dis 密集光流
内部AI (INSIDE AI)
Before we get into what self-supervised attention means, let’s get an intuition of optical flow estimation and how it serves as an approach for tracking objects by both humans and computer vision systems.
在了解自我监督注意力的含义之前,让我们先了解一下光流估计,以及它如何用作人类和计算机视觉系统跟踪对象的方法。
It is a consensus that object tracking is a fundamental ability that is developed by a human baby at an early age of about two to three months. However, at the level of neurophysiology, the actual working mechanism of the human visual system still remains somewhat obscure. Similar to the human visual system, computer vision systems also widely use tracking for various applications like video surveillance and autonomous driving. The objective of a tracking algorithm is to relocate a particular set of objects in a given video sequence that it has identified in the initial frames. In the research literature related to tracking, it is studied under two major categories namely Visual Object Tracking (VOT) and Semisupervised Video Object Segmentation (Semi-VOS). The first one (VOT) aims to track objects by relocalizing object bounding boxes throughout the video sequence. Whereas the latter (Semi-VOS) tracks objects at a more fine-grained level through a pixel-level segmentation mask. In this blog, we will discuss the original idea behind the latter approach i.e Dense Optical Flow Estimation and how this kind of dense tracking approach is achieved through self-supervised attention mechanisms.
人们一致认为,对象跟踪是人类婴儿在大约两到三个月的早期发展出的一项基本能力。 然而,在神经生理学的水平上,人类视觉系统的实际工作机制仍然有些模糊。 类似于人类视觉系统,计算机视觉系统还广泛地将跟踪用于各种应用,例如视频监视和自动驾驶。 跟踪算法的目的是在给定视频序列中已在初始帧中识别出的一组特定对象进行重新定位。 在与跟踪有关的研究文献中,对它进行了两个主要类别的研究,即视觉对象跟踪(VOT)和半监督视频对象分割(Semi-VOS)。 第一个(VOT)旨在通过在整个视频序列中重新定位对象边界框来跟踪对象。 后者(Semi-VOS)通过像素级分割蒙版以更细粒度的级别跟踪对象。 在此博客中,我们将讨论后一种方法(即密集光流估计)背后的原始思想,以及如何通过自我监督的注意力机制实现这种密集跟踪方法。
密集光流估计 (Dense Optical Flow Estimation)
Dense optical flow is one of the categories of the concept of Optical flow. Optical flow can be defined as the motion of objects between consecutive frames of a video sequence, as a consequence of relative motion between the object and camera. To explain the same in a scientific language, we can say that optical flow is the distribution of apparent velocities of movement of brightness patterns in an image that arises from the relative motion of objects and the viewer. Optical flow is studied as Sparse optical flow and Dense optical flow. Sparse optical flow derives flow vectors of only a few interesting pixels in the frame that either depict some edge or corner of an object. On the other hand, Dense optical flow derives flow vectors of all the pixels in a given frame, thus giving a higher accuracy at the cost of more computation and less speed.
密集光流是“光流”概念的类别之一。 由于对象和摄像机之间的相对运动,光流可以定义为视频序列的连续帧之间的对象运动。 为了用科学语言解释同样的情况,我们可以说光流是图像中亮度模式运动的视在速度分布,该速度是由于物体和观看者的相对运动而产生的。 研究光流为稀疏光流和密集光流 。 稀疏的光流仅得出帧中仅描述几个有趣像素的流矢量,这些像素描述了对象的某些边缘或拐角。 另一方面, 密集光流可得出给定帧中所有像素的流矢量,从而以更高的计算量和更快的速度为代价提供更高的精度。
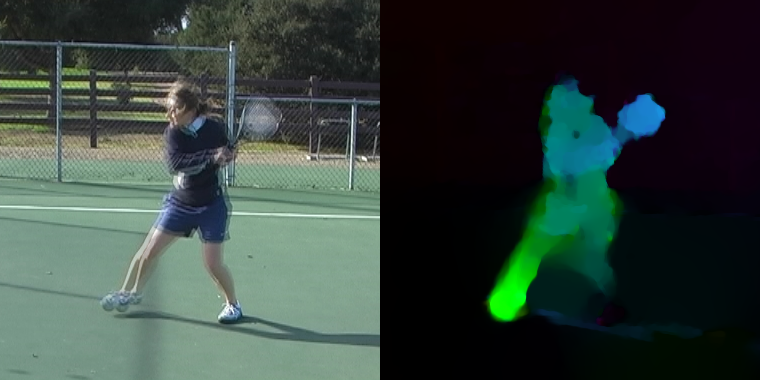
Dense optical flow computes one optical flow vector per pixel for every frame in the video sequence. Unlike sparse optical flow, this approach gives a more suitable output for applications such as video segmentation and structural learning from motion. Dense optical flow can be implemented by various methods. Among them, one of the simplest to use algorithm is the Farneback method. It is based on Gunner Farneback’s algorithm which is explained in “Two-Frame Motion Estimation Based on Polynomial Expansion” by Gunner Farneback in 2003. OpenCV provides the code function to this algorithm to find the dense optical flow. For a quick experience of what Farneback’s algorithm is, run the following code snippet.
