清理数据 python
内容 (Content)
- Identifying data types 识别数据类型
- Fixing the rows and columns 固定行和列
- Imputing/removing missing values 插补/删除缺失值
- Handling outliers 处理异常值
- Standardising the values 标准化值
- Fixing invalid values 修正无效值
- Filtering the data 过滤数据
1.识别数据类型 (1. Identifying Data Types)
- Find Categorical Data 查找分类数据
list(df.columns[df.dtypes == ‘object’])
列表(df.columns [df.dtypes =='object'])
But, Categorical data can exist in Numerical format. eg. , days of a month, months(1–12), waist-size (24–38).
但是,分类数据可以数字格式存在。 例如。 ,每月的某几天,几个月(1–12),腰围(24–38)。
2. Distinguish between Numerical and Categorical Data
2.区分数值数据和分类数据
df.nunique().sort_values()
df.nunique()。sort_values()
Categorical — The count of unique values should be 30 or less.
分类-唯一值的计数应小于或等于30。
对数值数据进行运算 (Perform operations on numerical data)
Correlations — should only be done on numeric variables.
关联-仅应在数字变量上进行。
uniqueCount = df.nunique()
uniqueCount = df.nunique()
numerical_columns = list (uniqueCount [ uniqueCount > 30 ].keys())
numeric_columns =列表(uniqueCount [uniqueCount> 30] .keys())
df[numerical_columns] .corr()
df [numerical_columns] .corr()
对数值数据进行可视化 (Perform Visualisations on numerical data)
Scatter plot should always be feed with numerical data each side.
散点图应始终在两侧馈送数值数据。
2.固定行和列 (2. Fixing the rows and columns)
2.1检查格式 (2.1 Check Formatting)
- Check the file format 检查文件格式
pd.read_csv() / pd.read_tsv()
pd.read_csv()/ pd.read_tsv()
2. Check the separator type
2.检查分隔符类型
pd.read_csv(sep = ‘|’)
pd.read_csv(sep ='|')
2.2固定行 (2.2 Fixing rows)
Ignore irrelevant rows — Rows other than the column name headers , use skiprows = 2
忽略不相关的行-列名标题以外的行,请使用skiprows = 2
- Delete summary rows — We derive such insights from dataset, hence not required. 删除摘要行-我们从数据集中获得此类见解,因此不是必需的。
- Delete extra rows — column number indicator, subsection, new page. 删除多余的行-列号指示符,小节,新页面。
df = df.loc[‘condition’]
df = df.loc ['condition']
2.3固定柱 (2.3 Fixing columns)
- Add column names if missing. 如果缺少,请添加列名称。
df.columns.values[i] = ‘Column_name’
df.columns.values [i] ='列名'
2. Rename columns consistently: Abbreviations, encoded columns.
2.一致地重命名列:缩写,编码列。
df.rename(columns = {‘old_name’ : ‘new_name’} )
df.rename(columns = {'old_name':'new_name'})
3. Align misaligned columns -> Manual fix on file.
3.对齐未对齐的列->手动修复文件。
4. Delete columns having less analytical value. Like name, (system-generated)id columns.
4.删除分析值较小的列。 像名称一样,(系统生成的)id列。
df.drop(columns = [‘col1’, ‘col2’])
df.drop(columns = ['col1','col2'])
df.drop([‘col1’, ‘col2’], axis = 1)
df.drop(['col1','col2'],轴= 1)
5. Split/merge columns to get more understandable data.
5.拆分/合并列以获取更多可理解的数据。
df[‘avg’] = (df[‘M1’] + df[‘M2’]) /2 ;
df ['avg'] =(df ['M1'] + df ['M2'])/ 2;
df[‘fullname’] = df[‘firstname] + ‘ ‘ + df[lastname]
df ['fullname'] = df ['firstname] +''+ df [lastname]
3.插补/删除缺失值 (3. Imputing/removing missing values)
3.1用空值替换空值 (3.1 Replace Empty values with Nulls)
Need to replace the empty strings or object NA,XX with nan
需要用nan替换空字符串或对象NA,XX
df.replace(r’^\s*$’, np.NaN, regex=True)
df.replace(r'^ \ s * $',np.NaN,regex = True)
df.replace(r’NA’, np.NaN, regex=True)
df.replace(r'NA',np.NaN,regex = True)
df.replace(r’XX’, np.NaN, regex=True)
df.replace(r'XX',np.NaN,regex = True)
3.2检查空值 (3.2 Check Nulls)
- List null values 列出空值
df.columns[df.isna().any()].tolist()
df.columns [df.isna()。any()]。tolist()
2. Check the Percentage of nulls
2.检查空值百分比
df.isnull().sum()/len(df)*100).sort_values(ascending = False)
df.isnull()。sum()/ len(df)* 100).sort_values(升序= False)
