Abstract
task:
social relation extraction(
SRE):
aims to infer
the social relation between two people
method:
FL-MSRE:a few-shot learning based approach to extractingsocial relations from both texts and face images
datasets:
presents three multimodal datasets annotated from four classical masterpieces and corresponding TV series
Introduction
SRE be of great value in reality:
can capture social connections and enable machines better
understand human behaviors
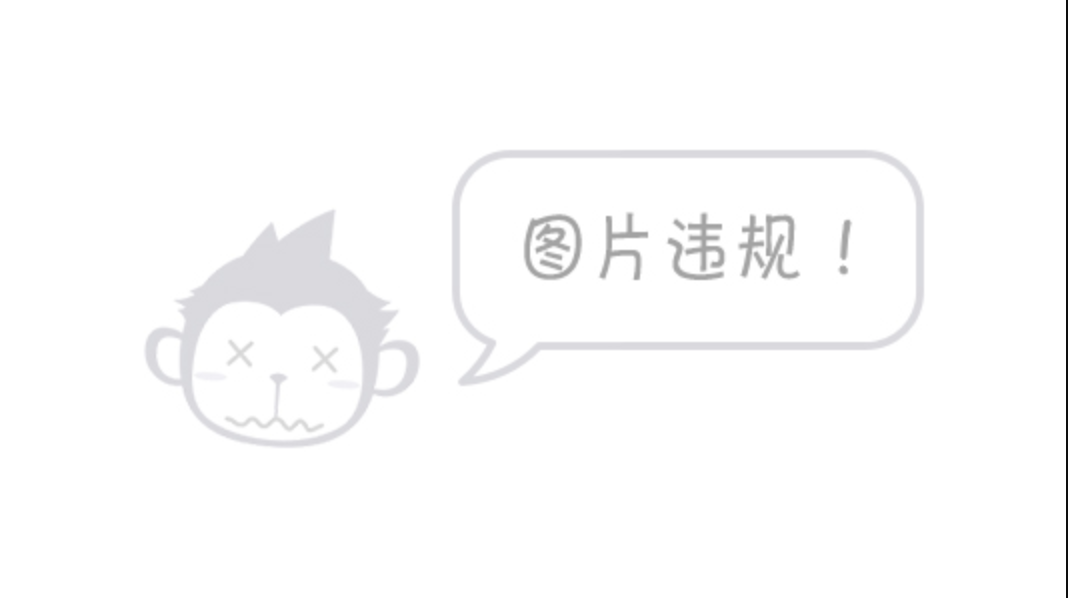
clarify the following two questions:
Can introducing face image information into a text-basedmodel improve the performance for SRE?
Can facial features extracted from different images
achieve similar performance as from the same image?
contributions:
present multimodal social relation datasets
propose a novel approach FL-MSRE for SRE
Extensive experiments demonstrate......
Related Work
task:
pres:unimodal(text only or image only) -> ours: multimodal(both text and image)
pres: multimodal(with only one image) -> ours: multimoday(a lists of images)
few-shot learning:
