电商评论文本情感分类(中文文本分类+中文词云图)
本项目包含:
1.中文文本处理
2.中文词云图绘制
3.中文词嵌入
4.基于textcnn的中文文本分类(Test_Acc=89.2000)
5.基于bert的中文文本分配(Test_Acc=0.91)
其他说明
完整代码获取
👉因为代码很长,部分Class做了隐藏处理,右上角fork后可展示全部,感觉对你有用的话可以点个赞~
👉本项目分为Bert和TextCNN,通过点击左侧Notebook列表中的Notebook进行不同模型实现的查看
👉textcnn使用cpu即可,Bert使用的是T4-GPU,运行时间:10个epoch大概100分钟
👉部分代码复用了参考文章中的,但是总是会有一些问题,做了部分修改
👉参数没有精调,精调后应该还会有提升
往期文章和专栏
👉往期文章可以关注我的专栏
下巴同学的数据加油小站
会不定期分享数据挖掘、机器学习、风控模型、深度学习、NLP等方向的学习项目,关注不一定能学到你想学的东西,但是可以学到我想学和正在学的东西😀
参考文章
1.Huggingface Transformers实战教程
2.kaggle-pytorch-text-classification-torchtext-lstm
3.中文文本分类
4.中文词向量
5.torchtext-Field详解
# !pip install transformers
# 导入transformers
import transformers
# from transformers import BertModel, BertTokenizer,BertConfig, AdamW, get_linear_schedule_with_warmup
from transformers import AutoModel, AutoTokenizer,AutoConfig, AdamW, get_linear_schedule_with_warmup
# 导入torch
import torch
from torch import nn, optim
from torch.utils.data import Dataset, DataLoader
import torch.nn.functional as F
# 常用包
import re
import numpy as np
import pandas as pd
import seaborn as sns
from pylab import rcParams
import matplotlib.pyplot as plt
from matplotlib import rc
from sklearn.model_selection import train_test_split
from sklearn.metrics import confusion_matrix, classification_report
from collections import defaultdict
from textwrap import wrap
%matplotlib inline
# 固定随机种子
RANDOM_SEED = 2022
np.random.seed(RANDOM_SEED)
torch.manual_seed(RANDOM_SEED)
device = torch.device("cuda:0" if torch.cuda.is_available() else "cpu")
device
device(type='cuda', index=0)
data = pd.read_csv('/home/mw/input/comment4cls4805/text2cls.csv')
data.drop('Unnamed: 0',axis=1,inplace=True)
data.head()
text | label | |
---|---|---|
0 | 酒店设计有特色,房间很舒服亦很美,位置于南门很方便出入,而且又有得免费上网。前台服务员不错,... | 1 |
1 | 地理位置不错,闹中取静。房间比较干净,布局合理。但是隔音效果太差了,有住简易客栈的感觉。临水... | 1 |
2 | 不错,下次还考虑入住。交通也方便,在餐厅吃的也不错。 | 1 |
3 | 地理位置比较便捷,逛街、旅游、办事都比较方便。老的宾馆新装修改的,房间内的设施简洁、干净,但... | 1 |
4 | 因为只住了一晚,所以没什么感觉,差不多四星吧。大堂的地砖很漂亮。房间小了一点。 | 1 |
data.info()
<class 'pandas.core.frame.DataFrame'>
RangeIndex: 10000 entries, 0 to 9999
Data columns (total 2 columns):
# Column Non-Null Count Dtype
--- ------ -------------- -----
0 text 10000 non-null object
1 label 10000 non-null int64
dtypes: int64(1), object(1)
memory usage: 156.4+ KB
data['label'].value_counts()
1 5000
0 5000
Name: label, dtype: int64
fig,ax = plt.subplots(figsize=(6,4), dpi=80)
sns.countplot(x=data.label, edgecolor="black", alpha=0.7, data=data)
