文章来自:https://leonardoaraujosantos.gitbooks.io
原文作者:Leonardo Araujo dos Santos
公众号:机器学习算法工程师
https://blog.csdn.net/byplane/article/details/52422997
https://mp.weixin.qq.com/s/oFWqM9HPhstk7H-GQY0O3g
作者:石文华
编辑:田 旭
翻译
relu层
如何在Python中实现ReLU层?
简而言之,relu层就是输入张量通过一个非线性的relu函数,得到输出,而不改变其空间或者深度信息
从上图可以看出,所有大于0的保持不变,而小于零的变为零。此外,空间信息和深度也是相同的
relu函数作为激活函数,具有以下功能:
1.易于计算(前向/反向传播),采用sigmoid函数作为激活函数时候(指数运算),计算量大,反向传播求误差梯度时,求导涉及除法,计算量相当大,而采用Relu激活函数,整个过程的计算量节省很多。
2.深度模型中受消失梯度的影响要小得多,对于深层网络,sigmoid函数反向传播时,很容易就出现梯度消失的情况(在sigmoid函数接近饱和区时,变换太缓慢,导数趋于0,这种情况会造成信息丢失),从而无法完成深层网络的训练。
3.如果你使用大的学习率,他们可能会不可逆转地死去,因为当一个非常大的梯度流过一个 ReLU 神经元,更新过参数之后,这个神经元再也不会对任何数据有激活现象了。这个神经元的梯度将一直都是0了。
1、前向传播
将所有小于0的数变成0,大于0的数保持不变,空间和深度信息保持不变。
python实现relu的前向传播:
import numpy as np
def relu_forward(x):
"""
Computes the forward pass for ReLU
Input: - x: Inputs, of any shape
Returns a tuple of :(out, cache)
The shape on the output is the same as the input
"""
relu = lambda x: x * (x > 0).astype(float)
out = relu(x)
# Cache input and return outputs
cache = x
return out, cache
if __name__ == "__main__":
input_ = np.random.normal(loc=0.0, scale=1.0, size=10)
print(input_)
print("###########################################")
print(relu_forward(input_))
2、反向传播
在前向传播的时候,我们对每个输入X=[x1,x2,x3]应用了max(0,x)函数,所以在反向传播的时候,小于0的元素,梯度dx等于0:
python实现relu 反向传播:
def relu_backward(dout, cache):
"""
Computes the backward pass for ReLU
Input:
:param dout: Upstream derivatives(上游衍生品) ,of any shape
:param cache: Previous input (used on forward propagation)
:return:
-dx: Gradient with respect to x
"""
# Inititalize dx with None and x with cache
dx, x = None, cache
# Make all positive elements in x equal to dout while all the other elements
dx = dout * (x >= 0)
# Return dx (gradient with respect to x)
return dx
Dropout层
Dropout是一种用于防止神经网络过度拟合的技术,你还可以使用L2正则化防止过拟合。
神经网路是怎么采用L2防止过拟合的呢?
下面是分类的错误率,可以发现使用了dropout之后错误率更低:
和其他正则化技术一样,使用dropout会使得训练损失稍稍恶化,但是模型的泛化能力却更好,因为如果我们的模型过于复杂(更多层或者更多神经元),模型就很可能过拟合,下面是训练和验证集上的损失情况,以及他们中有无dropout情况。
1、dropout工作原理
在训练期间,随机的选择一定比例的神经元,让它停止工作,如下图所示,这样泛化能力更好,因为你的网络层的不同的神经元会学习相同的“概念”。在测试阶段,不需要使用dropout.
2、在哪里使用dropout
通常会在全连接层使用dropout,但也可以在最大池化后使用dropout,从而产生某种图像噪声增强。
3、dropout的实现
为了实现某个神经元的失活,我们在前向传播过程中创建一个掩码(0和1),此掩码应用于训练期间的层的输出,并缓存以供以后在反向传播中使用。如前所述,这个dropout掩码只在训练中使用。
在反向传播中,我们对被激活的神经元感兴趣(我们需要将掩码保存为前向传播),这些被选中的神经元中,使用反向传播,失活的神经元没有可学习的参数,仅仅是输入x,反向传播返回dx。
4、dropout的功效
Dropout背后理念和集成模型很相似。在Drpout层,不同的神经元组合被关闭,这代表了一种不同的结构,所有这些不同的结构使用一个的子数据集并行地带权重训练,而权重总和为1。
如果Dropout层有 n 个神经元,那么会形成2^n个不同的子结构。在预测时,相当于集成这些模型并取均值。这种结构化的模型正则化技术有利于避免过拟合。
Dropout有效的另外一个视点是:由于神经元是随机选择的,所以可以减少神经元之间的相互依赖,从而确保提取出相互独立的重要特征。
5、python实现dropout的前向传播
import numpy as np
def dropout_forward(x, dropout_param):
"""
Performs the forward pass for (inverted) dropout.
