Keras运行GAN实例 2022.2.25
- 1、GAN简介
- 2、测试环境(Win11 64位 + GTX 1050Ti +CUDA 10.1 + cudnn 7.6.5 + Python 3.6 + tensorflow-gpu 2.3.1)
- 3、实例运行
- 3.1 [Adversarial Autoencoder](https://arxiv.org/abs/1511.05644)
- 3.2 [Auxiliary Classifier Generative Adversarial Network](https://arxiv.org/abs/1610.09585)
- 3.3 [Bidirectional Generative Adversarial Network](https://arxiv.org/abs/1605.09782)
- 3.4 [Boundary-Seeking Generative Adversarial Networks](https://arxiv.org/abs/1702.08431)
- 3.5 [Context-Conditional Generative Adversarial Networks](https://arxiv.org/abs/1611.06430)
- 3.6 [Conditional Generative Adversarial Nets](https://arxiv.org/abs/1411.1784)
- 3.7 [Coupled generative adversarial networks](https://arxiv.org/abs/1606.07536)
- 3.8 [Context Encoders: Feature Learning by Inpainting](https://arxiv.org/abs/1604.07379)
- 3.9 [Unpaired Image-to-Image Translation using Cycle-Consistent Adversarial Networks](https://arxiv.org/abs/1703.10593)
- 3.10 [Deep Convolutional Generative Adversarial Network](https://arxiv.org/abs/1511.06434)
- 3.11 [Discover Cross-Domain Relations with Generative Adversarial Networks](https://arxiv.org/abs/1703.05192)
- 3.12 [DualGAN: Unsupervised Dual Learning for Image-to-Image Translation](https://arxiv.org/abs/1704.02510)
- 3.13 [Generative Adversarial Network](https://arxiv.org/abs/1406.2661)
- 3.14 [InfoGAN: Interpretable Representation Learning by Information Maximizing Generative Adversarial Nets](https://arxiv.org/abs/1606.03657)
- 3.15 [Least Squares Generative Adversarial Networks](https://arxiv.org/abs/1611.04076)
- 3.16 [Image-to-Image Translation with Conditional Adversarial Networks](https://arxiv.org/abs/1611.07004)
- 3.17 [Unsupervised Pixel-Level Domain Adaptation with Generative Adversarial Networks](https://arxiv.org/abs/1612.05424)
- 3.18 [Wasserstein GAN](https://arxiv.org/abs/1701.07875)
- 4、应用总结
1、GAN简介
GAN
(Generative Adversarial Nets)是由Ian J. Goodfellow
等人在2014NIPS
会议上提出的一种网络,他们提出了一种利用对抗处理来估计生成式模型的新框架,该框架可同时训练两个模型:一个是生成模型G(Generator生成器),可等同于一个函数G(z)
;另一个是判别模型D(Descriminator判别器),也可等同于一个函数D(x)
。
生成器G
用于捕捉数据的分布规律从而生成新的假样本数据;判别器D
用来估计一个样本来自真实训练数据而非生成器G
。因此生成器Generator
的训练过程就是要最大化判别器Descriminator
的犯错概率,相当于生成器Generator要制造假样本,使得假样本尽可能地欺骗判别器Descriminator
,让判别器Descriminator
无法区分假样本到底是来自真实训练数据还是生成器,因此这个框架可认为是一个两个玩家间G
最小D
最大的游戏:G玩家要努力生成与真实样本尽可能接近的假样本,D玩家要准确判断一个样本是来自于真实数据还是G玩家,两个玩家在进行对抗,整个网络在进行对抗训练,从而最终让G玩家预测或生成尽可能新的样本数据。在任意的函数空间G和D,存在唯一解当且仅当G恢复了训练数据的分布,而此时D只等于
1
2
\frac{1}{2}
21。
1.1 理论分析
GAN
的原理就是用生成器G和判别器D进行对抗,本质上目的是去做预测和生成,要预测输入真实样本数据x
的概率分布p(x)
,预测的
x
—
—
输
入
的
真
实
样
本
数
据
x——输入的真实样本数据
x——输入的真实样本数据
z
—
—
噪
音
数
据
z——噪音数据
z——噪音数据
G
(
z
)
—
—
生
成
器
G(z)——生成器
G(z)——生成器
D
(
x
)
—
—
判
别
器
D(x)——判别器
D(x)——判别器
x
′
=
G
(
z
)
—
—
生
成
器
G
根
据
噪
音
z
生
成
的
假
样
本
x^{'} =G(z) ——生成器G根据噪音z 生成的假样本
x′=G(z)——生成器G根据噪音z生成的假样本
p
d
a
t
a
—
—
真
实
样
本
数
据
的
概
率
分
布
p_{data}——真实样本数据的概率分布
pdata——真实样本数据的概率分布
p
z
—
—
噪
音
数
据
的
先
验
概
率
分
布
,
由
生
成
器
G
来
隐
含
定
义
p_{z}——噪音数据的先验概率分布,由生成器G来隐含定义
pz——噪音数据的先验概率分布,由生成器G来隐含定义
p
g
—
—
生
成
器
G
预
估
真
实
样
本
数
据
的
概
率
分
布
p_{g}——生成器G预估真实样本数据的概率分布
pg——生成器G预估真实样本数据的概率分布
网
络
训
练
的
全
局
优
化
目
标
为
:
p
g
=
p
d
a
t
a
网络训练的全局优化目标为:p_{g} = p_{data}
网络训练的全局优化目标为:pg=pdata
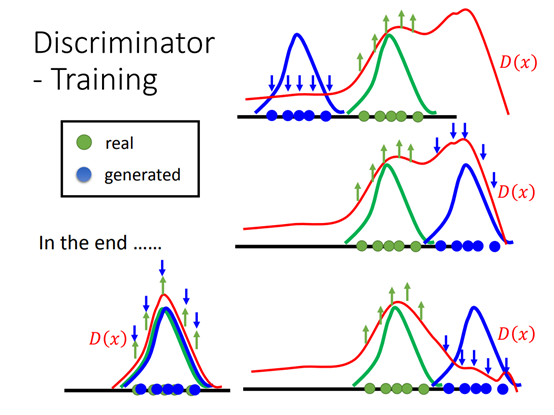
1.2 优缺点
GAN
的缺点主要包括:无法获得生成器预测样本数据概率分布
p
g
(
x
)
p_{g}(x)
pg(x)的明确显式表达;在训练期间,判别器D必须与生成器G保持较好的同步和更新。
GAN的
优势有:训练过程无需干预,大量函数都可应用到此模型,可计算性强,能够表示非常尖锐甚至退化的分布。进一步学习GAN
可参考:
- Goodfellow I, Pouget-Abadie J, Mirza M, et al. Generative adversarial nets[J]. Advances in neural information processing systems, 2014, 27.
- https://speech.ee.ntu.edu.tw/~tlkagk/slide/Tutorial_HYLee_GAN.pdf
- https://tensorflow.google.cn/tutorials/generative/style_transfer?hl=zh_cn
- https://keras.io/examples/generative/
- https://pytorch.org/tutorials/beginner/dcgan_faces_tutorial.html
2、测试环境(Win11 64位 + GTX 1050Ti +CUDA 10.1 + cudnn 7.6.5 + Python 3.6 + tensorflow-gpu 2.3.1)
本文测试环境是在Win11 64位
操作系统上进行的,显卡为GTX 1050Ti
,安装了CUDA 10.1
和cudnn7.6.5
,最后利用Anaconda
创建Python 3.6
的虚拟环境,利用pip
安装了所需的依赖包tensorflow-gpu 2.3.1
。
python 3.6
所安装的依赖包如下(pip install 包名==版本号
):
absl-py==1.0.0
astunparse==1.6.3
cachetools==4.2.4
certifi==2021.10.8
charset-normalizer==2.0.12
cycler==0.11.0
dataclasses==0.8
gast==0.3.3
google-auth==2.6.0
google-auth-oauthlib==0.4.6
google-pasta==0.2.0
grpcio==1.43.0
h5py==2.10.0
idna==3.3
importlib-metadata==4.8.3
Keras-Preprocessing==1.1.2
kiwisolver==1.3.1
Markdown==3.3.6
matplotlib==3.3.4
numpy==1.18.5
oauthlib==3.2.0
opt-einsum==3.3.0
pandas==1.1.5
Pillow==8.4.0
protobuf==3.19.4
pyasn1==0.4.8
pyasn1-modules==0.2.8
pyparsing==3.0.7
python-dateutil==2.8.2
pytz==2021.3
requests==2.27.1
requests-oauthlib==1.3.1
rsa==4.8
scipy==1.2.1
six==1.16.0
tensorboard==2.8.0
tensorboard-data-server==0.6.1
tensorboard-plugin-wit==1.8.1
tensorflow-addons==0.14.0
tensorflow-gpu==2.3.1
tensorflow-gpu-estimator==2.3.0
termcolor==1.1.0
typeguard==2.13.3
typing_extensions==4.1.1
urllib3==1.26.8
Werkzeug==2.0.3
wincertstore==0.2
wordcloud==1.8.1
wrapt==1.12.1
zipp==3.4.0
3、实例运行
所用实例均来自于eriklindernoren/Keras-GAN,为了进一步学习各种GAN的效果,尝试用示例代码运行,源码中训练迭代次数的值通常很大(3000~30000
),由于电脑性能较差特意减少了迭代次数(1000~2000
)来学习,因而运行结果可能不够好,无法证明模型效果不佳。
3.1 Adversarial Autoencoder
代码及运行结果
MergeLayer.py
from tensorflow.keras.layers import Layer
import tensorflow.keras.backend as K
class MergeLayer(Layer):
def __init__(self, **kwargs):
super(MergeLayer, self).__init__(**kwargs)
def compute_output_shape(self, input_shape):
return (input_shape[0][0], input_shape[0][1])
def call(self, x, mask=None):
final_output = x[0] + K.random_normal(K.shape(x[0])) * K.exp(x[1] / 2)
return final_output
aae.py
from __future__ import print_function, division
from MergeLayer import MergeLayer
from tensorflow import keras
from tensorflow.keras.datasets import mnist
from tensorflow.keras.layers import Input, Dense, Reshape, Flatten, Dropout, multiply, GaussianNoise
from tensorflow.keras.layers import BatchNormalization, Activation, Embedding, ZeroPadding2D
from tensorflow.keras.layers import MaxPooling2D, Concatenate
from tensorflow.keras.layers import LeakyReLU
from tensorflow.keras.layers import UpSampling2D, Conv2D
from tensorflow.keras.models import Sequential, Model
from tensorflow.keras.optimizers import Adam
from tensorflow.keras import losses
from tensorflow.keras.utils import to_categorical
import tensorflow.keras.backend as K
import matplotlib.pyplot as plt
import numpy as np
class AdversarialAutoencoder():
def __init__(self):
self.img_rows = 28
self.img_cols = 28
self.channels = 1
self.img_shape = (self.img_rows, self.img_cols, self.channels)
self.latent_dim = 10
optimizer = Adam(0.0002, 0.5)
# Build and compile the discriminator
self.discriminator = self.build_discriminator()
self.discriminator.compile(loss='binary_crossentropy',
optimizer=optimizer,
metrics=['accuracy'])
# Build the encoder / decoder
self.encoder = self.build_encoder()
self.decoder = self.build_decoder()
img = Input(shape=self.img_shape)
# The generator takes the image, encodes it and reconstructs it
# from the encoding
encoded_repr = self.encoder(img)
reconstructed_img = self.decoder(encoded_repr)
# For the adversarial_autoencoder model we will only train the generator
self.discriminator.trainable = False
# The discriminator determines validity of the encoding
validity = self.discriminator(encoded_repr)
# The adversarial_autoencoder model (stacked generator and discriminator)
self.adversarial_autoencoder = Model(img, [reconstructed_img, validity])
self.adversarial_autoencoder.compile(loss=['mse', 'binary_crossentropy'],
loss_weights=[0.999, 0.001],
optimizer=optimizer)
def build_encoder(self):
# Encoder
img = Input(shape=self.img_shape)
h = Flatten()(img)
h = Dense(512)(h)
h = LeakyReLU(alpha=0.2)(h)
h = Dense(512)(h)
h = LeakyReLU(alpha=0.2)(h)
mu = Dense(self.latent_dim)(h)
log_var = Dense(self.latent_dim)(h)
latent_repr = MergeLayer()([mu, log_var])
return Model(img, latent_repr)
def build_decoder(self):
model = Sequential()
model.add(Dense(512, input_dim=self.latent_dim))
model.add(LeakyReLU(alpha=0.2))
model.add(Dense(512))
model.add(LeakyReLU(alpha=0.2))
model.add(Dense(np.prod(self.img_shape), activation='tanh'))
model.add(Reshape(self.img_shape))
model.summary()
z = Input(shape=(self.latent_dim,))
img = model(z)
return Model(z, img)
def build_discriminator(self):
model = Sequential()
model.add(Dense(512, input_dim=self.latent_dim))
model.add(LeakyReLU(alpha=0.2))
model.add(Dense(256))
model.add(LeakyReLU(alpha=0.2))
model.add(Dense(1, activation="sigmoid"))
model.summary()
encoded_repr = Input(shape=(self.latent_dim, ))
validity = model(encoded_repr)
return Model(encoded_repr, validity)
def train(self, epochs, batch_size=128, sample_interval=50):
# Load the dataset
(X_train, _), (_, _) = mnist.load_data()
# Rescale -1 to 1
X_train = (X_train.astype(np.float32) - 127.5) / 127.5
X_train = np.expand_dims(X_train, axis=3)
# Adversarial ground truths
valid = np.ones((batch_size, 1))
fake = np.zeros((batch_size, 1))
for epoch in range(epochs):
# ---------------------
# Train Discriminator
# ---------------------
# Select a random batch of images
idx = np.random.randint(0, X_train.shape[0], batch_size)
imgs = X_train[idx]
latent_fake = self.encoder.predict(imgs)
latent_real = np.random.normal(size=(batch_size, self.latent_dim))
# Train the discriminator
d_loss_real = self.discriminator.train_on_batch(latent_real, valid)
d_loss_fake = self.discriminator.train_on_batch(latent_fake, fake)
d_loss = 0.5 * np.add(d_loss_real, d_loss_fake)
# ---------------------
# Train Generator
# ---------------------
# Train the generator
g_loss = self.adversarial_autoencoder.train_on_batch(imgs, [imgs, valid])
# Plot the progress
print ("%d [D loss: %f, acc: %.2f%%] [G loss: %f, mse: %f]" % (epoch, d_loss[0], 100*d_loss[1], g_loss[0], g_loss[1]))
# If at save interval => save generated image samples
if epoch % sample_interval == 0:
self.sample_images(epoch)
def sample_images(self, epoch):
r, c = 5, 5
z = np.random.normal(size=(r*c, self.latent_dim))
gen_imgs = self.decoder.predict(z)
gen_imgs = 0.5 * gen_imgs + 0.5
fig, axs = plt.subplots(r, c)
cnt = 0
for i in range(r):
for j in range(c):
axs[i,j].imshow(gen_imgs[cnt, :,:,0], cmap='gray')
axs[i,j].axis('off')
cnt += 1
fig.savefig("images/mnist_%d.png" % epoch)
plt.close()
def save_model(self):
def save(model, model_name):
model_path = "saved_model/%s.json" % model_name
weights_path = "saved_model/%s_weights.hdf5" % model_name
options = {"file_arch": model_path,
"file_weight": weights_path}
json_string = model.to_json()
open(options['file_arch'], 'w').write(json_string)
model.save_weights(options['file_weight'])
save(self.generator, "aae_generator")
save(self.discriminator, "aae_discriminator")
if __name__ == '__main__':
aae = AdversarialAutoencoder()
aae.train(epochs=10000, batch_size=32, sample_interval=200)
3.2 Auxiliary Classifier Generative Adversarial Network
代码及运行结果
acgan.py
from __future__ import print_function, division
from tensorflow.keras.datasets import mnist
from tensorflow.keras import layers
from tensorflow.keras.layers import Input, Dense, Reshape, Flatten, Dropout, multiply
from tensorflow.keras.layers import BatchNormalization, Activation, Embedding, ZeroPadding2D
from tensorflow.keras.layers import LeakyReLU
from tensorflow.keras.layers import UpSampling2D, Conv2D
from tensorflow.keras.models import Model,Sequential
from tensorflow.keras.optimizers import Adam
import matplotlib.pyplot as plt
import numpy as np
class ACGAN():
def __init__(self):
# Input shape
self.img_rows = 28
self.img_cols = 28
self.channels = 1
self.img_shape = (self.img_rows, self.img_cols, self.channels)
self.num_classes = 10
self.latent_dim = 100
optimizer = Adam(0.0002, 0.5)
losses = ['binary_crossentropy', 'sparse_categorical_crossentropy']
# Build and compile the discriminator
self.discriminator = self.build_discriminator()
self.discriminator.compile(loss=losses,
optimizer=optimizer,
metrics=['accuracy'])
# Build the generator
self.generator = self.build_generator()
# The generator takes noise and the target label as input
# and generates the corresponding digit of that label
noise = Input(shape=(self.latent_dim,))
label = Input(shape=(1,))
img = self.generator([noise, label])
# For the combined model we will only train the generator
self.discriminator.trainable = False
# The discriminator takes generated image as input and determines validity
# and the label of that image
valid, target_label = self.discriminator(img)
# The combined model (stacked generator and discriminator)
# Trains the generator to fool the discriminator
self.combined = Model([noise, label], [valid, target_label])
self.combined.compile(loss=losses,
optimizer=optimizer)
def build_generator(self):
model = Sequential()
{
model.add(Dense(128 * 7 * 7, activation="relu", input_dim=self.latent_dim)),
model.add(Reshape((7, 7, 128))),
model.add(BatchNormalization(momentum=0.8)),
model.add(UpSampling2D()),
model.add(Conv2D(128, kernel_size=3, padding="same")),
model.add(Activation("relu")),
model.add(BatchNormalization(momentum=0.8)),
model.add(UpSampling2D()),
model.add(Conv2D(64, kernel_size=3, padding="same")),
model.add(Activation("relu")),
model.add(BatchNormalization(momentum=0.8)),
model.add(Conv2D(self.channels, kernel_size=3, padding='same')),
model.add(Activation("tanh"))
}
model.summary()
noise = Input(shape=(self.latent_dim,))
label = Input(shape=(1,), dtype='int32')
label_embedding = Flatten()(Embedding(self.num_classes, self.latent_dim)(label))
model_input = multiply([noise, label_embedding])
img = model(model_input)
return Model([noise, label], img)
def build_discriminator(self):
model = Sequential()
model.add(Conv2D(16, kernel_size=3, strides=2, input_shape=self.img_shape, padding="same"))
model.add(LeakyReLU(alpha=0.2))
model.add(Dropout(0.25))
model.add(Conv2D(32, kernel_size=3, strides=2, padding="same"))
model.add(ZeroPadding2D(padding=((0,1),(0,1))))
model.add(LeakyReLU(alpha=0.2))
model.add(Dropout(0.25))
model.add(BatchNormalization(momentum=0.8))
model.add(Conv2D(64, kernel_size=3, strides=2, padding="same"))
model.add(LeakyReLU(alpha=0.2))
model.add(Dropout(0.25))
model.add(BatchNormalization(momentum=0.8))
model.add(Conv2D(128, kernel_size=3, strides=1, padding="same"))
model.add(LeakyReLU(alpha=0.2))
model.add(Dropout(0.25))
model.add(Flatten())
model.summary()
img = Input(shape=self.img_shape)
# Extract feature representation
features = model(img)