密集光流为视频序列中的每一帧每个像素计算一个光流矢量。 与稀疏光流不同,此方法可为视频分割和从运动中学习结构等应用提供更合适的输出。 密集的光流可以通过各种方法来实现。 其中,最简单使用的算法之一是Farneback方法。 它是基于枪手Farneback的算法,这是在“两帧运动估测基于多项式扩张”的枪手Farneback在2003年解释的OpenCV提供的代码功能,该算法找到密集的光流。 为了快速了解Farneback的算法,请运行以下代码段。
After running the above code, you will get the following output (right side) in a video(Dense-optical-flow.mp4)
运行上面的代码后,您将在视频(Dense-optical-flow.mp4)中获得以下输出(右侧)
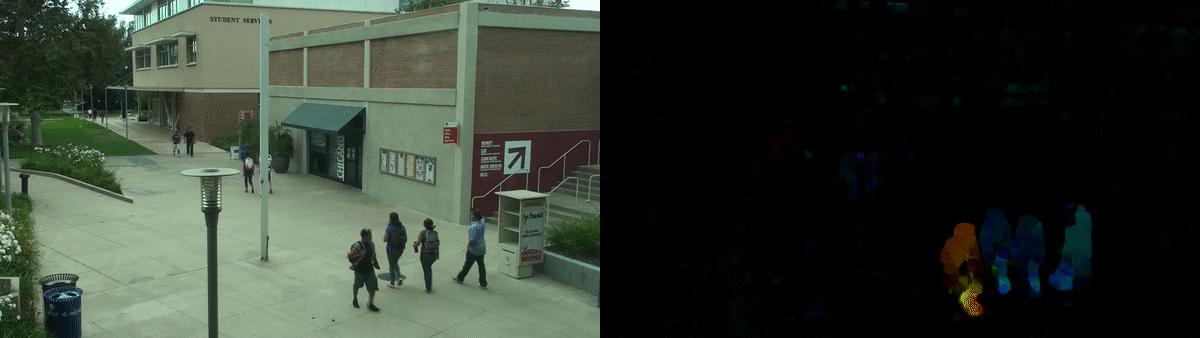
The Farneback algorithm is an effective technique to estimate the motion of certain image features by comparing two consecutive frames from a video sequence. The algorithm first uses the polynomial expansion transform to approximate the windows of image frames through the quadratic polynomials. Polynomial expansion transform is a signal transform designed exclusively in the spatial domain and can be used for signals of any dimensionality. The method observes the translation of the polynomial transforms to estimate displacement fields from polynomial expansion coefficients. This method then computes the dense optical flow after a series of iterative refinements. In the implementation code, the algorithm computes the direction and magnitude of optical flow from a two-channel array of flow vectors (dx/dt, dy/dt). The computed direction and magnitude are then visualized by the value of HSV color representation which is set to a maximum of 255 for optimal visibility.
