import numpy as np
import pandas as pd
class QLearningTable:
def __init__(self, actions, learning_rate=0.01, reward_decay=0.9, e_greedy=0.9):
self.actions = actions
self.lr = learning_rate
self.gamma = reward_decay
self.epsilon = e_greedy
self.q_table = pd.DataFrame(columns=self.actions, dtype=np.float64)
def choose_action(self, observation):
self.check_state_exist(observation)
if np.random.uniform() < self.epsilon:
state_action = self.q_table.loc[observation,:]
action = np.random.choice(state_action[state_action==np.max(state_action)].index)
else:
action = np.random.choice(self.actions)
return action
def learn(self, s, a, r, s_):
self.check_state_exist(s_)
q_predict = self.q_table.loc[s, a]
if s_ != 'terminal':
q_target = r + self.gamma*self.q_table.loc[s_, :].max()
else:
q_target = r
self.q_table.loc[s, a] += self.lr*(q_target-q_predict)
def check_state_exist(self, state):
if state not in self.q_table.index:
self.q_table = self.q_table.append(pd.Series([0]*len(self.actions),index=self.q_table.columns,name=state))
强化学习之 Qlearning
最新推荐文章于 2024-08-15 01:55:01 发布
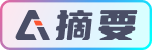