sns.despine()
plt.xlabel('label count')
plt.title("Distribution of label count")
plt.tight_layout()
for p in ax.patches:
ax.annotate(f'\n{p.get_height()}', (p.get_x(), p.get_height()+5), color='black', size=10)
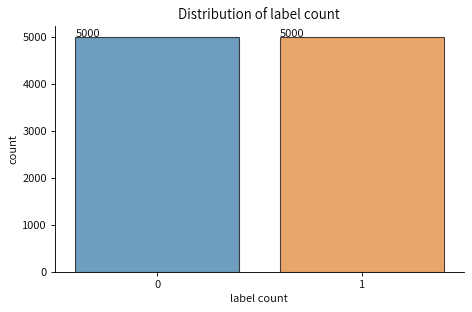
data['text_len']=data['text'].map(len)
data['text'].map(len).describe()
count 10000.000000
mean 109.083700
std 126.177622
min 4.000000
25% 34.000000
50% 69.000000
75% 135.000000
max 1985.000000
Name: text, dtype: float64
fig,ax = plt.subplots(figsize=(6,4), dpi=80)
plt.hist(data['text_len'],bins=10)
(array([8.672e+03, 9.680e+02, 2.440e+02, 7.700e+01, 1.800e+01, 1.600e+01,
3.000e+00, 0.000e+00, 0.000e+00, 2.000e+00]),
array([ 4. , 202.1, 400.2, 598.3, 796.4, 994.5, 1192.6, 1390.7,
1588.8, 1786.9, 1985. ]),
<BarContainer object of 10 artists>)
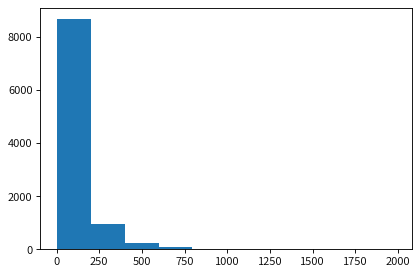
sum(data['text_len']>250) # text文本长度大于250的个数
907
sum(data['text_len']>500) # text文本长度大于500的个数
205
PRE_TRAINED_MODEL_NAME = 'bert-base-chinese'
tokenizer = AutoTokenizer.from_pretrained(PRE_TRAINED_MODEL_NAME)
tokenizer
Downloading: 0%| | 0.00/29.0 [00:00<?, ?B/s]
Downloading: 0%| | 0.00/624 [00:00<?, ?B/s]
Downloading: 0%| | 0.00/110k [00:00<?, ?B/s]
Downloading: 0%| | 0.00/269k [00:00<?, ?B/s]
PreTrainedTokenizerFast(name_or_path='bert-base-chinese', vocab_size=21128, model_max_len=512, is_fast=True, padding_side='right', truncation_side='right', special_tokens={'unk_token': '[UNK]', 'sep_token': '[SEP]', 'pad_token': '[PAD]', 'cls_token': '[CLS]', 'mask_token': '[MASK]'})
token_lens = []
for txt in data.text:
tokens = tokenizer.encode(txt, max_length=512)
token_lens.append(len(tokens))
Truncation was not explicitly activated but `max_length` is provided a specific value, please use `truncation=True` to explicitly truncate examples to max length. Defaulting to 'longest_first' truncation strategy. If you encode pairs of sequences (GLUE-style) with the tokenizer you can select this strategy more precisely by providing a specific strategy to `truncation`.
sns.distplot(token_lens)
plt.xlim([0, 512]);
plt.xlabel('Token count');
/opt/conda/lib/python3.7/site-packages/seaborn/distributions.py:2619: FutureWarning: `distplot` is a deprecated function and will be removed in a future version. Please adapt your code to use either `displot` (a figure-level function with similar flexibility) or `histplot` (an axes-level function for histograms).
