目录
二、University-1652 Dataset Introduction
2. NetWork Architecture and loss Function
(1) Generic features vs. learned features.
(2) Ground-view query vs. drone-view query
一、Abstract
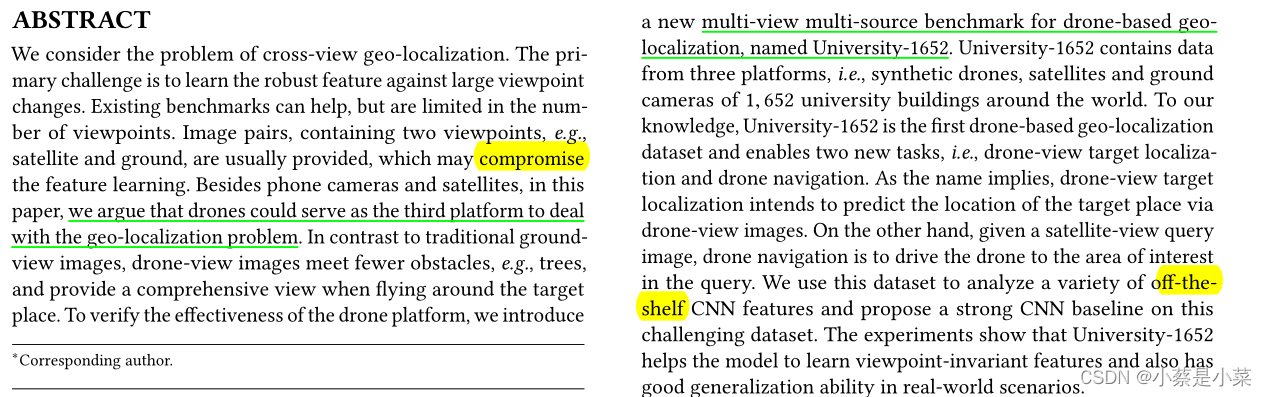
1. 交叉视觉定位地理定位现面临的最大的挑战是:在大量的视角中提取定位目标的鲁棒性特征
2. 论文创新点:在街景视角和卫星图视角的情况下添加了一个无人机视角作为第三方是视角
为证实无人机视角的有效性,引入多视角多源基准的数据---University1652(包含三个视角的数据--街景图、卫星图、无人机图),可以完成:无人机视角目标定位和无人机导航
3. 论文的主要内容
Task1:Drone-----Satelite
Given one drone-view image or video, the task aims to find the most similar satellite-view image to localize the target building in the satellite view
Task2: Satelite-----Drone
Given one satellite-view image, the drone intends to find the most relevant place (drone-
view images) that it has passed by.
Task3: Compare the generic feature trained on extremely large datasets with the viewpoint invariant feature learned on the proposed dataset.
Task4: Evaluate three basic models and three different loss terms, including contrastive loss 、triplet loss and instance loss.
二、University-1652 Dataset Introduction
1.Dataset Descirption
1652 architectures of 72 universities around the world as target locations. We do not select landmarks as the target.
For ground-view images, we first collect the data from the streetview images near the target buildings from Google Map. Specifically,we manually collect the images in different aspects of the building.However,retrieved images often contain lots of noise images, including indoor
environments and duplicates. we apply the ResNet-18 model
trained on the Place dataset to detect indoor images, and follow the setting in to remove the identical images that belong to two different buildings.
Espectially,For the drone-view images, due to the
unaffordable cost of the real-world flight, we leverage the 3D models provided by Google Earth to simulate the real drone camera.
2. Evaluation Protocol
1. 数据集的分类
We note that there are no overlapping universities in the training and test sets.
The rest 250 buildings are added to the gallery as distractors
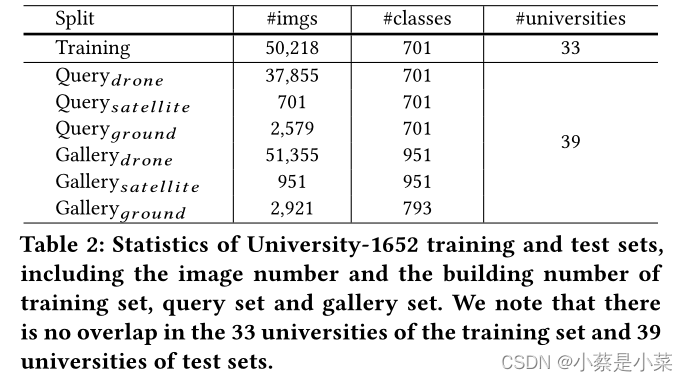
2. 匹配精度评估
之前的匹配论文中大都采用Recall@K作为匹配精确度的评估,但是在我们所做的实验中,在检索库中会出现多张不同视角的正确匹配图像,于是实验引入了AP(average precision)
三、交叉视野匹配
1. Feature Representations
主要工作:比较两类特征
为了进行公平对照,所有的网络结构均采用ResNet-50。
2. NetWork Architecture and loss Function
NetWork Architecture
model (I) and model(II)是为了比较drone和ground视角的优越性
model(III) 是将模型扩展到三分支CNN,在后面的实验中,Fs 和 Fd共享权重
we could view every plac as one class to train a classification model.
Loss Function
Intance loss: The main idea is that a shared classifier could enforce the images of different sources mapping to one shared feature space.
Wshare 是街景图与无人机图在匹配网络中共享权重,这样可以对提取的特征有一个限制(论文实验证实这样做提高了匹配的精确度)
四、实验部分
1. 实验的参数设置
- 采用ResNet-50预训练我们的Image Net(自然数据集1000类)数据集,作为我们的基准模型(参数模型)
- 去掉原始的分类器,在池化层后加入一个512维度的全连接层和一个分类层。
- 这个模型通过SGD(随机梯度下降法)优化,下降梯度为0.9;新增加的层,学习率设置为0.01;其余层的学习率为0.001;下降率是0.75。
- 模型训练时,图片被resize为256*256的大小。
- 相似度匹配:余弦距离用来计算库中的查询和候选图像的相似度,反馈的结果根据相似度进行排列。
2. 实验结果
-
(1) Generic features vs. learned features.
论文中的方法以更少的特征取得更好的匹配结果
-
(2) Ground-view query vs. drone-view query
-
(3)Multiple queries.
实验通过无人机与地面摄像机进行多角度采集图片,两者匹配度进行对比。
四、消融研究/进一步的讨论
-
1.Effect of loss objectives.
-
2. Effect of sharing weights
In our baseline model,Fs and Fd share the weights.
When sharing weights, drone-view images could help regularize the model, and the model, therefore, achieves better Rank@1 and AP accuracy.
-
3. Effect of the image size.
The authers speculate that the larger input size is too different from the size of the pretrained weight on ImageNet, which
is 224 × 224. As a result, the input size of 512 does not perform well.