:param x: Input data, of any shape
:param dropout_param: A dictionary with the following keys: (p, test/train,seed)
:return: (out, cache)
"""
# Get the current dropout mode, p, and seed
p, mode = dropout_param['p'], dropout_param['mode']
if 'seed' in dropout_param:
np.random.seed(dropout_param['seed'])
# Initialization of outputs and mask
mask = None
out = None
if mode == 'train':
# Create an apply mask (normally p=0.5 for half of neurons), we scale all
# by p to avoid having to multiply by p on backpropagation, this is called
# inverted dropout
mask = (np.random.rand(*x.shape) < p) / p
# Apply mask
out = x * mask
elif mode == 'test':
# During prediction no mask is used
mask = None
out = x
# Save mask and dropout parameters for backpropagation
cache = (dropout_param, mask)
# Convert "out" type and return output and cache
out = out.astype(x.dtype, copy=False)
return out, cache
if __name__ == "__main__":
x = np.random.rand(3, 2)
dropout_param = {'p': 0.5, 'mode': 'train', 'seed': 0}
out, cache = dropout_forward(x, dropout_param)
print(out)
print('++++++++++++++++++++++++++++++++===')
print(cache)
6、python实现dropout的反向传播
def dropout_backward(dout, cache):
"""
Perform the backward pass for (inverted) dropout.
:param dout: Upstream derivatives, of any shape
:param cache: (dropout_param, mask) from dropout_forward.
:return: dx
"""
# Recover dropout parameters(p, mask, mode) from cache
dropout_param, mask = cache
mode = dropout_param['mode']
dx = None
# Back propagate(传播)(Dropout layer has no parameters just input X)
if mode == 'train':
# Just back propagate dout from the neurons that ware used during dropout
dx = dout * mask
elif mode == 'test':
# Disable dropout during prediction/test
dx = dout
return dx
卷积层
简单的说,卷积层所做的工作就是对输入的特征图应用卷积算子,卷积核的个数是输出特征图的深度。下面我们介绍一下相关的参数:
- N:批处理大小(4d张量上的图像数)
- F:卷积层上的滤波器个数
- kW/kH:内核宽度/高度(通常我们使用方形卷积核,kW=kH)
- H/W:图像高度/宽度(通常H=W)
- H’/W’:卷积图像高度/宽度(如果使用适当的填充,则与输入相同)
- Stride:卷积滑动窗口将要移动的像素数。
- Padding:将0添加到图像的边框,以保持输入和输出大小相同。
- Depth:输入特征图的深度(如输入为RGB图像则深度为3)
1、前向传播
在前向传播过程中,我们用不同的过滤器“卷积”输入,每个过滤器将在图像上寻找不同的特征。
没有搞明白这个里面怎么体现不同卷积对应的不同特征的
2、python实现卷积层的前向传播
def conv_forward_naive(x, w, b, conv_param):
"""
Computes the forward pass for the Convolution layer.(Naive)
:param x: Input data of shape (N, C, H, W)
:param w: Filter weights of shape(F, C, HH, WW)
:param b: Biases, of shape(F,)
:param conv_param: A dictionary with the following keys:
- 'stride': How much pixels the sliding window will travel
- 'pad': The number of pixels that will be used to zero-pad the input.