3. Profilers — Check Null value visualizations.
3.事件探查器—检查“空值”可视化。
3.3删除行/列 (3.3 Removing Rows/Columns)
- Removing the outlier data 删除异常数据
df = df[df[‘field1’] < Outliers]
df = df [df ['field1'] <离群值]
2. Removing data if the target value is missing
2.如果缺少目标值,则删除数据
df1 = df[~df[‘target-value’].isnull()].copy()
df1 = df [〜df ['target-value']。isnull()]。copy()
3. High Percentage of nulls
3.高百分比的空值
df.drop(columns = [‘col1’, ‘col2’]) / df.drop([‘col1’, ‘col2’], axis = 1)
df.drop(columns = ['col1','col2'])/ df.drop(['col1','col2'],轴= 1)
df = df.loc[:, df.isnull().mean() < .95]
df = df.loc [:, df.isnull()。mean()<.95]
3.4.1缺失值类型 (3.4.1 Missing Values Types)
MCAR: It stands for Missing Completely At Random. The reason behind a missing value is not dependent on any other feature.
MCAR :代表随机完全丢失。 缺少值的原因不依赖于任何其他功能。
MAR: It stands for Missing At Random. The reason behind a missing value may be associated with some other features.
MAR :代表“随机失踪”。 缺少值的原因可能与某些其他功能有关。
MNAR: It stands for Missing Not At Random. There is a specific reason behind a missing value.
MNAR :代表不随机丢失。 缺少值背后有特定原因。
3.4.2缺失值处理 (3.4.2 Missing Values Treatment)
df.[field].fillna() → fill null values
df。[field] .fillna() →填充空值
- Replacing all nulls with zeros → 用零替换所有空值→
df.fillna(0)
df.fillna(0)
2. Imputing values using Mean, Median, Mode
2.使用均值,中位数,众数估算值
Mean — continuous, if data set does not have outliers.
均值-连续(如果数据集没有异常值)。
df[‘field1’].mean()
df ['field1']。mean()
Median — Has outliers
中位数-有异常值
df[‘field1’].median()
df ['field1']。median()
Mode — Max occurrence value, categorical.
模式-最大发生值(绝对)。
df[‘field1’].mode()[0]
df ['field1']。mode()[0]
3. Fill with a relevant value by looking at other columns of the same row.
3.通过查看同一行的其他列来填充相关值。
df[‘field’] = df.apply(lambda x : transform(x), axis =1)
df ['field'] = df.apply(lambda x:transform(x),axis = 1)
4.处理异常值 (4. Handling outliers)
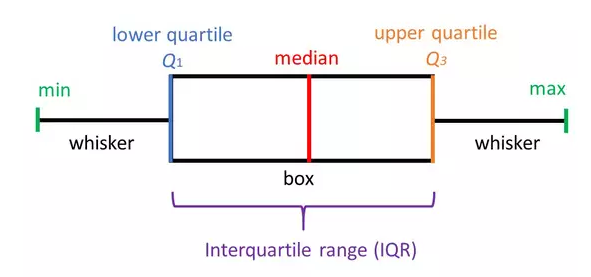
Q3 = np.percentile(df[‘field1’], 75)
Q3 = np.percentile(df ['field1'],75)
Q1 = np.percentile(df[‘field1’], 25)
Q1 = np.percentile(df ['field1'],25)
IQR = Q3 — Q1
IQR = Q3-Q1
Outliers = Q3 + 1.5 * IQR
离群值= Q3 + 1.5 * IQR
df = df[df[‘field1’] < Outliers] df[‘field1’].plot(kind=’box’)
df = df [df ['field1'] <离群值] df ['field1']。plot(kind ='box')
5.标准化值 (5. Standardising the values)
Standardise Precision — for better presentation of data. e.g. change 4.5312341 kg to 4.53 kg.
标准化精度 -更好地呈现数据。 例如,将4.5312341公斤更改为4.53公斤。
df[‘field’] = df[‘field’] .apply(lambda x : round(x,2))
df ['field'] = df ['field'] .apply(lambda x:round(x,2))
2. Scale Values/Standardise Units — Ensure all observations under one variable are expressed in a common and consistent unit.
2. 标度值/标准化单位 -确保在一个变量下的所有观察值均以相同且一致的单位表示。
df[‘field’] = df[‘field’] .apply(lambda x : transform(x))
df ['field'] = df ['field'] .apply(lambda x:transform(x))
3. Standardise Format — It is important to standardise the format of other elements such as date and name. e.g., change 23/10/16 to 2016/10/23.