# Determine validity and label of the image
validity = Dense(1, activation="sigmoid")(features)
label = Dense(self.num_classes, activation="softmax")(features)
return Model(img, [validity, label])
def train(self, epochs, batch_size=128, sample_interval=50):
# Load the dataset
(X_train, y_train), (_, _) = mnist.load_data()
# Configure inputs
X_train = (X_train.astype(np.float32) - 127.5) / 127.5
X_train = np.expand_dims(X_train, axis=3)
y_train = y_train.reshape(-1, 1)
# Adversarial ground truths
valid = np.ones((batch_size, 1))
fake = np.zeros((batch_size, 1))
for epoch in range(epochs):
# ---------------------
# Train Discriminator
# ---------------------
# Select a random batch of images
idx = np.random.randint(0, X_train.shape[0], batch_size)
imgs = X_train[idx]
# Sample noise as generator input
noise = np.random.normal(0, 1, (batch_size, self.latent_dim))
# The labels of the digits that the generator tries to create an
# image representation of
sampled_labels = np.random.randint(0, 10, (batch_size, 1))
# Generate a half batch of new images
gen_imgs = self.generator.predict([noise, sampled_labels])
# Image labels. 0-9
img_labels = y_train[idx]
# Train the discriminator
d_loss_real = self.discriminator.train_on_batch(imgs, [valid, img_labels])
d_loss_fake = self.discriminator.train_on_batch(gen_imgs, [fake, sampled_labels])
d_loss = 0.5 * np.add(d_loss_real, d_loss_fake)
# ---------------------
# Train Generator
# ---------------------
# Train the generator
g_loss = self.combined.train_on_batch([noise, sampled_labels], [valid, sampled_labels])
# Plot the progress
print ("%d [D loss: %f, acc.: %.2f%%, op_acc: %.2f%%] [G loss: %f]" % (epoch, d_loss[0], 100*d_loss[3], 100*d_loss[4], g_loss[0]))
# If at save interval => save generated image samples
if epoch % sample_interval == 0:
self.save_model()
self.sample_images(epoch)
def sample_images(self, epoch):
r, c = 10, 10
noise = np.random.normal(0, 1, (r * c, self.latent_dim))
sampled_labels = np.array([num for _ in range(r) for num in range(c)])
gen_imgs = self.generator.predict([noise, sampled_labels])
# Rescale images 0 - 1
gen_imgs = 0.5 * gen_imgs + 0.5
fig, axs = plt.subplots(r, c)
cnt = 0
for i in range(r):
for j in range(c):
axs[i,j].imshow(gen_imgs[cnt,:,:,0], cmap='gray')
axs[i,j].axis('off')
cnt += 1
fig.savefig("images/%d.png" % epoch)
plt.close()
def save_model(self):
def save(model, model_name):
model_path = "saved_model/%s.json" % model_name
weights_path = "saved_model/%s_weights.hdf5" % model_name
options = {"file_arch": model_path,
"file_weight": weights_path}
json_string = model.to_json()
open(options['file_arch'], 'w').write(json_string)
model.save_weights(options['file_weight'])
save(self.generator, "generator")
save(self.discriminator, "discriminator")
if __name__ == '__main__':
acgan = ACGAN()
acgan.train(epochs=14000, batch_size=32, sample_interval=200)
3.3 Bidirectional Generative Adversarial Network
代码及运行结果
bigan.py
from __future__ import print_function, division
from tensorflow.keras.datasets import mnist
from tensorflow.keras.layers import Input, Dense, Reshape, Flatten, Dropout, multiply, GaussianNoise
from tensorflow.keras.layers import BatchNormalization, Activation, Embedding, ZeroPadding2D
from tensorflow.keras.layers import MaxPooling2D, concatenate
from tensorflow.keras.layers import LeakyReLU
from tensorflow.keras.layers import UpSampling2D, Conv2D
from tensorflow.keras.models import Sequential, Model
from tensorflow.keras.optimizers import Adam
from tensorflow.keras import losses
from tensorflow.keras.utils import to_categorical
import tensorflow.keras.backend as K
import matplotlib.pyplot as plt
import numpy as np
class BIGAN():
def __init__(self):
self.img_rows = 28
self.img_cols = 28
self.channels = 1
self.img_shape = (self.img_rows, self.img_cols, self.channels)
self.latent_dim = 100
optimizer = Adam(0.0002, 0.5)
# Build and compile the discriminator
self.discriminator = self.build_discriminator()
self.discriminator.compile(loss=['binary_crossentropy'],
optimizer=optimizer,
metrics=['accuracy'])
# Build the generator
self.generator = self.build_generator()
# Build the encoder
self.encoder = self.build_encoder()
# The part of the bigan that trains the discriminator and encoder
self.discriminator.trainable = False
# Generate image from sampled noise
z = Input(shape=(self.latent_dim, ))
img_ = self.generator(z)
# Encode image
img = Input(shape=self.img_shape)
z_ = self.encoder(img)
# Latent -> img is fake, and img -> latent is valid
fake = self.discriminator([z, img_])
valid = self.discriminator([z_, img])
# Set up and compile the combined model
# Trains generator to fool the discriminator
self.bigan_generator = Model([z, img], [fake, valid])
self.bigan_generator.compile(loss=['binary_crossentropy', 'binary_crossentropy'],
optimizer=optimizer)
def build_encoder(self):
model = Sequential()
model.add(Flatten(input_shape=self.img_shape))
model.add(Dense(512))
model.add(LeakyReLU(alpha=0.2))
model.add(BatchNormalization(momentum=0.8))
model.add(Dense(512))
model.add(LeakyReLU(alpha=0.2))
model.add(BatchNormalization(momentum=0.8))
model.add(Dense(self.latent_dim))
model.summary()
img = Input(shape=self.img_shape)
z = model(img)
return Model(img, z)
def build_generator(self):
model = Sequential()
model.add(Dense(512, input_dim=self.latent_dim))
model.add(LeakyReLU(alpha=0.2))
model.add(BatchNormalization(momentum=0.8))
model.add(Dense(512))
model.add(LeakyReLU(alpha=0.2))
model.add(BatchNormalization(momentum=0.8))
model.add(Dense(np.prod(self.img_shape), activation='tanh'))
model.add(Reshape(self.img_shape))
model.summary()
z = Input(shape=(self.latent_dim,))
gen_img = model(z)
return Model(z, gen_img)
def build_discriminator(self):
z = Input(shape=(self.latent_dim, ))
img = Input(shape=self.img_shape)
d_in = concatenate([z, Flatten()(img)])
model = Dense(1024)(d_in)
model = LeakyReLU(alpha=0.2)(model)
model = Dropout(0.5)(model)
model = Dense(1024)(model)
model = LeakyReLU(alpha=0.2)(model)
model = Dropout(0.5)(model)
model = Dense(1024)(model)
model = LeakyReLU(alpha=0.2)(model)
model = Dropout(0.5)(model)
validity = Dense(1, activation="sigmoid")(model)
return Model([z, img], validity)
def train(self, epochs, batch_size=128, sample_interval=50):
# Load the dataset
(X_train, _), (_, _) = mnist.load_data()
# Rescale -1 to 1
X_train = (X_train.astype(np.float32) - 127.5) / 127.5
X_train = np.expand_dims(X_train, axis=3)
# Adversarial ground truths
valid = np.ones((batch_size, 1))
fake = np.zeros((batch_size, 1))
for epoch in range(epochs):
# ---------------------
# Train Discriminator
# ---------------------
# Sample noise and generate img
z = np.random.normal(size=(batch_size, self.latent_dim))
imgs_ = self.generator.predict(z)
# Select a random batch of images and encode
idx = np.random.randint(0, X_train.shape[0], batch_size)
imgs = X_train[idx]
z_ = self.encoder.predict(imgs)
# Train the discriminator (img -> z is valid, z -> img is fake)
d_loss_real = self.discriminator.train_on_batch([z_, imgs], valid)
d_loss_fake = self.discriminator.train_on_batch([z, imgs_], fake)
d_loss = 0.5 * np.add(d_loss_real, d_loss_fake)
# ---------------------
# Train Generator
# ---------------------
# Train the generator (z -> img is valid and img -> z is is invalid)
g_loss = self.bigan_generator.train_on_batch([z, imgs], [valid, fake])
# Plot the progress
print ("%d [D loss: %f, acc: %.2f%%] [G loss: %f]" % (epoch, d_loss[0], 100*d_loss[1], g_loss[0]))
# If at save interval => save generated image samples
if epoch % sample_interval == 0:
self.sample_interval(epoch)
def sample_interval(self, epoch):
r, c = 5, 5
z = np.random.normal(size=(25, self.latent_dim))
gen_imgs = self.generator.predict(z)
gen_imgs = 0.5 * gen_imgs + 0.5
fig, axs = plt.subplots(r, c)
cnt = 0
for i in range(r):
for j in range(c):
axs[i,j].imshow(gen_imgs[cnt, :,:,0], cmap='gray')
axs[i,j].axis('off')
cnt += 1
fig.savefig("images/mnist_%d.png" % epoch)
plt.close()
if __name__ == '__main__':
bigan = BIGAN()
bigan.train(epochs=2000, batch_size=32, sample_interval=400)
3.4 Boundary-Seeking Generative Adversarial Networks
代码及运行结果
bgan.py
from __future__ import print_function, division
from tensorflow.keras.datasets import mnist
from tensorflow.keras.layers import Input, Dense, Reshape, Flatten, Dropout
from tensorflow.keras.layers import BatchNormalization, Activation, ZeroPadding2D
from tensorflow.keras.layers import LeakyReLU
from tensorflow.keras.layers import UpSampling2D, Conv2D
from tensorflow.keras.models import Sequential, Model
from tensorflow.keras.optimizers import Adam
import tensorflow.keras.backend as K
import matplotlib.pyplot as plt
import sys
import numpy as np
class BGAN():
"""Reference: https://wiseodd.github.io/techblog/2017/03/07/boundary-seeking-gan/"""
def __init__(self):
self.img_rows = 28
self.img_cols = 28
self.channels = 1
self.img_shape = (self.img_rows, self.img_cols, self.channels)
self.latent_dim = 100
optimizer = Adam(0.0002, 0.5)
# Build and compile the discriminator
self.discriminator = self.build_discriminator()
self.discriminator.compile(loss='binary_crossentropy',
optimizer=optimizer,
metrics=['accuracy'])
# Build the generator
self.generator = self.build_generator()
# The generator takes noise as input and generated imgs
z = Input(shape=(self.latent_dim,))
img = self.generator(z)
# For the combined model we will only train the generator
self.discriminator.trainable = False
# The valid takes generated images as input and determines validity
valid = self.discriminator(img)
# The combined model (stacked generator and discriminator)
# Trains the generator to fool the discriminator
self.combined = Model(z, valid)
self.combined.compile(loss=self.boundary_loss, optimizer=optimizer)
def build_generator(self):
model = Sequential()
model.add(Dense(256, input_dim=self.latent_dim))
model.add(LeakyReLU(alpha=0.2))
model.add(BatchNormalization(momentum=0.8))
model.add(Dense(512))
model.add(LeakyReLU(alpha=0.2))
model.add(BatchNormalization(momentum=0.8))
model.add(Dense(1024))
model.add(LeakyReLU(alpha=0.2))
model.add(BatchNormalization(momentum=0.8))
model.add(Dense(np.prod(self.img_shape), activation='tanh'))
model.add(Reshape(self.img_shape))
model.summary()
noise = Input(shape=(self.latent_dim,))
img = model(noise)
return Model(noise, img)
def build_discriminator(self):
model = Sequential()
model.add(Flatten(input_shape=self.img_shape))
model.add(Dense(512))
model.add(LeakyReLU(alpha=0.2))
model.add(Dense(256))
model.add(LeakyReLU(alpha=0.2))
model.add(Dense(1, activation='sigmoid'))
model.summary()
img = Input(shape=self.img_shape)
validity = model(img)
return Model(img, validity)
def boundary_loss(self, y_true, y_pred):
"""
Boundary seeking loss.
Reference: https://wiseodd.github.io/techblog/2017/03/07/boundary-seeking-gan/
"""
return 0.5 * K.mean((K.log(y_pred) - K.log(1 - y_pred))**2)
def train(self, epochs, batch_size=128, sample_interval=50):
# Load the dataset
(X_train, _), (_, _) = mnist.load_data()
# Rescale -1 to 1
X_train = X_train / 127.5 - 1.
X_train = np.expand_dims(X_train, axis=3)
# Adversarial ground truths
valid = np.ones((batch_size, 1))
fake = np.zeros((batch_size, 1))
for epoch in range(epochs):
# ---------------------
# Train Discriminator
# ---------------------
# Select a random batch of images
idx = np.random.randint(0, X_train.shape[0], batch_size)
imgs = X_train[idx]
noise = np.random.normal(0, 1, (batch_size, self.latent_dim))
# Generate a batch of new images
gen_imgs = self.generator.predict(noise)
# Train the discriminator
d_loss_real = self.discriminator.train_on_batch(imgs, valid)
d_loss_fake = self.discriminator.train_on_batch(gen_imgs, fake)
d_loss = 0.5 * np.add(d_loss_real, d_loss_fake)
# ---------------------
# Train Generator
# ---------------------
g_loss = self.combined.train_on_batch(noise, valid)
# Plot the progress
print ("%d [D loss: %f, acc.: %.2f%%] [G loss: %f]" % (epoch, d_loss[0], 100*d_loss[1], g_loss))
# If at save interval => save generated image samples
if epoch % sample_interval == 0:
self.sample_images(epoch)
def sample_images(self, epoch):
r, c = 5, 5
noise = np.random.normal(0, 1, (r * c, self.latent_dim))
gen_imgs = self.generator.predict(noise)
# Rescale images 0 - 1
gen_imgs = 0.5 * gen_imgs + 0.5
fig, axs = plt.subplots(r, c)
cnt = 0
for i in range(r):
for j in range(c):
axs[i,j].imshow(gen_imgs[cnt, :,:,0], cmap='gray')
axs[i,j].axis('off')
cnt += 1
fig.savefig("images/mnist_%d.png" % epoch)
plt.close()
if __name__ == '__main__':
bgan = BGAN()
bgan.train(epochs=3000, batch_size=32, sample_interval=200)
3.5 Context-Conditional Generative Adversarial Networks
代码及运行结果
instancenormalization.py
from tensorflow.keras.layers import Layer, InputSpec
from tensorflow.keras import initializers, regularizers, constraints
from tensorflow.keras import backend as K
class InstanceNormalization(Layer):
"""Instance normalization layer.
Normalize the activations of the previous layer at each step,
i.e. applies a transformation that maintains the mean activation
close to 0 and the activation standard deviation close to 1.
# Arguments
axis: Integer, the axis that should be normalized
(typically the features axis).
For instance, after a `Conv2D` layer with
`data_format="channels_first"`,
set `axis=1` in `InstanceNormalization`.
Setting `axis=None` will normalize all values in each
instance of the batch.
Axis 0 is the batch dimension. `axis` cannot be set to 0 to avoid errors.
epsilon: Small float added to variance to avoid dividing by zero.
center: If True, add offset of `beta` to normalized tensor.
If False, `beta` is ignored.
scale: If True, multiply by `gamma`.
If False, `gamma` is not used.
When the next layer is linear (also e.g. `nn.relu`),
this can be disabled since the scaling
will be done by the next layer.
beta_initializer: Initializer for the beta weight.
gamma_initializer: Initializer for the gamma weight.
beta_regularizer: Optional regularizer for the beta weight.
gamma_regularizer: Optional regularizer for the gamma weight.
beta_constraint: Optional constraint for the beta weight.
gamma_constraint: Optional constraint for the gamma weight.
# Input shape
Arbitrary. Use the keyword argument `input_shape`
(tuple of integers, does not include the samples axis)
when using this layer as the first layer in a Sequential model.
# Output shape
Same shape as input.
