12os.chdir(‘F:\python_study\pachong\工作细胞’)
13def gethtml(url):
14
15 browser = webdriver.PhantomJS()
16 browser.get(url)
17 browser.implicitly_wait(10)
18 return(browser)
19
20def getComment(url):
21
22 browser = gethtml(url)
23 i = 1
24 AllArticle = pd.DataFrame(columns = [‘id’,‘author’,‘comment’,‘stars1’,‘stars2’,‘stars3’,‘stars4’,‘stars5’,‘unlike’,‘like’])
25 print(‘连接成功,开始爬取数据’)
26 while True:
27
28 xpath1 = ‘//*[@id=“app”]/div[2]/div[2]/div/div[1]/div/div/div[4]/div/div/ul/li[{}]’.format(i)
29 try:
30 target = browser.find_element_by_xpath(xpath1)
31 except:
32 print(‘全部爬完’)
33 break
34
35 author = target.find_element_by_xpath(‘div[1]/div[2]’).text
36 comment = target.find_element_by_xpath(‘div[2]/div’).text
37 stars1 = target.find_element_by_xpath(‘div[1]/div[3]/span/i[1]’).get_attribute(‘class’)
38 stars2 = target.find_element_by_xpath(‘div[1]/div[3]/span/i[2]’).get_attribute(‘class’)
39 stars3 = target.find_element_by_xpath(‘div[1]/div[3]/span/i[3]’).get_attribute(‘class’)
40 stars4 = target.find_element_by_xpath(‘div[1]/div[3]/span/i[4]’).get_attribute(‘class’)
41 stars5 = target.find_element_by_xpath(‘div[1]/div[3]/span/i[5]’).get_attribute(‘class’)
42 date = target.find_element_by_xpath(‘div[1]/div[4]’).text
43 like = target.find_element_by_xpath(‘div[3]/div[1]’).text
44 unlike = target.find_element_by_xpath(‘div[3]/div[2]’).text
45
46
47 comments = pd.DataFrame([i,author,comment,stars1,stars2,stars3,stars4,stars5,like,unlike]).T
