ai用于定位?
Artificial intelligence (AI), the broad discipline of creating intelligent machines, has been very well established in the everyday technology and adopted in popular culture more than any other technological achievement. Daily, we interact with intelligent systems while sending an email, unlocking our phones, using social media, receiving recommendations for shopping, travel, entertainment etc. AI is using technology like machine learning (ML)*, natural language processing (NLP)*, computer vision etc., in order to carry out tasks which normally require human intelligence, for example problem solving or learning. But how much can we trust AI for applications that are critical like healthcare, aviation or security? To illustrate the importance of this question, imagine a computer vision system providing false information on skin cancer detection, or a self-driving car unable to detect an obstacle in front of it. Such critical applications require absolutely rapid identification, timely responses, reliability and security assurance, topics that are currently addressed by researchers.
人工智能(AI)是创建智能机器的广泛学科,已经在日常技术中得到了很好的确立,并且在流行文化中的应用比其他任何技术成就都多。 每天,我们与智能系统进行交互,同时发送电子邮件,解锁手机,使用社交媒体,接收购物,旅行,娱乐等方面的建议。人工智能正在使用机器学习(ML)*,自然语言处理(NLP)*,计算机视觉等,以执行通常需要人类智能的任务,例如解决问题或学习。 但是,对于医疗保健,航空或安全等至关重要的应用程序,我们可以信任AI多少呢? 为了说明这个问题的重要性,可以想象一下一个计算机视觉系统,它提供有关皮肤癌检测的虚假信息,或者是一辆自动驾驶汽车,无法检测到前方的障碍物。 此类关键应用程序需要绝对快速的识别,及时的响应,可靠性和安全性保证,这是研究人员当前正在解决的主题。
EURECOM’s professor Dr. Maria A. Zuluaga, expert in AI and Data Science will guide us through, on what is AI and how it can be of use for critical applications like healthcare.
EURECOM的教授AI和数据科学专家 Maria A. Zuluaga博士将指导我们学习什么是AI以及如何将其用于医疗保健等关键应用。
Q. What is artificial intelligence and machine learning, terms used so often and sometimes interchangeably?
问:什么是人工智能和机器学习,这些术语经常且有时可互换使用?
MAZ: Defining Artificial Intelligence is not easy, taking into account that the field is vast and more of a multidisciplinary entity. In a sense, AI is an umbrella discipline that covers anything related to making machines smart, i.e. a car, a TV or a software application. Along with AI we often come across another field, Machine Learning (ML), a subset of AI, referring to systems that can learn by themselves . Contrary to classic programming, which is designed to carry out specific tasks, predetermined a priori, ML algorithms are trained on a given set of data, in order to learn how to give answers to complex questions. There are several types of ML algorithms, such as supervised*, unsupervised*, reinforcement* and deep learning* as indicative examples.
MAZ:考虑到该领域广阔且更多是多学科实体,定义人工智能并不容易。 从某种意义上说,人工智能是一门总括的学科,涵盖了与使机器智能化(即汽车,电视或软件应用程序)有关的所有内容。 与AI一起,我们经常遇到另一个领域,即机器学习(ML) ,这是AI的子集,指的是可以自行学习的系统。 与旨在执行特定任务的经典编程相反,预先确定的先验ML算法在给定的数据集上进行训练,以便学习如何给出复杂问题的答案。 机器学习算法有几种类型,例如指导性*,非指导性*,强化*和深度学习*。
*See Glossary below for AI beginner-friendly explanations
*有关AI初学者友好的解释,请参见下面的词汇表
Q. What are the challenges you face in particular for AI on critical applications like healthcare and what is your approach to address them?
问:您对于在诸如医疗保健等关键应用上的AI尤其面临着哪些挑战?您将如何应对这些挑战?
