3. FAST / BRIEF/ ORB
3.1 FAST Algorithm for Corner Detection
Theory
Many feature detectors are really good, but not fast enough.
As a solution, FAST (Features from Accelerated Segment Test) algorithm was proposed by Edward Rosten and Tom Drummond in their paper “Machine learning for high-speed corner detection” in 2006 (Later revised it in 2010).
Feature Detection using FAST
(1) Select a pixel p p p in the image which is to be identified as an interest point or not. Let its intensity be I p I_p Ip.
(2) Select appropriate threshold value t t t.
(3) Consider a circle of 16 pixels around the pixel under test. (See the image below)
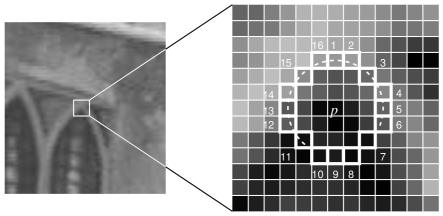
(4) Now the pixel p p p is a corner if there exists a set of n n n contiguous pixels in the circle (of 16 pixels), which are all brighter than I p + t I_p + t Ip+t or all darker than I p − t I_p − t Ip−t.
(Shown as white dash lines in the above image). n n n was chosen to be 12.
(5) A high-speed test was proposed to exclude a large number of non-corners.
This test examines only the four pixels at 1, 9, 5 and 13 (First 1 and 9 are tested if they are too brighter or darker. If so, then checks 5 and 13).
If p p p is a corner, then at least three of these must all be brighter than I p + t I_p + t Ip+t or darker than I p − t I_p − t Ip−t, else p cannot be a corner.
The full segment test criterion can then be applied to the passed candidates by examining all pixels in the circle.
This detector in itself exhibits high performance, but there are several weaknesses:
-
It does not reject as many candidates for n < 12.
-
The choice of pixels is not optimal because its efficiency depends on ordering of the questions and distribution of corner appearances.
-
Results of high-speed tests are thrown away.
-
Multiple features are detected adjacent to one another.
-
Q: first point means it could be faster?
-
Q: in the second point, what about the corner appearances?
First 3 points are addressed with a machine learning approach.
Last one is addressed using non-maximal suppression.
Machine Learning a Corner Detector
(1) Select a set of images for training (preferably from the target application domain)
(2) Run FAST algorithm in every images to find feature points.
(3) For every feature point, store the 16 pixels around it as a vector. Do it for all the images to get feature vector P P P.
(4) Each pixel (say x) in these 16 pixels can have one of the following three states:
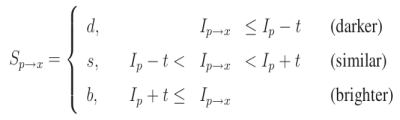
(5) Depending on these states, the feature vector P P P is subdivided into 3 subsets, P d P_d Pd, P s P_s Ps, P b P_b Pb.
(6) Define a new boolean variable, K p K_p Kp, which is true if p p p is a corner and false otherwise.
(7) Use the ID3 algorithm (decision tree classifier) to query each subset using the variable K p K_p Kp for the knowledge about the true class. It selects the x which yields the most information about whether the candidate pixel is a corner, measured by the entropy of K p K_p Kp.
(8) This is recursively applied to all the subsets until its entropy is zero.
(9) The decision tree so created is used for fast detection in other images.
Non-maximal Suppression
Detecting multiple interest points in adjacent locations is another problem.
It is solved by using Non-maximum Suppression.
(1) Compute a score function, V V V for all the detected feature points. V V V is the sum of absolute difference between p p p and 16 surrounding pixels values.
(2) Consider two adjacent keypoints and compute their V V V values.
(3) Discard the one with lower V V V value.
Summary
It is several times faster than other existing corner detectors.
But it is not robust to high levels of noise. It is dependant on a threshold.
3.2 BRIEF (Binary Robust Independent Elementary Features)
Theory
Creating a vector for thousands of features takes a lot of memory which are not feasible for resouce-constraint applications especially for embedded systems.