# References
- [Layer Normalization](https://arxiv.org/abs/1607.06450)
- [Instance Normalization: The Missing Ingredient for Fast Stylization](
https://arxiv.org/abs/1607.08022)
"""
def __init__(self,
axis=None,
epsilon=1e-3,
center=True,
scale=True,
beta_initializer='zeros',
gamma_initializer='ones',
beta_regularizer=None,
gamma_regularizer=None,
beta_constraint=None,
gamma_constraint=None,
**kwargs):
super(InstanceNormalization, self).__init__(**kwargs)
self.supports_masking = True
self.axis = axis
self.epsilon = epsilon
self.center = center
self.scale = scale
self.beta_initializer = initializers.get(beta_initializer)
self.gamma_initializer = initializers.get(gamma_initializer)
self.beta_regularizer = regularizers.get(beta_regularizer)
self.gamma_regularizer = regularizers.get(gamma_regularizer)
self.beta_constraint = constraints.get(beta_constraint)
self.gamma_constraint = constraints.get(gamma_constraint)
def build(self, input_shape):
ndim = len(input_shape)
if self.axis == 0:
raise ValueError('Axis cannot be zero')
if (self.axis is not None) and (ndim == 2):
raise ValueError('Cannot specify axis for rank 1 tensor')
self.input_spec = InputSpec(ndim=ndim)
if self.axis is None:
shape = (1,)
else:
shape = (input_shape[self.axis],)
if self.scale:
self.gamma = self.add_weight(shape=shape,
name='gamma',
initializer=self.gamma_initializer,
regularizer=self.gamma_regularizer,
constraint=self.gamma_constraint)
else:
self.gamma = None
if self.center:
self.beta = self.add_weight(shape=shape,
name='beta',
initializer=self.beta_initializer,
regularizer=self.beta_regularizer,
constraint=self.beta_constraint)
else:
self.beta = None
self.built = True
def call(self, inputs, training=None):
input_shape = K.int_shape(inputs)
reduction_axes = list(range(0, len(input_shape)))
if self.axis is not None:
del reduction_axes[self.axis]
del reduction_axes[0]
mean = K.mean(inputs, reduction_axes, keepdims=True)
stddev = K.std(inputs, reduction_axes, keepdims=True) + self.epsilon
normed = (inputs - mean) / stddev
broadcast_shape = [1] * len(input_shape)
if self.axis is not None:
broadcast_shape[self.axis] = input_shape[self.axis]
if self.scale:
broadcast_gamma = K.reshape(self.gamma, broadcast_shape)
normed = normed * broadcast_gamma
if self.center:
broadcast_beta = K.reshape(self.beta, broadcast_shape)
normed = normed + broadcast_beta
return normed
def get_config(self):
config = {
'axis': self.axis,
'epsilon': self.epsilon,
'center': self.center,
'scale': self.scale,
'beta_initializer': initializers.serialize(self.beta_initializer),
'gamma_initializer': initializers.serialize(self.gamma_initializer),
'beta_regularizer': regularizers.serialize(self.beta_regularizer),
'gamma_regularizer': regularizers.serialize(self.gamma_regularizer),
'beta_constraint': constraints.serialize(self.beta_constraint),
'gamma_constraint': constraints.serialize(self.gamma_constraint)
}
base_config = super(InstanceNormalization, self).get_config()
return dict(list(base_config.items()) + list(config.items()))
ccgan.py
from __future__ import print_function, division
from tensorflow.keras.datasets import mnist
from instancenormalization import InstanceNormalization # 下载的instancenormalization.py导入到这里,不用pip install keras-contrib
from tensorflow.keras.layers import Input, Dense, Reshape, Flatten, Dropout, multiply, GaussianNoise
from tensorflow.keras.layers import BatchNormalization, Activation, Embedding, ZeroPadding2D
from tensorflow.keras.layers import Concatenate
from tensorflow.keras.layers import LeakyReLU
from tensorflow.keras.layers import UpSampling2D, Conv2D
from tensorflow.keras.models import Sequential, Model
from tensorflow.keras.optimizers import Adam
from tensorflow.keras import losses
from tensorflow.keras.utils import to_categorical
import tensorflow.keras.backend as K
import scipy
import scipy.misc
import matplotlib.pyplot as plt
import numpy as np
class CCGAN():
def __init__(self):
self.img_rows = 32
self.img_cols = 32
self.channels = 1
self.img_shape = (self.img_rows, self.img_cols, self.channels)
self.mask_height = 10
self.mask_width = 10
self.num_classes = 10
# Number of filters in first layer of generator and discriminator
self.gf = 32
self.df = 32
optimizer = Adam(0.0002, 0.5)
# Build and compile the discriminator
self.discriminator = self.build_discriminator()
self.discriminator.compile(loss=['mse', 'categorical_crossentropy'],
loss_weights=[0.5, 0.5],
optimizer=optimizer,
metrics=['accuracy'])
# Build the generator
self.generator = self.build_generator()
# The generator takes noise as input and generates imgs
masked_img = Input(shape=self.img_shape)
gen_img = self.generator(masked_img)
# For the combined model we will only train the generator
self.discriminator.trainable = False
# The valid takes generated images as input and determines validity
valid, _ = self.discriminator(gen_img)
# The combined model (stacked generator and discriminator)
# Trains the generator to fool the discriminator
self.combined = Model(masked_img , valid)
self.combined.compile(loss=['mse'],
optimizer=optimizer)
def build_generator(self):
"""U-Net Generator"""
def conv2d(layer_input, filters, f_size=4, bn=True):
"""Layers used during downsampling"""
d = Conv2D(filters, kernel_size=f_size, strides=2, padding='same')(layer_input)
d = LeakyReLU(alpha=0.2)(d)
if bn:
d = BatchNormalization(momentum=0.8)(d)
return d
def deconv2d(layer_input, skip_input, filters, f_size=4, dropout_rate=0):
"""Layers used during upsampling"""
u = UpSampling2D(size=2)(layer_input)
u = Conv2D(filters, kernel_size=f_size, strides=1, padding='same', activation='relu')(u)
if dropout_rate:
u = Dropout(dropout_rate)(u)
u = BatchNormalization(momentum=0.8)(u)
u = Concatenate()([u, skip_input])
return u
img = Input(shape=self.img_shape)
# Downsampling
d1 = conv2d(img, self.gf, bn=False)
d2 = conv2d(d1, self.gf*2)
d3 = conv2d(d2, self.gf*4)
d4 = conv2d(d3, self.gf*8)
# Upsampling
u1 = deconv2d(d4, d3, self.gf*4)
u2 = deconv2d(u1, d2, self.gf*2)
u3 = deconv2d(u2, d1, self.gf)
u4 = UpSampling2D(size=2)(u3)
output_img = Conv2D(self.channels, kernel_size=4, strides=1, padding='same', activation='tanh')(u4)
return Model(img, output_img)
def build_discriminator(self):
img = Input(shape=self.img_shape)
model = Sequential()
model.add(Conv2D(64, kernel_size=4, strides=2, padding='same', input_shape=self.img_shape))
model.add(LeakyReLU(alpha=0.8))
model.add(Conv2D(128, kernel_size=4, strides=2, padding='same'))
model.add(LeakyReLU(alpha=0.2))
model.add(InstanceNormalization())
model.add(Conv2D(256, kernel_size=4, strides=2, padding='same'))
model.add(LeakyReLU(alpha=0.2))
model.add(InstanceNormalization())
model.summary()
img = Input(shape=self.img_shape)
features = model(img)
validity = Conv2D(1, kernel_size=4, strides=1, padding='same')(features)
label = Flatten()(features)
label = Dense(self.num_classes+1, activation="softmax")(label)
return Model(img, [validity, label])
def mask_randomly(self, imgs):
y1 = np.random.randint(0, self.img_rows - self.mask_height, imgs.shape[0])
y2 = y1 + self.mask_height
x1 = np.random.randint(0, self.img_rows - self.mask_width, imgs.shape[0])
x2 = x1 + self.mask_width
masked_imgs = np.empty_like(imgs)
for i, img in enumerate(imgs):
masked_img = img.copy()
_y1, _y2, _x1, _x2 = y1[i], y2[i], x1[i], x2[i],
masked_img[_y1:_y2, _x1:_x2, :] = 0
masked_imgs[i] = masked_img
return masked_imgs
def train(self, epochs, batch_size=128, sample_interval=50):
# Load the dataset
(X_train, y_train), (_, _) = mnist.load_data()
# Rescale MNIST to 32x32
X_train = np.array([scipy.misc.imresize(x, [self.img_rows, self.img_cols]) for x in X_train])
# Rescale -1 to 1
X_train = (X_train.astype(np.float32) - 127.5) / 127.5
X_train = np.expand_dims(X_train, axis=3)
y_train = y_train.reshape(-1, 1)
# Adversarial ground truths
valid = np.ones((batch_size, 4, 4, 1))
fake = np.zeros((batch_size, 4, 4, 1))
for epoch in range(epochs):
# ---------------------
# Train Discriminator
# ---------------------
# Sample half batch of images
idx = np.random.randint(0, X_train.shape[0], batch_size)
imgs = X_train[idx]
labels = y_train[idx]
masked_imgs = self.mask_randomly(imgs)
# Generate a half batch of new images
gen_imgs = self.generator.predict(masked_imgs)
# One-hot encoding of labels
labels = to_categorical(labels, num_classes=self.num_classes+1)
fake_labels = to_categorical(np.full((batch_size, 1), self.num_classes), num_classes=self.num_classes+1)
# Train the discriminator
d_loss_real = self.discriminator.train_on_batch(imgs, [valid, labels])
d_loss_fake = self.discriminator.train_on_batch(gen_imgs, [fake, fake_labels])
d_loss = 0.5 * np.add(d_loss_real, d_loss_fake)
# ---------------------
# Train Generator
# ---------------------
# Train the generator
g_loss = self.combined.train_on_batch(masked_imgs, valid)
# Plot the progress
print ("%d [D loss: %f, op_acc: %.2f%%] [G loss: %f]" % (epoch, d_loss[0], 100*d_loss[4], g_loss))
# If at save interval => save generated image samples
if epoch % sample_interval == 0:
# Select a random half batch of images
idx = np.random.randint(0, X_train.shape[0], 6)
imgs = X_train[idx]
self.sample_images(epoch, imgs)
self.save_model()
def sample_images(self, epoch, imgs):
r, c = 3, 6
masked_imgs = self.mask_randomly(imgs)
gen_imgs = self.generator.predict(masked_imgs)
imgs = (imgs + 1.0) * 0.5
masked_imgs = (masked_imgs + 1.0) * 0.5
gen_imgs = (gen_imgs + 1.0) * 0.5
gen_imgs = np.where(gen_imgs < 0, 0, gen_imgs)
fig, axs = plt.subplots(r, c)
for i in range(c):
axs[0,i].imshow(imgs[i, :, :, 0], cmap='gray')
axs[0,i].axis('off')
axs[1,i].imshow(masked_imgs[i, :, :, 0], cmap='gray')
axs[1,i].axis('off')
axs[2,i].imshow(gen_imgs[i, :, :, 0], cmap='gray')
axs[2,i].axis('off')
fig.savefig("images/%d.png" % epoch)
plt.close()
def save_model(self):
def save(model, model_name):
model_path = "saved_model/%s.json" % model_name
weights_path = "saved_model/%s_weights.hdf5" % model_name
options = {"file_arch": model_path,
"file_weight": weights_path}
json_string = model.to_json()
open(options['file_arch'], 'w').write(json_string)
model.save_weights(options['file_weight'])
save(self.generator, "ccgan_generator")
save(self.discriminator, "ccgan_discriminator")
if __name__ == '__main__':
ccgan = CCGAN()
ccgan.train(epochs=2000, batch_size=32, sample_interval=200)
3.6 Conditional Generative Adversarial Nets
代码及运行结果
cgan.py
from __future__ import print_function, division
from tensorflow.keras.datasets import mnist
from tensorflow.keras.layers import Input, Dense, Reshape, Flatten, Dropout, multiply
from tensorflow.keras.layers import BatchNormalization, Activation, Embedding, ZeroPadding2D
from tensorflow.keras.layers import LeakyReLU
from tensorflow.keras.layers import UpSampling2D, Conv2D
from tensorflow.keras.models import Sequential, Model
from tensorflow.keras.optimizers import Adam
import matplotlib.pyplot as plt
import numpy as np
class CGAN():
def __init__(self):
# Input shape
self.img_rows = 28
self.img_cols = 28
self.channels = 1
self.img_shape = (self.img_rows, self.img_cols, self.channels)
self.num_classes = 10
self.latent_dim = 100
optimizer = Adam(0.0002, 0.5)
# Build and compile the discriminator
self.discriminator = self.build_discriminator()
self.discriminator.compile(loss=['binary_crossentropy'],
optimizer=optimizer,
metrics=['accuracy'])
# Build the generator
self.generator = self.build_generator()
# The generator takes noise and the target label as input
# and generates the corresponding digit of that label
noise = Input(shape=(self.latent_dim,))
label = Input(shape=(1,))
img = self.generator([noise, label])
# For the combined model we will only train the generator
self.discriminator.trainable = False
# The discriminator takes generated image as input and determines validity
# and the label of that image
valid = self.discriminator([img, label])
# The combined model (stacked generator and discriminator)
# Trains generator to fool discriminator
self.combined = Model([noise, label], valid)
self.combined.compile(loss=['binary_crossentropy'],
optimizer=optimizer)
def build_generator(self):
model = Sequential()
model.add(Dense(256, input_dim=self.latent_dim))
model.add(LeakyReLU(alpha=0.2))
model.add(BatchNormalization(momentum=0.8))
model.add(Dense(512))
model.add(LeakyReLU(alpha=0.2))
model.add(BatchNormalization(momentum=0.8))
model.add(Dense(1024))
model.add(LeakyReLU(alpha=0.2))
model.add(BatchNormalization(momentum=0.8))
model.add(Dense(np.prod(self.img_shape), activation='tanh'))
model.add(Reshape(self.img_shape))
model.summary()
noise = Input(shape=(self.latent_dim,))
label = Input(shape=(1,), dtype='int32')
label_embedding = Flatten()(Embedding(self.num_classes, self.latent_dim)(label))
model_input = multiply([noise, label_embedding])
img = model(model_input)
return Model([noise, label], img)
def build_discriminator(self):
model = Sequential()
model.add(Dense(512, input_dim=np.prod(self.img_shape)))
model.add(LeakyReLU(alpha=0.2))
model.add(Dense(512))
model.add(LeakyReLU(alpha=0.2))
model.add(Dropout(0.4))
model.add(Dense(512))
model.add(LeakyReLU(alpha=0.2))
model.add(Dropout(0.4))
model.add(Dense(1, activation='sigmoid'))
model.summary()
img = Input(shape=self.img_shape)
label = Input(shape=(1,), dtype='int32')
label_embedding = Flatten()(Embedding(self.num_classes, np.prod(self.img_shape))(label))
flat_img = Flatten()(img)
model_input = multiply([flat_img, label_embedding])
validity = model(model_input)
return Model([img, label], validity)
def train(self, epochs, batch_size=128, sample_interval=50):
# Load the dataset
(X_train, y_train), (_, _) = mnist.load_data()
# Configure input
X_train = (X_train.astype(np.float32) - 127.5) / 127.5
X_train = np.expand_dims(X_train, axis=3)
y_train = y_train.reshape(-1, 1)
# Adversarial ground truths
valid = np.ones((batch_size, 1))
fake = np.zeros((batch_size, 1))
for epoch in range(epochs):
# ---------------------
# Train Discriminator
# ---------------------
# Select a random half batch of images
idx = np.random.randint(0, X_train.shape[0], batch_size)
imgs, labels = X_train[idx], y_train[idx]
# Sample noise as generator input
noise = np.random.normal(0, 1, (batch_size, 100))
# Generate a half batch of new images
gen_imgs = self.generator.predict([noise, labels])
# Train the discriminator
d_loss_real = self.discriminator.train_on_batch([imgs, labels], valid)
d_loss_fake = self.discriminator.train_on_batch([gen_imgs, labels], fake)
d_loss = 0.5 * np.add(d_loss_real, d_loss_fake)
# ---------------------
# Train Generator
# ---------------------
# Condition on labels
sampled_labels = np.random.randint(0, 10, batch_size).reshape(-1, 1)
# Train the generator
g_loss = self.combined.train_on_batch([noise, sampled_labels], valid)
# Plot the progress
print ("%d [D loss: %f, acc.: %.2f%%] [G loss: %f]" % (epoch, d_loss[0], 100*d_loss[1], g_loss))
# If at save interval => save generated image samples
if epoch % sample_interval == 0:
self.sample_images(epoch)
def sample_images(self, epoch):
r, c = 2, 5
noise = np.random.normal(0, 1, (r * c, 100))
sampled_labels = np.arange(0, 10).reshape(-1, 1)
gen_imgs = self.generator.predict([noise, sampled_labels])
# Rescale images 0 - 1
gen_imgs = 0.5 * gen_imgs + 0.5
fig, axs = plt.subplots(r, c)
cnt = 0
for i in range(r):
for j in range(c):
axs[i,j].imshow(gen_imgs[cnt,:,:,0], cmap='gray')
axs[i,j].set_title("Digit: %d" % sampled_labels[cnt])
axs[i,j].axis('off')
cnt += 1
fig.savefig("images/%d.png" % epoch)
plt.close()
if __name__ == '__main__':
cgan = CGAN()
cgan.train(epochs=1000, batch_size=32, sample_interval=200)
3.7 Coupled generative adversarial networks
代码及运行结果
cogan.py
from __future__ import print_function, division
import scipy
from tensorflow.keras.datasets import mnist
from tensorflow.keras.layers import Input, Dense, Reshape, Flatten, Dropout
from tensorflow.keras.layers import BatchNormalization, Activation, ZeroPadding2D
from tensorflow.keras.layers import LeakyReLU
from tensorflow.keras.layers import UpSampling2D, Conv2D
from tensorflow.keras.models import Sequential, Model
from tensorflow.keras.optimizers import Adam
import matplotlib.pyplot as plt
import sys
import numpy as np
class COGAN():
"""Reference: https://wiseodd.github.io/techblog/2017/02/18/coupled_gan/"""
def __init__(self):
self.img_rows = 28
self.img_cols = 28
self.channels = 1
self.img_shape = (self.img_rows, self.img_cols, self.channels)
self.latent_dim = 100
optimizer = Adam(0.0002, 0.5)
# Build and compile the discriminator
self.d1, self.d2 = self.build_discriminators()