Farneback算法是一种有效的技术,可以通过比较视频序列中的两个连续帧来估计某些图像特征的运动。 该算法首先使用 多项式展开变换通过二次多项式逼近图像帧的窗口。 多项式展开变换是专门在空间域中设计的信号变换,可用于任何维数的信号。 该方法观察多项式变换的平移,以根据多项式展开系数估计位移场。 然后,该方法在一系列迭代优化之后计算密集的光流。 在实现代码中,该算法从两通道流量矢量数组(dx / dt,dy / dt)计算光流的方向和大小。 然后,通过设置为最大可见度的最大值为255的HSV颜色表示的值来可视化计算出的方向和大小。
深度学习用于密集光流估计 (Deep Learning for Dense Optical Flow Estimation)
Historically, the problem of optical flow is an optimization problem. After the recent developments in deep learning, many researchers have applied deep learning to solve this optimization problem by processing consecutive video frames as input to calculate the optical flow of the object in motion. Although these approaches just process two consecutive frames at a time, still the essence of a video is captured in these two frames. The main thing that distinguishes videos from images is that videos possess a temporal structure in addition to the spatial structure of the images. However, videos also have other modalities such as sound, but they are of no use in this case. Therefore consecutive frame stream can be interpreted as a collection of images operating in a specific temporal resolution (fps). This means that data in a video is encoded not only spatially but also sequentially, which makes classifying videos quite interesting and yet challenging at the same time.
从历史上看,光流问题是一种优化问题。 在深度学习的最新发展之后,许多研究人员已经应用深度学习通过处理连续的视频帧作为输入来计算运动对象的光流来解决此优化问题。 尽管这些方法一次仅处理两个连续的帧,但仍在这两个帧中捕获了视频的本质。 区分视频和图像的主要方面是,视频除了具有图像的空间结构外,还具有时间结构。 但是,视频还具有其他形式,例如声音,但是在这种情况下它们没有用。 因此,连续的帧流可以解释为以特定时间分辨率(fps)运行的图像的集合。 这意味着视频中的数据不仅在空间上而且还在顺序上进行编码,这使得对视频进行分类非常有趣并且同时具有挑战性。

Generally, deep neural networks require a large amount of training data to learn and optimize the approximation functions. But in the case of optical flow estimation, training data is particularly hard to obtain. The major reason behind this is the difficulty of accurately labeling video footage for the exact motion of every point of an image to subpixel accuracy. Therefore to address the issue of labeling video data, computer graphics are used to simulate massive realistic worlds through instructions. As the instructions are known, the motion of every pixel in the video frame sequence is already known. Some of the recent research that attempts to solve the optical flow problems are PWC-Nets, ADLAB-PRFlow, and FlowNet. Optical flow is widely inherited by many applications like vehicle tracking and traffic analysis through object detection and multi-object tracking by feature-based optical flow techniques from either from a stationary camera or cameras attached to vehicles.
通常,深度神经网络需要大量的训练数据来学习和优化近似函数。 但是在光流估计的情况下,特别难以获得训练数据。 其背后的主要原因是难以准确标记视频素材,以使图像的每个点的精确运动达到亚像素精度。 因此,为了解决标记视频数据的问题,计算机图形用于通过指令来模拟大量现实世界。 如已知的指令,视频帧序列中每个像素的运动是已知的。 尝试解决光流问题的一些最新研究是PWC-Net,ADLAB-PRFlow和FlowNet。 光流已被许多应用程序广泛继承,例如通过对象检测进行的车辆跟踪和交通分析以及通过基于特征的光流技术进行的多对象跟踪来自固定摄像机或安装在车辆上的摄像机。
自我监督的深度学习跟踪 (Self-Supervised Deep Learning for Tracking)
As mentioned earlier, visual tracking is integral for many tasks like recognition, interaction, and geometry under the domain of video analysis. But at the same time using deep learning for these tasks becomes infeasible due to the huge requirement of labeled video data. Anyway, to achieve high performance, large-scale tracking datasets become necessary which in turn requires extensive efforts and thus makes the deep learning approach more impractical and expensive. Keeping this in mind, recent researchers have put their faith in a promising approach to make the machines learn without human supervision (labeled data) by leveraging large amounts of unlabeled and raw video data. This quest for self-supervised learning started with a research proposal from the Google research team that suggested to make a visual tracking system by training a model on a proxy task of video colorization that doesn’t require any additional labeled data (self-supervision). However, the research suggested that instead of making the model predict the color of the input grayscale frame, it must learn to copy the colors from a set of reference frame, thus leading to the rise of a pointing mechanism that is able to track the spatial feature of a video sequence in a temporal setup. Visualizations and experiments of these self-supervised methods suggest that, although the network is trained without any human supervision, a mechanism for visual feature tracking automatically emerges inside the network. After plenty of training on unlabeled video collected from the internet, the self-supervised model was able to track any segmented region specified in the initial frame of the video frame sequence. However, the self-supervised deep learning methods are trained on an assumption that the color in the frame sequence is temporally stable. Clearly, there are exceptions, like colorful lights can turn on and off in the video.
