论文标题
S2WAT: Image Style Transfer via Hierarchical Vision Transformer Using Strips Window Attention
S2WAT: 基于条带窗口注意力的分层视觉Transformer图像风格迁移
论文链接
S2WAT: Image Style Transfer via Hierarchical Vision Transformer Using Strips Window Attention论文下载
论文作者
Chiyu Zhang, Xiaogang Xu, Lei Wang, Zaiyan Dai, Jun Yang
内容简介
本文提出了一种新的图像风格迁移框架,称为条带窗口注意力Transformer(S2WAT),旨在解决传统Transformer在局部建模方面的不足。S2WAT通过引入多种窗口形状的注意力计算,能够同时捕获短距离和长距离的依赖关系。该方法采用“Attn Merge”策略,动态确定不同窗口注意力的空间权重,从而有效整合局部和全局特征。通过在多个代表性数据集上的广泛实验,S2WAT在风格迁移任务中表现出色,超越了现有的最先进方法。本文的贡献在于提出了一种新颖的分层Transformer架构,能够同时处理多种风格,并有效缓解局部性问题。
分点关键点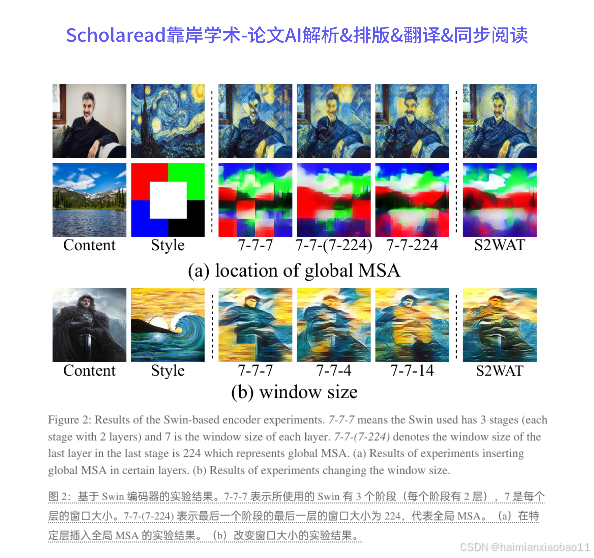
-
S2WAT框架
- S2WAT是一个分层Transformer框架,专门设计用于图像风格迁移。它通过条带窗口注意力机制(SpW Attention)来捕获局部和全局特征,解决了传统方法在局部建模上的不足。
-
条带窗口注意力机制
- SpW Attention结合了三种窗口类型:水平条带、垂直条带和方形窗口。通过这些不同形状的窗口,S2WAT能够在提取非局部特征的同时,关注局部信息,从而实现更好的风格迁移效果。
-
Attn Merge策略
- “Attn Merge”方法通过计算不同窗口注意力之间的空间相关性,动态合并注意力输出。这种方法相较于静态合并策略(如求和和拼接)具有更高的灵活性和有效性,能够增强风格迁移的效果。
-
实验结果与性能
- 在多个公开数据集上的实验表明,S2WAT在风格迁移任务中表现出色,达到了最先进的性能。通过定量和定性分析,验证了该框架的有效性和实用性。
- 在多个公开数据集上的实验表明,S2WAT在风格迁移任务中表现出色,达到了最先进的性能。通过定量和定性分析,验证了该框架的有效性和实用性。
论文代码
代码链接:https://github.com/AlienZhang1996/S2WAT
中文关键词
- 图像风格迁移
- 分层Transformer
- 条带窗口注意力
- Attn Merge
- 短距离和长距离依赖
- 计算机视觉
AAAI论文合集:
希望这些论文能帮到你!如果觉得有用,记得点赞关注哦~ 后续还会更新更多论文合集!!