N: Mini-batch size
C: Input depth(ie 3 for RGB images)
H/W: Image height/width
F: Number of filters on convolution layer (will be the output depth)
HH/WW: Kernel Height/width
:returns a tuple of:
- out: Output data, of shape(N, F, H', W') where H' and W' are given by
H' = 1 + (H + 2 * pad - HH)/stride
W' = 1 + (W + 2 * pad - WW)/stride
- cache: (x, w, b, conv_param)
"""
out = None
N, C, H, W = x.shape
F, C, HH, WW = w.shape
# Get parameters
P = conv_param['pad']
S = conv_param['stride']
# Calculate output size, and initialization output volume
H_R = 1 + (H + 2 * P - HH) / S
W_R = 1 + (W + 2 * P - WW) / S
out = np.zeros((N, F, H_R, W_R))
# Pad images with zeros on the border (Used to keep spatial information)
x_pad = np.lib.pad(x, ((0, 0), (0, 0), (P, P), (P, P)), 'constant', constant_values=0)
# Apply the convolution
for n in range(N): # For each element on batch
for depth in range(F):
for r in range(0, H, S): # Slide(滑动) vertically(垂直) taking stride into account
for c in range(0, W, S): # Slide horizontally(水平) taking stride into account
out[n, depth, r/S, c/S] = np.sum(x_pad[n, :, r, r+HH, c: c+WW] * w[depth, :, :, :]) + b[depth]
# Cache parameters and inputs for back propagation and return output volume(卷积)
cache = (x, w, b, conv_param)
return out, cache
3、反向传播
为了更好的理解,这里使用1维卷积来理解卷积层的反向传播,2维的也类似。
输入信号为X=[x0,x1,x2,x3,x4],参数为W=[w0,w1,w2],不使用padding,卷积之后的结果是:Y=[y0,y1,y2],这里Y = X * flip(W),flip可以看作是180度的旋转。
现在我们使用计算图来表示,并且加上一个偏差,通过观察可以发现这个过程跟全连接层类似,不同之处在于卷积核可以使得权重共享。
现在来看反向传播 :
向后追踪计算图,反向传播可以表示为以下的公式:
意味着损失值随着输入进行变化,由上图可以看出。
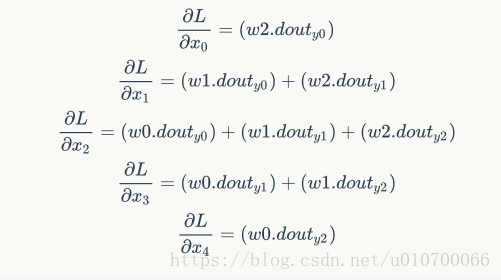
注意:
dX跟X大小相同,所以我们需要进行填充
dout跟Y大小相同,在本例中为3(渐变输入)
为了节省编程工作量,我们将梯度的计算采用卷积的形式
在dX梯度上,所有元素都乘以W,所以我们可能会对W和dout进行卷积操作
1d卷积的输出尺寸计算公式:outputSize=(InputSize-KernelSize+2P)+1,
我们期望的尺寸是3,由于原始输入尺寸是3,并且我们将与也有3个元素的W矩阵进行卷积。所以我们需要用2个零填充输入,之后再进行卷积,就可以得到尺寸为3的输出。
就卷积而言:
根据链式法则,求损失函数对各个参数的偏导:
再次查看从图表中得到的表达式,可以将它们表示为dout和X之间的卷积。同样,由于输出将是3个元素,因此不需要进行填充。
就卷积的计算而言,
如果将X看成是卷积核,而dout看做输入信号,则:
对于偏差,计算将类似于全连接层。 基本上我们每个过滤器有一个偏差,计算如下:

4、python实现卷积的反向传播
def conv_backward_naive(dout, cache):
"""
Computes the backward for the Convolution layer.(Naive)
:param dout: Upstream derivatives.
