Week 4主要讲解了神经网络(Neural Network, NN),用于处理更复杂的非线性回归问题。
对于non-linear hypothesis,我们可以构建多项式来搭建模型,但是如果有很多features的话,logistic regression的代价会很昂贵,神经网络就可以用来处理更复杂的非线性模型。
Model Representation
通过神经网络来表达假设函数。基础的神经由树突(dendrites)作为输入渠道,轴突(axons)作为输出渠道。基础的神经网络也一般由三层input layer, hidden layer, 和output layer组成。输入层包含由各个feature构成的units,输出层就是假设函数的预测结果,通过层层的隐藏层映射,我们可以使神经网络完成更复杂的模型。
在神经网络中,我们在classification中使用sigmoid(logistic) activation function 1 1 + e − θ T x \frac{1}{1+e^{-θ^Tx}} 1+e−θTx1 进行映射,θ parameters也被称作weights,每一层都有它自己的weights Θ ( j ) \Theta^{(j)} Θ(j)。如果第j层有 s j s_j sj个units,第j + 1层有 s j + 1 s_{j+1} sj+1个units,那么 Θ ( j ) \Theta^{(j)} Θ(j)是个 s j + 1 s_{j+1} sj+1 x ( s j + 1 ) (s_j + 1) (sj+1)大小的矩阵。“+1”是因为bias unit的存在。
在输出层前的每层都有一个
a
0
(
j
)
a_0^{(j)}
a0(j),值永远为1,被称作bias unit,在计算下一层的输出的时候,要记得加上它。
在神经网络中,前馈传播算法(Feedforward Propagation Algorithm)就是从输入层的activation开始,向前来到第一个隐藏层,然后到第二个隐藏层,最终到输出层的过程。在最后一步到输出层的过程,和逻辑回归一样。
从第二层开始,
z
(
j
)
=
Θ
(
j
−
1
)
a
(
j
−
1
)
z^{(j)} = \Theta^{(j-1)}a^{(j-1)}
z(j)=Θ(j−1)a(j−1)
第j层的向量,
a
(
j
)
=
g
(
z
(
j
)
)
a^{(j)} = g(z^{(j)})
a(j)=g(z(j))
在输出层j+1,
h
Θ
(
x
)
=
a
(
j
+
1
)
=
g
(
z
(
j
+
1
)
)
h_\Theta(x) = a^{(j+1)} = g(z^{(j+1)})
hΘ(x)=a(j+1)=g(z(j+1)),
Θ
(
j
)
\Theta^{(j)}
Θ(j)只有一行
Application
可以用神经网络来实现一些简单的逻辑映射如AND,OR,NOT,也可以实现更复杂的逻辑映射如XOR,XNOR。
Exercise 3:实现多分类与神经网络 - Matlab
通过多分类与神经网络方法,分别实现手写数字的识别。
1. Training Data
数据包含5000个手写数字的样本,每个样本是一个20x20像素的灰度图,并被转化为一个400维度的向量。随机选取100个样本展示如图。
2. Multi-class Classification
将Ex2的逻辑回归模型拓展成由多个one-vs-all的逻辑回归模型组成的多分类模型。
2.1 Regularized Logic Regression
如Ex2中一样,在lrCostFunction.m文件中补充完整regularized logic regression的cost function和gradient。
J = (log(sigmoid(theta' * X')) * y + log(1 - sigmoid(theta' * X')) * (1 - y)) / (-m);
grad = (X' * (sigmoid(X * theta) - y)) / m;
2.2 One-vs-all Classification
通过训练多个regularized logistic regression来实现one-vs-all的多分类classification。通过fmincg函数(不是Ex2中的fminunc函数)来训练classifier,fmincg与fminunc类似,但是在处理大量parameters的时候比fminunc效率更高。在oneVsAll.m文件中完成对10个类别(数字1-10)分别地训练classifier。我们一共有5000个样本,每个样本有400个features,所以初始化θ为一个有401个元素的vector。all_theta是一个10 x 401的矩阵,每一行代表一个classifier的θ参数。
function [all_theta] = oneVsAll(X, y, num_labels, lambda)
%ONEVSALL trains multiple logistic regression classifiers and returns all
%the classifiers in a matrix all_theta, where the i-th row of all_theta
%corresponds to the classifier for label i
% [all_theta] = ONEVSALL(X, y, num_labels, lambda) trains num_labels
% logistic regression classifiers and returns each of these classifiers
% in a matrix all_theta, where the i-th row of all_theta corresponds
% to the classifier for label i
% Some useful variables
m = size(X, 1);
n = size(X, 2);
% You need to return the following variables correctly
all_theta = zeros(num_labels, n + 1);
% Add ones to the X data matrix
X = [ones(m, 1) X];
% ====================== YOUR CODE HERE ======================
% Instructions: You should complete the following code to train num_labels
% logistic regression classifiers with regularization
% parameter lambda.