48 comments.columns = [‘id’,‘author’,‘comment’,‘stars1’,‘stars2’,‘stars3’,‘stars4’,‘stars5’,‘unlike’,‘like’]
49 AllArticle = pd.concat([AllArticle,comments],axis = 0)
50 browser.execute_script(“arguments[0].scrollIntoView();”, target)
51 i = i + 1
52 if i%100 == 0:
53 print(‘已爬取{}条’.format(i))
54 AllArticle = AllArticle.reset_index(drop = True)
55 return AllArticle
56
57url = ‘https://www.bilibili.com/bangumi/media/md102392/?from=search&seid=8935536260089373525#short’
58result = getComment(url)
59#result.to_csv(‘工作细胞爬虫.csv’,index = False)
这种方法爬取失败之后,一直不知道该怎么处理,刚好最近看到网上有大神爬猫眼评论的文章,照葫芦画瓢尝试了一下,居然成功了,而且爬的速度也很快,十来分钟就全爬完了,思路是找到评论对应的Json文件,然后获取Json中的数据,过程如下。
在Google浏览器中按**F12**打开卡发者工具后,选择**Network**
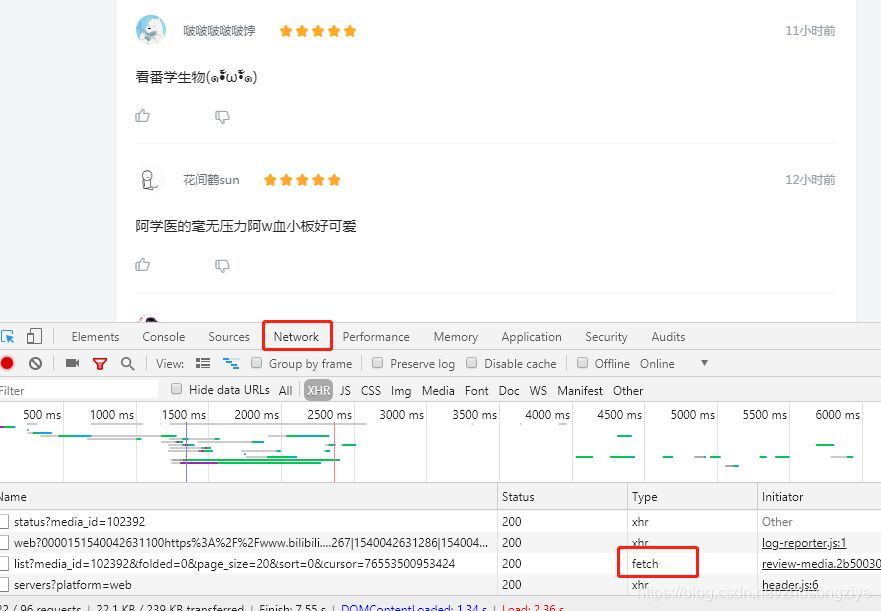
往下滑动,会发现过一段时间,会出现一个**fetch**,右键打开后发现,里面就是20条记录,有所有我们需要的内容,json格式。
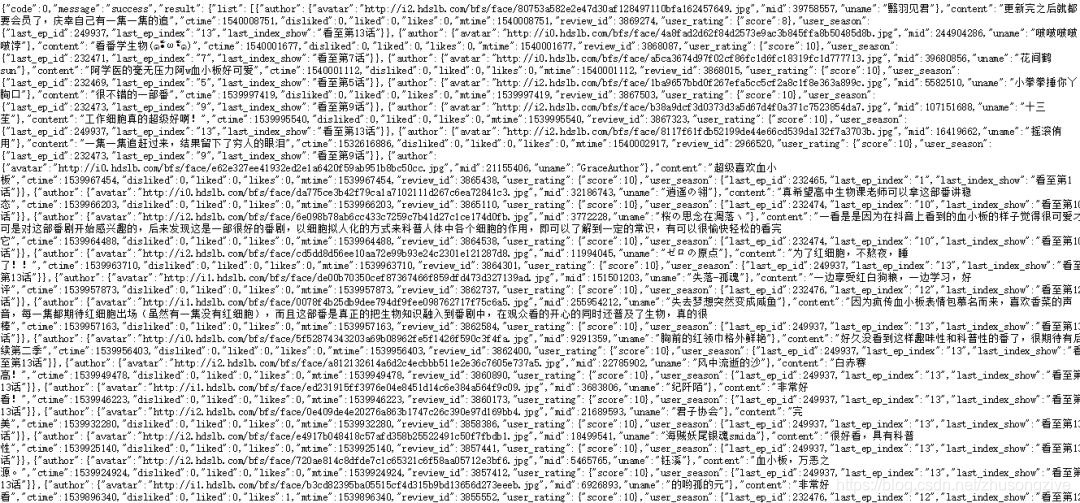
所以现在需要做的就是去找这些json文件的路径的规律。多看几条之后,就发现了规律:
第一个json:
**https://bangumi.bilibili.com/review/web\_api/short/list?****media\_id=102392&folded=0&page\_size=20&sort=0**
第二个json:
**https://bangumi.bilibili.com/review/web\_api/short/list?media\_id=102392&folded=0&page\_size=20&sort=0&cursor=76553500953424**
第三个json:
**https://bangumi.bilibili.com/review/web\_api/short/list?media\_id=102392&folded=0&page\_size=20&sort=0&cursor=76549205971454**
显然所有的json路径的前半部分都是一样,都是在第一条json之后加上不同的cursor = xxxxx,所以只要能找到**cursor**值的规律,就可以用循环的办法,爬完所有的json,这个值看上去没什么规律,最后发现,每一个json路径中cursor值就藏在前一个json的最后一条评论中