如前所述,视觉跟踪是视频分析领域中许多任务(如识别,交互和几何)不可或缺的。 但是同时,由于标记视频数据的巨大需求,将深度学习用于这些任务变得不可行。 无论如何,为了实现高性能,大规模的跟踪数据集变得必要,而这又需要大量的努力,因此使深度学习方法更加不切实际和昂贵。 牢记这一点,最近的研究人员将他们的信念放在了一种有前途的方法上,该方法通过利用大量未标记和原始的视频数据来使机器无需人工监督(标记数据)即可学习。 这项自我监督学习的追求始于Google研究小组的一项研究建议,该建议建议通过训练不需要任何附加标签数据的视频着色代理任务模型来构建视觉跟踪系统(自我监督) 。 但是,研究表明,与其让模型预测输入灰度框的颜色,不如从模型参考框的集合中学习复制颜色,从而导致能够跟踪空间的指向机制的兴起。时间设置中视频序列的特征。 这些自我监督方法的可视化和实验表明,尽管在没有任何人工监督的情况下训练了网络,但网络内部自动出现了一种视觉特征跟踪机制。 在对从互联网收集的未标记视频进行大量训练之后,自我监督模型能够跟踪在视频帧序列的初始帧中指定的任何分段区域。 然而,在帧序列中的颜色在时间上是稳定的假设下训练自我监督的深度学习方法。 显然,也有例外,例如可以在视频中打开和关闭彩灯。
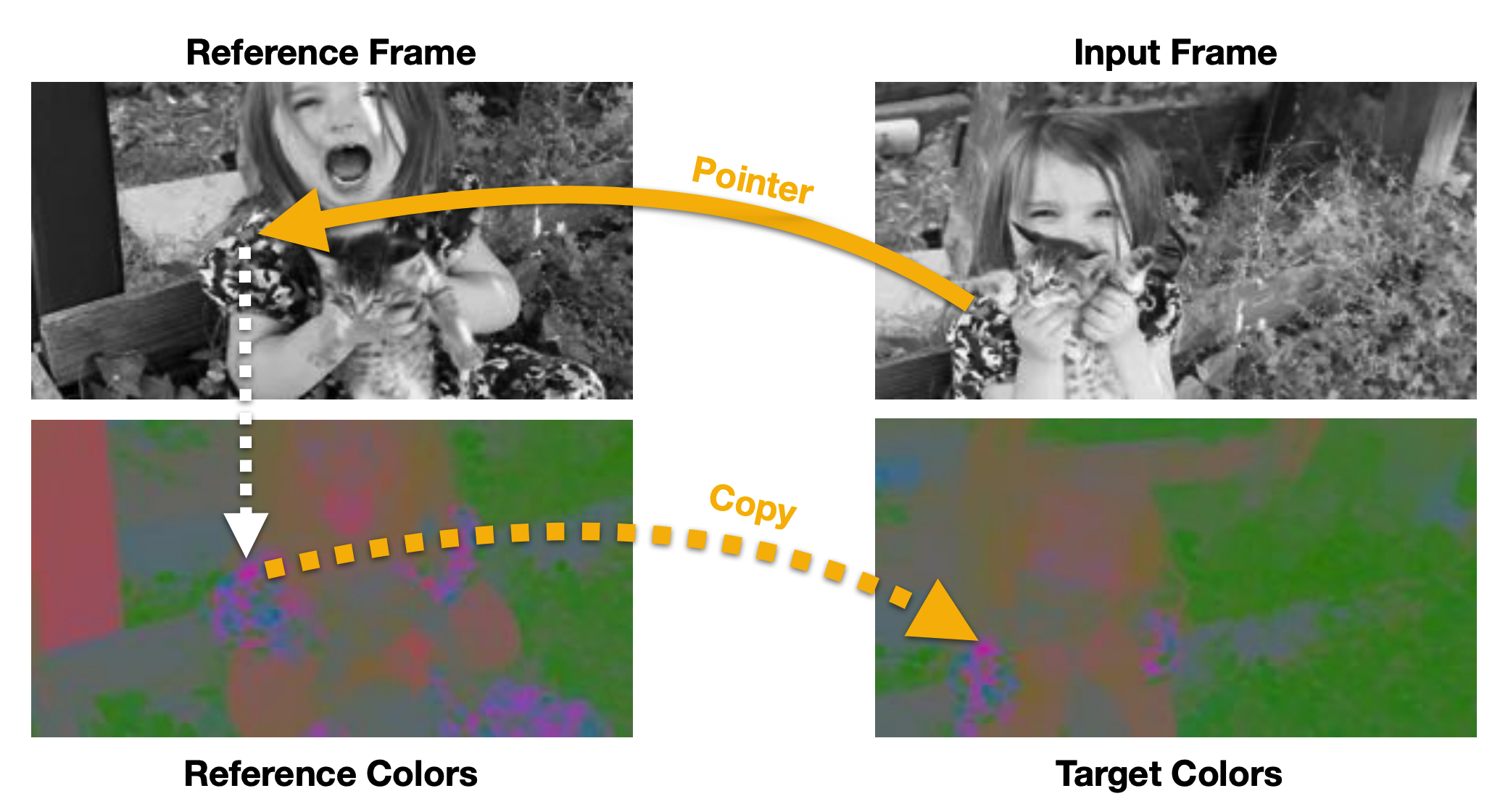
The objective of self-supervised learning in tracking is to learn feature embedding that is suitable for matching correspondences along the frame sequence of a video. The correspondence flow is learned by exploiting the natural spatial-temporal coherence in the frame sequence. Correspondence flow can be understood as the feature similarity flow existing between consecutive frames. In simple language, this approach learns a pointer mechanism that can reconstruct a target image by copying pixel information from a set of reference frames. Therefore to make such a model, there are certain precautions a researcher must keep in mind while designing the architecture. First, we must prevent the model from learning trivial solution of this task ( e.g. matching consecutive frames based on low-level color features). Second, we must make the tracker drifting less severe. Tracker drifting (TD) is mainly caused due to occlusion of objects, complex object deformation, and random illumination changes. TD is usually handled by training recursive models over long temporal windows with cycle consistency and scheduled sampling.