%
% Hint: theta(:) will return a column vector.
%
% Hint: You can use y == c to obtain a vector of 1's and 0's that tell you
% whether the ground truth is true/false for this class.
%
% Note: For this assignment, we recommend using fmincg to optimize the cost
% function. It is okay to use a for-loop (for c = 1:num_labels) to
% loop over the different classes.
%
% fmincg works similarly to fminunc, but is more efficient when we
% are dealing with large number of parameters.
%
% Example Code for fmincg:
%
% % Set Initial theta
% initial_theta = zeros(n + 1, 1);
%
% % Set options for fminunc
% options = optimset('GradObj', 'on', 'MaxIter', 50);
%
% % Run fmincg to obtain the optimal theta
% % This function will return theta and the cost
% [theta] = ...
% fmincg (@(t)(lrCostFunction(t, X, (y == c), lambda)), ...
% initial_theta, options);
%
initial_theta = zeros(n + 1, 1);
options = optimset('GradObj', 'on', 'MaxIter', 50);
for c = 1 : num_labels
all_theta(c, :) = fmincg(@(t)(lrCostFunction(t, X, (y == c),lambda)), initial_theta, options);
end
% =========================================================================
end
2.3 One-vs-all Prediction
在训练好classifier后就可以用其进行预测,并评估这个模型。在predictOneVsAll.m中补充预测函数的代码。这行补充的代码是求矩阵(X * all_theta’)中每行的最大值,并用p记录矩阵每行代码的最大值索引。[~, index] = max()中,~表示最大值,index表示最大值的位置。X是一个5000 x 401的矩阵,all_theta是一个10 x 401的矩阵,所以通过X * all_theta’来获取一个5000 x 10的矩阵,每一列表示5000个样本在某个classifier下的预测结果,每一行表示1一个样本在10个classifier下的预测结果。通过设置max函数参数来获取每行的最大值的位置。
function p = predictOneVsAll(all_theta, X)
%PREDICT Predict the label for a trained one-vs-all classifier. The labels
%are in the range 1..K, where K = size(all_theta, 1).
% p = PREDICTONEVSALL(all_theta, X) will return a vector of predictions
% for each example in the matrix X. Note that X contains the examples in
% rows. all_theta is a matrix where the i-th row is a trained logistic
% regression theta vector for the i-th class. You should set p to a vector
% of values from 1..K (e.g., p = [1; 3; 1; 2] predicts classes 1, 3, 1, 2
% for 4 examples)
m = size(X, 1);
num_labels = size(all_theta, 1);
% You need to return the following variables correctly
p = zeros(size(X, 1), 1);
% Add ones to the X data matrix
X = [ones(m, 1) X];
% ====================== YOUR CODE HERE ======================
% Instructions: Complete the following code to make predictions using
% your learned logistic regression parameters (one-vs-all).
% You should set p to a vector of predictions (from 1 to
% num_labels).
%
% Hint: This code can be done all vectorized using the max function.
% In particular, the max function can also return the index of the
% max element, for more information see 'help max'. If your examples
% are in rows, then, you can use max(A, [], 2) to obtain the max
% for each row.