在python中可以直接把json转成字典,cursor值就是最后一条评论中键cursor的值,简直不要太容易。
所以爬的思路就很清晰了,从一个json开始,爬完20条评论后,获取最后一个评论中的cursor值,更改路径之后获取第二个json,重复上面的过程,直到爬完所有的json。
至于如何知道爬完了所有json,也很容易,每个json中一个**total键**,表示了当前一共有多少条评论,所以只需要写一个while循环,当爬到的评论数达到total值时停止。
爬的过程中还发现,有些json中的评论数不够20条,如果每次用20去定位,中间会报错停止,需要注意一下。所以又加了一行代码,每次获得json后,通过**len()**函数得到当前json中一共包含多少条评论,cursor在最后一个评论中。
以上是整个爬的思路,我们最终爬到以下信息
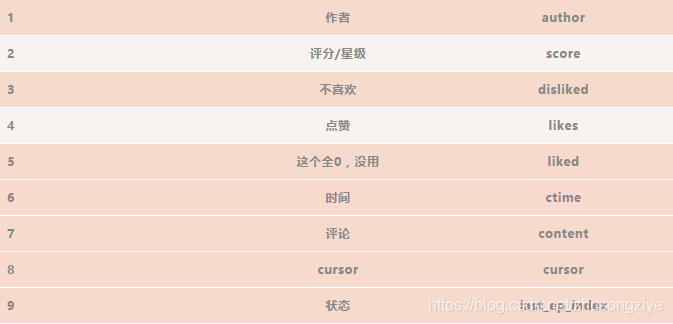
需要说明的地方,一个是**liked**按照字面意思应该是用户的点赞数,但爬完才发现全是0,没有用。另一个是关于时间,里面有**ctime**和**mtime**两个跟时间有关的值,看了几个,基本都是一样的,有个别不太一样,差的不多,就只取了ctime,我猜可能一个是点击进去的时间,一个是评论提交时间,但没法验证,就随便取一个算了,ctime的编码很奇怪,比如某一个是ctime = 1540001677,渣渣之前没有见过这种编码方式,请教了大佬之后知道,这个是Linux系统上的时间表示方式,是1970年1月1日0时0分0秒到当时时点的秒数,python中可以直接用**time.gmtime()**函数转化成年月日小时分钟秒的格式。还有**last\_ep\_index**里面存的是用户当前的看剧状态,比如看至第13话,第6话之类的,但后来发现很不准,绝大多数用户没有last\_ep\_index值,所以也没有分析这个变量。
代码如下
1import requests
2from fake_useragent import UserAgent
3import json
4import pandas as pd
5import time
6import datetime
7headers = { “User-Agent”: UserAgent(verify_ssl=False).random}
8comment_api = ‘https://bangumi.bilibili.com/review/web_api/short/list?media_id=102392&folded=0&page_size=20&sort=0’
9
10# 发送get请求
11response_comment = requests.get(comment_api,headers = headers)
12json_comment = response_comment.text
13json_comment = json.loads(json_comment)
14
15total = json_comment[‘result’][‘total’]
16