warnings.warn(msg, FutureWarning)
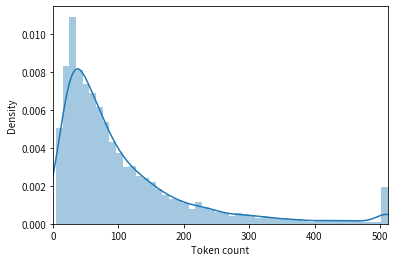
class EnterpriseDataset(Dataset):
def __init__(self,texts,labels,tokenizer,max_len):
self.texts=texts
self.labels=labels
self.tokenizer=tokenizer
self.max_len=max_len
def __len__(self):
return len(self.texts)
def __getitem__(self,item):
"""
item 为数据索引,迭代取第item条数据
"""
text=str(self.texts[item])
label=self.labels[item]
encoding=self.tokenizer.encode_plus(
text,
add_special_tokens=True,
max_length=self.max_len,
return_token_type_ids=True,
pad_to_max_length=True,
return_attention_mask=True,
return_tensors='pt',
)
# print(encoding['input_ids'])
return {
'texts':text,
'input_ids':encoding['input_ids'].flatten(),
'attention_mask':encoding['attention_mask'].flatten(),
# toeken_type_ids:0
'labels':torch.tensor(label,dtype=torch.long)
}
df_train, df_test = train_test_split(data, test_size=0.2, random_state=RANDOM_SEED)
df_val, df_test = train_test_split(df_test, test_size=0.5, random_state=RANDOM_SEED)
df_train.shape, df_val.shape, df_test.shape
((8000, 3), (1000, 3), (1000, 3))
def create_data_loader(df,tokenizer,max_len,batch_size):
ds=EnterpriseDataset(
texts=df['text'].values,
labels=df['label'].values,
tokenizer=tokenizer,
max_len=max_len
)
return DataLoader(
ds,
batch_size=batch_size,
# num_workers=4 # windows多线程
)
BATCH_SIZE = 4
MAX_LEN=500
train_data_loader = create_data_loader(df_train, tokenizer, MAX_LEN, BATCH_SIZE)
val_data_loader = create_data_loader(df_val, tokenizer, MAX_LEN, BATCH_SIZE)
test_data_loader = create_data_loader(df_test, tokenizer, MAX_LEN, BATCH_SIZE)
next(iter(train_data_loader))
/opt/conda/lib/python3.7/site-packages/transformers/tokenization_utils_base.py:2342: FutureWarning: The `pad_to_max_length` argument is deprecated and will be removed in a future version, use `padding=True` or `padding='longest'` to pad to the longest sequence in the batch, or use `padding='max_length'` to pad to a max length. In this case, you can give a specific length with `max_length` (e.g. `max_length=45`) or leave max_length to None to pad to the maximal input size of the model (e.g. 512 for Bert).
FutureWarning,
{'texts': ['这个宾馆就在王府井步行街旁.地点相当好.价格也算优惠.但原本预定住5天,结果第二天就退房了.因为该宾馆的空调竟然停用.原因是北京市的供暖系统已经停用.后来和其他饭店求证才知道是胡扯...害我度过了一个寒冷的北京之夜.',
'不亏是园林酒店.酒店面积超大,住宿区分很多楼,并有面积不小的绿化.非常适合休闲度假,唯一欠缺一点的就是不直接面对海.',
'日本人真的是超多,老酒店房间布局还可以,就是地毯怪味太多,房间空气不太好,窗户不好打开。服务还行,交通方便,去火车站、快轨、景点都很方便,就是在火车站旁边环境比较乱。房间价格稍贵,基本上还比较有竞争力。',
'早餐有还不如无!这傍边就是长途汽车站,可想而知这外面的环境是个什么样子!住在这里的人更多的是中转旅客的样子,我见过尼姑,和尚!房间设施也就比招待所好点的样子,比较旧的酒店,名字虽然很时尚,但是酒店真的没有可比性。有个米兰俱乐部,估计是happy的地方,还有个桑拿按摩的地方,这个不用我多说大家也晓得是干吗的,每天晚上10点准时房间电话响起,招不招就随你了。大家自己掂量着吧。'],
'input_ids': tensor([[ 101, 6821, 702, ..., 0, 0, 0],
[ 101, 679, 755, ..., 0, 0, 0],
[ 101, 3189, 3315, ..., 0, 0, 0],
[ 101, 3193, 7623, ..., 0, 0, 0]]),
'attention_mask': tensor([[1, 1, 1, ..., 0, 0, 0],
[1, 1, 1, ..., 0, 0, 0],
[1, 1, 1, ..., 0, 0, 0],
[1, 1, 1, ..., 0, 0, 0]]),
'labels': tensor([0, 1, 1, 0])}
w_data = next(iter(train_data_loader))
w_data.keys()
dict_keys(['texts', 'input_ids', 'attention_mask', 'labels'])
print(w_data['input_ids'].shape)
print(w_data['attention_mask'].shape)
print(w_data['labels'].shape)
torch.Size([4, 500])
torch.Size([4, 500])
torch.Size([4])
bert_model = AutoModel.from_pretrained(PRE_TRAINED_MODEL_NAME)
Downloading: 0%| | 0.00/412M [00:00<?, ?B/s]
Some weights of the model checkpoint at bert-base-chinese were not used when initializing BertModel: ['cls.predictions.transform.dense.weight', 'cls.predictions.transform.LayerNorm.bias', 'cls.seq_relationship.bias', 'cls.predictions.transform.dense.bias', 'cls.predictions.decoder.weight', 'cls.seq_relationship.weight', 'cls.predictions.bias', 'cls.predictions.transform.LayerNorm.weight']
- This IS expected if you are initializing BertModel from the checkpoint of a model trained on another task or with another architecture (e.g. initializing a BertForSequenceClassification model from a BertForPreTraining model).