self.d1.compile(loss='binary_crossentropy',
optimizer=optimizer,
metrics=['accuracy'])
self.d2.compile(loss='binary_crossentropy',
optimizer=optimizer,
metrics=['accuracy'])
# Build the generator
self.g1, self.g2 = self.build_generators()
# The generator takes noise as input and generated imgs
z = Input(shape=(self.latent_dim,))
img1 = self.g1(z)
img2 = self.g2(z)
# For the combined model we will only train the generators
self.d1.trainable = False
self.d2.trainable = False
# The valid takes generated images as input and determines validity
valid1 = self.d1(img1)
valid2 = self.d2(img2)
# The combined model (stacked generators and discriminators)
# Trains generators to fool discriminators
self.combined = Model(z, [valid1, valid2])
self.combined.compile(loss=['binary_crossentropy', 'binary_crossentropy'],
optimizer=optimizer)
def build_generators(self):
# Shared weights between generators
model = Sequential()
model.add(Dense(256, input_dim=self.latent_dim))
model.add(LeakyReLU(alpha=0.2))
model.add(BatchNormalization(momentum=0.8))
model.add(Dense(512))
model.add(LeakyReLU(alpha=0.2))
model.add(BatchNormalization(momentum=0.8))
noise = Input(shape=(self.latent_dim,))
feature_repr = model(noise)
# Generator 1
g1 = Dense(1024)(feature_repr)
g1 = LeakyReLU(alpha=0.2)(g1)
g1 = BatchNormalization(momentum=0.8)(g1)
g1 = Dense(np.prod(self.img_shape), activation='tanh')(g1)
img1 = Reshape(self.img_shape)(g1)
# Generator 2
g2 = Dense(1024)(feature_repr)
g2 = LeakyReLU(alpha=0.2)(g2)
g2 = BatchNormalization(momentum=0.8)(g2)
g2 = Dense(np.prod(self.img_shape), activation='tanh')(g2)
img2 = Reshape(self.img_shape)(g2)
model.summary()
return Model(noise, img1), Model(noise, img2)
def build_discriminators(self):
img1 = Input(shape=self.img_shape)
img2 = Input(shape=self.img_shape)
# Shared discriminator layers
model = Sequential()
model.add(Flatten(input_shape=self.img_shape))
model.add(Dense(512))
model.add(LeakyReLU(alpha=0.2))
model.add(Dense(256))
model.add(LeakyReLU(alpha=0.2))
img1_embedding = model(img1)
img2_embedding = model(img2)
# Discriminator 1
validity1 = Dense(1, activation='sigmoid')(img1_embedding)
# Discriminator 2
validity2 = Dense(1, activation='sigmoid')(img2_embedding)
return Model(img1, validity1), Model(img2, validity2)
def train(self, epochs, batch_size=128, sample_interval=50):
# Load the dataset
(X_train, _), (_, _) = mnist.load_data()
# Rescale -1 to 1
X_train = (X_train.astype(np.float32) - 127.5) / 127.5
X_train = np.expand_dims(X_train, axis=3)
# Images in domain A and B (rotated)
X1 = X_train[:int(X_train.shape[0]/2)]
X2 = X_train[int(X_train.shape[0]/2):]
X2 = scipy.ndimage.interpolation.rotate(X2, 90, axes=(1, 2))
# Adversarial ground truths
valid = np.ones((batch_size, 1))
fake = np.zeros((batch_size, 1))
for epoch in range(epochs):
# ----------------------
# Train Discriminators
# ----------------------
# Select a random batch of images
idx = np.random.randint(0, X1.shape[0], batch_size)
imgs1 = X1[idx]
imgs2 = X2[idx]
# Sample noise as generator input
noise = np.random.normal(0, 1, (batch_size, 100))
# Generate a batch of new images
gen_imgs1 = self.g1.predict(noise)
gen_imgs2 = self.g2.predict(noise)
# Train the discriminators
d1_loss_real = self.d1.train_on_batch(imgs1, valid)
d2_loss_real = self.d2.train_on_batch(imgs2, valid)
d1_loss_fake = self.d1.train_on_batch(gen_imgs1, fake)
d2_loss_fake = self.d2.train_on_batch(gen_imgs2, fake)
d1_loss = 0.5 * np.add(d1_loss_real, d1_loss_fake)
d2_loss = 0.5 * np.add(d2_loss_real, d2_loss_fake)
# ------------------
# Train Generators
# ------------------
g_loss = self.combined.train_on_batch(noise, [valid, valid])
# Plot the progress
print ("%d [D1 loss: %f, acc.: %.2f%%] [D2 loss: %f, acc.: %.2f%%] [G loss: %f]" \
% (epoch, d1_loss[0], 100*d1_loss[1], d2_loss[0], 100*d2_loss[1], g_loss[0]))
# If at save interval => save generated image samples
if epoch % sample_interval == 0:
self.sample_images(epoch)
def sample_images(self, epoch):
r, c = 4, 4
noise = np.random.normal(0, 1, (r * int(c/2), 100))
gen_imgs1 = self.g1.predict(noise)
gen_imgs2 = self.g2.predict(noise)
gen_imgs = np.concatenate([gen_imgs1, gen_imgs2])
# Rescale images 0 - 1
gen_imgs = 0.5 * gen_imgs + 0.5
fig, axs = plt.subplots(r, c)
cnt = 0
for i in range(r):
for j in range(c):
axs[i,j].imshow(gen_imgs[cnt, :,:,0], cmap='gray')
axs[i,j].axis('off')
cnt += 1
fig.savefig("images/mnist_%d.png" % epoch)
plt.close()
if __name__ == '__main__':
gan = COGAN()
gan.train(epochs=1000, batch_size=32, sample_interval=200)
3.8 Context Encoders: Feature Learning by Inpainting
代码及运行结果
cifar-10-python.tar.gz下载
context_encoder.py
from __future__ import print_function, division
import os
from tensorflow.python.keras.datasets.cifar import load_batch
from tensorflow.keras.datasets import cifar10
from tensorflow.keras.layers import Input, Dense, Reshape, Flatten, Dropout, multiply, GaussianNoise
from tensorflow.keras.layers import BatchNormalization, Activation, Embedding, ZeroPadding2D
from tensorflow.keras.layers import MaxPooling2D
from tensorflow.keras.layers import LeakyReLU
from tensorflow.keras.layers import UpSampling2D, Conv2D
from tensorflow.keras.models import Sequential, Model
from tensorflow.keras.optimizers import Adam
from tensorflow.keras import losses
from tensorflow.keras.utils import to_categorical
import tensorflow.keras.backend as K
import matplotlib.pyplot as plt
import numpy as np
class ContextEncoder():
def __init__(self):
self.img_rows = 32
self.img_cols = 32
self.mask_height = 8
self.mask_width = 8
self.channels = 3
self.num_classes = 2
self.img_shape = (self.img_rows, self.img_cols, self.channels)
self.missing_shape = (self.mask_height, self.mask_width, self.channels)
optimizer = Adam(0.0002, 0.5)
# Build and compile the discriminator
self.discriminator = self.build_discriminator()
self.discriminator.compile(loss='binary_crossentropy',
optimizer=optimizer,
metrics=['accuracy'])
# Build the generator
self.generator = self.build_generator()
# The generator takes noise as input and generates the missing
# part of the image
masked_img = Input(shape=self.img_shape)
gen_missing = self.generator(masked_img)
# For the combined model we will only train the generator
self.discriminator.trainable = False
# The discriminator takes generated images as input and determines
# if it is generated or if it is a real image
valid = self.discriminator(gen_missing)
# The combined model (stacked generator and discriminator)
# Trains generator to fool discriminator
self.combined = Model(masked_img , [gen_missing, valid])
self.combined.compile(loss=['mse', 'binary_crossentropy'],
loss_weights=[0.999, 0.001],
optimizer=optimizer)
def build_generator(self):
model = Sequential()
# Encoder
model.add(Conv2D(32, kernel_size=3, strides=2, input_shape=self.img_shape, padding="same"))
model.add(LeakyReLU(alpha=0.2))
model.add(BatchNormalization(momentum=0.8))
model.add(Conv2D(64, kernel_size=3, strides=2, padding="same"))
model.add(LeakyReLU(alpha=0.2))
model.add(BatchNormalization(momentum=0.8))
model.add(Conv2D(128, kernel_size=3, strides=2, padding="same"))
model.add(LeakyReLU(alpha=0.2))
model.add(BatchNormalization(momentum=0.8))
model.add(Conv2D(512, kernel_size=1, strides=2, padding="same"))
model.add(LeakyReLU(alpha=0.2))
model.add(Dropout(0.5))
# Decoder
model.add(UpSampling2D())
model.add(Conv2D(128, kernel_size=3, padding="same"))
model.add(Activation('relu'))
model.add(BatchNormalization(momentum=0.8))
model.add(UpSampling2D())
model.add(Conv2D(64, kernel_size=3, padding="same"))
model.add(Activation('relu'))
model.add(BatchNormalization(momentum=0.8))
model.add(Conv2D(self.channels, kernel_size=3, padding="same"))
model.add(Activation('tanh'))
model.summary()
masked_img = Input(shape=self.img_shape)
gen_missing = model(masked_img)
return Model(masked_img, gen_missing)
def build_discriminator(self):
model = Sequential()
model.add(Conv2D(64, kernel_size=3, strides=2, input_shape=self.missing_shape, padding="same"))
model.add(LeakyReLU(alpha=0.2))
model.add(BatchNormalization(momentum=0.8))
model.add(Conv2D(128, kernel_size=3, strides=2, padding="same"))
model.add(LeakyReLU(alpha=0.2))
model.add(BatchNormalization(momentum=0.8))
model.add(Conv2D(256, kernel_size=3, padding="same"))
model.add(LeakyReLU(alpha=0.2))
model.add(BatchNormalization(momentum=0.8))
model.add(Flatten())
model.add(Dense(1, activation='sigmoid'))
model.summary()
img = Input(shape=self.missing_shape)
validity = model(img)
return Model(img, validity)
def mask_randomly(self, imgs):
y1 = np.random.randint(0, self.img_rows - self.mask_height, imgs.shape[0])
y2 = y1 + self.mask_height
x1 = np.random.randint(0, self.img_rows - self.mask_width, imgs.shape[0])
x2 = x1 + self.mask_width
masked_imgs = np.empty_like(imgs)
missing_parts = np.empty((imgs.shape[0], self.mask_height, self.mask_width, self.channels))
for i, img in enumerate(imgs):
masked_img = img.copy()
_y1, _y2, _x1, _x2 = y1[i], y2[i], x1[i], x2[i]
missing_parts[i] = masked_img[_y1:_y2, _x1:_x2, :].copy()
masked_img[_y1:_y2, _x1:_x2, :] = 0
masked_imgs[i] = masked_img
return masked_imgs, missing_parts, (y1, y2, x1, x2)
def train(self, epochs, batch_size=128, sample_interval=50):
# Load the dataset
num_train_samples = 50000
path = 'cifar-10-batches-py'
x_train = np.empty((num_train_samples, 3, 32, 32), dtype='uint8')
y_train = np.empty((num_train_samples,), dtype='uint8')
for i in range(1, 6):
fpath = os.path.join(path, 'data_batch_' + str(i))
(x_train[(i - 1) * 10000:i * 10000, :, :, :],
y_train[(i - 1) * 10000:i * 10000]) = load_batch(fpath)
fpath = os.path.join(path, 'test_batch')
x_test, y_test = load_batch(fpath)
y_train = np.reshape(y_train, (len(y_train), 1))
y_test = np.reshape(y_test, (len(y_test), 1))
if K.image_data_format() == 'channels_last':
x_train = x_train.transpose(0, 2, 3, 1)
x_test = x_test.transpose(0, 2, 3, 1)
x_test = x_test.astype(x_train.dtype)
y_test = y_test.astype(y_train.dtype)
# (X_train, y_train), (_, _) = cifar10.load_data()
X_train,Y_train = x_train,y_train
# Extract dogs and cats
X_cats = X_train[(y_train == 3).flatten()]
X_dogs = X_train[(y_train == 5).flatten()]
X_train = np.vstack((X_cats, X_dogs))
# Rescale -1 to 1
X_train = X_train / 127.5 - 1.
y_train = y_train.reshape(-1, 1)
# Adversarial ground truths
valid = np.ones((batch_size, 1))
fake = np.zeros((batch_size, 1))
for epoch in range(epochs):
# ---------------------
# Train Discriminator
# ---------------------
# Select a random batch of images
idx = np.random.randint(0, X_train.shape[0], batch_size)
imgs = X_train[idx]
masked_imgs, missing_parts, _ = self.mask_randomly(imgs)
# Generate a batch of new images
gen_missing = self.generator.predict(masked_imgs)
# Train the discriminator
d_loss_real = self.discriminator.train_on_batch(missing_parts, valid)
d_loss_fake = self.discriminator.train_on_batch(gen_missing, fake)
d_loss = 0.5 * np.add(d_loss_real, d_loss_fake)
# ---------------------
# Train Generator
# ---------------------
g_loss = self.combined.train_on_batch(masked_imgs, [missing_parts, valid])
# Plot the progress
print ("%d [D loss: %f, acc: %.2f%%] [G loss: %f, mse: %f]" % (epoch, d_loss[0], 100*d_loss[1], g_loss[0], g_loss[1]))
# If at save interval => save generated image samples
if epoch % sample_interval == 0:
idx = np.random.randint(0, X_train.shape[0], 6)
imgs = X_train[idx]
self.sample_images(epoch, imgs)
def sample_images(self, epoch, imgs):
r, c = 3, 6
masked_imgs, missing_parts, (y1, y2, x1, x2) = self.mask_randomly(imgs)
gen_missing = self.generator.predict(masked_imgs)
imgs = 0.5 * imgs + 0.5
masked_imgs = 0.5 * masked_imgs + 0.5
gen_missing = 0.5 * gen_missing + 0.5
fig, axs = plt.subplots(r, c)
for i in range(c):
axs[0,i].imshow(imgs[i, :,:])
axs[0,i].axis('off')
axs[1,i].imshow(masked_imgs[i, :,:])
axs[1,i].axis('off')
filled_in = imgs[i].copy()
filled_in[y1[i]:y2[i], x1[i]:x2[i], :] = gen_missing[i]
axs[2,i].imshow(filled_in)
axs[2,i].axis('off')
fig.savefig("images/%d.png" % epoch)
plt.close()
def save_model(self):
def save(model, model_name):
model_path = "saved_model/%s.json" % model_name
weights_path = "saved_model/%s_weights.hdf5" % model_name
options = {"file_arch": model_path,
"file_weight": weights_path}
json_string = model.to_json()
open(options['file_arch'], 'w').write(json_string)
model.save_weights(options['file_weight'])
save(self.generator, "generator")
save(self.discriminator, "discriminator")
if __name__ == '__main__':
context_encoder = ContextEncoder()
context_encoder.train(epochs=3000, batch_size=64, sample_interval=50)
3.9 Unpaired Image-to-Image Translation using Cycle-Consistent Adversarial Networks
代码及运行结果
data_loader.py
import scipy
from glob import glob
import numpy as np
class DataLoader():
def __init__(self, dataset_name, img_res=(128, 128)):
self.dataset_name = dataset_name
self.img_res = img_res
def load_data(self, domain, batch_size=1, is_testing=False):
data_type = "train%s" % domain if not is_testing else "test%s" % domain
path = glob('./datasets/%s/%s/*' % (self.dataset_name, data_type))
batch_images = np.random.choice(path, size=batch_size)
imgs = []
for img_path in batch_images:
img = self.imread(img_path)
if not is_testing:
img = scipy.misc.imresize(img, self.img_res)
if np.random.random() > 0.5:
img = np.fliplr(img)
else:
img = scipy.misc.imresize(img, self.img_res)
imgs.append(img)
imgs = np.array(imgs)/127.5 - 1.
return imgs
def load_batch(self, batch_size=1, is_testing=False):
data_type = "train" if not is_testing else "val"
path_A = glob('./datasets/%s/%sA/*' % (self.dataset_name, data_type))
path_B = glob('./datasets/%s/%sB/*' % (self.dataset_name, data_type))
self.n_batches = int(min(len(path_A), len(path_B)) / batch_size)
total_samples = self.n_batches * batch_size
# Sample n_batches * batch_size from each path list so that model sees all
# samples from both domains
path_A = np.random.choice(path_A, total_samples, replace=False)
path_B = np.random.choice(path_B, total_samples, replace=False)
for i in range(self.n_batches-1):
batch_A = path_A[i*batch_size:(i+1)*batch_size]
batch_B = path_B[i*batch_size:(i+1)*batch_size]
imgs_A, imgs_B = [], []
for img_A, img_B in zip(batch_A, batch_B):
img_A = self.imread(img_A)
img_B = self.imread(img_B)
img_A = scipy.misc.imresize(img_A, self.img_res)
img_B = scipy.misc.imresize(img_B, self.img_res)
if not is_testing and np.random.random() > 0.5:
img_A = np.fliplr(img_A)
img_B = np.fliplr(img_B)
imgs_A.append(img_A)
imgs_B.append(img_B)
imgs_A = np.array(imgs_A)/127.5 - 1.
imgs_B = np.array(imgs_B)/127.5 - 1.
yield imgs_A, imgs_B
def load_img(self, path):
img = self.imread(path)
img = scipy.misc.imresize(img, self.img_res)
img = img/127.5 - 1.
return img[np.newaxis, :, :, :]
def imread(self, path):
return scipy.misc.imread(path, mode='RGB').astype(np.float)
instancenormalization.py
from tensorflow.keras.layers import Layer, InputSpec
from tensorflow.keras import initializers, regularizers, constraints
from tensorflow.keras import backend as K
class InstanceNormalization(Layer):
"""Instance normalization layer.
Normalize the activations of the previous layer at each step,
i.e. applies a transformation that maintains the mean activation
close to 0 and the activation standard deviation close to 1.
# Arguments
axis: Integer, the axis that should be normalized
(typically the features axis).
For instance, after a `Conv2D` layer with
`data_format="channels_first"`,
set `axis=1` in `InstanceNormalization`.
Setting `axis=None` will normalize all values in each
instance of the batch.
Axis 0 is the batch dimension. `axis` cannot be set to 0 to avoid errors.
epsilon: Small float added to variance to avoid dividing by zero.
center: If True, add offset of `beta` to normalized tensor.
If False, `beta` is ignored.
scale: If True, multiply by `gamma`.
If False, `gamma` is not used.
When the next layer is linear (also e.g. `nn.relu`),
this can be disabled since the scaling
will be done by the next layer.
beta_initializer: Initializer for the beta weight.
gamma_initializer: Initializer for the gamma weight.
beta_regularizer: Optional regularizer for the beta weight.
gamma_regularizer: Optional regularizer for the gamma weight.
beta_constraint: Optional constraint for the beta weight.
gamma_constraint: Optional constraint for the gamma weight.
# Input shape
Arbitrary. Use the keyword argument `input_shape`
(tuple of integers, does not include the samples axis)
when using this layer as the first layer in a Sequential model.
# Output shape
Same shape as input.
