ACM上的会议文章,中科院自动化所的工作,用于做宏表情与微表情的检测
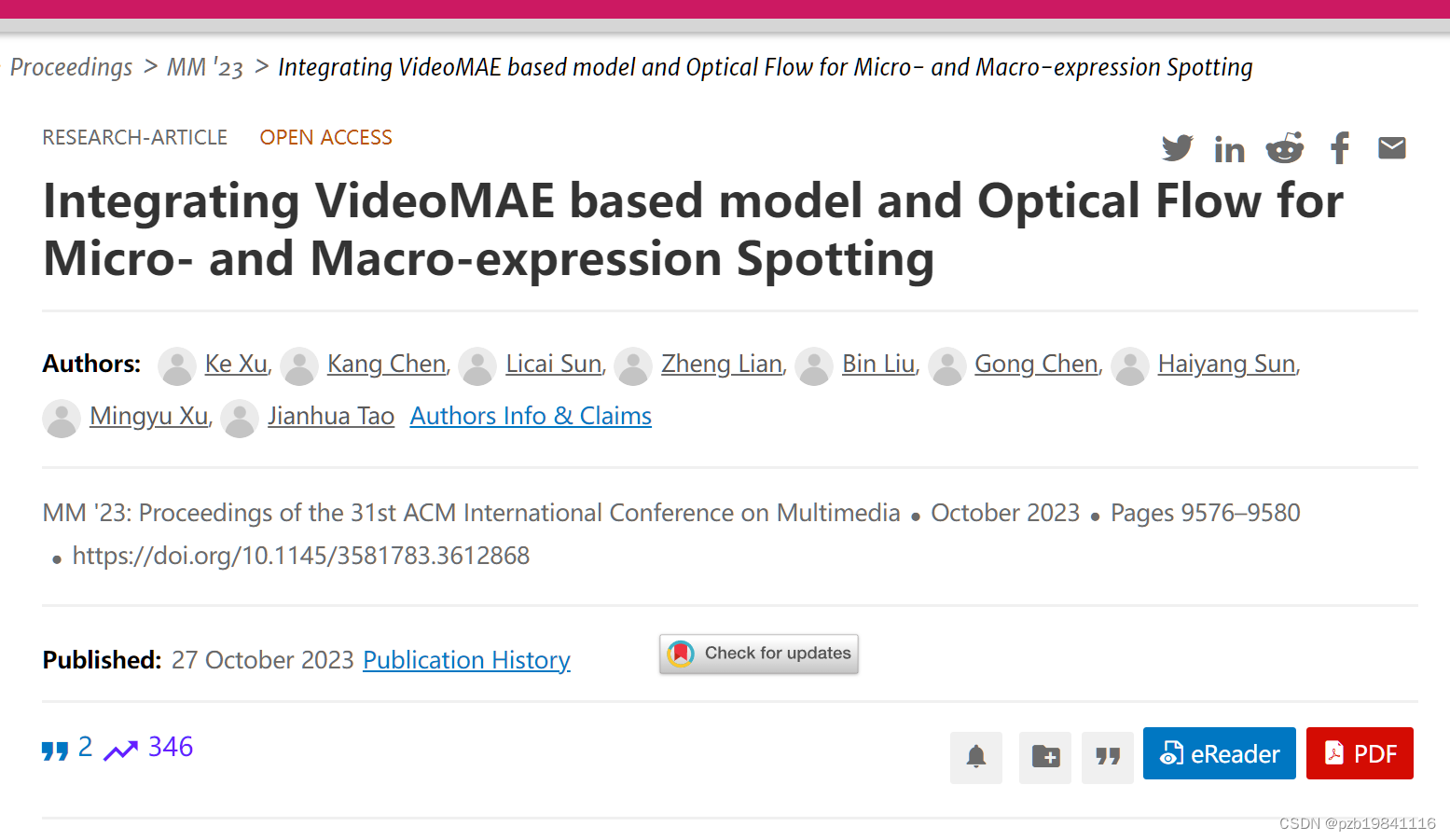
摘要:
In this paper, we propose a pre-trained model combined with the optical flow method to improve the accuracy and robustness of macro- and micro-expression spotting.
本文提出了一种结合预训练模型和光流方法来提高宏表情和微表情检测的准确性和鲁棒性。
1.简介
In general, tasks related to micro-expression include two main aspects: micro-expression spotting in a long video and emotion recognition in micro-expression clips
一般而言,与微表情相关的任务主要包括两个方面:长视频中的微表情识别和微表情片段中的情绪识别
At the same time, in a long video, there will inevitably be blinking and shaking of the head and other factors to interfere with, which makes micro-expression spotting task challenging.
同时,