- This IS NOT expected if you are initializing BertModel from the checkpoint of a model that you expect to be exactly identical (initializing a BertForSequenceClassification model from a BertForSequenceClassification model).
# bert_model
BertModel(
(embeddings): BertEmbeddings(
(word_embeddings): Embedding(21128, 768, padding_idx=0)
(position_embeddings): Embedding(512, 768)
(token_type_embeddings): Embedding(2, 768)
(LayerNorm): LayerNorm((768,), eps=1e-12, elementwise_affine=True)
(dropout): Dropout(p=0.1, inplace=False)
)
(encoder): BertEncoder(
(layer): ModuleList(
(0): BertLayer(
(attention): BertAttention(
(self): BertSelfAttention(
(query): Linear(in_features=768, out_features=768, bias=True)
(key): Linear(in_features=768, out_features=768, bias=True)
(value): Linear(in_features=768, out_features=768, bias=True)
(dropout): Dropout(p=0.1, inplace=False)
)
(output): BertSelfOutput(
(dense): Linear(in_features=768, out_features=768, bias=True)
(LayerNorm): LayerNorm((768,), eps=1e-12, elementwise_affine=True)
(dropout): Dropout(p=0.1, inplace=False)
)
)
(intermediate): BertIntermediate(
(dense): Linear(in_features=768, out_features=3072, bias=True)
(intermediate_act_fn): GELUActivation()
)
(output): BertOutput(
(dense): Linear(in_features=3072, out_features=768, bias=True)
(LayerNorm): LayerNorm((768,), eps=1e-12, elementwise_affine=True)
(dropout): Dropout(p=0.1, inplace=False)
)
)
(1): BertLayer(
(attention): BertAttention(
(self): BertSelfAttention(
(query): Linear(in_features=768, out_features=768, bias=True)
(key): Linear(in_features=768, out_features=768, bias=True)
(value): Linear(in_features=768, out_features=768, bias=True)
(dropout): Dropout(p=0.1, inplace=False)
)
(output): BertSelfOutput(
(dense): Linear(in_features=768, out_features=768, bias=True)
(LayerNorm): LayerNorm((768,), eps=1e-12, elementwise_affine=True)
(dropout): Dropout(p=0.1, inplace=False)
)
)
(intermediate): BertIntermediate(
(dense): Linear(in_features=768, out_features=3072, bias=True)
(intermediate_act_fn): GELUActivation()
)
(output): BertOutput(
(dense): Linear(in_features=3072, out_features=768, bias=True)
(LayerNorm): LayerNorm((768,), eps=1e-12, elementwise_affine=True)
(dropout): Dropout(p=0.1, inplace=False)
)
)
(2): BertLayer(
(attention): BertAttention(
(self): BertSelfAttention(
(query): Linear(in_features=768, out_features=768, bias=True)
(key): Linear(in_features=768, out_features=768, bias=True)
(value): Linear(in_features=768, out_features=768, bias=True)
(dropout): Dropout(p=0.1, inplace=False)
)
(output): BertSelfOutput(
(dense): Linear(in_features=768, out_features=768, bias=True)
(LayerNorm): LayerNorm((768,), eps=1e-12, elementwise_affine=True)
(dropout): Dropout(p=0.1, inplace=False)
)
)
(intermediate): BertIntermediate(
(dense): Linear(in_features=768, out_features=3072, bias=True)
(intermediate_act_fn): GELUActivation()
)
(output): BertOutput(
(dense): Linear(in_features=3072, out_features=768, bias=True)
(LayerNorm): LayerNorm((768,), eps=1e-12, elementwise_affine=True)
(dropout): Dropout(p=0.1, inplace=False)
)
)
(3): BertLayer(
(attention): BertAttention(
(self): BertSelfAttention(
(query): Linear(in_features=768, out_features=768, bias=True)
(key): Linear(in_features=768, out_features=768, bias=True)
(value): Linear(in_features=768, out_features=768, bias=True)
(dropout): Dropout(p=0.1, inplace=False)
)
(output): BertSelfOutput(
(dense): Linear(in_features=768, out_features=768, bias=True)
(LayerNorm): LayerNorm((768,), eps=1e-12, elementwise_affine=True)
(dropout): Dropout(p=0.1, inplace=False)
)
)
(intermediate): BertIntermediate(
(dense): Linear(in_features=768, out_features=3072, bias=True)
(intermediate_act_fn): GELUActivation()
)
(output): BertOutput(
(dense): Linear(in_features=3072, out_features=768, bias=True)
(LayerNorm): LayerNorm((768,), eps=1e-12, elementwise_affine=True)
(dropout): Dropout(p=0.1, inplace=False)
)
)
(4): BertLayer(
(attention): BertAttention(
(self): BertSelfAttention(