# References
- [Layer Normalization](https://arxiv.org/abs/1607.06450)
- [Instance Normalization: The Missing Ingredient for Fast Stylization](
https://arxiv.org/abs/1607.08022)
"""
def __init__(self,
axis=None,
epsilon=1e-3,
center=True,
scale=True,
beta_initializer='zeros',
gamma_initializer='ones',
beta_regularizer=None,
gamma_regularizer=None,
beta_constraint=None,
gamma_constraint=None,
**kwargs):
super(InstanceNormalization, self).__init__(**kwargs)
self.supports_masking = True
self.axis = axis
self.epsilon = epsilon
self.center = center
self.scale = scale
self.beta_initializer = initializers.get(beta_initializer)
self.gamma_initializer = initializers.get(gamma_initializer)
self.beta_regularizer = regularizers.get(beta_regularizer)
self.gamma_regularizer = regularizers.get(gamma_regularizer)
self.beta_constraint = constraints.get(beta_constraint)
self.gamma_constraint = constraints.get(gamma_constraint)
def build(self, input_shape):
ndim = len(input_shape)
if self.axis == 0:
raise ValueError('Axis cannot be zero')
if (self.axis is not None) and (ndim == 2):
raise ValueError('Cannot specify axis for rank 1 tensor')
self.input_spec = InputSpec(ndim=ndim)
if self.axis is None:
shape = (1,)
else:
shape = (input_shape[self.axis],)
if self.scale:
self.gamma = self.add_weight(shape=shape,
name='gamma',
initializer=self.gamma_initializer,
regularizer=self.gamma_regularizer,
constraint=self.gamma_constraint)
else:
self.gamma = None
if self.center:
self.beta = self.add_weight(shape=shape,
name='beta',
initializer=self.beta_initializer,
regularizer=self.beta_regularizer,
constraint=self.beta_constraint)
else:
self.beta = None
self.built = True
def call(self, inputs, training=None):
input_shape = K.int_shape(inputs)
reduction_axes = list(range(0, len(input_shape)))
if self.axis is not None:
del reduction_axes[self.axis]
del reduction_axes[0]
mean = K.mean(inputs, reduction_axes, keepdims=True)
stddev = K.std(inputs, reduction_axes, keepdims=True) + self.epsilon
normed = (inputs - mean) / stddev
broadcast_shape = [1] * len(input_shape)
if self.axis is not None:
broadcast_shape[self.axis] = input_shape[self.axis]
if self.scale:
broadcast_gamma = K.reshape(self.gamma, broadcast_shape)
normed = normed * broadcast_gamma
if self.center:
broadcast_beta = K.reshape(self.beta, broadcast_shape)
normed = normed + broadcast_beta
return normed
def get_config(self):
config = {
'axis': self.axis,
'epsilon': self.epsilon,
'center': self.center,
'scale': self.scale,
'beta_initializer': initializers.serialize(self.beta_initializer),
'gamma_initializer': initializers.serialize(self.gamma_initializer),
'beta_regularizer': regularizers.serialize(self.beta_regularizer),
'gamma_regularizer': regularizers.serialize(self.gamma_regularizer),
'beta_constraint': constraints.serialize(self.beta_constraint),
'gamma_constraint': constraints.serialize(self.gamma_constraint)
}
base_config = super(InstanceNormalization, self).get_config()
return dict(list(base_config.items()) + list(config.items()))
cyclegan.py
from __future__ import print_function, division
import scipy
from tensorflow.keras.datasets import mnist
from instancenormalization import InstanceNormalization
from tensorflow.keras.layers import Input, Dense, Reshape, Flatten, Dropout, Concatenate
from tensorflow.keras.layers import BatchNormalization, Activation, ZeroPadding2D
from tensorflow.keras.layers import LeakyReLU
from tensorflow.keras.layers import UpSampling2D, Conv2D
from tensorflow.keras.models import Sequential, Model
from tensorflow.keras.optimizers import Adam
import datetime
import matplotlib.pyplot as plt
import sys
from data_loader import DataLoader
import numpy as np
import os
class CycleGAN():
def __init__(self):
# Input shape
self.img_rows = 128
self.img_cols = 128
self.channels = 3
self.img_shape = (self.img_rows, self.img_cols, self.channels)
# Configure data loader
self.dataset_name = 'apple2orange'
self.data_loader = DataLoader(dataset_name=self.dataset_name,
img_res=(self.img_rows, self.img_cols))
# Calculate output shape of D (PatchGAN)
patch = int(self.img_rows / 2**4)
self.disc_patch = (patch, patch, 1)
# Number of filters in the first layer of G and D
self.gf = 32
self.df = 64
# Loss weights
self.lambda_cycle = 10.0 # Cycle-consistency loss
self.lambda_id = 0.1 * self.lambda_cycle # Identity loss
optimizer = Adam(0.0002, 0.5)
# Build and compile the discriminators
self.d_A = self.build_discriminator()
self.d_B = self.build_discriminator()
self.d_A.compile(loss='mse',
optimizer=optimizer,
metrics=['accuracy'])
self.d_B.compile(loss='mse',
optimizer=optimizer,
metrics=['accuracy'])
#-------------------------
# Construct Computational
# Graph of Generators
#-------------------------
# Build the generators
self.g_AB = self.build_generator()
self.g_BA = self.build_generator()
# Input images from both domains
img_A = Input(shape=self.img_shape)
img_B = Input(shape=self.img_shape)
# Translate images to the other domain
fake_B = self.g_AB(img_A)
fake_A = self.g_BA(img_B)
# Translate images back to original domain
reconstr_A = self.g_BA(fake_B)
reconstr_B = self.g_AB(fake_A)
# Identity mapping of images
img_A_id = self.g_BA(img_A)
img_B_id = self.g_AB(img_B)
# For the combined model we will only train the generators
self.d_A.trainable = False
self.d_B.trainable = False
# Discriminators determines validity of translated images
valid_A = self.d_A(fake_A)
valid_B = self.d_B(fake_B)
# Combined model trains generators to fool discriminators
self.combined = Model(inputs=[img_A, img_B],
outputs=[ valid_A, valid_B,
reconstr_A, reconstr_B,
img_A_id, img_B_id ])
self.combined.compile(loss=['mse', 'mse',
'mae', 'mae',
'mae', 'mae'],
loss_weights=[ 1, 1,
self.lambda_cycle, self.lambda_cycle,
self.lambda_id, self.lambda_id ],
optimizer=optimizer)
def build_generator(self):
"""U-Net Generator"""
def conv2d(layer_input, filters, f_size=4):
"""Layers used during downsampling"""
d = Conv2D(filters, kernel_size=f_size, strides=2, padding='same')(layer_input)
d = LeakyReLU(alpha=0.2)(d)
d = InstanceNormalization()(d)
return d
def deconv2d(layer_input, skip_input, filters, f_size=4, dropout_rate=0):
"""Layers used during upsampling"""
u = UpSampling2D(size=2)(layer_input)
u = Conv2D(filters, kernel_size=f_size, strides=1, padding='same', activation='relu')(u)
if dropout_rate:
u = Dropout(dropout_rate)(u)
u = InstanceNormalization()(u)
u = Concatenate()([u, skip_input])
return u
# Image input
d0 = Input(shape=self.img_shape)
# Downsampling
d1 = conv2d(d0, self.gf)
d2 = conv2d(d1, self.gf*2)
d3 = conv2d(d2, self.gf*4)
d4 = conv2d(d3, self.gf*8)
# Upsampling
u1 = deconv2d(d4, d3, self.gf*4)
u2 = deconv2d(u1, d2, self.gf*2)
u3 = deconv2d(u2, d1, self.gf)
u4 = UpSampling2D(size=2)(u3)
output_img = Conv2D(self.channels, kernel_size=4, strides=1, padding='same', activation='tanh')(u4)
return Model(d0, output_img)
def build_discriminator(self):
def d_layer(layer_input, filters, f_size=4, normalization=True):
"""Discriminator layer"""
d = Conv2D(filters, kernel_size=f_size, strides=2, padding='same')(layer_input)
d = LeakyReLU(alpha=0.2)(d)
if normalization:
d = InstanceNormalization()(d)
return d
img = Input(shape=self.img_shape)
d1 = d_layer(img, self.df, normalization=False)
d2 = d_layer(d1, self.df*2)
d3 = d_layer(d2, self.df*4)
d4 = d_layer(d3, self.df*8)
validity = Conv2D(1, kernel_size=4, strides=1, padding='same')(d4)
return Model(img, validity)
def train(self, epochs, batch_size=1, sample_interval=50):
start_time = datetime.datetime.now()
# Adversarial loss ground truths
valid = np.ones((batch_size,) + self.disc_patch)
fake = np.zeros((batch_size,) + self.disc_patch)
for epoch in range(epochs):
for batch_i, (imgs_A, imgs_B) in enumerate(self.data_loader.load_batch(batch_size)):
# ----------------------
# Train Discriminators
# ----------------------
# Translate images to opposite domain
fake_B = self.g_AB.predict(imgs_A)
fake_A = self.g_BA.predict(imgs_B)
# Train the discriminators (original images = real / translated = Fake)
dA_loss_real = self.d_A.train_on_batch(imgs_A, valid)
dA_loss_fake = self.d_A.train_on_batch(fake_A, fake)
dA_loss = 0.5 * np.add(dA_loss_real, dA_loss_fake)
dB_loss_real = self.d_B.train_on_batch(imgs_B, valid)
dB_loss_fake = self.d_B.train_on_batch(fake_B, fake)
dB_loss = 0.5 * np.add(dB_loss_real, dB_loss_fake)
# Total disciminator loss
d_loss = 0.5 * np.add(dA_loss, dB_loss)
# ------------------
# Train Generators
# ------------------
# Train the generators
g_loss = self.combined.train_on_batch([imgs_A, imgs_B],
[valid, valid,
imgs_A, imgs_B,
imgs_A, imgs_B])
elapsed_time = datetime.datetime.now() - start_time
# Plot the progress
print ("[Epoch %d/%d] [Batch %d/%d] [D loss: %f, acc: %3d%%] [G loss: %05f, adv: %05f, recon: %05f, id: %05f] time: %s " \
% ( epoch, epochs,
batch_i, self.data_loader.n_batches,
d_loss[0], 100*d_loss[1],
g_loss[0],
np.mean(g_loss[1:3]),
np.mean(g_loss[3:5]),
np.mean(g_loss[5:6]),
elapsed_time))
# If at save interval => save generated image samples
if batch_i % sample_interval == 0:
self.sample_images(epoch, batch_i)
def sample_images(self, epoch, batch_i):
os.makedirs('images/%s' % self.dataset_name, exist_ok=True)
r, c = 2, 3
imgs_A = self.data_loader.load_data(domain="A", batch_size=1, is_testing=True)
imgs_B = self.data_loader.load_data(domain="B", batch_size=1, is_testing=True)
# Demo (for GIF)
#imgs_A = self.data_loader.load_img('datasets/apple2orange/testA/n07740461_1541.jpg')
#imgs_B = self.data_loader.load_img('datasets/apple2orange/testB/n07749192_4241.jpg')
# Translate images to the other domain
fake_B = self.g_AB.predict(imgs_A)
fake_A = self.g_BA.predict(imgs_B)
# Translate back to original domain
reconstr_A = self.g_BA.predict(fake_B)
reconstr_B = self.g_AB.predict(fake_A)
gen_imgs = np.concatenate([imgs_A, fake_B, reconstr_A, imgs_B, fake_A, reconstr_B])
# Rescale images 0 - 1
gen_imgs = 0.5 * gen_imgs + 0.5
titles = ['Original', 'Translated', 'Reconstructed']
fig, axs = plt.subplots(r, c)
cnt = 0
for i in range(r):
for j in range(c):
axs[i,j].imshow(gen_imgs[cnt])
axs[i, j].set_title(titles[j])
axs[i,j].axis('off')
cnt += 1
fig.savefig("images/%s/%d_%d.png" % (self.dataset_name, epoch, batch_i))
plt.close()
if __name__ == '__main__':
gan = CycleGAN()
gan.train(epochs=10, batch_size=1, sample_interval=200)
3.10 Deep Convolutional Generative Adversarial Network
代码及运行结果
dcgan.py
from __future__ import print_function, division
from tensorflow.keras.datasets import mnist
from tensorflow.keras.layers import Input, Dense, Reshape, Flatten, Dropout
from tensorflow.keras.layers import BatchNormalization, Activation, ZeroPadding2D
from tensorflow.keras.layers import LeakyReLU
from tensorflow.keras.layers import UpSampling2D, Conv2D
from tensorflow.keras.models import Sequential, Model
from tensorflow.keras.optimizers import Adam
import matplotlib.pyplot as plt
import sys
import numpy as np
class DCGAN():
def __init__(self):
# Input shape
self.img_rows = 28
self.img_cols = 28
self.channels = 1
self.img_shape = (self.img_rows, self.img_cols, self.channels)
self.latent_dim = 100
optimizer = Adam(0.0002, 0.5)
# Build and compile the discriminator
self.discriminator = self.build_discriminator()
self.discriminator.compile(loss='binary_crossentropy',
optimizer=optimizer,
metrics=['accuracy'])
# Build the generator
self.generator = self.build_generator()
# The generator takes noise as input and generates imgs
z = Input(shape=(self.latent_dim,))
img = self.generator(z)
# For the combined model we will only train the generator
self.discriminator.trainable = False
# The discriminator takes generated images as input and determines validity
valid = self.discriminator(img)
# The combined model (stacked generator and discriminator)
# Trains the generator to fool the discriminator
self.combined = Model(z, valid)
self.combined.compile(loss='binary_crossentropy', optimizer=optimizer)
def build_generator(self):
model = Sequential()
model.add(Dense(128 * 7 * 7, activation="relu", input_dim=self.latent_dim))
model.add(Reshape((7, 7, 128)))
model.add(UpSampling2D())
model.add(Conv2D(128, kernel_size=3, padding="same"))
model.add(BatchNormalization(momentum=0.8))
model.add(Activation("relu"))
model.add(UpSampling2D())
model.add(Conv2D(64, kernel_size=3, padding="same"))
model.add(BatchNormalization(momentum=0.8))
model.add(Activation("relu"))
model.add(Conv2D(self.channels, kernel_size=3, padding="same"))
model.add(Activation("tanh"))
model.summary()
noise = Input(shape=(self.latent_dim,))
img = model(noise)
return Model(noise, img)
def build_discriminator(self):
model = Sequential()
model.add(Conv2D(32, kernel_size=3, strides=2, input_shape=self.img_shape, padding="same"))
model.add(LeakyReLU(alpha=0.2))
model.add(Dropout(0.25))
model.add(Conv2D(64, kernel_size=3, strides=2, padding="same"))
model.add(ZeroPadding2D(padding=((0,1),(0,1))))
model.add(BatchNormalization(momentum=0.8))
model.add(LeakyReLU(alpha=0.2))
model.add(Dropout(0.25))
model.add(Conv2D(128, kernel_size=3, strides=2, padding="same"))
model.add(BatchNormalization(momentum=0.8))
model.add(LeakyReLU(alpha=0.2))
model.add(Dropout(0.25))
model.add(Conv2D(256, kernel_size=3, strides=1, padding="same"))
model.add(BatchNormalization(momentum=0.8))
model.add(LeakyReLU(alpha=0.2))
model.add(Dropout(0.25))
model.add(Flatten())
model.add(Dense(1, activation='sigmoid'))
model.summary()
img = Input(shape=self.img_shape)
validity = model(img)
return Model(img, validity)
def train(self, epochs, batch_size=128, save_interval=50):
# Load the dataset
(X_train, _), (_, _) = mnist.load_data()
# Rescale -1 to 1
X_train = X_train / 127.5 - 1.
X_train = np.expand_dims(X_train, axis=3)
# Adversarial ground truths
valid = np.ones((batch_size, 1))
fake = np.zeros((batch_size, 1))
for epoch in range(epochs):
# ---------------------
# Train Discriminator
# ---------------------
# Select a random half of images
idx = np.random.randint(0, X_train.shape[0], batch_size)
imgs = X_train[idx]
# Sample noise and generate a batch of new images
noise = np.random.normal(0, 1, (batch_size, self.latent_dim))
gen_imgs = self.generator.predict(noise)
# Train the discriminator (real classified as ones and generated as zeros)
d_loss_real = self.discriminator.train_on_batch(imgs, valid)
d_loss_fake = self.discriminator.train_on_batch(gen_imgs, fake)
d_loss = 0.5 * np.add(d_loss_real, d_loss_fake)
# ---------------------
# Train Generator
# ---------------------
# Train the generator (wants discriminator to mistake images as real)
g_loss = self.combined.train_on_batch(noise, valid)
# Plot the progress
print ("%d [D loss: %f, acc.: %.2f%%] [G loss: %f]" % (epoch, d_loss[0], 100*d_loss[1], g_loss))
# If at save interval => save generated image samples
if epoch % save_interval == 0:
self.save_imgs(epoch)
def save_imgs(self, epoch):
r, c = 5, 5
noise = np.random.normal(0, 1, (r * c, self.latent_dim))
gen_imgs = self.generator.predict(noise)
# Rescale images 0 - 1
gen_imgs = 0.5 * gen_imgs + 0.5
fig, axs = plt.subplots(r, c)
cnt = 0
for i in range(r):
for j in range(c):
axs[i,j].imshow(gen_imgs[cnt, :,:,0], cmap='gray')
axs[i,j].axis('off')
cnt += 1
fig.savefig("images/mnist_%d.png" % epoch)
plt.close()
if __name__ == '__main__':
dcgan = DCGAN()
dcgan.train(epochs=4000, batch_size=32, save_interval=50)
3.11 Discover Cross-Domain Relations with Generative Adversarial Networks
代码及运行结果
edges2shoes.tar.gz下载
discogan.py
from __future__ import print_function, division
import scipy
from tensorflow.keras.datasets import mnist
from instancenormalization import InstanceNormalization
from tensorflow.keras.layers import Input, Dense, Reshape, Flatten, Dropout, Concatenate
from tensorflow.keras.layers import BatchNormalization, Activation, ZeroPadding2D
from tensorflow.keras.layers import LeakyReLU
from tensorflow.keras.layers import UpSampling2D, Conv2D
from tensorflow.keras.models import Sequential, Model
from tensorflow.keras.optimizers import Adam
import datetime
import matplotlib.pyplot as plt
import sys
from data_loader import DataLoader
import numpy as np
import os
class DiscoGAN():
def __init__(self):
# Input shape
self.img_rows = 128
self.img_cols = 128
self.channels = 3
self.img_shape = (self.img_rows, self.img_cols, self.channels)
# Configure data loader
self.dataset_name = 'edges2shoes'
self.data_loader = DataLoader(dataset_name=self.dataset_name,
img_res=(self.img_rows, self.img_cols))
# Calculate output shape of D (PatchGAN)
patch = int(self.img_rows / 2**4)
self.disc_patch = (patch, patch, 1)
# Number of filters in the first layer of G and D
self.gf = 64
self.df = 64
optimizer = Adam(0.0002, 0.5)
# Build and compile the discriminators
self.d_A = self.build_discriminator()
self.d_B = self.build_discriminator()
self.d_A.compile(loss='mse',
optimizer=optimizer,
metrics=['accuracy'])
self.d_B.compile(loss='mse',
optimizer=optimizer,
metrics=['accuracy'])
#-------------------------
# Construct Computational
# Graph of Generators
#-------------------------
# Build the generators
self.g_AB = self.build_generator()
self.g_BA = self.build_generator()
# Input images from both domains
img_A = Input(shape=self.img_shape)
img_B = Input(shape=self.img_shape)
# Translate images to the other domain
fake_B = self.g_AB(img_A)
fake_A = self.g_BA(img_B)
# Translate images back to original domain
reconstr_A = self.g_BA(fake_B)
reconstr_B = self.g_AB(fake_A)
# For the combined model we will only train the generators
self.d_A.trainable = False