:param cache: A tuple of (x, w, b, conv_param) as in conv_forward_naive
:returns: a tuple of: (dw, dx, db) gradients
"""
dx, dw, db = None, None, None
x, w, b, conv_param = cache
N, F, H_R, W_R = dout.shape
N, C, H, W = x.shape
F, C, HH, WW = w.shape
P = conv_param['pad']
S = conv_param['stride']
# Do zero padding on x_pad
x_pad = np.lib.pad(x, ((0, 0), (0, 0), (P, P), (P, P)), 'constant', constant_values=0)
# Initialization outputs
dx = np.zeros(x_pad.shape)
dw = np.zeros(w.shape)
db = np.zeros(b.shape)
# Calculate dx, with 2 extra col/row that will be deleted
for n in range(N): # For each element on batch
for depth in range(F): # For each filter
for r in range(0, H, S): # Slide vertically taking stride into account
for c in range(0, W, S): # Slide horizontally taking stride into account
dx[n, :, r: r+HH, c: c+WW] += dout[n, depth, r/S, c/S] * w[depth, :, :, :]
# Calculate db, 1 scalar bias per filter .so it's just a matter of summing
# all elements of dout per filter
for depth in range(F):
db[depth] = np.sum(dout[:, depth, :, :])
return dx, dw, db
5、卷积运算转换为矩阵运算
使用矩阵运算,能够使得运算速度更快,但也会消耗更多的内存。
5.1 Im2col
前面的代码,使用的是for循环来实现卷积,运算速度不够快,在本节中,我们将学习如何使用矩阵运算来实现卷积,首先,卷积是内核过滤器和它移动之后在图像上选择的区域之间的点积,如果我们在内存上扩展所有可能的窗口并将点积作为矩阵运算,运算速度将更快,但内存的消耗也会更大。
例如,输入图片为227*227*3,卷积核为11*11*3,步长为4,padding为0,进行卷积运算的时候,我们可以将卷积核在输入图片上采样的11*11*3大小的像素块(感受野)拉伸为大小为11*11*3=363的列向量,227*227*3大小的图片,又有步长为4,padding为0,卷积之后的宽高计算方式为(227-11)/4)+1=55,所以采样之后得到55*55个11*11*3大小的像素块(感受野),最终可以得到尺寸为363*3025的输出矩阵X_col,(3025由55*55得到,表示有3025个感受野)
总结一下,如何计算im2col输出的大小:
[img_height, img_width, img_channels] = size(img);
newImgHeight = floor(((img_height + 2*P - ksize) / S)+1);
newImgWidth = floor(((img_width + 2*P - ksize) / S)+1);
cols = single(zeros((img_channels*ksize*ksize),(newImgHeight * newImgWidth)));
卷积核也进行类似的伸展,假设有96个大小为11*11*3的卷积核,通过im2col函数之后,得到96*363的矩阵W_col.
将图像和卷积核转换之后,卷积操作就变成了简单的矩阵乘法运算,这个例子中,W_col(96*363)c乘以X_col(363*3025)得到的矩阵是96*3025,最后可以重塑为55*55*96,重塑可以定义一个col2im的函数来实现。
5.2前向传播计算图
下图是前向传播中使用im2col之后的计算图,输入为4*4*3,步长为1,padding为0,卷积核大小为2*2,卷积核个数为1:
前向传播代码如下:
def conv_forward_naive(x, w, b, conv_param):
"""
A naive implementation of the forward pass for a convolutional layer.
The input consists of N data points, each with C channels, height H and width
W. We convolve each input with F different filters, where each filter spans
all C channels and has height HH and width HH.
Input:
- x: Input data of shape (N, C, H, W)
- w: Filter weights of shape (F, C, HH, WW)
- b: Biases, of shape (F,)
- conv_param: A dictionary with the following keys:
- 'stride': The number of pixels between adjacent receptive fields in the
horizontal and vertical directions.
- 'pad': The number of pixels that will be used to zero-pad the input.
Returns a tuple of:
- out: Output data, of shape (N, F, H', W') where H' and W' are given by
H' = 1 + (H + 2 * pad - HH) / stride
W' = 1 + (W + 2 * pad - WW) / stride
- cache: (x, w, b, conv_param)
"""
out = None
pad_num = conv_param['pad']
stride = conv_param['stride']
N,C,H,W = x.shape
F,C,HH,WW = w.shape
H_prime = (H+2*pad_num-HH) // stride + 1
W_prime = (W+2*pad_num-WW) // stride + 1
out = np.zeros([N,F,H_prime,W_prime])
#im2col
for im_num in range(N):
im = x[im_num,:,:,:]
im_pad = np.pad(im,((0,0),(pad_num,pad_num),(pad_num,pad_num)),'constant')
im_col = im2col(im_pad,HH,WW,stride)
filter_col = np.reshape(w,(F,-1))
mul = im_col.dot(filter_col.T) + b
out[im_num,:,:,:] = col2im(mul,H_prime,W_prime,1)
cache = (x, w, b, conv_param)
return out, cache
im2col函数:
def im2col(x,hh,ww,stride):
"""
Args:
x: image matrix to be translated into columns, (C,H,W)
hh: filter height
ww: filter width
stride: stride
Returns:
col: (new_h*new_w,hh*ww*C) matrix, each column is a cube that will convolve with a filter
new_h = (H-hh) // stride + 1, new_w = (W-ww) // stride + 1
"""
c,h,w = x.shape
new_h = (h-hh) // stride + 1
new_w = (w-ww) // stride + 1
col = np.zeros([new_h*new_w,c*hh*ww])
for i in range(new_h):
for j in range(new_w):
patch = x[...,i*stride:i*stride+hh,j*stride:j*stride+ww]
col[i*new_w+j,:] = np.reshape(patch,-1)
return col
5.3反向传播图
使用im2col,计算图类似于具有相同格式的FC层:
,不同之处在于有一堆重塑,转置和im2col块。
关于在反向传播期间的重塑和转置,只需要再次使用另一个重塑或转置来反转它们的操作,需要注意的是,如果在向前传播期间使用行优先进行重塑,反向传播中也要使用行优先。
im2col反向传播操作时。无法实现简单的重塑。这是因为感受野实际上是重合的(取决于步长),所以需要将感受野相交的地方的梯度相加。
反向传播代码:
def conv_backward_naive(dout, cache):
"""
A naive implementation of the backward pass for a convolutional layer.