(query): Linear(in_features=768, out_features=768, bias=True)
(key): Linear(in_features=768, out_features=768, bias=True)
(value): Linear(in_features=768, out_features=768, bias=True)
(dropout): Dropout(p=0.1, inplace=False)
)
(output): BertSelfOutput(
(dense): Linear(in_features=768, out_features=768, bias=True)
(LayerNorm): LayerNorm((768,), eps=1e-12, elementwise_affine=True)
(dropout): Dropout(p=0.1, inplace=False)
)
)
(intermediate): BertIntermediate(
(dense): Linear(in_features=768, out_features=3072, bias=True)
(intermediate_act_fn): GELUActivation()
)
(output): BertOutput(
(dense): Linear(in_features=3072, out_features=768, bias=True)
(LayerNorm): LayerNorm((768,), eps=1e-12, elementwise_affine=True)
(dropout): Dropout(p=0.1, inplace=False)
)
)
(5): BertLayer(
(attention): BertAttention(
(self): BertSelfAttention(
(query): Linear(in_features=768, out_features=768, bias=True)
(key): Linear(in_features=768, out_features=768, bias=True)
(value): Linear(in_features=768, out_features=768, bias=True)
(dropout): Dropout(p=0.1, inplace=False)
)
(output): BertSelfOutput(
(dense): Linear(in_features=768, out_features=768, bias=True)
(LayerNorm): LayerNorm((768,), eps=1e-12, elementwise_affine=True)
(dropout): Dropout(p=0.1, inplace=False)
)
)
(intermediate): BertIntermediate(
(dense): Linear(in_features=768, out_features=3072, bias=True)
(intermediate_act_fn): GELUActivation()
)
(output): BertOutput(
(dense): Linear(in_features=3072, out_features=768, bias=True)
(LayerNorm): LayerNorm((768,), eps=1e-12, elementwise_affine=True)
(dropout): Dropout(p=0.1, inplace=False)
)
)
(6): BertLayer(
(attention): BertAttention(
(self): BertSelfAttention(
(query): Linear(in_features=768, out_features=768, bias=True)
(key): Linear(in_features=768, out_features=768, bias=True)
(value): Linear(in_features=768, out_features=768, bias=True)
(dropout): Dropout(p=0.1, inplace=False)
)
(output): BertSelfOutput(
(dense): Linear(in_features=768, out_features=768, bias=True)
(LayerNorm): LayerNorm((768,), eps=1e-12, elementwise_affine=True)
(dropout): Dropout(p=0.1, inplace=False)
)
)
(intermediate): BertIntermediate(
(dense): Linear(in_features=768, out_features=3072, bias=True)
(intermediate_act_fn): GELUActivation()
)
(output): BertOutput(
(dense): Linear(in_features=3072, out_features=768, bias=True)
(LayerNorm): LayerNorm((768,), eps=1e-12, elementwise_affine=True)
(dropout): Dropout(p=0.1, inplace=False)
)
)
(7): BertLayer(
(attention): BertAttention(
(self): BertSelfAttention(
(query): Linear(in_features=768, out_features=768, bias=True)
(key): Linear(in_features=768, out_features=768, bias=True)
(value): Linear(in_features=768, out_features=768, bias=True)
(dropout): Dropout(p=0.1, inplace=False)
)
(output): BertSelfOutput(
(dense): Linear(in_features=768, out_features=768, bias=True)
(LayerNorm): LayerNorm((768,), eps=1e-12, elementwise_affine=True)
(dropout): Dropout(p=0.1, inplace=False)
)
)
(intermediate): BertIntermediate(
(dense): Linear(in_features=768, out_features=3072, bias=True)
(intermediate_act_fn): GELUActivation()
)
(output): BertOutput(
(dense): Linear(in_features=3072, out_features=768, bias=True)
(LayerNorm): LayerNorm((768,), eps=1e-12, elementwise_affine=True)
(dropout): Dropout(p=0.1, inplace=False)
)
)
(8): BertLayer(
(attention): BertAttention(
(self): BertSelfAttention(
(query): Linear(in_features=768, out_features=768, bias=True)
(key): Linear(in_features=768, out_features=768, bias=True)
(value): Linear(in_features=768, out_features=768, bias=True)
(dropout): Dropout(p=0.1, inplace=False)
)
(output): BertSelfOutput(
(dense): Linear(in_features=768, out_features=768, bias=True)
(LayerNorm): LayerNorm((768,), eps=1e-12, elementwise_affine=True)
(dropout): Dropout(p=0.1, inplace=False)
)
)
(intermediate): BertIntermediate(
(dense): Linear(in_features=768, out_features=3072, bias=True)
(intermediate_act_fn): GELUActivation()
)
(output): BertOutput(
(dense): Linear(in_features=3072, out_features=768, bias=True)