prototypical network
Multimodal Learning :略
Multimodal Social Relation Datasets 略
The Proposed Approach FL-MSRE
Problem Formulation
Every entity
e
consists of two parts:
a bounding box:
b
e
= (
x
1
, y
1
, x
2
, y
2
)
a character name:
c
e
follow the
N
way
K
shot setting
input tuple:(
s, h, t, g
h
, g
t
, r
)
denotes sentence、head entity、tail entity、the image containing the face of h、the image containing the face of t、the relation between h and t
Overview
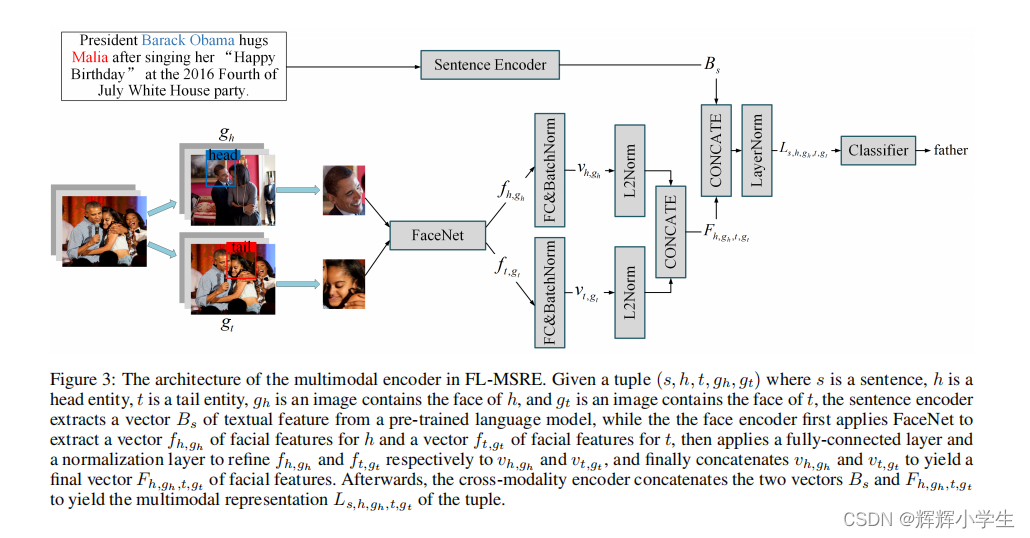
Multimodal Encoder
Sentence Encoder
Prototypical Network
Experiments
The Baseline Approach
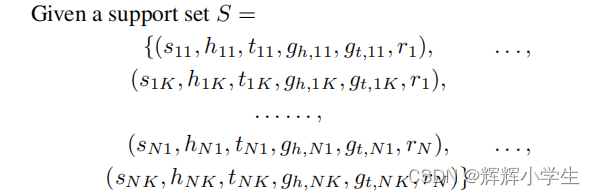
Experiments
The Baseline Approach :BERT (
The BERT encoder is also fifine-tuned with prototypical
network )
Image Sampling
two methods for image sampling: the same image&&different images(s have their own advantages)
Experiment Setting
Dataset Analysis and Splits
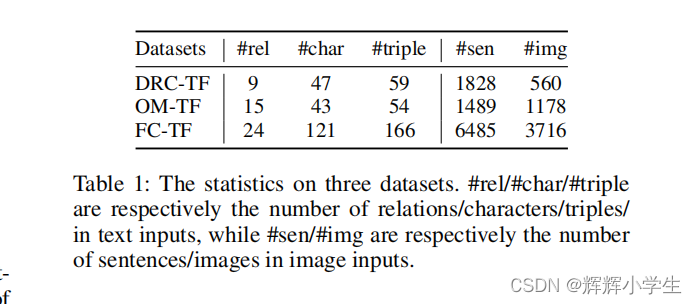
Implementation Details
Result Analysis

Cross-Dataset Analysis
Answering the Two Questions
Case Study
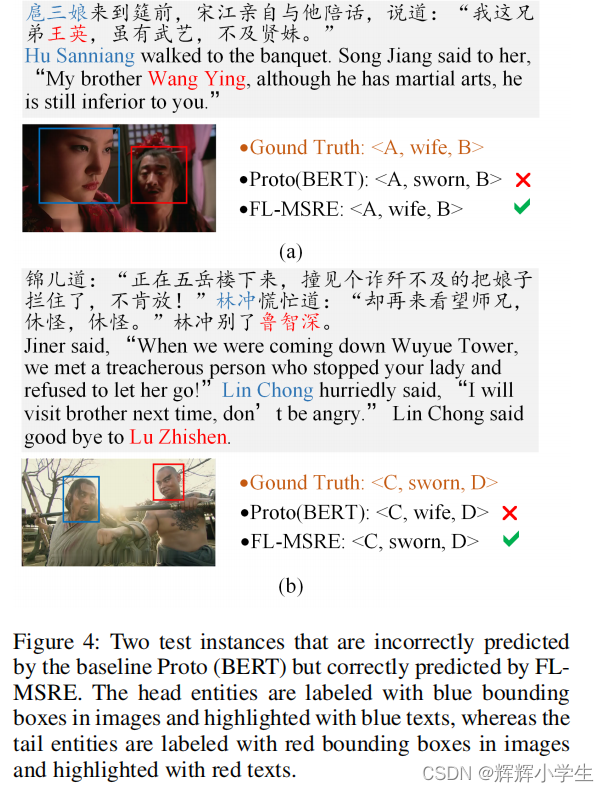
Conclusion 略