17cols = [‘author’,‘score’,‘disliked’,‘likes’,‘liked’,‘ctime’,‘score’,‘content’,‘last_ep_index’,‘cursor’]
18dataall = pd.DataFrame(index = range(total),columns = cols)
19
20
21j = 0
22while j <total:
23 n = len(json_comment[‘result’][‘list’])
24 for i in range(n):
25 dataall.loc[j,‘author’] = json_comment[‘result’][‘list’][i][‘author’][‘uname’]
26 dataall.loc[j,‘score’] = json_comment[‘result’][‘list’][i][‘user_rating’][‘score’]
27 dataall.loc[j,‘disliked’] = json_comment[‘result’][‘list’][i][‘disliked’]
28 dataall.loc[j,‘likes’] = json_comment[‘result’][‘list’][i][‘likes’]
29 dataall.loc[j,‘liked’] = json_comment[‘result’][‘list’][i][‘liked’]
30 dataall.loc[j,‘ctime’] = json_comment[‘result’][‘list’][i][‘ctime’]
31 dataall.loc[j,‘content’] = json_comment[‘result’][‘list’][i][‘content’]
32 dataall.loc[j,‘cursor’] = json_comment[‘result’][‘list’][n-1][‘cursor’]
33 j+= 1
34 try:
35 dataall.loc[j,‘last_ep_index’] = json_comment[‘result’][‘list’][i][‘user_season’][‘last_ep_index’]
36 except:
37 pass
38
39 comment_api1 = comment_api + ‘&cursor=’ + dataall.loc[j-1,‘cursor’]
40 response_comment = requests.get(comment_api1,headers = headers)
41 json_comment = response_comment.text
42 json_comment = json.loads(json_comment)
43
44 if j % 50 ==0:
45 print(‘已完成 {}% !’.format(round(j/total*100,2)))
46 time.sleep(0.5)
47
48
49
50dataall = dataall.fillna(0)
51
52def getDate(x):
53 x = time.gmtime(x)