(LayerNorm): LayerNorm((768,), eps=1e-12, elementwise_affine=True)
(dropout): Dropout(p=0.1, inplace=False)
)
)
(9): BertLayer(
(attention): BertAttention(
(self): BertSelfAttention(
(query): Linear(in_features=768, out_features=768, bias=True)
(key): Linear(in_features=768, out_features=768, bias=True)
(value): Linear(in_features=768, out_features=768, bias=True)
(dropout): Dropout(p=0.1, inplace=False)
)
(output): BertSelfOutput(
(dense): Linear(in_features=768, out_features=768, bias=True)
(LayerNorm): LayerNorm((768,), eps=1e-12, elementwise_affine=True)
(dropout): Dropout(p=0.1, inplace=False)
)
)
(intermediate): BertIntermediate(
(dense): Linear(in_features=768, out_features=3072, bias=True)
(intermediate_act_fn): GELUActivation()
)
(output): BertOutput(
(dense): Linear(in_features=3072, out_features=768, bias=True)
(LayerNorm): LayerNorm((768,), eps=1e-12, elementwise_affine=True)
(dropout): Dropout(p=0.1, inplace=False)
)
)
(10): BertLayer(
(attention): BertAttention(
(self): BertSelfAttention(
(query): Linear(in_features=768, out_features=768, bias=True)
(key): Linear(in_features=768, out_features=768, bias=True)
(value): Linear(in_features=768, out_features=768, bias=True)
(dropout): Dropout(p=0.1, inplace=False)
)
(output): BertSelfOutput(
(dense): Linear(in_features=768, out_features=768, bias=True)
(LayerNorm): LayerNorm((768,), eps=1e-12, elementwise_affine=True)
(dropout): Dropout(p=0.1, inplace=False)
)
)
(intermediate): BertIntermediate(
(dense): Linear(in_features=768, out_features=3072, bias=True)
(intermediate_act_fn): GELUActivation()
)
(output): BertOutput(
(dense): Linear(in_features=3072, out_features=768, bias=True)
(LayerNorm): LayerNorm((768,), eps=1e-12, elementwise_affine=True)
(dropout): Dropout(p=0.1, inplace=False)
)
)
(11): BertLayer(
(attention): BertAttention(
(self): BertSelfAttention(
(query): Linear(in_features=768, out_features=768, bias=True)
(key): Linear(in_features=768, out_features=768, bias=True)
(value): Linear(in_features=768, out_features=768, bias=True)
(dropout): Dropout(p=0.1, inplace=False)
)
(output): BertSelfOutput(
(dense): Linear(in_features=768, out_features=768, bias=True)
(LayerNorm): LayerNorm((768,), eps=1e-12, elementwise_affine=True)
(dropout): Dropout(p=0.1, inplace=False)
)
)
(intermediate): BertIntermediate(
(dense): Linear(in_features=768, out_features=3072, bias=True)
(intermediate_act_fn): GELUActivation()
)
(output): BertOutput(
(dense): Linear(in_features=3072, out_features=768, bias=True)
(LayerNorm): LayerNorm((768,), eps=1e-12, elementwise_affine=True)
(dropout): Dropout(p=0.1, inplace=False)
)
)
)
)
(pooler): BertPooler(
(dense): Linear(in_features=768, out_features=768, bias=True)
(activation): Tanh()
)
)
class EnterpriseDangerClassifier(nn.Module):
def __init__(self, n_classes):
super(EnterpriseDangerClassifier, self).__init__()
self.bert = bert_model.from_pretrained(PRE_TRAINED_MODEL_NAME)
self.drop = nn.Dropout(p=0.3)
self.out = nn.Linear(self.bert.config.hidden_size, n_classes) # 两个类别
def forward(self, input_ids, attention_mask):
_, pooled_output = self.bert(
input_ids=input_ids,
attention_mask=attention_mask,
return_dict = False
)
output = self.drop(pooled_output) # dropout
return self.out(output)
class_names=[0,1]
model = EnterpriseDangerClassifier(len(class_names))
model = model.to(device)
Some weights of the model checkpoint at bert-base-chinese were not used when initializing BertModel: ['cls.predictions.transform.dense.weight', 'cls.predictions.transform.LayerNorm.bias', 'cls.seq_relationship.bias', 'cls.predictions.transform.dense.bias', 'cls.predictions.decoder.weight', 'cls.seq_relationship.weight', 'cls.predictions.bias', 'cls.predictions.transform.LayerNorm.weight']
- This IS expected if you are initializing BertModel from the checkpoint of a model trained on another task or with another architecture (e.g. initializing a BertForSequenceClassification model from a BertForPreTraining model).
