前言:在实际的json序列化过程中,json的dump(dumps)方式比较慢,浪费时间,有没有一种比较快速的替代方式(非自己手动实现并优化)? 使用优化后的orjson库代替json库
在实际的项目中 当我们序列化一个矩阵时,以CV任务中传入图像数组为例,比如传入的数组大小为 [10,640,640,3]
下面是两个不同json库的dumps时间:
1、Json
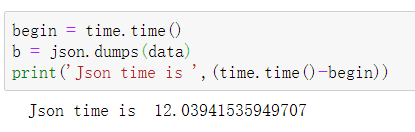
2、Orjson
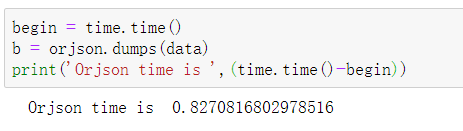
3、Conclution
总的来说,orjson库的处理方式比json的处理方式快了约14倍(在该例子中),可以作为json的替代品
参考:Choosing a faster JSON library for Python