import numpy as np
import matplotlib.pyplot as plt
th1 = np.random.random()
th2 = np.random.random()
th3 = np.random.random()
th0 = np.array([th2, th3])
a = 0.0000001
x = np.array([[2104, 3], [1600, 3], [2400, 3], [1416, 2], [3000, 4]])
t = np.array([400, 330, 369, 232, 540])
eps = 1e-4
e0 = 200
e1 = 200
e2 = 200
i = 0
while e0 >= eps or e1 >= eps or e2 >= eps:
e0 = (np.sum((x[i, 0:1] * th0[i])) + th1 * 1 - t[i])
e1 = e0 * x[i, 0]
e2 = e0 * x[i, 1]
th1 = th1 - a * e0
th2 = th2 - a * e1
th3 = th3 - a * e2
i +=1
print(th1, th2, th3)
随机梯度下降
最新推荐文章于 2024-07-31 14:47:45 发布
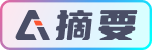