self.d_B.trainable = False
# Discriminators determines validity of translated images
valid_A = self.d_A(fake_A)
valid_B = self.d_B(fake_B)
# Objectives
# + Adversarial: Fool domain discriminators
# + Translation: Minimize MAE between e.g. fake B and true B
# + Cycle-consistency: Minimize MAE between reconstructed images and original
self.combined = Model(inputs=[img_A, img_B],
outputs=[ valid_A, valid_B,
fake_B, fake_A,
reconstr_A, reconstr_B ])
self.combined.compile(loss=['mse', 'mse',
'mae', 'mae',
'mae', 'mae'],
optimizer=optimizer)
def build_generator(self):
"""U-Net Generator"""
def conv2d(layer_input, filters, f_size=4, normalize=True):
"""Layers used during downsampling"""
d = Conv2D(filters, kernel_size=f_size, strides=2, padding='same')(layer_input)
d = LeakyReLU(alpha=0.2)(d)
if normalize:
d = InstanceNormalization()(d)
return d
def deconv2d(layer_input, skip_input, filters, f_size=4, dropout_rate=0):
"""Layers used during upsampling"""
u = UpSampling2D(size=2)(layer_input)
u = Conv2D(filters, kernel_size=f_size, strides=1, padding='same', activation='relu')(u)
if dropout_rate:
u = Dropout(dropout_rate)(u)
u = InstanceNormalization()(u)
u = Concatenate()([u, skip_input])
return u
# Image input
d0 = Input(shape=self.img_shape)
# Downsampling
d1 = conv2d(d0, self.gf, normalize=False)
d2 = conv2d(d1, self.gf*2)
d3 = conv2d(d2, self.gf*4)
d4 = conv2d(d3, self.gf*8)
d5 = conv2d(d4, self.gf*8)
d6 = conv2d(d5, self.gf*8)
d7 = conv2d(d6, self.gf*8)
# Upsampling
u1 = deconv2d(d7, d6, self.gf*8)
u2 = deconv2d(u1, d5, self.gf*8)
u3 = deconv2d(u2, d4, self.gf*8)
u4 = deconv2d(u3, d3, self.gf*4)
u5 = deconv2d(u4, d2, self.gf*2)
u6 = deconv2d(u5, d1, self.gf)
u7 = UpSampling2D(size=2)(u6)
output_img = Conv2D(self.channels, kernel_size=4, strides=1,
padding='same', activation='tanh')(u7)
return Model(d0, output_img)
def build_discriminator(self):
def d_layer(layer_input, filters, f_size=4, normalization=True):
"""Discriminator layer"""
d = Conv2D(filters, kernel_size=f_size, strides=2, padding='same')(layer_input)
d = LeakyReLU(alpha=0.2)(d)
if normalization:
d = InstanceNormalization()(d)
return d
img = Input(shape=self.img_shape)
d1 = d_layer(img, self.df, normalization=False)
d2 = d_layer(d1, self.df*2)
d3 = d_layer(d2, self.df*4)
d4 = d_layer(d3, self.df*8)
validity = Conv2D(1, kernel_size=4, strides=1, padding='same')(d4)
return Model(img, validity)
def train(self, epochs, batch_size=128, sample_interval=50):
start_time = datetime.datetime.now()
# Adversarial loss ground truths
valid = np.ones((batch_size,) + self.disc_patch)
fake = np.zeros((batch_size,) + self.disc_patch)
for epoch in range(epochs):
for batch_i, (imgs_A, imgs_B) in enumerate(self.data_loader.load_batch(batch_size)):
# ----------------------
# Train Discriminators
# ----------------------
# Translate images to opposite domain
fake_B = self.g_AB.predict(imgs_A)
fake_A = self.g_BA.predict(imgs_B)
# Train the discriminators (original images = real / translated = Fake)
dA_loss_real = self.d_A.train_on_batch(imgs_A, valid)
dA_loss_fake = self.d_A.train_on_batch(fake_A, fake)
dA_loss = 0.5 * np.add(dA_loss_real, dA_loss_fake)
dB_loss_real = self.d_B.train_on_batch(imgs_B, valid)
dB_loss_fake = self.d_B.train_on_batch(fake_B, fake)
dB_loss = 0.5 * np.add(dB_loss_real, dB_loss_fake)
# Total disciminator loss
d_loss = 0.5 * np.add(dA_loss, dB_loss)
# ------------------
# Train Generators
# ------------------
# Train the generators
g_loss = self.combined.train_on_batch([imgs_A, imgs_B], [valid, valid, \
imgs_B, imgs_A, \
imgs_A, imgs_B])
elapsed_time = datetime.datetime.now() - start_time
# Plot the progress
print ("[%d] [%d/%d] time: %s, [d_loss: %f, g_loss: %f]" % (epoch, batch_i,
self.data_loader.n_batches,
elapsed_time,
d_loss[0], g_loss[0]))
# If at save interval => save generated image samples
if batch_i % sample_interval == 0:
self.sample_images(epoch, batch_i)
def sample_images(self, epoch, batch_i):
os.makedirs('images/%s' % self.dataset_name, exist_ok=True)
r, c = 2, 3
imgs_A, imgs_B = self.data_loader.load_data(batch_size=1, is_testing=True)
# Translate images to the other domain
fake_B = self.g_AB.predict(imgs_A)
fake_A = self.g_BA.predict(imgs_B)
# Translate back to original domain
reconstr_A = self.g_BA.predict(fake_B)
reconstr_B = self.g_AB.predict(fake_A)
gen_imgs = np.concatenate([imgs_A, fake_B, reconstr_A, imgs_B, fake_A, reconstr_B])
# Rescale images 0 - 1
gen_imgs = 0.5 * gen_imgs + 0.5
titles = ['Original', 'Translated', 'Reconstructed']
fig, axs = plt.subplots(r, c)
cnt = 0
for i in range(r):
for j in range(c):
axs[i,j].imshow(gen_imgs[cnt])
axs[i, j].set_title(titles[j])
axs[i,j].axis('off')
cnt += 1
fig.savefig("images/%s/%d_%d.png" % (self.dataset_name, epoch, batch_i))
plt.close()
if __name__ == '__main__':
gan = DiscoGAN()
gan.train(epochs=5, batch_size=1, sample_interval=200)
3.12 DualGAN: Unsupervised Dual Learning for Image-to-Image Translation
代码及运行结果
dualgan.py
from __future__ import print_function, division
import scipy
from tensorflow.keras.datasets import mnist
from tensorflow.keras.layers import Input, Dense, Reshape, Flatten, Dropout, Concatenate
from tensorflow.keras.layers import BatchNormalization, Activation, ZeroPadding2D
from tensorflow.keras.layers import LeakyReLU
from tensorflow.keras.layers import UpSampling2D, Conv2D
from tensorflow.keras.models import Sequential, Model
from tensorflow.keras.optimizers import RMSprop, Adam
from tensorflow.keras.utils import to_categorical
import tensorflow.keras.backend as K
import matplotlib.pyplot as plt
import sys
import numpy as np
class DUALGAN():
def __init__(self):
self.img_rows = 28
self.img_cols = 28
self.channels = 1
self.img_dim = self.img_rows*self.img_cols
optimizer = Adam(0.0002, 0.5)
# Build and compile the discriminators
self.D_A = self.build_discriminator()
self.D_A.compile(loss=self.wasserstein_loss,
optimizer=optimizer,
metrics=['accuracy'])
self.D_B = self.build_discriminator()
self.D_B.compile(loss=self.wasserstein_loss,
optimizer=optimizer,
metrics=['accuracy'])
#-------------------------
# Construct Computational
# Graph of Generators
#-------------------------
# Build the generators
self.G_AB = self.build_generator()
self.G_BA = self.build_generator()
# For the combined model we will only train the generators
self.D_A.trainable = False
self.D_B.trainable = False
# The generator takes images from their respective domains as inputs
imgs_A = Input(shape=(self.img_dim,))
imgs_B = Input(shape=(self.img_dim,))
# Generators translates the images to the opposite domain
fake_B = self.G_AB(imgs_A)
fake_A = self.G_BA(imgs_B)
# The discriminators determines validity of translated images
valid_A = self.D_A(fake_A)
valid_B = self.D_B(fake_B)
# Generators translate the images back to their original domain
recov_A = self.G_BA(fake_B)
recov_B = self.G_AB(fake_A)
# The combined model (stacked generators and discriminators)
self.combined = Model(inputs=[imgs_A, imgs_B], outputs=[valid_A, valid_B, recov_A, recov_B])
self.combined.compile(loss=[self.wasserstein_loss, self.wasserstein_loss, 'mae', 'mae'],
optimizer=optimizer,
loss_weights=[1, 1, 100, 100])
def build_generator(self):
X = Input(shape=(self.img_dim,))
model = Sequential()
model.add(Dense(256, input_dim=self.img_dim))
model.add(LeakyReLU(alpha=0.2))
model.add(BatchNormalization(momentum=0.8))
model.add(Dropout(0.4))
model.add(Dense(512))
model.add(LeakyReLU(alpha=0.2))
model.add(BatchNormalization(momentum=0.8))
model.add(Dropout(0.4))
model.add(Dense(1024))
model.add(LeakyReLU(alpha=0.2))
model.add(BatchNormalization(momentum=0.8))
model.add(Dropout(0.4))
model.add(Dense(self.img_dim, activation='tanh'))
X_translated = model(X)
return Model(X, X_translated)
def build_discriminator(self):
img = Input(shape=(self.img_dim,))
model = Sequential()
model.add(Dense(512, input_dim=self.img_dim))
model.add(LeakyReLU(alpha=0.2))
model.add(Dense(256))
model.add(LeakyReLU(alpha=0.2))
model.add(BatchNormalization(momentum=0.8))
model.add(Dense(1))
validity = model(img)
return Model(img, validity)
def sample_generator_input(self, X, batch_size):
# Sample random batch of images from X
idx = np.random.randint(0, X.shape[0], batch_size)
return X[idx]
def wasserstein_loss(self, y_true, y_pred):
return K.mean(y_true * y_pred)
def train(self, epochs, batch_size=128, sample_interval=50):
# Load the dataset
(X_train, _), (_, _) = mnist.load_data()
# Rescale -1 to 1
X_train = (X_train.astype(np.float32) - 127.5) / 127.5
# Domain A and B (rotated)
X_A = X_train[:int(X_train.shape[0]/2)]
X_B = scipy.ndimage.interpolation.rotate(X_train[int(X_train.shape[0]/2):], 90, axes=(1, 2))
X_A = X_A.reshape(X_A.shape[0], self.img_dim)
X_B = X_B.reshape(X_B.shape[0], self.img_dim)
clip_value = 0.01
n_critic = 4
# Adversarial ground truths
valid = -np.ones((batch_size, 1))
fake = np.ones((batch_size, 1))
for epoch in range(epochs):
# Train the discriminator for n_critic iterations
for _ in range(n_critic):
# ----------------------
# Train Discriminators
# ----------------------
# Sample generator inputs
imgs_A = self.sample_generator_input(X_A, batch_size)
imgs_B = self.sample_generator_input(X_B, batch_size)
# Translate images to their opposite domain
fake_B = self.G_AB.predict(imgs_A)
fake_A = self.G_BA.predict(imgs_B)
# Train the discriminators
D_A_loss_real = self.D_A.train_on_batch(imgs_A, valid)
D_A_loss_fake = self.D_A.train_on_batch(fake_A, fake)
D_B_loss_real = self.D_B.train_on_batch(imgs_B, valid)
D_B_loss_fake = self.D_B.train_on_batch(fake_B, fake)
D_A_loss = 0.5 * np.add(D_A_loss_real, D_A_loss_fake)
D_B_loss = 0.5 * np.add(D_B_loss_real, D_B_loss_fake)
# Clip discriminator weights
for d in [self.D_A, self.D_B]:
for l in d.layers:
weights = l.get_weights()
weights = [np.clip(w, -clip_value, clip_value) for w in weights]
l.set_weights(weights)
# ------------------
# Train Generators
# ------------------
# Train the generators
g_loss = self.combined.train_on_batch([imgs_A, imgs_B], [valid, valid, imgs_A, imgs_B])
# Plot the progress
print ("%d [D1 loss: %f] [D2 loss: %f] [G loss: %f]" \
% (epoch, D_A_loss[0], D_B_loss[0], g_loss[0]))
# If at save interval => save generated image samples
if epoch % sample_interval == 0:
self.save_imgs(epoch, X_A, X_B)
def save_imgs(self, epoch, X_A, X_B):
r, c = 4, 4
# Sample generator inputs
imgs_A = self.sample_generator_input(X_A, c)
imgs_B = self.sample_generator_input(X_B, c)
# Images translated to their opposite domain
fake_B = self.G_AB.predict(imgs_A)
fake_A = self.G_BA.predict(imgs_B)
gen_imgs = np.concatenate([imgs_A, fake_B, imgs_B, fake_A])
gen_imgs = gen_imgs.reshape((r, c, self.img_rows, self.img_cols, 1))
# Rescale images 0 - 1
gen_imgs = 0.5 * gen_imgs + 0.5
fig, axs = plt.subplots(r, c)
cnt = 0
for i in range(r):
for j in range(c):
axs[i,j].imshow(gen_imgs[i, j, :,:,0], cmap='gray')
axs[i,j].axis('off')
cnt += 1
fig.savefig("images/mnist_%d.png" % epoch)
plt.close()
if __name__ == '__main__':
gan = DUALGAN()
gan.train(epochs=2000, batch_size=32, sample_interval=200)
3.13 Generative Adversarial Network
代码及运行结果
gan.py
from __future__ import print_function, division
from tensorflow.keras.datasets import mnist
from tensorflow.keras.layers import Input, Dense, Reshape, Flatten, Dropout
from tensorflow.keras.layers import BatchNormalization, Activation, ZeroPadding2D
from tensorflow.keras.layers import LeakyReLU
from tensorflow.keras.layers import UpSampling2D, Conv2D
from tensorflow.keras.models import Sequential, Model
from tensorflow.keras.optimizers import Adam
import matplotlib.pyplot as plt
import sys
import numpy as np
class GAN():
def __init__(self):
self.img_rows = 28
self.img_cols = 28
self.channels = 1
self.img_shape = (self.img_rows, self.img_cols, self.channels)
self.latent_dim = 100
optimizer = Adam(0.0002, 0.5)
# Build and compile the discriminator
self.discriminator = self.build_discriminator()
self.discriminator.compile(loss='binary_crossentropy',
optimizer=optimizer,
metrics=['accuracy'])
# Build the generator
self.generator = self.build_generator()
# The generator takes noise as input and generates imgs
z = Input(shape=(self.latent_dim,))
img = self.generator(z)
# For the combined model we will only train the generator
self.discriminator.trainable = False
# The discriminator takes generated images as input and determines validity
validity = self.discriminator(img)
# The combined model (stacked generator and discriminator)
# Trains the generator to fool the discriminator
self.combined = Model(z, validity)
self.combined.compile(loss='binary_crossentropy', optimizer=optimizer)
def build_generator(self):
model = Sequential()
model.add(Dense(256, input_dim=self.latent_dim))
model.add(LeakyReLU(alpha=0.2))
model.add(BatchNormalization(momentum=0.8))
model.add(Dense(512))
model.add(LeakyReLU(alpha=0.2))
model.add(BatchNormalization(momentum=0.8))
model.add(Dense(1024))
model.add(LeakyReLU(alpha=0.2))
model.add(BatchNormalization(momentum=0.8))
model.add(Dense(np.prod(self.img_shape), activation='tanh'))
model.add(Reshape(self.img_shape))
model.summary()
noise = Input(shape=(self.latent_dim,))
img = model(noise)
return Model(noise, img)
def build_discriminator(self):
model = Sequential()
model.add(Flatten(input_shape=self.img_shape))
model.add(Dense(512))
model.add(LeakyReLU(alpha=0.2))
model.add(Dense(256))
model.add(LeakyReLU(alpha=0.2))
model.add(Dense(1, activation='sigmoid'))
model.summary()
img = Input(shape=self.img_shape)
validity = model(img)
return Model(img, validity)
def train(self, epochs, batch_size=128, sample_interval=50):
# Load the dataset
(X_train, _), (_, _) = mnist.load_data()
# Rescale -1 to 1
X_train = X_train / 127.5 - 1.
X_train = np.expand_dims(X_train, axis=3)
# Adversarial ground truths
valid = np.ones((batch_size, 1))
fake = np.zeros((batch_size, 1))
for epoch in range(epochs):
# ---------------------
# Train Discriminator
# ---------------------
# Select a random batch of images
idx = np.random.randint(0, X_train.shape[0], batch_size)
imgs = X_train[idx]
noise = np.random.normal(0, 1, (batch_size, self.latent_dim))
# Generate a batch of new images
gen_imgs = self.generator.predict(noise)
# Train the discriminator
d_loss_real = self.discriminator.train_on_batch(imgs, valid)
d_loss_fake = self.discriminator.train_on_batch(gen_imgs, fake)
d_loss = 0.5 * np.add(d_loss_real, d_loss_fake)
# ---------------------
# Train Generator
# ---------------------
noise = np.random.normal(0, 1, (batch_size, self.latent_dim))
# Train the generator (to have the discriminator label samples as valid)
g_loss = self.combined.train_on_batch(noise, valid)
# Plot the progress
print ("%d [D loss: %f, acc.: %.2f%%] [G loss: %f]" % (epoch, d_loss[0], 100*d_loss[1], g_loss))
# If at save interval => save generated image samples
if epoch % sample_interval == 0:
self.sample_images(epoch)
def sample_images(self, epoch):
r, c = 5, 5
noise = np.random.normal(0, 1, (r * c, self.latent_dim))
gen_imgs = self.generator.predict(noise)
# Rescale images 0 - 1
gen_imgs = 0.5 * gen_imgs + 0.5
fig, axs = plt.subplots(r, c)
cnt = 0
for i in range(r):
for j in range(c):
axs[i,j].imshow(gen_imgs[cnt, :,:,0], cmap='gray')
axs[i,j].axis('off')
cnt += 1
fig.savefig("images/%d.png" % epoch)
plt.close()
if __name__ == '__main__':
gan = GAN()
gan.train(epochs=30000, batch_size=32, sample_interval=200)
3.14 InfoGAN: Interpretable Representation Learning by Information Maximizing Generative Adversarial Nets
代码及运行结果
infogan.py
from __future__ import print_function, division
from tensorflow.keras.datasets import mnist
from tensorflow.keras.layers import Input, Dense, Reshape, Flatten, Dropout, multiply, concatenate
from tensorflow.keras.layers import BatchNormalization, Activation, Embedding, ZeroPadding2D, Lambda
from tensorflow.keras.layers import LeakyReLU
from tensorflow.keras.layers import UpSampling2D, Conv2D
from tensorflow.keras.models import Sequential, Model
from tensorflow.keras.optimizers import Adam
from tensorflow.keras.utils import to_categorical
import tensorflow.keras.backend as K
import matplotlib.pyplot as plt
import numpy as np
class INFOGAN():
def __init__(self):
self.img_rows = 28
self.img_cols = 28
self.channels = 1
self.num_classes = 10
self.img_shape = (self.img_rows, self.img_cols, self.channels)
self.latent_dim = 72
optimizer = Adam(0.0002, 0.5)
losses = ['binary_crossentropy', self.mutual_info_loss]
# Build and the discriminator and recognition network
self.discriminator, self.auxilliary = self.build_disk_and_q_net()
self.discriminator.compile(loss=['binary_crossentropy'],
optimizer=optimizer,
metrics=['accuracy'])
# Build and compile the recognition network Q
self.auxilliary.compile(loss=[self.mutual_info_loss],
optimizer=optimizer,
metrics=['accuracy'])
# Build the generator
self.generator = self.build_generator()
# The generator takes noise and the target label as input
# and generates the corresponding digit of that label
gen_input = Input(shape=(self.latent_dim,))
img = self.generator(gen_input)
# For the combined model we will only train the generator
self.discriminator.trainable = False
# The discriminator takes generated image as input and determines validity
valid = self.discriminator(img)
# The recognition network produces the label
target_label = self.auxilliary(img)
# The combined model (stacked generator and discriminator)
self.combined = Model(gen_input, [valid, target_label])