- This IS NOT expected if you are initializing BertModel from the checkpoint of a model that you expect to be exactly identical (initializing a BertForSequenceClassification model from a BertForSequenceClassification model).
EPOCHS = 5 # 训练轮数
optimizer = AdamW(model.parameters(), lr=2e-5, correct_bias=False)
total_steps = len(train_data_loader) * EPOCHS
scheduler = get_linear_schedule_with_warmup(
optimizer,
num_warmup_steps=0,
num_training_steps=total_steps
)
loss_fn = nn.CrossEntropyLoss().to(device)
/opt/conda/lib/python3.7/site-packages/transformers/optimization.py:310: FutureWarning: This implementation of AdamW is deprecated and will be removed in a future version. Use the PyTorch implementation torch.optim.AdamW instead, or set `no_deprecation_warning=True` to disable this warning
FutureWarning,
def train_epoch(
model,
data_loader,
loss_fn,
optimizer,
device,
scheduler,
n_examples
):
model = model.train() # train模式
losses = []
correct_predictions = 0
for d in data_loader:
input_ids = d["input_ids"].to(device)
attention_mask = d["attention_mask"].to(device)
targets = d["labels"].to(device)
outputs = model(
input_ids=input_ids,
attention_mask=attention_mask
)
_, preds = torch.max(outputs, dim=1)
loss = loss_fn(outputs, targets)
correct_predictions += torch.sum(preds == targets)
losses.append(loss.item())
loss.backward()
nn.utils.clip_grad_norm_(model.parameters(), max_norm=1.0)
optimizer.step()
scheduler.step()
optimizer.zero_grad()
return correct_predictions.double() / n_examples, np.mean(losses)
def eval_model(model, data_loader, loss_fn, device, n_examples):
model = model.eval() # 验证预测模式
losses = []
correct_predictions = 0
with torch.no_grad():
for d in data_loader:
input_ids = d["input_ids"].to(device)
attention_mask = d["attention_mask"].to(device)
targets = d["labels"].to(device)
outputs = model(
input_ids=input_ids,
attention_mask=attention_mask
)
_, preds = torch.max(outputs, dim=1)
loss = loss_fn(outputs, targets)
correct_predictions += torch.sum(preds == targets)
losses.append(loss.item())
return correct_predictions.double() / n_examples, np.mean(losses)
history = defaultdict(list) # 记录10轮loss和acc
best_accuracy = 0
for epoch in range(EPOCHS):
print(f'Epoch {epoch + 1}/{EPOCHS}')
print('-' * 10)
train_acc, train_loss = train_epoch(
model,
train_data_loader,
loss_fn,
optimizer,
device,
scheduler,
len(df_train)
)
print(f'Train loss {train_loss} accuracy {train_acc}')
val_acc, val_loss = eval_model(
model,
val_data_loader,
loss_fn,
device,
len(df_val)
)
print(f'Val loss {val_loss} accuracy {val_acc}')
print()
history['train_acc'].append(train_acc)
history['train_loss'].append(train_loss)
history['val_acc'].append(val_acc)
history['val_loss'].append(val_loss)
if val_acc > best_accuracy:
torch.save(model.state_dict(), 'best_model_state.bin')
best_accuracy = val_acc
Epoch 1/10
----------
Train loss 0.4483157523704431 accuracy 0.8758750000000001
Val loss 0.3820066763390787 accuracy 0.916
Epoch 2/10
----------
Train loss 0.32049993686759265 accuracy 0.929625
Val loss 0.40485098455194385 accuracy 0.924
Epoch 3/10
----------
