变量名、共享变量、变量空间的相关关系
相关函数
- 几点说明
- tf.name_scope() 主要管理op的命名空间的函数,也可以对变量进行命名
- tf.variable_scope 主要管理变量空间的函数
- get_variable和Variable 中的name指的计算图中的name,与程序中的变量名无关
- tf.get_variable()创建的变量名不受tf.name_scope的影响,即创建的变量的name没有name_scope定义的前缀,
- tf.get_variable() 在未指定共享变量时,如果重名会报错
- tf.Variable()会自动检测有没有变量重名,一律创建新的变量,如果有则会自行处理,即在name后面加上_+处理
- tf.Variable()受到tf.name_scope的影响
- 用tf.name_scope创建相同的域名的时候,会自动对重复的域名进行处理
- 用tf.variable_scope创建相同的域名的时候,会自动对重复的域名进行处理
- 指定共享变量的方式:2种with tf.variable_scope(scope_name,reuse=True),
tf.get_variable_scope().reuse_variables() - 共享变量主要与tf.get_variable()和tf.variable_scope有关
以上的几点是自己总结的根据下面的代码
import tensorflow as tf
import numpy as np
import matplotlib.pyplot as plt
import os
def add_layer(inputs,in_size,out_size,n_layer,activation_funtion=None):
layer_name='layer%s'%n_layer
with tf.name_scope('layer'):
with tf.name_scope('weight'):
Weights=tf.Variable(tf.random_normal([in_size,out_size]),name='W')
if activation_funtion==None:
Weights_test=tf.Variable(tf.random_normal([in_size,out_size]),name='W')
print(Weights_test.name)
print(Weights.name)
tf.summary.histogram(layer_name+'/Weights',Weights)
with tf.name_scope('biases'):
biases=tf.Variable(tf.zeros([1,out_size])+0.1,name='b')
tf.summary.histogram(layer_name+'/biases',biases)
with tf.name_scope('Wx_plus_b'):
Wx_plus_b=tf.matmul(inputs,Weights)+biases
if activation_funtion is None:
output=Wx_plus_b
else:
output=activation_funtion(Wx_plus_b)
tf.summary.histogram(layer_name+'/output',output)
return output
x_data = np.linspace(-1,1,300, dtype=np.float32)[:, np.newaxis]
noise = np.random.normal(0, 0.05, x_data.shape).astype(np.float32)
y_data = np.square(x_data) - 0.5 + noise
with tf.name_scope('inputs'):
xs=tf.placeholder(tf.float32,[None,1],name='x_in')
ys=tf.placeholder(tf.float32,[None,1],name='y_in')
l1=add_layer(xs,1,10,n_layer=1,activation_funtion=tf.nn.relu)
prediction=add_layer(l1,10,1,n_layer=2,activation_funtion=None)
with tf.name_scope('loss'):
loss=tf.reduce_mean(tf.square(ys-prediction))
tf.summary.scalar('loss',loss)
optimizer=tf.train.GradientDescentOptimizer(0.1)
with tf.name_scope('train'):
train=optimizer.minimize(loss)
init = tf.global_variables_initializer()
sess=tf.Session()
sess.run(init)
merged=tf.summary.merge_all()
writer=tf.summary.FileWriter("logs/",sess.graph)
for i in range(1000):
sess.run(train,feed_dict={xs:x_data,ys:y_data})
if i+1%50==0:
rs=sess.run(merged,feed_dict={xs:x_data,ys:y_data})
writer.add_summary(rs,i)
with tf.variable_scope("a_variable_scope") as scope:
initializer = tf.constant_initializer(value=3)
var2 = tf.get_variable(name='var3', shape=[1], dtype=tf.float32, initializer=initializer)
var21=tf.get_variable(name='wangjuin',shape=[1])
var3_reuse = tf.get_variable(name='var2', shape=[1])
var4 = tf.get_variable(name='var4', shape=[1])
scope.reuse_variables()
var4_reuse = tf.get_variable(name='var4', shape=[1])
vartest = tf.Variable(name='var5',initial_value=[4], dtype=tf.float32)
var5 = tf.Variable(name='vartest',initial_value=[4], dtype=tf.float32)
with tf.name_scope('layer_junjun'):
initializer = tf.constant_initializer(value=3)
var210=tf.Variable(name='ddddd',initial_value=[4], dtype=tf.float32)
with tf.name_scope('layer_junjun'):
initializer = tf.constant_initializer(value=3)
var211=tf.Variable(name='ddddd',initial_value=[4], dtype=tf.float32)
test_reuse = tf.get_variable(name='var4', shape=[1])
with tf.variable_scope("scope_junjun") as scope:
initializer = tf.constant_initializer(value=3)
var110=tf.Variable(name='ddddd',initial_value=[4], dtype=tf.float32)
with tf.variable_scope("scope_junjun") as scope:
initializer = tf.constant_initializer(value=3)
var120=tf.Variable(name='ddddd',initial_value=[4], dtype=tf.float32)
with tf.Session() as sess:
sess.run(tf.global_variables_initializer())
print(var2.name)
print(var21.name)
print(sess.run(var2))
print(var3_reuse.name)
print(sess.run(var3_reuse))
print(var4.name)
print(sess.run(var4))
print(var4_reuse.name)
print(sess.run(var4_reuse))
print(vartest.name)
print(var5.name)
print(var210.name)
print(var211.name)
print(test_reuse.name)
print(var110.name)
print(var120.name)
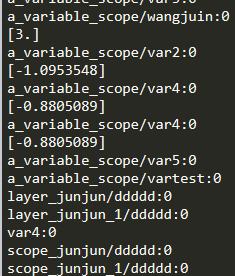