal MOPs, LSMOPs are much more difficult to be solved, as they have the exponentially increased number of decision variables in search space. As a result, most existing MOEAs show their limitations on tackling LSMOPs, since their search ability is far away from enough to find the true PS in such a huge search space.
To alleviate this issue and enhance the search ability of MOEAs on solving LSMOPs, an improved WOF framework, namely iWOF, is designed in this paper.
近年来,大规模多目标优化(LSMOPs)成为多目标优化中的一个热点问题,它指的是涉及大量决策变量的优化问题。与普通的MOPs相比,LSMOPs的求解难度要大得多,因为LSMOPs在搜索空间中决策变量的数量呈指数增长。因此,大多数现有的MOEAs在处理LSMOPs方面都存在局限性,因为它们的搜索能力远远不足以在如此巨大的搜索空间中找到真正的PS。
为了解决这一问题,提高MOEAs在求解LSMOPs时的搜索能力,本文设计了一种改进的WOF框架,即iWOF。
本文的主要贡献在于:
- 为了提高求解LSMOPs的性能,提出了一种新的进化搜索策略,在面对不同特性的复杂问题时,可以有效地提高性能。
- 本文提出了一种改进的WOF结构。
2、前期工作
2.1 大规模优化简评
略。
2.2 动机
Particularly, an effective framework based on problem transformation is proposed, namely WOF [19], in which a problem transformation scheme is applied to reduce the dimensionality of the search space. However, the evolving numbers for both weight optimization and original optimization are fixed and only one single search operator is adopted in WOF, which may be not so efficient for solving various LSMOPs. To solve the above shortcomings, an improved WOF framework called iWOF is proposed in this article, aiming to improve the performance of WOF.
特别地,提出了一种基于问题变换的有效框架,即WOF,其中采用问题变换方案来降低搜索空间的维数。然而,由于权重优化和原优化的演化数都是固定的,且WOF中只采用了一个搜索算子,这对于求解各种LSMOPs可能不是很有效。针对上述不足,本文提出了一种改进的WOF框架,即iWOF,旨在提高WOF的性能。
3、提出的算法
3.1 iWOF框架
框架如图:
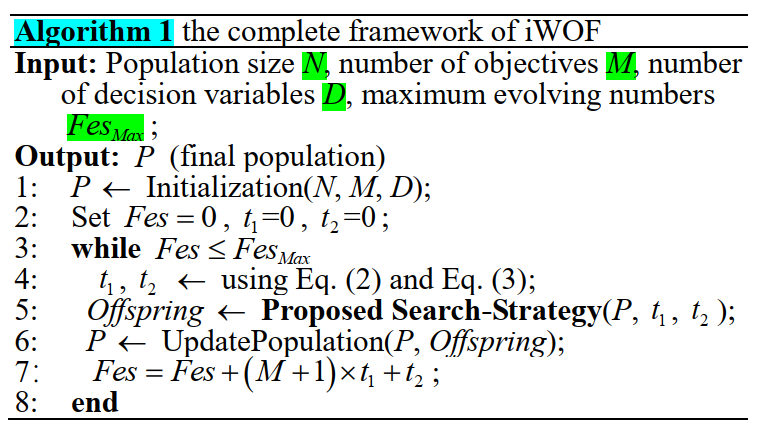
种群初始化(line 1)、参数设置(line 2);
进入主循环:执行动态调整策略(line 3),根据公式1和公式2算出t1和t2(line 4),对种群 P P P执行提出的搜索策略(line 5),采用与WOF相同的种群更新策略(line 6,这里还是要看一下原文,一两句话说不清楚),更新当前的评估次数(line 7);
最后输出非支配解。
3.2 基于进化次数的动态调整策略
与原有WOF不同,iWOF中的两种优化方法,即权值优化方法和原有优化方法,采用基于当前进化阶段调整进化数的动态调整策略,动态调整进化数。需要注意的是,本文采用的权值优化方法与WOF中的相同。
t 1 = { 500 × (