54 return(pd.Timestamp(datetime.datetime(x[0],x[1],x[2],x[3],x[4],x[5])))
55
56dataall[‘date’] = dataall.ctime.apply(lambda x:getDate(x))
57
58dataall.to_csv(‘bilibilib_gongzuoxibao.xlsx’,index = False)
**03 影评分析**
最终一共爬到了**17398**条影评数据。里面的date是用ctime转过来的,接下来对数据进行一些分析,数据分析通过python3.6完成,代码见文末。
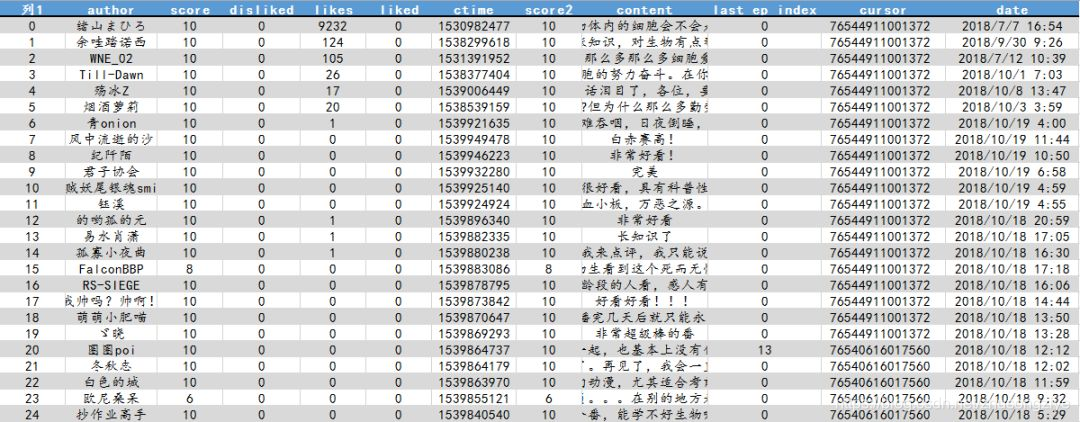
评分分布
评分取值范围为2、4、6、8、10分,对应1-5颗星
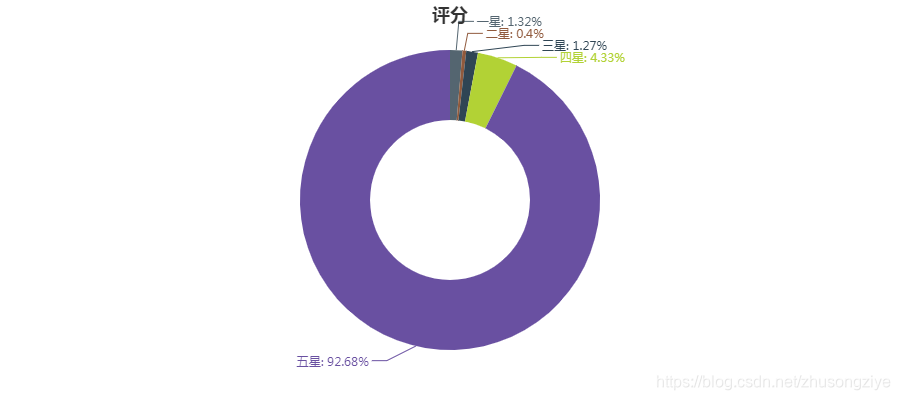
可以看出,几乎所有的用户都给了这部动漫五星好评,影响力可见一斑。
评分时间分布
将这部动漫从上线至今所有的评分按日进行平均,观察评分随时间的变化情况
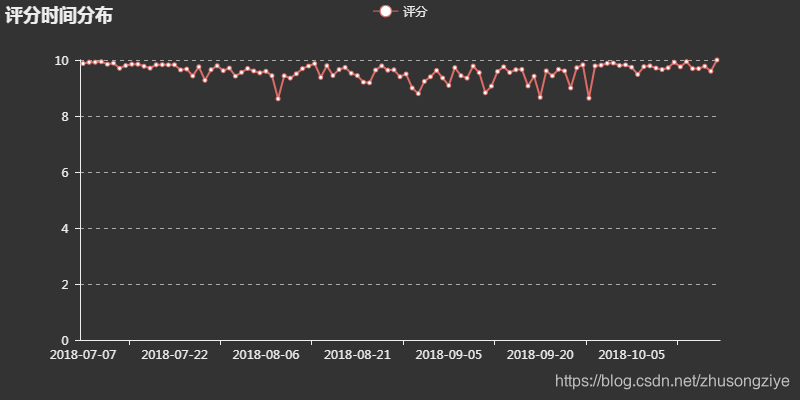
可以看出,评分一直居高不下,尤其起始和结束时都接近满分,足见这是一部良好开端、圆满结束的良心作品。
每日评论数
看完评分之后,再看看评论相关的数据,我最感兴趣的是,这些评论的时间分布是怎么样的,统计了每一日的评论数之后,得到了评论数的分布图
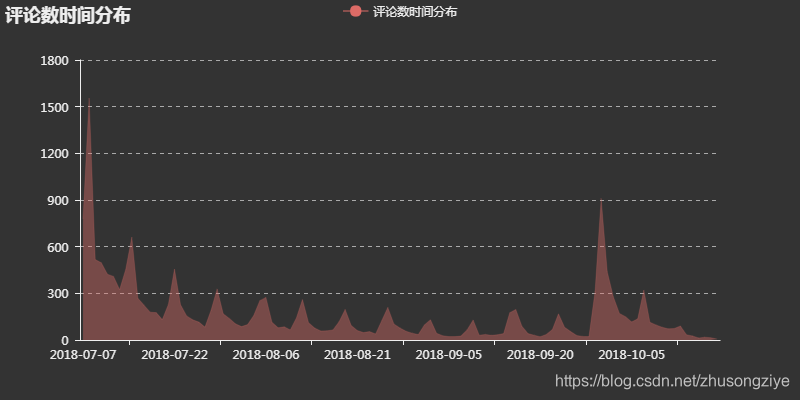
基本上是每出了新的一话,大家看完后就会在短评中分享自己的感受,当然同样是起始和结束阶段的评论数最多,对比同期的百度指数
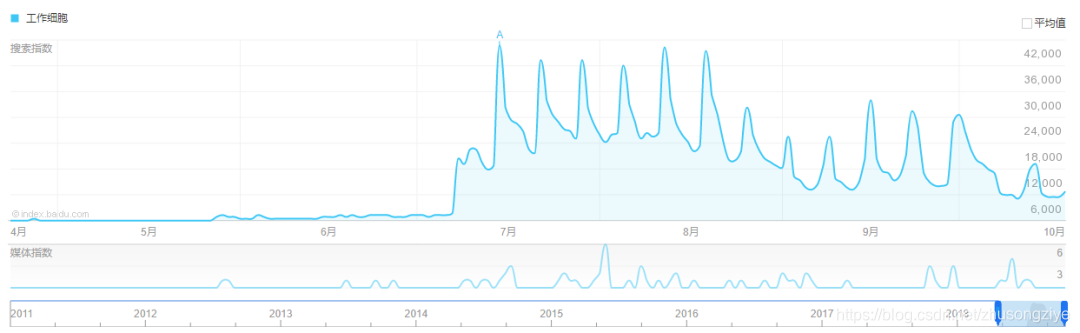
评论日内分布
除了每日的评论数,也想分析一下评论的日内趋势,用户都喜欢在每日的什么时间进行评论?将评论分24个小时求和汇总后,得到了下图
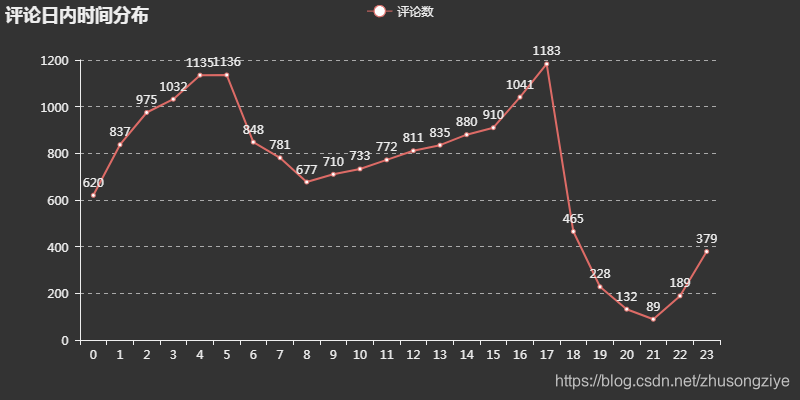
不过这个结果就不是很理想了,横轴是时间,纵轴是评论数,中午到下午的趋势上升可以理解,晚上七八点没有人评论反倒是凌晨三四点评论数最多,这个就很反常了,可能是评论在系统中上线的时间有一定偏差?
好评字数
### 最后
> **🍅 硬核资料**:关注即可领取PPT模板、简历模板、行业经典书籍PDF。
> **🍅 技术互助**:技术群大佬指点迷津,你的问题可能不是问题,求资源在群里喊一声。
> **🍅 面试题库**:由技术群里的小伙伴们共同投稿,热乎的大厂面试真题,持续更新中。
> **🍅 知识体系**:含编程语言、算法、大数据生态圈组件(Mysql、Hive、Spark、Flink)、数据仓库、Python、前端等等。
**网上学习资料一大堆,但如果学到的知识不成体系,遇到问题时只是浅尝辄止,不再深入研究,那么很难做到真正的技术提升。**
**[需要这份系统化学习资料的朋友,可以戳这里无偿获取](https://bbs.csdn.net/topics/618317507)**
**一个人可以走的很快,但一群人才能走的更远!不论你是正从事IT行业的老鸟或是对IT行业感兴趣的新人,都欢迎加入我们的的圈子(技术交流、学习资源、职场吐槽、大厂内推、面试辅导),让我们一起学习成长!**