from __future__ import print_function from functools import reduce class VectorOp(object): def dot(x, y): # 计算两个向量x和y的内积,然后利用reduce求和 return reduce(lambda a, b: a + b, VectorOp.element_multiply(x, y), 0.0) def element_multiply(x, y): return list(map(lambda x_y: x_y[0] * x_y[1], zip(x, y))) def element_add(x, y): # 将两个向量x和y按元素相加 return list(map(lambda x_y: x_y[0] + x_y[1], zip(x, y))) def scala_multiply(v, s): # 将向量v中的每个元素和标量s相乘 return map(lambda e: e * s, v) class Perceptron(object): def __init__(self, input_num, activator): self.activator = activator # 权重向量初始化为0 self.weights = [0.0] * input_num # 偏置项初始化为0 self.bias = 0.0 def __str__(self): return 'weights\t:%s\nbias\t:%f\n' % (self.weights, self.bias) def predict(self, input_vec): # 计算向量input_vec[x1,x2,x3...]和weights[w1,w2,w3,...]的内积 # 然后加上bias return self.activator( VectorOp.dot(input_vec, self.weights) + self.bias) def train(self, input_vecs, labels, iteration, rate): for i in range(iteration): self._one_iteration(input_vecs, labels, rate) def _one_iteration(self, input_vecs, labels, rate): samples = zip(input_vecs, labels) # 对每个样本,按照感知器规则更新权重 for (input_vec, label) in samples: # 计算感知器在当前权重下的输出 output = self.predict(input_vec) # 更新权重 self._update_weights(input_vec, output, label, rate) def _update_weights(self, input_vec, output, label, rate): delta = label - output self.weights = VectorOp.element_add( self.weights, VectorOp.scala_multiply(input_vec, rate * delta)) # 更新bias self.bias += rate * delta def f(x): return 1 if x > 0 else 0 def get_training_dataset(): # 构建训练数据 # 输入向量列表 input_vecs = [[1, 1], [0, 0], [1, 0], [0, 1]] # 期望的输出列表,注意要与输入一一对应 # [1,1] -> 1, [0,0] -> 0, [1,0] -> 0, [0,1] -> 0 labels = [1, 0, 0, 0] return input_vecs, labels def train_and_perceptron(): # 创建感知器,输入参数个数为2(因为and是二元函数),激活函数为f p = Perceptron(2, f) # 训练,迭代10轮, 学习速率为0.1 input_vecs, labels = get_training_dataset() p.train(input_vecs, labels, 10, 0.1) # 返回训练好的感知器 return p if __name__ == '__main__': # 训练and感知器 and_perception = train_and_perceptron() # 打印训练获得的权重 print(and_perception) # 测试 print('1 and 1 = %d' % and_perception.predict([1, 1])) print('0 and 0 = %d' % and_perception.predict([0, 0])) print('1 and 0 = %d' % and_perception.predict([1, 0])) print('0 and 1 = %d' % and_perception.predict([0, 1]))
感知器(作业)
最新推荐文章于 2021-09-11 09:49:25 发布
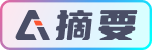