self.combined.compile(loss=losses,
optimizer=optimizer)
def build_generator(self):
model = Sequential()
model.add(Dense(128 * 7 * 7, activation="relu", input_dim=self.latent_dim))
model.add(Reshape((7, 7, 128)))
model.add(BatchNormalization(momentum=0.8))
model.add(UpSampling2D())
model.add(Conv2D(128, kernel_size=3, padding="same"))
model.add(Activation("relu"))
model.add(BatchNormalization(momentum=0.8))
model.add(UpSampling2D())
model.add(Conv2D(64, kernel_size=3, padding="same"))
model.add(Activation("relu"))
model.add(BatchNormalization(momentum=0.8))
model.add(Conv2D(self.channels, kernel_size=3, padding='same'))
model.add(Activation("tanh"))
gen_input = Input(shape=(self.latent_dim,))
img = model(gen_input)
model.summary()
return Model(gen_input, img)
def build_disk_and_q_net(self):
img = Input(shape=self.img_shape)
# Shared layers between discriminator and recognition network
model = Sequential()
model.add(Conv2D(64, kernel_size=3, strides=2, input_shape=self.img_shape, padding="same"))
model.add(LeakyReLU(alpha=0.2))
model.add(Dropout(0.25))
model.add(Conv2D(128, kernel_size=3, strides=2, padding="same"))
model.add(ZeroPadding2D(padding=((0,1),(0,1))))
model.add(LeakyReLU(alpha=0.2))
model.add(Dropout(0.25))
model.add(BatchNormalization(momentum=0.8))
model.add(Conv2D(256, kernel_size=3, strides=2, padding="same"))
model.add(LeakyReLU(alpha=0.2))
model.add(Dropout(0.25))
model.add(BatchNormalization(momentum=0.8))
model.add(Conv2D(512, kernel_size=3, strides=2, padding="same"))
model.add(LeakyReLU(alpha=0.2))
model.add(Dropout(0.25))
model.add(BatchNormalization(momentum=0.8))
model.add(Flatten())
img_embedding = model(img)
# Discriminator
validity = Dense(1, activation='sigmoid')(img_embedding)
# Recognition
q_net = Dense(128, activation='relu')(img_embedding)
label = Dense(self.num_classes, activation='softmax')(q_net)
# Return discriminator and recognition network
return Model(img, validity), Model(img, label)
def mutual_info_loss(self, c, c_given_x):
"""The mutual information metric we aim to minimize"""
eps = 1e-8
conditional_entropy = K.mean(- K.sum(K.log(c_given_x + eps) * c, axis=1))
entropy = K.mean(- K.sum(K.log(c + eps) * c, axis=1))
return conditional_entropy + entropy
def sample_generator_input(self, batch_size):
# Generator inputs
sampled_noise = np.random.normal(0, 1, (batch_size, 62))
sampled_labels = np.random.randint(0, self.num_classes, batch_size).reshape(-1, 1)
sampled_labels = to_categorical(sampled_labels, num_classes=self.num_classes)
return sampled_noise, sampled_labels
def train(self, epochs, batch_size=128, sample_interval=50):
# Load the dataset
(X_train, y_train), (_, _) = mnist.load_data()
# Rescale -1 to 1
X_train = (X_train.astype(np.float32) - 127.5) / 127.5
X_train = np.expand_dims(X_train, axis=3)
y_train = y_train.reshape(-1, 1)
# Adversarial ground truths
valid = np.ones((batch_size, 1))
fake = np.zeros((batch_size, 1))
for epoch in range(epochs):
# ---------------------
# Train Discriminator
# ---------------------
# Select a random half batch of images
idx = np.random.randint(0, X_train.shape[0], batch_size)
imgs = X_train[idx]
# Sample noise and categorical labels
sampled_noise, sampled_labels = self.sample_generator_input(batch_size)
gen_input = np.concatenate((sampled_noise, sampled_labels), axis=1)
# Generate a half batch of new images
gen_imgs = self.generator.predict(gen_input)
# Train on real and generated data
d_loss_real = self.discriminator.train_on_batch(imgs, valid)
d_loss_fake = self.discriminator.train_on_batch(gen_imgs, fake)
# Avg. loss
d_loss = 0.5 * np.add(d_loss_real, d_loss_fake)
# ---------------------
# Train Generator and Q-network
# ---------------------
g_loss = self.combined.train_on_batch(gen_input, [valid, sampled_labels])
# Plot the progress
print ("%d [D loss: %.2f, acc.: %.2f%%] [Q loss: %.2f] [G loss: %.2f]" % (epoch, d_loss[0], 100*d_loss[1], g_loss[1], g_loss[2]))
# If at save interval => save generated image samples
if epoch % sample_interval == 0:
self.sample_images(epoch)
def sample_images(self, epoch):
r, c = 10, 10
fig, axs = plt.subplots(r, c)
for i in range(c):
sampled_noise, _ = self.sample_generator_input(c)
label = to_categorical(np.full(fill_value=i, shape=(r,1)), num_classes=self.num_classes)
gen_input = np.concatenate((sampled_noise, label), axis=1)
gen_imgs = self.generator.predict(gen_input)
gen_imgs = 0.5 * gen_imgs + 0.5
for j in range(r):
axs[j,i].imshow(gen_imgs[j,:,:,0], cmap='gray')
axs[j,i].axis('off')
fig.savefig("images/%d.png" % epoch)
plt.close()
def save_model(self):
def save(model, model_name):
model_path = "saved_model/%s.json" % model_name
weights_path = "saved_model/%s_weights.hdf5" % model_name
options = {"file_arch": model_path,
"file_weight": weights_path}
json_string = model.to_json()
open(options['file_arch'], 'w').write(json_string)
model.save_weights(options['file_weight'])
save(self.generator, "generator")
save(self.discriminator, "discriminator")
if __name__ == '__main__':
infogan = INFOGAN()
infogan.train(epochs=1000, batch_size=128, sample_interval=50)
3.15 Least Squares Generative Adversarial Networks
代码及运行结果
lsgan.py
from __future__ import print_function, division
from tensorflow.keras.datasets import mnist
from tensorflow.keras.layers import Input, Dense, Reshape, Flatten, Dropout
from tensorflow.keras.layers import BatchNormalization, Activation, ZeroPadding2D
from tensorflow.keras.layers import LeakyReLU
from tensorflow.keras.layers import UpSampling2D, Conv2D
from tensorflow.keras.models import Sequential, Model
from tensorflow.keras.optimizers import Adam
import matplotlib.pyplot as plt
import sys
import numpy as np
class LSGAN():
def __init__(self):
self.img_rows = 28
self.img_cols = 28
self.channels = 1
self.img_shape = (self.img_rows, self.img_cols, self.channels)
self.latent_dim = 100
optimizer = Adam(0.0002, 0.5)
# Build and compile the discriminator
self.discriminator = self.build_discriminator()
self.discriminator.compile(loss='mse',
optimizer=optimizer,
metrics=['accuracy'])
# Build the generator
self.generator = self.build_generator()
# The generator takes noise as input and generated imgs
z = Input(shape=(self.latent_dim,))
img = self.generator(z)
# For the combined model we will only train the generator
self.discriminator.trainable = False
# The valid takes generated images as input and determines validity
valid = self.discriminator(img)
# The combined model (stacked generator and discriminator)
# Trains generator to fool discriminator
self.combined = Model(z, valid)
# (!!!) Optimize w.r.t. MSE loss instead of crossentropy
self.combined.compile(loss='mse', optimizer=optimizer)
def build_generator(self):
model = Sequential()
model.add(Dense(256, input_dim=self.latent_dim))
model.add(LeakyReLU(alpha=0.2))
model.add(BatchNormalization(momentum=0.8))
model.add(Dense(512))
model.add(LeakyReLU(alpha=0.2))
model.add(BatchNormalization(momentum=0.8))
model.add(Dense(1024))
model.add(LeakyReLU(alpha=0.2))
model.add(BatchNormalization(momentum=0.8))
model.add(Dense(np.prod(self.img_shape), activation='tanh'))
model.add(Reshape(self.img_shape))
model.summary()
noise = Input(shape=(self.latent_dim,))
img = model(noise)
return Model(noise, img)
def build_discriminator(self):
model = Sequential()
model.add(Flatten(input_shape=self.img_shape))
model.add(Dense(512))
model.add(LeakyReLU(alpha=0.2))
model.add(Dense(256))
model.add(LeakyReLU(alpha=0.2))
# (!!!) No softmax
model.add(Dense(1))
model.summary()
img = Input(shape=self.img_shape)
validity = model(img)
return Model(img, validity)
def train(self, epochs, batch_size=128, sample_interval=50):
# Load the dataset
(X_train, _), (_, _) = mnist.load_data()
# Rescale -1 to 1
X_train = (X_train.astype(np.float32) - 127.5) / 127.5
X_train = np.expand_dims(X_train, axis=3)
# Adversarial ground truths
valid = np.ones((batch_size, 1))
fake = np.zeros((batch_size, 1))
for epoch in range(epochs):
# ---------------------
# Train Discriminator
# ---------------------
# Select a random batch of images
idx = np.random.randint(0, X_train.shape[0], batch_size)
imgs = X_train[idx]
# Sample noise as generator input
noise = np.random.normal(0, 1, (batch_size, self.latent_dim))
# Generate a batch of new images
gen_imgs = self.generator.predict(noise)
# Train the discriminator
d_loss_real = self.discriminator.train_on_batch(imgs, valid)
d_loss_fake = self.discriminator.train_on_batch(gen_imgs, fake)
d_loss = 0.5 * np.add(d_loss_real, d_loss_fake)
# ---------------------
# Train Generator
# ---------------------
g_loss = self.combined.train_on_batch(noise, valid)
# Plot the progress
print ("%d [D loss: %f, acc.: %.2f%%] [G loss: %f]" % (epoch, d_loss[0], 100*d_loss[1], g_loss))
# If at save interval => save generated image samples
if epoch % sample_interval == 0:
self.sample_images(epoch)
def sample_images(self, epoch):
r, c = 5, 5
noise = np.random.normal(0, 1, (r * c, self.latent_dim))
gen_imgs = self.generator.predict(noise)
# Rescale images 0 - 1
gen_imgs = 0.5 * gen_imgs + 0.5
fig, axs = plt.subplots(r, c)
cnt = 0
for i in range(r):
for j in range(c):
axs[i,j].imshow(gen_imgs[cnt, :,:,0], cmap='gray')
axs[i,j].axis('off')
cnt += 1
fig.savefig("images/mnist_%d.png" % epoch)
plt.close()
if __name__ == '__main__':
gan = LSGAN()
gan.train(epochs=30000, batch_size=32, sample_interval=200)
3.16 Image-to-Image Translation with Conditional Adversarial Networks
代码及运行结果
pix2pix.py
from __future__ import print_function, division
import scipy
from tensorflow.keras.datasets import mnist
from instancenormalization import InstanceNormalization
from tensorflow.keras.layers import Input, Dense, Reshape, Flatten, Dropout, Concatenate
from tensorflow.keras.layers import BatchNormalization, Activation, ZeroPadding2D
from tensorflow.keras.layers import LeakyReLU
from tensorflow.keras.layers import UpSampling2D, Conv2D
from tensorflow.keras.models import Sequential, Model
from tensorflow.keras.optimizers import Adam
import datetime
import matplotlib.pyplot as plt
import sys
from data_loader import DataLoader
import numpy as np
import os
class Pix2Pix():
def __init__(self):
# Input shape
self.img_rows = 256
self.img_cols = 256
self.channels = 3
self.img_shape = (self.img_rows, self.img_cols, self.channels)
# Configure data loader
self.dataset_name = 'facades'
self.data_loader = DataLoader(dataset_name=self.dataset_name,
img_res=(self.img_rows, self.img_cols))
# Calculate output shape of D (PatchGAN)
patch = int(self.img_rows / 2**4)
self.disc_patch = (patch, patch, 1)
# Number of filters in the first layer of G and D
self.gf = 64
self.df = 64
optimizer = Adam(0.0002, 0.5)
# Build and compile the discriminator
self.discriminator = self.build_discriminator()
self.discriminator.compile(loss='mse',
optimizer=optimizer,
metrics=['accuracy'])
#-------------------------
# Construct Computational
# Graph of Generator
#-------------------------
# Build the generator
self.generator = self.build_generator()
# Input images and their conditioning images
img_A = Input(shape=self.img_shape)
img_B = Input(shape=self.img_shape)
# By conditioning on B generate a fake version of A
fake_A = self.generator(img_B)
# For the combined model we will only train the generator
self.discriminator.trainable = False
# Discriminators determines validity of translated images / condition pairs
valid = self.discriminator([fake_A, img_B])
self.combined = Model(inputs=[img_A, img_B], outputs=[valid, fake_A])
self.combined.compile(loss=['mse', 'mae'],
loss_weights=[1, 100],
optimizer=optimizer)
def build_generator(self):
"""U-Net Generator"""
def conv2d(layer_input, filters, f_size=4, bn=True):
"""Layers used during downsampling"""
d = Conv2D(filters, kernel_size=f_size, strides=2, padding='same')(layer_input)
d = LeakyReLU(alpha=0.2)(d)
if bn:
d = BatchNormalization(momentum=0.8)(d)
return d
def deconv2d(layer_input, skip_input, filters, f_size=4, dropout_rate=0):
"""Layers used during upsampling"""
u = UpSampling2D(size=2)(layer_input)
u = Conv2D(filters, kernel_size=f_size, strides=1, padding='same', activation='relu')(u)
if dropout_rate:
u = Dropout(dropout_rate)(u)
u = BatchNormalization(momentum=0.8)(u)
u = Concatenate()([u, skip_input])
return u
# Image input
d0 = Input(shape=self.img_shape)
# Downsampling
d1 = conv2d(d0, self.gf, bn=False)
d2 = conv2d(d1, self.gf*2)
d3 = conv2d(d2, self.gf*4)
d4 = conv2d(d3, self.gf*8)
d5 = conv2d(d4, self.gf*8)
d6 = conv2d(d5, self.gf*8)
d7 = conv2d(d6, self.gf*8)
# Upsampling
u1 = deconv2d(d7, d6, self.gf*8)
u2 = deconv2d(u1, d5, self.gf*8)
u3 = deconv2d(u2, d4, self.gf*8)
u4 = deconv2d(u3, d3, self.gf*4)
u5 = deconv2d(u4, d2, self.gf*2)
u6 = deconv2d(u5, d1, self.gf)
u7 = UpSampling2D(size=2)(u6)
output_img = Conv2D(self.channels, kernel_size=4, strides=1, padding='same', activation='tanh')(u7)
return Model(d0, output_img)
def build_discriminator(self):
def d_layer(layer_input, filters, f_size=4, bn=True):
"""Discriminator layer"""
d = Conv2D(filters, kernel_size=f_size, strides=2, padding='same')(layer_input)
d = LeakyReLU(alpha=0.2)(d)
if bn:
d = BatchNormalization(momentum=0.8)(d)
return d
img_A = Input(shape=self.img_shape)
img_B = Input(shape=self.img_shape)
# Concatenate image and conditioning image by channels to produce input
combined_imgs = Concatenate(axis=-1)([img_A, img_B])
d1 = d_layer(combined_imgs, self.df, bn=False)
d2 = d_layer(d1, self.df*2)
d3 = d_layer(d2, self.df*4)
d4 = d_layer(d3, self.df*8)
validity = Conv2D(1, kernel_size=4, strides=1, padding='same')(d4)
return Model([img_A, img_B], validity)
def train(self, epochs, batch_size=1, sample_interval=50):
start_time = datetime.datetime.now()
# Adversarial loss ground truths
valid = np.ones((batch_size,) + self.disc_patch)
fake = np.zeros((batch_size,) + self.disc_patch)
for epoch in range(epochs):
for batch_i, (imgs_A, imgs_B) in enumerate(self.data_loader.load_batch(batch_size)):
# ---------------------
# Train Discriminator
# ---------------------
# Condition on B and generate a translated version
fake_A = self.generator.predict(imgs_B)
# Train the discriminators (original images = real / generated = Fake)
d_loss_real = self.discriminator.train_on_batch([imgs_A, imgs_B], valid)
d_loss_fake = self.discriminator.train_on_batch([fake_A, imgs_B], fake)
d_loss = 0.5 * np.add(d_loss_real, d_loss_fake)
# -----------------
# Train Generator
# -----------------
# Train the generators
g_loss = self.combined.train_on_batch([imgs_A, imgs_B], [valid, imgs_A])
elapsed_time = datetime.datetime.now() - start_time
# Plot the progress
print ("[Epoch %d/%d] [Batch %d/%d] [D loss: %f, acc: %3d%%] [G loss: %f] time: %s" % (epoch, epochs,
batch_i, self.data_loader.n_batches,
d_loss[0], 100*d_loss[1],
g_loss[0],
elapsed_time))
# If at save interval => save generated image samples
if batch_i % sample_interval == 0:
self.sample_images(epoch, batch_i)
def sample_images(self, epoch, batch_i):
os.makedirs('images/%s' % self.dataset_name, exist_ok=True)
r, c = 3, 3
imgs_A, imgs_B = self.data_loader.load_data(batch_size=3, is_testing=True)
fake_A = self.generator.predict(imgs_B)
gen_imgs = np.concatenate([imgs_B, fake_A, imgs_A])
# Rescale images 0 - 1
gen_imgs = 0.5 * gen_imgs + 0.5
titles = ['Condition', 'Generated', 'Original']
fig, axs = plt.subplots(r, c)
cnt = 0
for i in range(r):
for j in range(c):
axs[i,j].imshow(gen_imgs[cnt])
axs[i, j].set_title(titles[i])
axs[i,j].axis('off')
cnt += 1
fig.savefig("images/%s/%d_%d.png" % (self.dataset_name, epoch, batch_i))
plt.close()
if __name__ == '__main__':
gan = Pix2Pix()
gan.train(epochs=200, batch_size=1, sample_interval=200)
3.17 Unsupervised Pixel-Level Domain Adaptation with Generative Adversarial Networks
代码及运行结果
keras_mnistm.pkl.gz下载
instanceNormalization.py
from tensorflow.keras.layers import Layer, InputSpec
from tensorflow.keras import initializers, regularizers, constraints
from tensorflow.keras import backend as K
class InstanceNormalization(Layer):
"""Instance normalization layer.
Normalize the activations of the previous layer at each step,
i.e. applies a transformation that maintains the mean activation
close to 0 and the activation standard deviation close to 1.
# Arguments
axis: Integer, the axis that should be normalized
(typically the features axis).
For instance, after a `Conv2D` layer with
`data_format="channels_first"`,
set `axis=1` in `InstanceNormalization`.
Setting `axis=None` will normalize all values in each
instance of the batch.
Axis 0 is the batch dimension. `axis` cannot be set to 0 to avoid errors.
epsilon: Small float added to variance to avoid dividing by zero.
center: If True, add offset of `beta` to normalized tensor.
If False, `beta` is ignored.
scale: If True, multiply by `gamma`.
If False, `gamma` is not used.
When the next layer is linear (also e.g. `nn.relu`),
this can be disabled since the scaling
will be done by the next layer.
beta_initializer: Initializer for the beta weight.
gamma_initializer: Initializer for the gamma weight.
beta_regularizer: Optional regularizer for the beta weight.
gamma_regularizer: Optional regularizer for the gamma weight.
beta_constraint: Optional constraint for the beta weight.
gamma_constraint: Optional constraint for the gamma weight.
# Input shape
Arbitrary. Use the keyword argument `input_shape`
(tuple of integers, does not include the samples axis)
when using this layer as the first layer in a Sequential model.
# Output shape
Same shape as input.
