决策树CART
原理
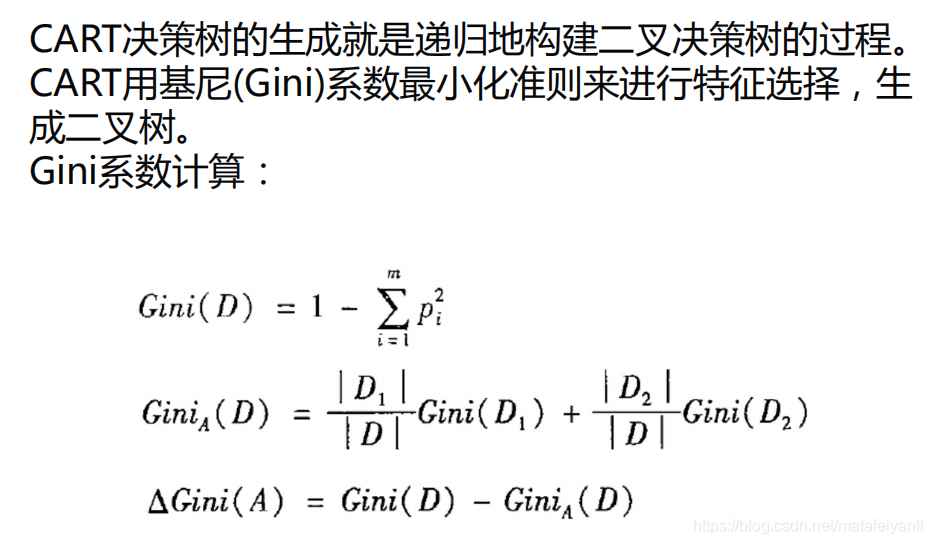
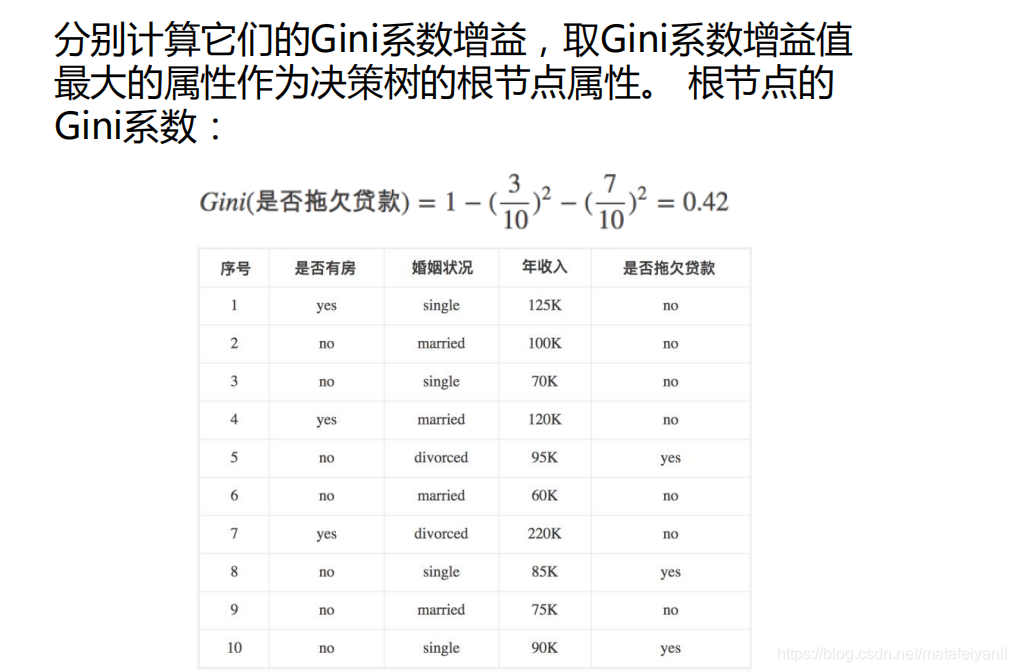
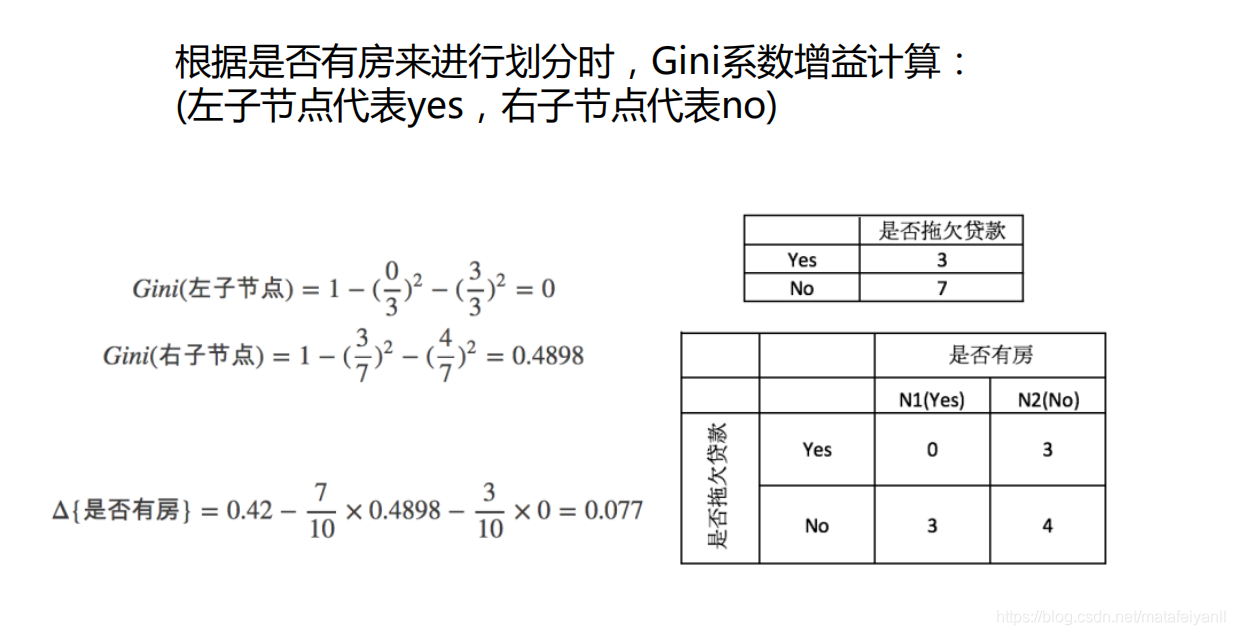
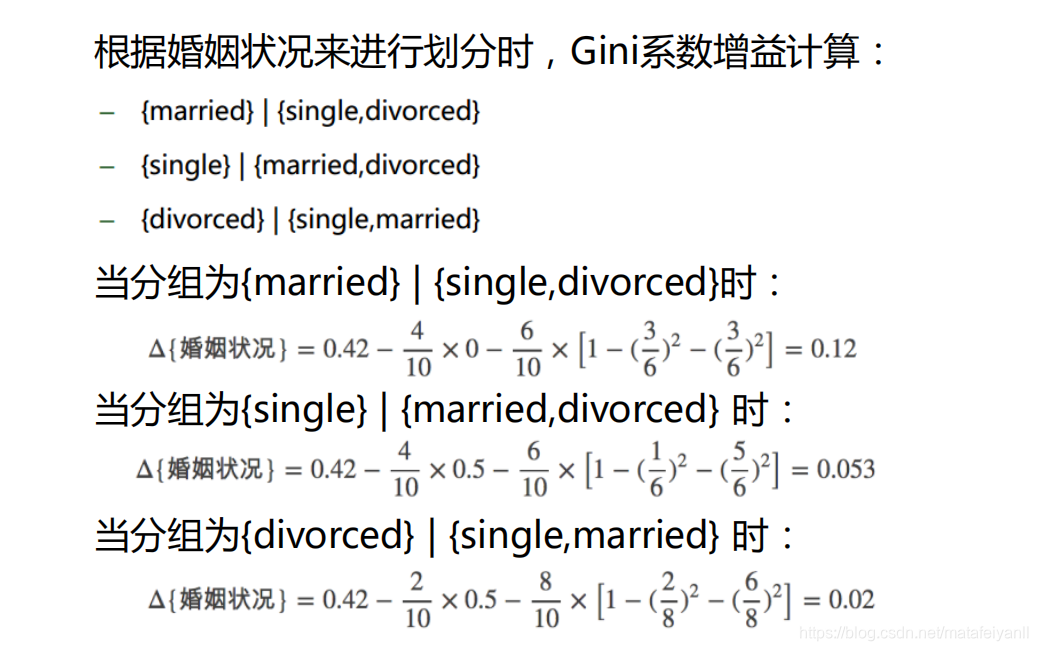
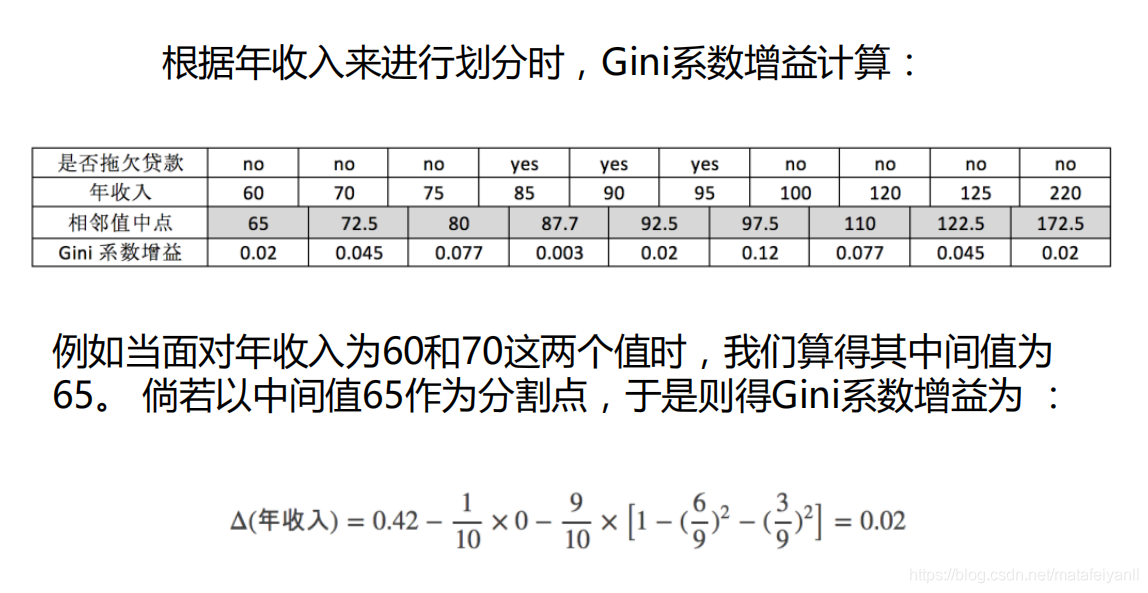
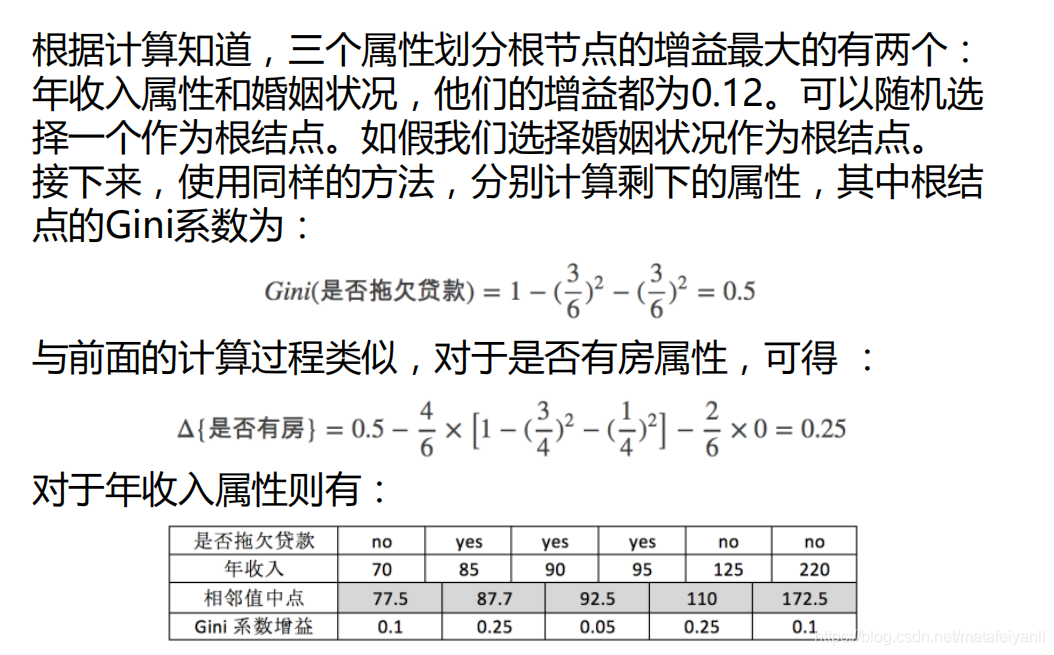
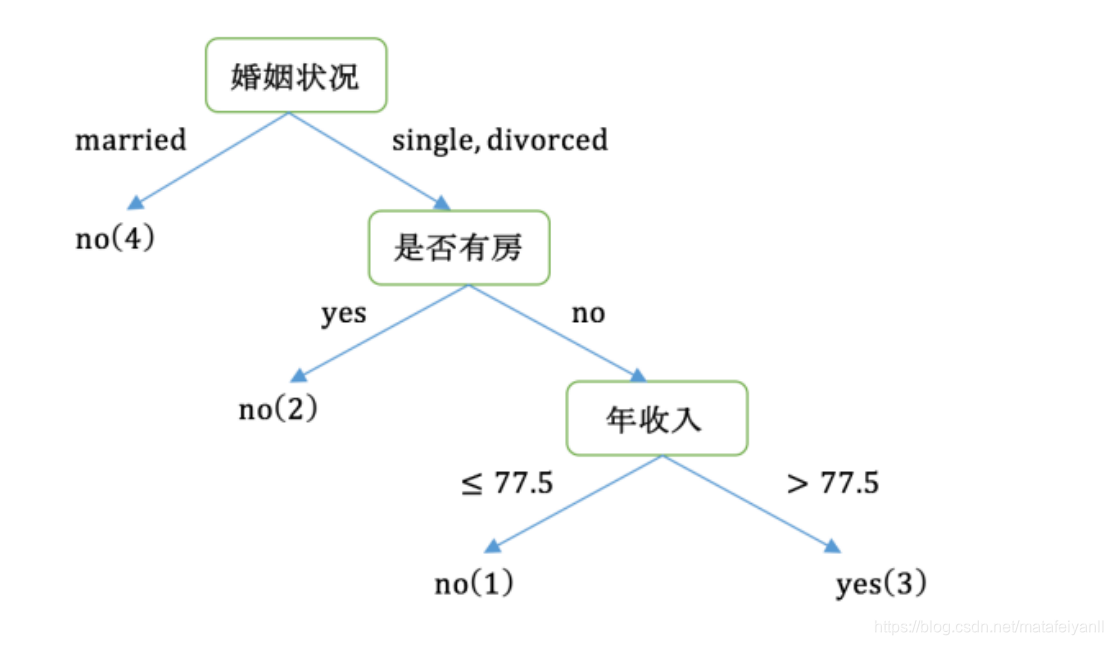
优缺点
优点:
小规模数据集有效
缺点:
处理连续变量不好
类别较多时,错误增加的比较快
不能处理大量数据
算法实现
from sklearn import tree
import numpy as np
# 载入数据
data = np.genfromtxt("cart.csv", delimiter=",")
x_data = data[1:,1:-1]
y_data = data[1:,-1]
# 创建决策树模型
model = tree.DecisionTreeClassifier()
# 输入数据建立模型
model.fit(x_data, y_data)
# 导出决策树
import graphviz # http://www.graphviz.org/
dot_data = tree.export_graphviz(model,
out_file = None,