Larger the memory, longer the time it takes for matching.
But all these dimensions may not be needed for actual matching.
We can compress it using several methods like PCA, LDA, LSH (Locality Sensitive Hashing) to convert descriptors in floating point numbers to binary strings.
These binary strings are used to match features using Hamming distance, which provides better speed-up because finding hamming distance is just applying XOR and bit count, which are very fast in modern CPUs with SSE instructions.
But here, we need to find the descriptors first, then only we can apply hashing, which doesn’t solve our initial problem on memory.
BRIEF provides a shortcut to find the binary strings directly without finding descriptors.
It takes smoothened image patch and selects a set of n d n_d nd (x,y) location pairs in an unique way. Then some pixel intensity comparisons are done on these location pairs.
For eg, let first location pairs be p p p and q q q. If I ( p ) < I ( q ) I(p) < I(q) I(p)<I(q), then its result is 1, else it is 0. This is applied for all the n d n_d nd location pairs to get a n d n_d nd-dimensional bitstring.
This n d n_d nd can be 128, 256 or 512. OpenCV supports all of these, but by default, it would be 256 (OpenCV represents it in bytes. So the values will be 16, 32 and 64).
So once you get this, you can use Hamming Distance to match these descriptors.
One important point is that BRIEF is a feature descriptor, it doesn’t provide any method to find the features.
So you will have to use any other feature detectors like SIFT, SURF etc.
The paper recommends to use CenSurE which is a fast detector and BRIEF works even slightly better.
In short, BRIEF is a faster method feature descriptor calculation and matching.
It also provides high recognition rate unless there is large in-plane rotation.
3.3 ORB (Oriented FAST and Rotated BRIEF)
Theory
ORB is basically a fusion of FAST keypoint detector and BRIEF descriptor with many modifications to enhance the performance.
First it use FAST to find keypoints, then apply Harris corner measure to find top N points among them. It also use pyramid to produce multiscale-features.
But one problem is that, FAST doesn’t compute the orientation.
So what about rotation invariance? Authors came up with following modification.
It computes the intensity weighted centroid of the patch with located corner at center. The direction of the vector from this corner point to centroid gives the orientation.
To improve the rotation invariance, moments are computed with x and y which should be in a circular region of radius r r r, where r r r is the size of the patch.
Now for descriptors, ORB use BRIEF descriptors. But BRIEF performs poorly with rotation.
So what ORB does is to “steer” BRIEF according to the orientation of keypoints.
For any feature set of n n n binary tests at location ( x i , y i ) (x_i, y_i) (xi,yi), define a 2 × n 2 \times n 2×n matrix, S S S which contains the coordinates of these pixels. Then using the orientation of patch, θ \theta θ, its rotation matrix is found and rotates the S S S to get steered(rotated) version S θ S_\theta Sθ.
ORB discretize the angle to increments of 2 π / 30 2 \pi /30 2π/30 (12 degrees), and construct a lookup table of precomputed BRIEF patterns. As long as the keypoint orientation θ \theta θ is consistent across views, the correct set of points S θ S_\theta Sθ will be used to compute its descriptor.
BRIEF has an important property that each bit feature has a large variance and a mean near 0.5.
But once it is oriented along keypoint direction, it loses this property and become more distributed. High variance makes a feature more discriminative, since it responds differentially to inputs.
Another desirable property is to have the tests uncorrelated, since then each test will contribute to the result.
To resolve all these, ORB runs a greedy search among all possible binary tests to find the ones that have both high variance and means close to 0.5, as well as being uncorrelated. The result is called rBRIEF.
For descriptor matching, multi-probe LSH which improves on the traditional LSH, is used.
The paper says ORB is much faster than SURF and SIFT and ORB descriptor works better than SURF.
ORB is a good choice in low-power devices for panorama stitching etc.
References
FAST Algorithm for Corner Detection
BRIEF (Binary Robust Independent Elementary Features)
ORB (Oriented FAST and Rotated BRIEF)