Train loss 0.24836466495708737 accuracy 0.94825
Val loss 0.28995410920120773 accuracy 0.9400000000000001
Epoch 4/10
----------
Train loss 0.17626817778327677 accuracy 0.966375
Val loss 0.4069448918822454 accuracy 0.921
Epoch 5/10
----------
Train loss 0.1305098664256002 accuracy 0.976
Val loss 0.308055481798714 accuracy 0.9400000000000001
Epoch 6/10
----------
Train loss 0.10725883625710776 accuracy 0.9805
Val loss 0.4173983749264153 accuracy 0.929
Epoch 7/10
----------
Train loss 0.08332291013808207 accuracy 0.987
Val loss 0.43353290081146406 accuracy 0.929
Epoch 8/10
----------
Train loss 0.0521099294915748 accuracy 0.9915
Val loss 0.4972276862194994 accuracy 0.928
Epoch 9/10
----------
Train loss 0.0361898203453311 accuracy 0.99475
Val loss 0.5580290119879937 accuracy 0.926
Epoch 10/10
----------
Train loss 0.02941038768033286 accuracy 0.9958750000000001
Val loss 0.551199046919297 accuracy 0.924
train_acc = []
for i in range(10):
a = history['train_acc'][i].cpu().numpy()
train_acc.append(a)
val_acc = []
for i in range(10):
a = history['val_acc'][i].cpu().numpy()
val_acc.append(a)
plt.plot(train_acc, label='train accuracy')
plt.plot(val_acc, label='validation accuracy')
plt.title('Training history')
plt.ylabel('Accuracy')
plt.xlabel('Epoch')
plt.legend()
plt.ylim([0.8, 1]);
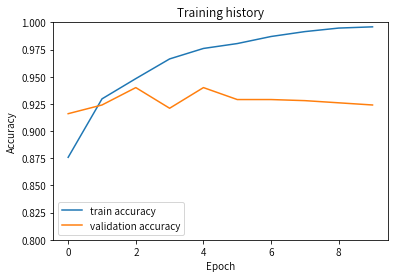
test_acc, _ = eval_model(
model,
test_data_loader,
loss_fn,
device,
len(df_test)
)
test_acc.item()
/opt/conda/lib/python3.7/site-packages/transformers/tokenization_utils_base.py:2342: FutureWarning: The `pad_to_max_length` argument is deprecated and will be removed in a future version, use `padding=True` or `padding='longest'` to pad to the longest sequence in the batch, or use `padding='max_length'` to pad to a max length. In this case, you can give a specific length with `max_length` (e.g. `max_length=45`) or leave max_length to None to pad to the maximal input size of the model (e.g. 512 for Bert).
FutureWarning,
0.91
def get_predictions(model, data_loader):
model = model.eval()
raw_texts = []
predictions = []
prediction_probs = []
real_values = []
with torch.no_grad():
for d in data_loader:
texts = d["texts"]
input_ids = d["input_ids"].to(device)
attention_mask = d["attention_mask"].to(device)
targets = d["labels"].to(device)
outputs = model(
input_ids=input_ids,
attention_mask=attention_mask
)
_, preds = torch.max(outputs, dim=1) # 类别
probs = F.softmax(outputs, dim=1) # 概率
raw_texts.extend(texts)
predictions.extend(preds)
prediction_probs.extend(probs)
real_values.extend(targets)
predictions = torch.stack(predictions).cpu()
prediction_probs = torch.stack(prediction_probs).cpu()
real_values = torch.stack(real_values).cpu()
return raw_texts, predictions, prediction_probs, real_values
y_texts, y_pred, y_pred_probs, y_test = get_predictions(
model,
test_data_loader
)
print(classification_report(y_test, y_pred, target_names=[str(label) for label in class_names])) # 分类报告
precision recall f1-score support
0 0.92 0.91 0.91 510
1 0.90 0.91 0.91 490
accuracy 0.91 1000
macro avg 0.91 0.91 0.91 1000
weighted avg 0.91 0.91 0.91 1000