feature_names = ['house_yes','house_no','single','married','divorced','income'],
class_names = ['no','yes'],
filled = True,
rounded = True,
special_characters = True)
graph = graphviz.Source(dot_data)
graph.render('cart')
决策树-线性二分类举例
import matplotlib.pyplot as plt
import numpy as np
from sklearn.metrics import classification_report
from sklearn import tree
# 载入数据
data = np.genfromtxt("LR-testSet.csv", delimiter=",")
x_data = data[:,:-1]
y_data = data[:,-1]
plt.scatter(x_data[:,0],x_data[:,1],c=y_data)
plt.show()
# 创建决策树模型
model = tree.DecisionTreeClassifier()
# 输入数据建立模型
model.fit(x_data, y_data)
导出决策树
import graphviz # http://www.graphviz.org/
dot_data = tree.export_graphviz(model,
out_file = None,
feature_names = ['x','y'],
class_names = ['label0','label1'],
filled = True,
rounded = True,
special_characters = True)
graph = graphviz.Source(dot_data)
# 获取数据值所在的范围
x_min, x_max = x_data[:, 0].min() - 1, x_data[:, 0].max() + 1
y_min, y_max = x_data[:, 1].min() - 1, x_data[:, 1].max() + 1
# 生成网格矩阵
xx, yy = np.meshgrid(np.arange(x_min, x_max, 0.02),
np.arange(y_min, y_max, 0.02))
z = model.predict(np.c_[xx.ravel(), yy.ravel()])# ravel与flatten类似,多维数据转一维。flatten不会改变原始数据,ravel会改变原始数据
z = z.reshape(xx.shape)
# 等高线图
cs = plt.contourf(xx, yy, z)
# 样本散点图
plt.scatter(x_data[:, 0], x_data[:, 1], c=y_data)
plt.show()
predictions = model.predict(x_data)
print(classification_report(predictions,y_data))
决策树-非线性二分类举例
import matplotlib.pyplot as plt