MAZ. My research focuses on the successful use of ML techniques in critical domains facing high-risk decisions, such as healthcare. Critical applications are mostly challenged by three limiting factors: data complexity, low error tolerance and reliability. I address these challenges through the development of robust ML algorithms relying on the human-in-the-loop learning principle. In other words, the goal is not to develop AI software systems that will replace physicians, but to give them tools that will help them make more informed, relevant and fairer decisions, while keeping them in the loop. One major difficulty in medicine is that data, are by definition heterogeneous, complex and difficult to use. This is why traditional ML techniques are not particularly suited. In critical applications, quality and quantity of input data are important to obtain correct results. There is a need for methods based on interactivity and on evaluating the uncertainty of the results. Especially, we are able to reduce the dimensionality of the data needed, in order for ML algorithms to be trained efficiently and still provide robust results. This is a very useful contribution particularly for medical imaging and diagnosis, where available data for training algorithms do not exist in high quantity and require very specialised annotation processes.
玛兹 我的研究重点是在面临高风险决策(例如医疗保健)的关键领域成功使用机器学习技术。 关键应用程序主要受到三个限制因素的挑战: 数据复杂性,低容错性和可靠性。 我通过开发依赖于在环学习原理的健壮的ML算法来应对这些挑战。 换句话说,目标不是开发替代医生的AI软件系统,而是为他们提供工具,以帮助他们做出更明智,更相关和更公平的决策,同时保持他们的生命周期。 医学上的一个主要困难是,按照定义,数据是异构的,复杂的并且难以使用。 这就是为什么传统机器学习技术不是特别适合的原因。 在关键应用中,输入数据的质量和数量对于获得正确的结果很重要。 需要基于交互性和评估结果不确定性的方法。 尤其是,我们能够减少所需数据的维数,以便高效地训练ML算法并仍然提供可靠的结果。 尤其对于医学成像和诊断而言,这是一个非常有用的贡献,因为用于培训算法的可用数据并不大量存在,需要非常专门的注释过程。
Q. Could you give us examples of real-life critical applications of your research projects?
问:您能否举例说明您的研究项目在现实生活中的关键应用?
MAZ. Currently, in collaboration with University College London (UCL), we are developing interactive ML techniques, in order to ensure the timely medical diagnosis of critical situations for multiple sclerosis (MS) patients. Brain lesions detection for MS patients is a quite well known process. The challenge now is to be able to automatically detect, when such lesions touch or cross blood vessels in the brain, a situation that requires immediate medical attention. The cerebral blood vessel tree is so complex and really hard to annotate purely by using an algorithm, so humans are efficiently feeding the algorithm for high level of precision in annotations.
玛兹 当前,我们正在与伦敦大学学院(UCL)合作开发交互式ML技术,以确保对多发性硬化症(MS)患者的危急情况进行及时的医学诊断。 MS患者的脑部病变检测是众所周知的过程。 现在的挑战是,当这种病变接触或穿越大脑中的血管时,能够自动检测需要立即就医的情况。 脑血管树是如此复杂,并且真的很难仅通过使用算法来进行注释,因此人类正在高效地为算法提供注释,以实现较高的注释精度。
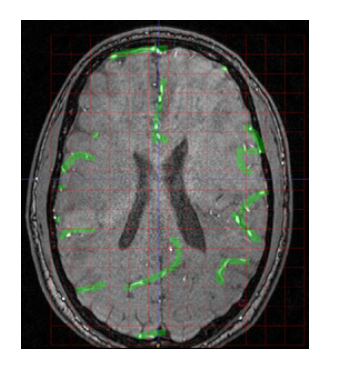
Another application we are currently pursuing, goes to the direction of personalised medicine. The idea is to use wearable devices, such as watches, t-shirts, etc. and analyse the collected physiological data of patients, in order to provide health recommendations. For example, in collaboration with clinicians in Monaco, we run a project to provide support to patients with chronic diseases, i.e. cardiac diseases, intersecting their data, with information gathered from smart city devices. Based on data for traffic, pollution etc. the algorithm will recommend to them personalised tips for their daily activities, i.e. how safe it is to go outside due to pollution, how long they should walk, whether to avoid physical strain in a humid weather etc.