跟踪中自我监督学习的目的是学习适合于沿着视频帧序列匹配对应关系的特征嵌入。 通过利用帧序列中的自然时空一致性来学习对应流。 对应流程可以理解为连续帧之间存在的特征相似度流程。 用简单的语言,这种方法学习了一种指针机制,该机制可以通过从一组参考帧中复制像素信息来重建目标图像。 因此,要建立这样的模型,研究人员在设计体系结构时必须牢记某些预防措施。 首先,我们必须防止模型学习此任务的琐碎解决方案(例如,基于低级色彩特征匹配连续帧)。 第二,我们必须使跟踪器的漂移不那么严重。 跟踪器漂移(TD)主要是由于物体的遮挡,复杂的物体变形和随机照明变化引起的。 TD通常通过在具有循环一致性和计划采样的较长时间窗口上训练递归模型来处理。
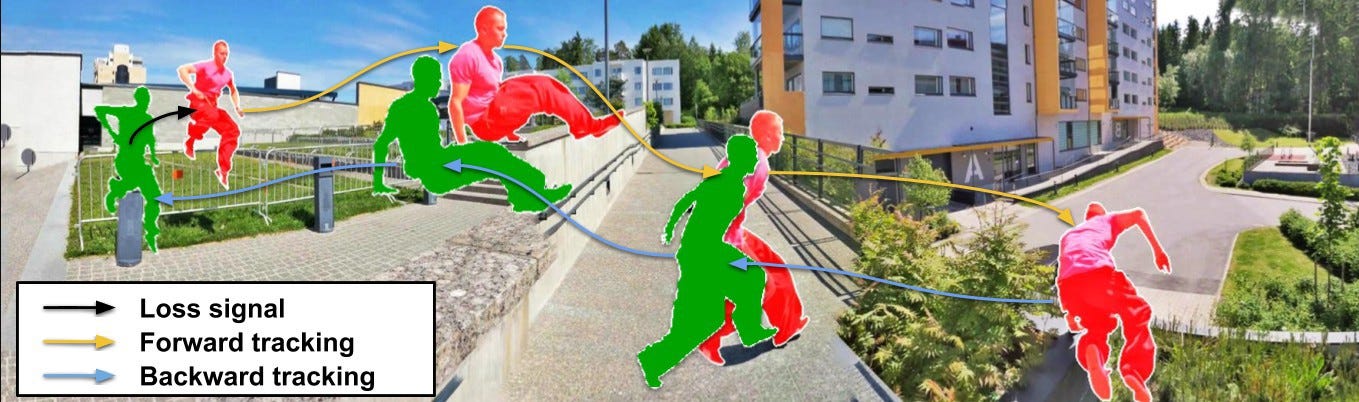
Finally, before we look under the hood of this pointer mechanism, let’s cover some of the above-mentioned points that one must consider while designing such models. First, it’s important to remember that correspondence matching is the fundamental building block of these models. Therefore there is a high probability that the model will learn a trivial solution while doing frame reconstruction by pixel-wise matching. To prevent the model from overfitting on a trivial solution, it is important to add color jittering and channel-wise dropout, so that model is forced to rely on low-level color information and must be robust to any kind of color jittering. Lastly, to handle TD, as suggested earlier, recursive training over long temporal windows with forward-backward consistency and scheduled sampling is the best way to alleviate the tracker drifting problem. If we apply the above-mentioned methods, we can be sure that the model robustness will increase and the approach will be able to exploit the spatial-temporal coherence of the video and colors will be able to act as a reliable supervision signal for learning correspondences.
最后,在介绍这种指针机制之前,让我们介绍一些在设计此类模型时必须考虑的上述要点。 首先,重要的是要记住,对应匹配是这些模型的基本组成部分。 因此,在通过逐像素匹配进行帧重构的同时,该模型很有可能学习到一个简单的解决方案。 为防止模型过度拟合,请添加颜色抖动和逐通道丢失,这一点很重要,因此模型必须被迫依赖于低级颜色信息,并且必须对任何类型的颜色抖动都具有鲁棒性。 最后,如前所述,要处理TD,在长时间窗上进行前向后一致性和计划采样的递归训练是缓解跟踪器漂移问题的最佳方法。 如果我们采用上述方法,则可以确保模型的鲁棒性将会提高,并且该方法将能够利用视频的时空一致性,并且颜色将能够充当学习对应关系的可靠监督信号。 。
引擎盖下的自我监督注意 (Self-supervised Attention under the Hood)
If you look deeper into what actually is the pointer mechanism that is being learned here, you will come to the conclusion that it is a type of attention mechanism. Yes, it’s ultimately the famous trio of QKV (Query-Key-Value, the basis of most attention mechanisms).