import numpy as np
from sklearn.metrics import classification_report
from sklearn import tree
from sklearn.model_selection import train_test_split
# 载入数据
data = np.genfromtxt("LR-testSet2.txt", delimiter=",")
x_data = data[:,:-1]
y_data = data[:,-1]
plt.scatter(x_data[:,0],x_data[:,1],c=y_data)
plt.show()
#分割数据
x_train,x_test,y_train,y_test = train_test_split(x_data, y_data)
# 创建决策树模型
# max_depth,树的深度
# min_samples_split 内部节点再划分所需最小样本数
model = tree.DecisionTreeClassifier(max_depth=7,min_samples_split=4)
# 输入数据建立模型
model.fit(x_train, y_train)
# 导出决策树
import graphviz # http://www.graphviz.org/
dot_data = tree.export_graphviz(model,
out_file = None,
feature_names = ['x','y'],
class_names = ['label0','label1'],
filled = True,
rounded = True,
special_characters = True)
graph = graphviz.Source(dot_data)
# 获取数据值所在的范围
x_min, x_max = x_data[:, 0].min() - 1, x_data[:, 0].max() + 1
y_min, y_max = x_data[:, 1].min() - 1, x_data[:, 1].max() + 1
# 生成网格矩阵
xx, yy = np.meshgrid(np.arange(x_min, x_max, 0.02),
np.arange(y_min, y_max, 0.02))
z = model.predict(np.c_[xx.ravel(), yy.ravel()])# ravel与flatten类似,多维数据转一维。flatten不会改变原始数据,ravel会改变原始数据
z = z.reshape(xx.shape)
# 等高线图
cs = plt.contourf(xx, yy, z)
# 样本散点图
plt.scatter(x_data[:, 0], x_data[:, 1], c=y_data)
plt.show()
predictions = model.predict(x_train)
print(classification_report(predictions,y_train))
predictions = model.predict(x_test)
print(classification_report(predictions,y_test))