Inputs:
- dout: Upstream derivatives.
- cache: A tuple of (x, w, b, conv_param) as in conv_forward_naive
Returns a tuple of:
- dx: Gradient with respect to x
- dw: Gradient with respect to w
- db: Gradient with respect to b
"""
dx, dw, db = None, None, None
x, w, b, conv_param = cache
pad_num = conv_param['pad']
stride = conv_param['stride']
N,C,H,W = x.shape
F,C,HH,WW = w.shape
H_prime = (H+2*pad_num-HH) // stride + 1
W_prime = (W+2*pad_num-WW) // stride + 1
dw = np.zeros(w.shape)
dx = np.zeros(x.shape)
db = np.zeros(b.shape)
# We could calculate the bias by just summing over the right dimensions
# Bias gradient (Sum on dout dimensions (batch, rows, cols)
#db = np.sum(dout, axis=(0, 2, 3))
for i in range(N):
im = x[i,:,:,:]
im_pad = np.pad(im,((0,0),(pad_num,pad_num),(pad_num,pad_num)),'constant')
im_col = im2col(im_pad,HH,WW,stride)
filter_col = np.reshape(w,(F,-1)).T
dout_i = dout[i,:,:,:]
dbias_sum = np.reshape(dout_i,(F,-1))
dbias_sum = dbias_sum.T
#bias_sum = mul + b
db += np.sum(dbias_sum,axis=0)
dmul = dbias_sum
#mul = im_col * filter_col
dfilter_col = (im_col.T).dot(dmul)
dim_col = dmul.dot(filter_col.T)
dx_padded = col2im_back(dim_col,H_prime,W_prime,stride,HH,WW,C)
dx[i,:,:,:] = dx_padded[:,pad_num:H+pad_num,pad_num:W+pad_num]
dw += np.reshape(dfilter_col.T,(F,C,HH,WW))
return dx, dw, db
col2im函数:
def col2im(mul,h_prime,w_prime,C):
"""
Args:
mul: (h_prime*w_prime*w,F) matrix, each col should be reshaped to C*h_prime*w_prime when C>0, or h_prime*w_prime when C = 0
h_prime: reshaped filter height
w_prime: reshaped filter width
C: reshaped filter channel, if 0, reshape the filter to 2D, Otherwise reshape it to 3D
Returns:
if C == 0: (F,h_prime,w_prime) matrix
Otherwise: (F,C,h_prime,w_prime) matrix
"""
F = mul.shape[1]
if(C == 1):
out = np.zeros([F,h_prime,w_prime])
for i in range(F):
col = mul[:,i]
out[i,:,:] = np.reshape(col,(h_prime,w_prime))
else:
out = np.zeros([F,C,h_prime,w_prime])
for i in range(F):
col = mul[:,i]
out[i,:,:] = np.reshape(col,(C,h_prime,w_prime))
return out
col2im_back函数:
def col2im_back(dim_col,h_prime,w_prime,stride,hh,ww,c):
"""
Args:
dim_col: gradients for im_col,(h_prime*w_prime,hh*ww*c)
h_prime,w_prime: height and width for the feature map
strid: stride
hh,ww,c: size of the filters
Returns:
dx: Gradients for x, (C,H,W)
"""
H = (h_prime - 1) * stride + hh
W = (w_prime - 1) * stride + ww
dx = np.zeros([c,H,W])
for i in range(h_prime*w_prime):
row = dim_col[i,:]
h_start = (i / w_prime) * stride
w_start = (i % w_prime) * stride
dx[:,h_start:h_start+hh,w_start:w_start+ww] += np.reshape(row,(c,hh,ww))
return dx
5.4小案例
这里使用X[3x3]与W [2x2]进行卷积的简单示例,来帮助大家的理解。
池化层
池化层用于减少特征空间的维度,但是不会改变特征图的深度,它的左右有如下的几点:
- 减少了特征空间信息,内存的使用更少,计算速度也将快
- 防止过拟合
- 引入了位移不变性,更关注是否存在某些特征而不是特征具体的位置。比如最常见的max pooling,因为取一片区域的最大值,所以这个最大值在该区域内无论在哪,max-pooling之后都是它,相当于对微小位移的不变性。
使用的最多的是最大池化,如下图所示,最大池化像卷积核一样滑动窗,并在窗口上获得最大值作为输出。
参数有:
1. 输入:H1 x W1 x Depth_In x N.