# References
- [Layer Normalization](https://arxiv.org/abs/1607.06450)
- [Instance Normalization: The Missing Ingredient for Fast Stylization](
https://arxiv.org/abs/1607.08022)
"""
def __init__(self,
axis=None,
epsilon=1e-3,
center=True,
scale=True,
beta_initializer='zeros',
gamma_initializer='ones',
beta_regularizer=None,
gamma_regularizer=None,
beta_constraint=None,
gamma_constraint=None,
**kwargs):
super(InstanceNormalization, self).__init__(**kwargs)
self.supports_masking = True
self.axis = axis
self.epsilon = epsilon
self.center = center
self.scale = scale
self.beta_initializer = initializers.get(beta_initializer)
self.gamma_initializer = initializers.get(gamma_initializer)
self.beta_regularizer = regularizers.get(beta_regularizer)
self.gamma_regularizer = regularizers.get(gamma_regularizer)
self.beta_constraint = constraints.get(beta_constraint)
self.gamma_constraint = constraints.get(gamma_constraint)
def build(self, input_shape):
ndim = len(input_shape)
if self.axis == 0:
raise ValueError('Axis cannot be zero')
if (self.axis is not None) and (ndim == 2):
raise ValueError('Cannot specify axis for rank 1 tensor')
self.input_spec = InputSpec(ndim=ndim)
if self.axis is None:
shape = (1,)
else:
shape = (input_shape[self.axis],)
if self.scale:
self.gamma = self.add_weight(shape=shape,
name='gamma',
initializer=self.gamma_initializer,
regularizer=self.gamma_regularizer,
constraint=self.gamma_constraint)
else:
self.gamma = None
if self.center:
self.beta = self.add_weight(shape=shape,
name='beta',
initializer=self.beta_initializer,
regularizer=self.beta_regularizer,
constraint=self.beta_constraint)
else:
self.beta = None
self.built = True
def call(self, inputs, training=None):
input_shape = K.int_shape(inputs)
reduction_axes = list(range(0, len(input_shape)))
if self.axis is not None:
del reduction_axes[self.axis]
del reduction_axes[0]
mean = K.mean(inputs, reduction_axes, keepdims=True)
stddev = K.std(inputs, reduction_axes, keepdims=True) + self.epsilon
normed = (inputs - mean) / stddev
broadcast_shape = [1] * len(input_shape)
if self.axis is not None:
broadcast_shape[self.axis] = input_shape[self.axis]
if self.scale:
broadcast_gamma = K.reshape(self.gamma, broadcast_shape)
normed = normed * broadcast_gamma
if self.center:
broadcast_beta = K.reshape(self.beta, broadcast_shape)
normed = normed + broadcast_beta
return normed
def get_config(self):
config = {
'axis': self.axis,
'epsilon': self.epsilon,
'center': self.center,
'scale': self.scale,
'beta_initializer': initializers.serialize(self.beta_initializer),
'gamma_initializer': initializers.serialize(self.gamma_initializer),
'beta_regularizer': regularizers.serialize(self.beta_regularizer),
'gamma_regularizer': regularizers.serialize(self.gamma_regularizer),
'beta_constraint': constraints.serialize(self.beta_constraint),
'gamma_constraint': constraints.serialize(self.gamma_constraint)
}
base_config = super(InstanceNormalization, self).get_config()
return dict(list(base_config.items()) + list(config.items()))
data_loader.py
import scipy
from glob import glob
import numpy as np
from tensorflow.keras.datasets import mnist
from scipy.misc import imresize
import pickle
import os
import urllib
import gzip
class DataLoader():
"""Loads images from MNIST (domain A) and MNIST-M (domain B)"""
def __init__(self, img_res=(128, 128)):
self.img_res = img_res
self.mnistm_url = 'https://hub.fastgit.xyz/VanushVaswani/keras_mnistm/releases/download/1.0/keras_mnistm.pkl.gz'
self.setup_mnist(img_res)
self.setup_mnistm(img_res)
def normalize(self, images):
return images.astype(np.float32) / 127.5 - 1.
def setup_mnist(self, img_res):
print ("Setting up MNIST...")
if not os.path.exists('datasets/mnist_x.npy'):
# Load the dataset
(mnist_X, mnist_y), (_, _) = mnist.load_data()
# Normalize and rescale images
mnist_X = self.normalize(mnist_X)
mnist_X = np.array([imresize(x, img_res) for x in mnist_X])
mnist_X = np.expand_dims(mnist_X, axis=-1)
mnist_X = np.repeat(mnist_X, 3, axis=-1)
self.mnist_X, self.mnist_y = mnist_X, mnist_y
# Save formatted images
np.save('datasets/mnist_x.npy', self.mnist_X)
np.save('datasets/mnist_y.npy', self.mnist_y)
else:
self.mnist_X = np.load('datasets/mnist_x.npy')
self.mnist_y = np.load('datasets/mnist_y.npy')
print ("+ Done.")
def setup_mnistm(self, img_res):
print ("Setting up MNIST-M...")
if not os.path.exists('datasets/mnistm_x.npy'):
# Download the MNIST-M pkl file
filepath = 'datasets/keras_mnistm.pkl.gz'
if not os.path.exists(filepath.replace('.gz', '')):
print('+ Downloading ' + self.mnistm_url)
data = urllib.request.urlopen(self.mnistm_url)
with open(filepath, 'wb') as f:
f.write(data.read())
with open(filepath.replace('.gz', ''), 'wb') as out_f, \
gzip.GzipFile(filepath) as zip_f:
out_f.write(zip_f.read())
os.unlink(filepath)
# load MNIST-M images from pkl file
with open('datasets/keras_mnistm.pkl', "rb") as f:
data = pickle.load(f, encoding='bytes')
# Normalize and rescale images
mnistm_X = np.array(data[b'train'])
mnistm_X = self.normalize(mnistm_X)
mnistm_X = np.array([imresize(x, img_res) for x in mnistm_X])
self.mnistm_X, self.mnistm_y = mnistm_X, self.mnist_y.copy()
# Save formatted images
np.save('datasets/mnistm_x.npy', self.mnistm_X)
np.save('datasets/mnistm_y.npy', self.mnistm_y)
else:
self.mnistm_X = np.load('datasets/mnistm_x.npy')
self.mnistm_y = np.load('datasets/mnistm_y.npy')
print ("+ Done.")
def load_data(self, domain, batch_size=1):
X = self.mnist_X if domain == 'A' else self.mnistm_X
y = self.mnist_y if domain == 'A' else self.mnistm_y
idx = np.random.choice(list(range(len(X))), size=batch_size)
return X[idx], y[idx]
pixelda.py
from __future__ import print_function, division
import scipy
from tensorflow.keras.datasets import mnist
from instancenormalization import InstanceNormalization
from tensorflow.keras.layers import Input, Dense, Reshape, Flatten, Dropout, Concatenate
from tensorflow.keras.layers import BatchNormalization, Activation, ZeroPadding2D, Add
from tensorflow.keras.layers import LeakyReLU
from tensorflow.keras.layers import UpSampling2D, Conv2D
from tensorflow.keras.models import Sequential, Model
from tensorflow.keras.optimizers import Adam
from tensorflow.keras.utils import to_categorical
import datetime
import matplotlib.pyplot as plt
import sys
from data_loader import DataLoader
import numpy as np
import os
class PixelDA():
def __init__(self):
# Input shape
self.img_rows = 32
self.img_cols = 32
self.channels = 3
self.img_shape = (self.img_rows, self.img_cols, self.channels)
self.num_classes = 10
# Configure MNIST and MNIST-M data loader
self.data_loader = DataLoader(img_res=(self.img_rows, self.img_cols))
# Loss weights
lambda_adv = 10
lambda_clf = 1
# Calculate output shape of D (PatchGAN)
patch = int(self.img_rows / 2**4)
self.disc_patch = (patch, patch, 1)
# Number of residual blocks in the generator
self.residual_blocks = 6
optimizer = Adam(0.0002, 0.5)
# Number of filters in first layer of discriminator and classifier
self.df = 64
self.cf = 64
# Build and compile the discriminators
self.discriminator = self.build_discriminator()
self.discriminator.compile(loss='mse',
optimizer=optimizer,
metrics=['accuracy'])
# Build the generator
self.generator = self.build_generator()
# Build the task (classification) network
self.clf = self.build_classifier()
# Input images from both domains
img_A = Input(shape=self.img_shape)
img_B = Input(shape=self.img_shape)
# Translate images from domain A to domain B
fake_B = self.generator(img_A)
# Classify the translated image
class_pred = self.clf(fake_B)
# For the combined model we will only train the generator and classifier
self.discriminator.trainable = False
# Discriminator determines validity of translated images
valid = self.discriminator(fake_B)
self.combined = Model(img_A, [valid, class_pred])
self.combined.compile(loss=['mse', 'categorical_crossentropy'],
loss_weights=[lambda_adv, lambda_clf],
optimizer=optimizer,
metrics=['accuracy'])
def build_generator(self):
"""Resnet Generator"""
def residual_block(layer_input):
"""Residual block described in paper"""
d = Conv2D(64, kernel_size=3, strides=1, padding='same')(layer_input)
d = BatchNormalization(momentum=0.8)(d)
d = Activation('relu')(d)
d = Conv2D(64, kernel_size=3, strides=1, padding='same')(d)
d = BatchNormalization(momentum=0.8)(d)
d = Add()([d, layer_input])
return d
# Image input
img = Input(shape=self.img_shape)
l1 = Conv2D(64, kernel_size=3, padding='same', activation='relu')(img)
# Propogate signal through residual blocks
r = residual_block(l1)
for _ in range(self.residual_blocks - 1):
r = residual_block(r)
output_img = Conv2D(self.channels, kernel_size=3, padding='same', activation='tanh')(r)
return Model(img, output_img)
def build_discriminator(self):
def d_layer(layer_input, filters, f_size=4, normalization=True):
"""Discriminator layer"""
d = Conv2D(filters, kernel_size=f_size, strides=2, padding='same')(layer_input)
d = LeakyReLU(alpha=0.2)(d)
if normalization:
d = InstanceNormalization()(d)
return d
img = Input(shape=self.img_shape)
d1 = d_layer(img, self.df, normalization=False)
d2 = d_layer(d1, self.df*2)
d3 = d_layer(d2, self.df*4)
d4 = d_layer(d3, self.df*8)
validity = Conv2D(1, kernel_size=4, strides=1, padding='same')(d4)
return Model(img, validity)
def build_classifier(self):
def clf_layer(layer_input, filters, f_size=4, normalization=True):
"""Classifier layer"""
d = Conv2D(filters, kernel_size=f_size, strides=2, padding='same')(layer_input)
d = LeakyReLU(alpha=0.2)(d)
if normalization:
d = InstanceNormalization()(d)
return d
img = Input(shape=self.img_shape)
c1 = clf_layer(img, self.cf, normalization=False)
c2 = clf_layer(c1, self.cf*2)
c3 = clf_layer(c2, self.cf*4)
c4 = clf_layer(c3, self.cf*8)
c5 = clf_layer(c4, self.cf*8)
class_pred = Dense(self.num_classes, activation='softmax')(Flatten()(c5))
return Model(img, class_pred)
def train(self, epochs, batch_size=128, sample_interval=50):
half_batch = int(batch_size / 2)
# Classification accuracy on 100 last batches of domain B
test_accs = []
# Adversarial ground truths
valid = np.ones((batch_size, *self.disc_patch))
fake = np.zeros((batch_size, *self.disc_patch))
for epoch in range(epochs):
# ---------------------
# Train Discriminator
# ---------------------
imgs_A, labels_A = self.data_loader.load_data(domain="A", batch_size=batch_size)
imgs_B, labels_B = self.data_loader.load_data(domain="B", batch_size=batch_size)
# Translate images from domain A to domain B
fake_B = self.generator.predict(imgs_A)
# Train the discriminators (original images = real / translated = Fake)
d_loss_real = self.discriminator.train_on_batch(imgs_B, valid)
d_loss_fake = self.discriminator.train_on_batch(fake_B, fake)
d_loss = 0.5 * np.add(d_loss_real, d_loss_fake)
# --------------------------------
# Train Generator and Classifier
# --------------------------------
# One-hot encoding of labels
labels_A = to_categorical(labels_A, num_classes=self.num_classes)
# Train the generator and classifier
g_loss = self.combined.train_on_batch(imgs_A, [valid, labels_A])
#-----------------------
# Evaluation (domain B)
#-----------------------
pred_B = self.clf.predict(imgs_B)
test_acc = np.mean(np.argmax(pred_B, axis=1) == labels_B)
# Add accuracy to list of last 100 accuracy measurements
test_accs.append(test_acc)
if len(test_accs) > 100:
test_accs.pop(0)
# Plot the progress
print ( "%d : [D - loss: %.5f, acc: %3d%%], [G - loss: %.5f], [clf - loss: %.5f, acc: %3d%%, test_acc: %3d%% (%3d%%)]" % \
(epoch, d_loss[0], 100*float(d_loss[1]),
g_loss[1], g_loss[2], 100*float(g_loss[-1]),
100*float(test_acc), 100*float(np.mean(test_accs))))
# If at save interval => save generated image samples
if epoch % sample_interval == 0:
self.sample_images(epoch)
def sample_images(self, epoch):
r, c = 2, 5
imgs_A, _ = self.data_loader.load_data(domain="A", batch_size=5)
# Translate images to the other domain
fake_B = self.generator.predict(imgs_A)
gen_imgs = np.concatenate([imgs_A, fake_B])
# Rescale images 0 - 1
gen_imgs = 0.5 * gen_imgs + 0.5
#titles = ['Original', 'Translated']
fig, axs = plt.subplots(r, c)
cnt = 0
for i in range(r):
for j in range(c):
axs[i,j].imshow(gen_imgs[cnt])
#axs[i, j].set_title(titles[i])
axs[i,j].axis('off')
cnt += 1
fig.savefig("images/%d.png" % (epoch))
plt.close()
if __name__ == '__main__':
gan = PixelDA()
gan.train(epochs=3000, batch_size=32, sample_interval=500)
test.py
from __future__ import print_function, division
import scipy
import datetime
import matplotlib.pyplot as plt
import sys
from data_loader import DataLoader
import numpy as np
import os
# Configure MNIST and MNIST-M data loader
data_loader = DataLoader(img_res=(32, 32))
mnist, _ = data_loader.load_data(domain="A", batch_size=25)
mnistm, _ = data_loader.load_data(domain="B", batch_size=25)
r, c = 5, 5
for img_i, imgs in enumerate([mnist, mnistm]):
#titles = ['Original', 'Translated']
fig, axs = plt.subplots(r, c)
cnt = 0
for i in range(r):
for j in range(c):
axs[i,j].imshow(imgs[cnt])
#axs[i, j].set_title(titles[i])
axs[i,j].axis('off')
cnt += 1
fig.savefig("%d.png" % (img_i))
plt.close()
3.18 Wasserstein GAN
代码及运行结果
wgan.py
from __future__ import print_function, division
from tensorflow.keras.datasets import mnist
from tensorflow.keras.layers import Input, Dense, Reshape, Flatten, Dropout
from tensorflow.keras.layers import BatchNormalization, Activation, ZeroPadding2D
from tensorflow.keras.layers import LeakyReLU
from tensorflow.keras.layers import UpSampling2D, Conv2D
from tensorflow.keras.models import Sequential, Model
from tensorflow.keras.optimizers import RMSprop
import tensorflow.keras.backend as K
import matplotlib.pyplot as plt
import sys
import numpy as np
class WGAN():
def __init__(self):
self.img_rows = 28
self.img_cols = 28
self.channels = 1
self.img_shape = (self.img_rows, self.img_cols, self.channels)
self.latent_dim = 100
# Following parameter and optimizer set as recommended in paper
self.n_critic = 5
self.clip_value = 0.01
optimizer = RMSprop(lr=0.00005)
# Build and compile the critic
self.critic = self.build_critic()
self.critic.compile(loss=self.wasserstein_loss,
optimizer=optimizer,
metrics=['accuracy'])
# Build the generator
self.generator = self.build_generator()
# The generator takes noise as input and generated imgs
z = Input(shape=(self.latent_dim,))
img = self.generator(z)
# For the combined model we will only train the generator
self.critic.trainable = False
# The critic takes generated images as input and determines validity
valid = self.critic(img)
# The combined model (stacked generator and critic)
self.combined = Model(z, valid)
self.combined.compile(loss=self.wasserstein_loss,
optimizer=optimizer,
metrics=['accuracy'])
def wasserstein_loss(self, y_true, y_pred):
return K.mean(y_true * y_pred)
def build_generator(self):
model = Sequential()
model.add(Dense(128 * 7 * 7, activation="relu", input_dim=self.latent_dim))
model.add(Reshape((7, 7, 128)))
model.add(UpSampling2D())
model.add(Conv2D(128, kernel_size=4, padding="same"))
model.add(BatchNormalization(momentum=0.8))
model.add(Activation("relu"))
model.add(UpSampling2D())
model.add(Conv2D(64, kernel_size=4, padding="same"))
model.add(BatchNormalization(momentum=0.8))
model.add(Activation("relu"))
model.add(Conv2D(self.channels, kernel_size=4, padding="same"))
model.add(Activation("tanh"))
model.summary()
noise = Input(shape=(self.latent_dim,))
img = model(noise)
return Model(noise, img)
def build_critic(self):
model = Sequential()
model.add(Conv2D(16, kernel_size=3, strides=2, input_shape=self.img_shape, padding="same"))
model.add(LeakyReLU(alpha=0.2))
model.add(Dropout(0.25))
model.add(Conv2D(32, kernel_size=3, strides=2, padding="same"))
model.add(ZeroPadding2D(padding=((0,1),(0,1))))
model.add(BatchNormalization(momentum=0.8))
model.add(LeakyReLU(alpha=0.2))
model.add(Dropout(0.25))
model.add(Conv2D(64, kernel_size=3, strides=2, padding="same"))
model.add(BatchNormalization(momentum=0.8))
model.add(LeakyReLU(alpha=0.2))
model.add(Dropout(0.25))
model.add(Conv2D(128, kernel_size=3, strides=1, padding="same"))
model.add(BatchNormalization(momentum=0.8))
model.add(LeakyReLU(alpha=0.2))
model.add(Dropout(0.25))
model.add(Flatten())
model.add(Dense(1))
model.summary()
img = Input(shape=self.img_shape)
validity = model(img)
return Model(img, validity)
def train(self, epochs, batch_size=128, sample_interval=50):
# Load the dataset
(X_train, _), (_, _) = mnist.load_data()
# Rescale -1 to 1
X_train = (X_train.astype(np.float32) - 127.5) / 127.5
X_train = np.expand_dims(X_train, axis=3)
# Adversarial ground truths
valid = -np.ones((batch_size, 1))
fake = np.ones((batch_size, 1))
for epoch in range(epochs):
for _ in range(self.n_critic):
# ---------------------
# Train Discriminator
# ---------------------
# Select a random batch of images
idx = np.random.randint(0, X_train.shape[0], batch_size)
imgs = X_train[idx]
# Sample noise as generator input
noise = np.random.normal(0, 1, (batch_size, self.latent_dim))
# Generate a batch of new images
gen_imgs = self.generator.predict(noise)
# Train the critic
d_loss_real = self.critic.train_on_batch(imgs, valid)
d_loss_fake = self.critic.train_on_batch(gen_imgs, fake)
d_loss = 0.5 * np.add(d_loss_fake, d_loss_real)
# Clip critic weights
for l in self.critic.layers:
weights = l.get_weights()
weights = [np.clip(w, -self.clip_value, self.clip_value) for w in weights]
l.set_weights(weights)
# ---------------------
# Train Generator
# ---------------------
g_loss = self.combined.train_on_batch(noise, valid)
# Plot the progress
print ("%d [D loss: %f] [G loss: %f]" % (epoch, 1 - d_loss[0], 1 - g_loss[0]))
# If at save interval => save generated image samples
if epoch % sample_interval == 0:
self.sample_images(epoch)
def sample_images(self, epoch):
r, c = 5, 5
noise = np.random.normal(0, 1, (r * c, self.latent_dim))
gen_imgs = self.generator.predict(noise)
# Rescale images 0 - 1
gen_imgs = 0.5 * gen_imgs + 0.5
fig, axs = plt.subplots(r, c)
cnt = 0
for i in range(r):
for j in range(c):
axs[i,j].imshow(gen_imgs[cnt, :,:,0], cmap='gray')
axs[i,j].axis('off')
cnt += 1
fig.savefig("images/mnist_%d.png" % epoch)
plt.close()
if __name__ == '__main__':
wgan = WGAN()
wgan.train(epochs=1000, batch_size=32, sample_interval=50)
4、应用总结
总的来说,生成对抗网络GAN
在风格迁移、动画生成、图像超分辨率重建、图像修复、目标检测、人脸合成等方面展示出巨大的潜力和应用,学者们对其进行了众多的改进和提升,Keras、TensorFlow和PyTorch官网上都带有很多GAN
的示例代码,研究人员、开发者和学习爱好者都可以进行学习测试和研究,感受深度学习的魅力,不得不说,在大量的数据集训练基础上得到的模型参数,深度网络在捕捉深层抽象特征的能力确实令人不可思议,效果在一定程度上出奇制胜。