我们目前正在追求的另一种应用是个性化医学。 想法是使用可穿戴设备,例如手表,T恤衫等,并分析收集到的患者生理数据,以提供健康建议。 例如,我们与摩纳哥的临床医生合作,开展了一个项目,向患有慢性疾病(即心脏病)的患者提供支持,并与他们的数据相交,并从智能城市设备中收集信息。 基于交通,污染等数据,该算法将向他们推荐个性化的日常活动提示,例如因污染而出门的安全性,他们应该走多长时间,是否应避免在潮湿的天气下身体疲劳等。 。
Furthermore, note that the methods we develop are generic and transversal, and can be relevant to all critical application domains sharing the same difficulties. For example, we are working on a project with Orange (see recent publication [1]), to create an automated system to detect anomalies while monitoring signals on their IT system, allowing damage prevention and timely reaction to possible network issues. To reliably detect such anomalies, we use a method called “auto-encoders”, a type of neural network* which is able to encode a dataset and decode it in a lower dimension, keeping the most valuable information for the reconstruction. What is considered normality in data is much easier to model and because of the larger amount of normal data available, we have a small reconstruction error. On the contrary, anomalies when decoded exhibit a significantly higher estimation error leading to their successful detection.
此外,请注意,我们开发的方法是通用的和横向的,并且可能与共享相同困难的所有关键应用程序域有关。 例如,我们正在与Orange合作一个项目( 请参阅最新出版物[1] ),以创建一个自动系统来检测异常,同时监视其IT系统上的信号,从而防止损坏并及时响应可能的网络问题。 为了可靠地检测到此类异常,我们使用一种称为“自动编码器”的方法,这是一种神经网络*,能够对数据集进行编码并在较低的维度上对其进行解码,从而保留了最有价值的信息以进行重建。 数据中被认为是正态性的模型更容易建模,并且由于可用的正态数据量较大,因此重建误差较小。 相反,异常在解码时表现出明显更高的估计误差,从而导致其成功检测。
Q. What do you think of the future of AI and its technologies?
问:您如何看待AI及其技术的未来?
MAZ. There has been an exploding hype recently around AI and ML techniques and we are currently reaching a plateau for the field’s milestones. I think it is time to reflect now a bit deeper and look into what we can really achieve in the future through AI. Also, there has been a massive number of publications on the field and some unfortunate examples of mistakes found in studies. Therefore, it is highly important to increase our caution in reviewing and keep a critical mind in order to keep the essential and move forward based on more solid grounds, towards both theoretical and technical advancements on AI.
玛兹 近年来,有关AI和ML技术的炒作激增,而我们目前正达到该领域里程碑的平台。 我认为现在是时候进行更深入的思考,并探讨我们将来通过AI可以真正实现的目标。 同样,在该领域也有大量出版物,并且在研究中发现了一些不幸的错误例子。 因此,提高我们的审慎性和保持批判性思维以保持必要性并在更坚实的基础上前进,朝着AI的理论和技术发展迈进非常重要。
by Dora Matzakou for EURECOM
由Dora Matzakou为EURECOM
[1] J. Audibert, P. Michiardi, F. Guyard, S. Marti. “USAD: UnSupervised Anomaly Detection on Multivariate Time Series”. In: Proceedings of the 26th ACM SIGKDD Conference on Knowledge Discovery and Data Mining (KDD’20), August 23–27 2020, Virtual Event
[1] J. Audibert,P。Michiardi,F。Guyard,S。Marti。 “ USAD:多元时间序列上的无监督异常检测”。 于:2020年8月23日至27日,第26届ACM SIGKDD知识发现和数据挖掘会议论文集(KDD'20)

翻译自: https://medium.com/swlh/towards-ai-safely-used-for-our-health-here-is-how-5810d6101c8e
ai用于定位?