2. 步长:控制窗口滑动的像素数量的标量。
3. K:内核大小
4. 输出:H2 x W2 x Depth_Out x N:
由于池化层上没有可学习的参数,所以它的反向传播更简单。
最大池在其计算图上使用一系列最大节点。因此,最大池化层的反向传播包含在前向传播期间选择的所有元素和dout的掩码之间的乘积。
换句话说,最大池层的输入的梯度是由前向传播选择的元素的梯度和0组成的张量。
1、python实现池化层的前向传播
池化层上的窗口移动机制与卷积核相同,不同之处在于池化层的窗口是选择最大值。
import numpy as np
def max_pool_forward_naive(x, pool_param):
"""
Compute the forward max pooling (naive way)
:param x: 4d Input tensor, of shape (N, C, H, W)
:param pool_param: dictionary with the following keys:
- 'pool_heigh/width': Sliding window height/width
- 'stride': Sliding moving distance
N: Mini-batch size
C: Input depth (ie 3 for RGB images)
H/W: Image height/width
:returns: a tuple of (out, cache)
"""
# Get input tensor and parameter data
N, C, H, W = x.shape
S = pool_param['stride']
# Consider H_P and W_P as the sliding window height and width
H_P = pool_param['pool_height']
W_P = pool_param['pool_height']
# Calculate output size
out = None
HH = 1 + (H - H_P) / S
WW = 1 + (W - W_P) / S
out = np.zeros((N, C, HH, WW))
# Calculate output
for n in range(N): # For each element on batch
for depth in range(C): # For each input depth
for r in range(0, H, S): # Slide vertically taking stride into account
for c in range(0, W, S): # Slide horizontally taking stride into account
out[n, depth, r/S, c/S] += np.max(x[n, depth, r: r+H_P, c: c+W_P])
# Return output and save inputs and parameters to cache
cache = (x, pool_param)
return out, cache
2、python实现池化层的反向传播
def max_pool_backward_naive(dout, cache):
"""
Compute the backward propagation of max pooling (naive way)
:param dout: Upstream derivatives(衍生物), same size as cached x
:param cache: A tuple of (x, pool_param) as in the forward pass.
:returns:
- dx: Gradient with respect to x
"""
# Get data back from cache
x, pool_param = cache
# Get input tensor and parameter
N, C, H, W = x.shape
S = pool_param['stride']
# Consider H_P and W_P as the sliding window height and width
H_P = pool_param['pool_height']
W_P = pool_param['pool_height']
N, C, HH, WW = dout.shape
# Initialize dx
dx = None
dx = np.zeros(x.shape)
# Calculate dx (mask * dout)
for n in range(N): # For each element on batch
for depth in range(C): # For each input depth
for r in range(HH): # Slide vertically taking stride on the fly
for c in range(WW): # Slide horizontally taking stride on the fly
# Get window and calculate the mask
x_pool = x[n, depth, r * S: r * S + H_P, c * S: c * S + W_P]
mask = (x_pool == np.max(x_pool))
# Calculate mask * dout
dx[n, depth, r*S: r*S+H_P, c*S: c*S+W_P] = mask*dout[n, depth, r, c]
# Return dx
return dx