如果您更深入地了解这里实际学习的指针机制,您将得出结论,它是一种注意力机制。 是的,它最终是著名的QKV三人组(Query-Key-Value,大多数关注机制的基础)。

As we know, the goal of the self-supervised model is to learn robust correspondence matching by effectively encoding feature representations. In simple language, the ability to copy effectively is achieved by training on a proxy task, where the model learns to reconstruct a target frame by linearly combining pixel data from the reference frames, with the weights measuring the strength of correspondence between pixels. However, breaking down this process, we find that there is a triplet (Q, K, V) for every input frame we process. The Q, K, V refer to Query, Key, and Value. To reconstruct a pixel I¹ in the T¹ frame, an Attention mechanism is used for copying pixels from a subset of previous frames in the original sequence. Just, in this case, the query vector (Q) is the present frame’s(I¹) feature embedding (target frame), the key Vector is the previous frame’s(I⁰) feature embedding (reference frame). Now if we compute a dot product (.) between the query and key (Q.K) and take a softmax of the computed product, we can get a similarity between the present frame ( I¹ ) and the previous reference frame (I⁰). This computed similarity matrix when multiplied with a reference instance segmentation mask (V) during inference will give us a pointer for our target frame, thus achieving dense optical flow estimation. Therefore this pointer which is just a combination of Q, K, and V is the actual attention mechanism working under the hood of this self-supervised system.
众所周知,自我监督模型的目标是通过有效地编码特征表示来学习鲁棒的对应匹配。 用简单的语言来说,有效的复制能力是通过训练代理任务来实现的,在代理任务中,模型学习通过线性组合来自参考帧的像素数据,并使用权重测量像素之间的对应强度来重建目标帧。 但是,分解此过程,我们发现每个处理的输入帧都有一个三元组(Q,K,V)。 Q,K,V表示查询,键和值。 为了在T 1帧中重建像素I 1,使用注意力机制从原始序列的先前帧的子集中复制像素。 只是,在这种情况下,查询向量(Q)是当前帧的(I¹)特征嵌入(目标帧),关键向量是前一帧的(I⁰)特征嵌入(参考帧)。 现在,如果我们在查询和键(QK)之间计算一个点积(。)并取所计算乘积的softmax,我们可以得到当前帧(I 1)和先前参考帧(I 1)之间的相似性。 当在推理过程中将此计算的相似度矩阵与参考实例分割掩码(V)相乘时,将为我们提供目标帧的指针,从而实现密集的光流估计。 因此,该指针只是Q,K和V的组合,是在此自我监督系统的幕后起作用的实际注意力机制。
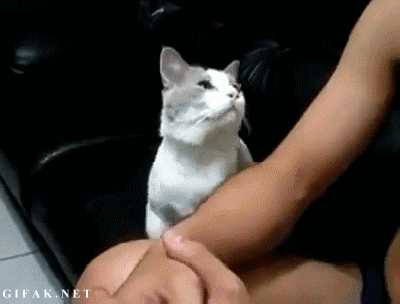
A key element in attention mechanism training is to establish a proper information bottleneck. To circumvent any learning shortcuts that the attention mechanism may resort to, the previously mentioned techniques of intentionally dropping the input color information and channel dropout are used. However, the choice of color spaces still plays an important role in training these attention mechanisms through self-supervision. Many research works have validated the conjecture that using decorrelated color space leads to better feature representations for self-supervised dense optical flow estimation. In simple language, using the LAB format image works better than the RGB format. This is because all RGB channels include a representation of brightness, making it highly correlate to the luminance in Lab, therefore acting as a weak information bottleneck.
注意机制培训的关键要素是建立适当的信息瓶颈。 为了规避注意力机制可能求助的任何学习捷径,使用了前面提到的有意删除输入颜色信息和通道丢失的技术。 然而,色彩空间的选择在通过自我监督来训练这些注意力机制中仍然扮演着重要的角色。 许多研究工作已经证实了这样的猜想,即使用去相关的色彩空间可以为自监督的密集光流估计带来更好的特征表示。 用简单的语言来说,使用LAB格式的图像要比RGB格式的效果更好。 这是因为所有RGB通道都包含亮度的表示形式,使其与Lab中的亮度高度相关,因此,它是一个较弱的信息瓶颈。
限制注意力以最小化物理内存成本 (Restricted Attention for minimizing physical memory costs)
The above-proposed attention mechanism usually comes with high physical memory cost. Therefore processing high-resolution information for correspondence matching can lead to large memory requirements and slower speed.
