json_to_txt
txt格式
环境
代码
转后路径
结果
txt格式
训练tensorflow版的yolov3需要的数据格式是txt的,具体要求如下
xxx/xxx.jpg 18.19,6.32,424.13,421.83,20 323.86,2.65,640.0,421.94,20
xxx/xxx.jpg 48,240,195,371,11 8,12,352,498,14
image_path x_min, y_min, x_max, y_max, class_id x_min, y_min ,…, class_id
make sure that x_max < width and y_max < height
针对tensorflow-yolov3,它的class_id 是从0开始到类别数-1. 而我自己的数据集的category_id部分是1,2,3,4,8,9,10
所以我还在代码中添加boundingBox所属类别部分 做了如下修改
id = boundingBox_per['category_id']
if(id in [8,9,10]):
id = id-4
else:
id = id-1
然后class.name 部分为1,2,3,4,8,9,10
环境
需要的环境是 python3
需要修改的地方:第10.11.12行
filename = 'instances_val.json'#修改成你的json文件名字
jsondir = '/home/chenbolin/data2/six/annotations/instances_val.json'#修改成你的json文件路径
img_root = '/home/chenbolin/data2/six/val/' #修改成你的图片路径f = open(jsondir,encoding='utf-8')
代码
我们的数据集是coco格式的,也就是要把json文件转成txt格式的,代码如下:
# coding=utf-8import json
import numpy as np
from xml.etree.ElementTree import Element, SubElement, tostring
from xml.dom.minidom import parseString
import os
# -----------------
# txt数据格式:path,xmin,ymin,xmax,ymax
# 每一行表示一个image
# --------------------
filename = 'instances_val.json'#修改成你的json文件名字
jsondir = '/home/chenbolin/data2/six/annotations/instances_val.json'#修改成你的json文件路径
img_root = '/home/chenbolin/data2/six/val/' #修改成你的图片路径f = open(jsondir,encoding='utf-8')
res = f.read()
data = json.loads(res)
# 保存数据的文件夹
folder = filename.split('.')[0]+'_txt'
if not os.path.exists(folder):
os.mkdir(folder)
# 首先得到数据的categories的关键字
category = data['categories']
category_id ={}
for category_per in category:
id = category_per['id']
cls = category_per['name']
category_id[id] = cls
print(category_id)
# 开始遍历字典,对每一个图像生成xml文件
imageID_all =[]
imageID_all_info = {}
for images_attr in list(data.keys()):
if images_attr == 'images':
# 遍历每一个图像
for data_per in data[images_attr]:
# 获取图像名字
image_name = data_per['file_name']
# 获取图像路径
#image_route = data_per['coco_url']
image_route = img_root+image_name
# 获取图像的像素和ID
image_width = data_per['width']
image_height = data_per['height']
image_id = data_per['id']
imageID_all.append(image_id)
imageID_all_info[image_id]={'width':image_width,'height':image_height,'path':image_route,'filename':image_name}
elif images_attr == 'annotations':
# 根据id遍历每张图像的bounding box
for imageID_per in imageID_all:
print(imageID_per)
# 根据图像ID,构建图像基本信息子目录
# 图像路径
image_path = imageID_all_info[imageID_per]['path']
# 每一张图片信息写在txt文件
# filename1 = imageID_all_info[imageID_per]['filename'].split('.')[0]
file_write = folder + '/' + filename.split('.')[0] + '.txt'
# 图像包含了多少个bounding box
boundingBox_image = [j for j in data[images_attr] if j['image_id']==imageID_per]
boundingBox_cord =''
path_cord =''
if len(boundingBox_image)==0:
path_cord = image_path +'/n'
with open(file_write, 'a+') as f:
f.write(path_cord)
continue
# 输出每张boundging box的坐标信息,以及所属类信息
for boundingBox_per in boundingBox_image:
# 添加boundingBox所属类的id
id = boundingBox_per['category_id']
# 位置信息转换,x,y,w,h转为xmin,ymin,xmax,ymax
x = boundingBox_per['bbox'][0]
y = boundingBox_per['bbox'][1]
w = boundingBox_per['bbox'][2]
h = boundingBox_per['bbox'][3]
xmin = str(x+1)
ymin = str(y+1)
xmax= str(round(x+w,2))
ymax=str(round(y+h,2))
boundingBox_cord += xmin +','+ymin+','+xmax+','+ymax+','+str(id)+' '
boundingBox_cord = boundingBox_cord.rstrip()
boundingBox_cord += '\n'
path_cord = image_path + ' '+boundingBox_cord
with open(file_write, 'a+') as f:
f.write(path_cord)
转后路径
-
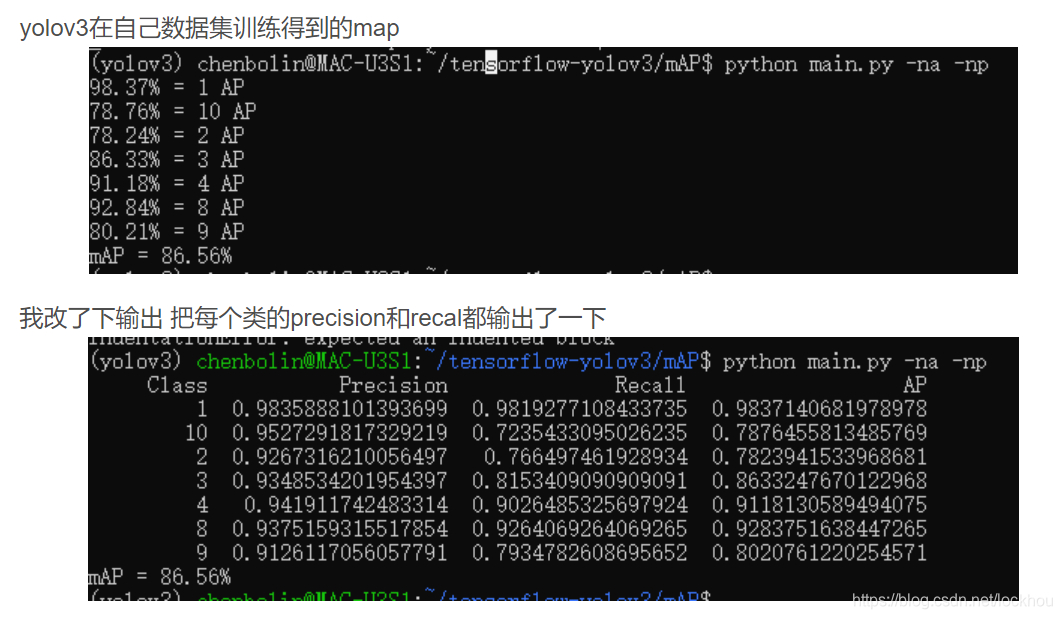