%
[~, p] = max(X * all_theta', [], 2);
% =========================================================================
end
pred = predictOneVsAll(all_theta, X);
fprintf('\nTraining Set Accuracy: %f\n', mean(double(pred == y)) * 100);
3. Neural Networks
利用前馈传播算法(Feedforward Propagation Algorithm)使用weights,也就是 Θ \Theta Θ来预测。Ex4会实现反向传播算法(BackPropagation Algorithm)。
3.1 Model Representation
构建一个三层神经网络,input layer有400个units(不包括一直输出1的bias unit),hidden layer有25个units,output layer有10个units对应10个数字类别。已经提供了训练好的25 x 401大小的
Θ
(
1
)
\Theta^{(1)}
Θ(1)与10 x 26大小的
Θ
(
2
)
\Theta^{(2)}
Θ(2),例如
Θ
(
1
)
\Theta^{(1)}
Θ(1)的第一行对应着第二层的第一个单位bias unit。
3.2 Feedforward Propagation and Prediction
在predict.m中完善前馈传播算法,并进行预测。注意需要扩充X的第一列,并且每次传到下一层的时候加上bias unit。
function p = predict(Theta1, Theta2, X)
%PREDICT Predict the label of an input given a trained neural network
% p = PREDICT(Theta1, Theta2, X) outputs the predicted label of X given the
% trained weights of a neural network (Theta1, Theta2)
% Useful values
m = size(X, 1);
num_labels = size(Theta2, 1);
% You need to return the following variables correctly
p = zeros(size(X, 1), 1);
% ====================== YOUR CODE HERE ======================
% Instructions: Complete the following code to make predictions using
% your learned neural network. You should set p to a
% vector containing labels between 1 to num_labels.
%
% Hint: The max function might come in useful. In particular, the max
% function can also return the index of the max element, for more
% information see 'help max'. If your examples are in rows, then, you
% can use max(A, [], 2) to obtain the max for each row.
%
% feedforward propagation
X = [ones(m, 1), X];
a_super_2 = sigmoid(Theta1 * X');
a_super_2 = [ones(1, m); a_super_2]; % add bias unit
a_super_3 = sigmoid(Theta2 * a_super_2);
% prediction
[~, p] = max(a_super_3', [], 2);
% =========================================================================
end
随机选取一个样本,进行预测并展示图片。预测结果与图片显示相同。
% Randomly permute examples
rp = randi(m);
% Predict
pred = predict(Theta1, Theta2, X(rp,:));
fprintf('\nNeural Network Prediction: %d (digit %d)\n', pred, mod(pred, 10));
% Display
displayData(X(rp, :));
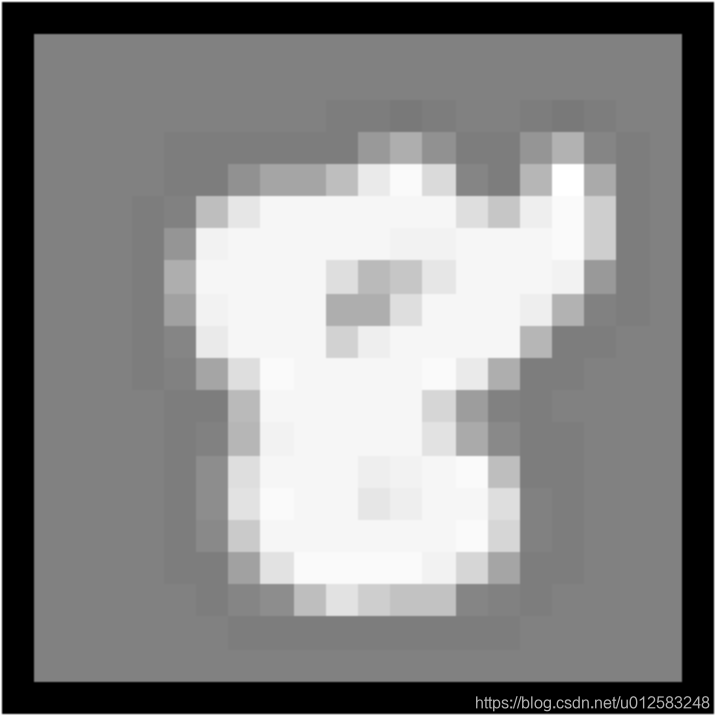
作业代码参考:
https://www.cnblogs.com/hapjin/p/6085278.html
https://www.cnblogs.com/hapjin/p/6085489.html
Ex3全部代码已上传Github。