bagging方法 套袋
A machine learning ensemble used to improve the accuracy and stability of algorithms in regression and statistical classification.
一种机器学习集合,用于提高回归和统计分类中算法的准确性和稳定性。
Ensemble machine learning can be mainly categorized into bagging and boosting. The bagging technique is useful for both regression and statistical classification. Bagging is used with decision trees, where it significantly raises the stability of models in the reduction of variance and improving accuracy, which eliminates the challenge of overfitting.
集成机器学习主要可以分为装袋和提升。 套袋技术可用于回归和统计分类。 套袋与决策树一起使用,可以显着提高模型在减少方差和提高准确性方面的稳定性,从而消除了过拟合的挑战。
介绍 (Introduction)
As mentioned before , two possibilities for making majority votes were bagging and boosting.The principle of bagging is rather simple and does not present any major difficulty for the implementation.The challenge lies in selecting the optimal parameters to build the voters, because we have a wide range of possibilities.
如前所述,袋装和提高票数是获得多数选票的两种可能性。袋装的原理很简单,在实施过程中不会遇到任何主要困难。挑战在于选择最佳参数来构建选民,因为我们有一个广泛的可能性。
The term introduced, bagging, is shorthand for the combination of bootstrapping and aggregating. It aims at producing an ensemble model that is more robust than the individual models composing it.
引入的术语“装袋”是自举和聚集的组合的简写。 它旨在产生一个整体模型,该模型比构成它的单个模型更健壮 。
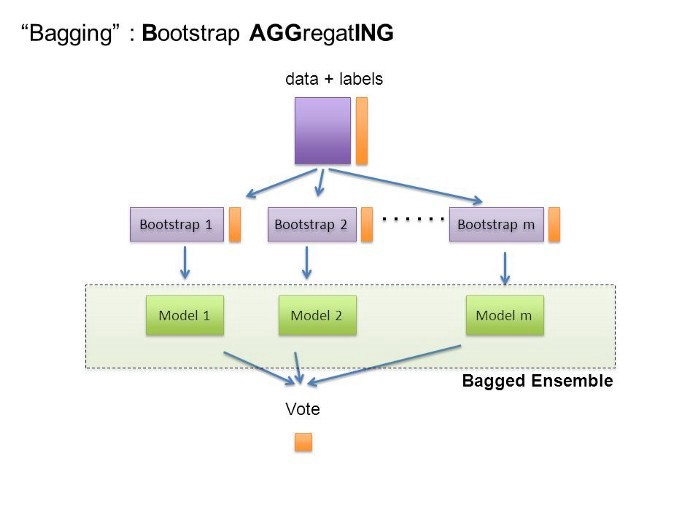
1.自举 (1. Bootstrapping)
Bootst