上述提议的注意机制通常带有很高的物理内存成本。 因此,处理用于对应匹配的高分辨率信息可能导致大量的内存需求和较慢的速度。
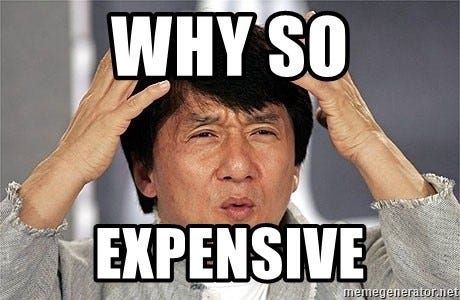
To circumvent the memory cost, ROI localization is used to estimate the candidate windows non-locally from memory banks. Intuitively, we can say that for temporally close frames, spatial-temporal coherence naturally exists in the frame sequence. This ROI localization leads to restricted attention as now the pixel in the target frame is only compared to spatially neighboring pixels of the reference frame. The number of comparable pixels is determined by the size of the dilated window in which the attention is restricted. The dilation rate of the window is proportional to the temporal distance between the present frame and the past frames in the memory bank. After computing the affinity matrix of the restricted attention region, fine-grained matching scores can be computed in a non-local manner. Therefore, with the proposed memory-augmented restricted attention mechanism, the model can efficiently process high-resolution information without incurring large physical memory costs.
为了规避存储成本,ROI本地化用于从存储库中非本地估计候选窗口。 直觉上,我们可以说,对于时间上接近的帧,在帧序列中自然存在时空相干性。 由于现在仅将目标帧中的像素与参考帧中的空间相邻像素进行比较,因此该ROI定位导致注意力受到限制。 可比较像素的数量由限制注意力的膨胀窗口的大小确定。 窗口的膨胀率与存储库中当前帧和过去帧之间的时间距离成比例。 在计算受限关注区域的亲和度矩阵之后,可以以非局部方式计算细粒度的匹配分数。 因此,通过提出的内存增强的受限注意机制,该模型可以有效地处理高分辨率信息,而不会产生大量的物理内存成本。
结论 (Conclusion)
In this blog, we started with an introduction to the concept of optical flow and studied its application in object tracking. We also studied how this concept inspired the deep learning tracking systems and how self-supervision and visual attention plays a key role in making these systems. The computed optical flow vectors open a myriad of possible applications that require such an in-depth scene understanding of videos. The discussed techniques are majorly applied to pedestrian tracking, autonomous vehicle navigation, and many more novel applications. The variety of applications where the optical flow can be applied is only limited by the ingenuity of its designers.
在此博客中,我们首先介绍了光流的概念,并研究了其在对象跟踪中的应用。 我们还研究了这一概念如何激发了深度学习跟踪系统,以及自我监督和视觉注意力如何在制造这些系统中发挥关键作用。 计算出的光流矢量打开了无数可能的应用,这些应用需要对视频进行如此深入的场景理解。 所讨论的技术主要应用于行人跟踪,自动驾驶汽车导航以及许多其他新颖的应用。 可以应用光流的各种应用仅受其设计人员的独创性限制。
In my personal opinion, self-supervision will soon serve as a strong competitor to its supervised counterpart because of its generalizability and flexibility. Self-supervision easily outperforms most of the supervised methods on unseen object categories, which reflects its importance and power in the coming time as we take our steps towards solving human intelligence.
我个人认为,由于自我监督的普遍性和灵活性,自我监督将很快成为其受监督同行的有力竞争者。 在看不见的物体类别上,自我监督很容易胜过大多数受监督的方法,这反映了在我们逐步解决人类智能的过程中,自我监督的重要性和力量。
My blogs are a reflection of what I worked on and simply convey my understanding of these topics. My interpretation of deep learning can be different from that of yours, but my interpretation can only be as inerrant as I am.
我的博客反映了我所做的工作,只是传达了我对这些主题的理解。 我对深度学习的解释可能与您的解释不同,但是我的解释只能像我一样错误。
dis 密集光流