3. 标准化格式—标准化其他元素(例如日期和名称)的格式很重要。 例如,将23/10/16更改为2016/10/23。
df[‘field1’] = pd.to_datetime(df[‘field1’], format = ’%d%b%Y:%H:%M:%S. %f’)
df ['field1'] = pd.to_datetime(df ['field1'],format ='%d%b%Y:%H:%M:%S。%f')
strftimes — Check the date format from this link.
strftimes-从此链接检查日期格式。
4. Standardise Case — String variables may take various casing styles, e.g. FULLCAPS, lowercase, Title Case, Sentence case, etc.
4. 标准化大小写 —字符串变量可以采用各种大小写样式,例如FULLCAPS,小写字母,标题大小写,句子大小写等。
df[‘field1’] = df[‘field1’].str.title()
df ['field1'] = df ['field1']。str.title()
5. Remove Characters — Remove extra characters such as common prefixes/suffixes, leading/trailing/multiple spaces.
5. 删除字符 -删除多余的字符,例如常见的前缀/后缀,前导/后缀/多个空格。
df[‘field1’] = arrests[‘field1’].str.replace(‘$’, ‘’ )
df ['field1'] =逮捕['field1']。str.replace('$','')
df[‘field1’] = df[‘field1’].str.strip()
df ['field1'] = df ['field1']。str.strip()
6.修正无效值 (6. Fixing invalid values)
Encode unicode properly — In case the data is being read as junk characters, try to change the encoding.
正确编码unicode-如果数据被读取为垃圾字符,请尝试更改编码。
df= pd.read_csv(‘file.csv’,encoding =’cp1252')
df = pd.read_csv('file.csv',encoding ='cp1252')
2. Convert incorrect data types — Change the incorrect data types to the correct data types for ease of analysis.
2. 转换不正确的数据类型—将不正确的数据类型更改为正确的数据类型,以便于分析。
df[‘field1’] = df[‘field1’].astype(int)
df ['field1'] = df ['field1']。astype(int)
df[toNumFieldsArray] = df[toNumFieldsArray].apply(pd.to_numeric, errors=’coerce’,axis=1)
df [toNumFieldsArray] = df [toNumFieldsArray] .apply(pd.to_numeric,errors ='coerce',axis = 1)
3. Correct values that lie beyond the range — If some values lie beyond the logical range.
3. 纠正超出范围的值-如果某些值超出逻辑范围。
df.[‘field1’].describe() -> min/max values
df。['field1']。describe()->最小值/最大值
df.[‘field1’].plot(kind = ‘box’)
df。['field1']。plot(kind ='box')
4. Correct values that do not belong to the list — Remove values that do not belong to a list. eg. a data set of blood groups of individuals, strings ‘E’ and ‘F’ are invalid values.
4. 纠正不属于列表的值—删除不属于列表的值。 例如。 在个人血型数据集中,字符串“ E”和“ F”是无效值。
df[‘field’].value_counts()
df ['field']。value_counts()
5. Fix incorrect structure — Values that do not follow a defined structure can be removed Eg. a phone number of 12 digits is an invalid value.
5. 修复错误的结构-可以删除不遵循定义的结构的值,例如。 12位电话号码是无效值。
df[‘len’] = df[‘filed1’].apply(lambda x : len(str(x)))
df ['len'] = df ['filed1']。apply(lambda x:len(str(x)))
6. Validate internal rules — Internal rules, if present, should be correct and consistent. eg. a product’s date of delivery cannot be less than date of purchase.
6. 验证内部规则-内部规则(如果存在)应正确且一致。 例如。 产品的交货日期不能少于购买日期。
df[df[‘field1’]>df[‘field2’]]
df [df ['field1']> df ['field2']]
7.过滤数据 (7. Filtering the data)
Deduplicate data — Remove identical rows and rows in which some columns are identical.
重复数据删除—删除相同的行和某些列相同的行。
df.drop_duplicates(subset =”field1",keep = first/last, inplace = True)
df.drop_duplicates(subset =“ field1”,keep = first / last,inplace = True)
2. Filter rows — Filter rows by segment and date period to obtain only rows that are relevant to the analysis.
2. 筛选行—按细分和日期期间筛选行,以仅获取与分析相关的行。
df = df.loc[‘condition’]
df = df.loc ['condition']
3. Filter columns — Filter columns that are relevant to the analysis.
3. 过滤器列-与分析相关的过滤器列。
df_derived = df.filter(regex = “^COMMON_EXP”, axis = 1)
df_derived = df.filter(regex =“ ^ COMMON_EXP”,轴= 1)
4. Binning Data — converting a numerical data to categorical.
4. 合并数据 -将数字数据转换为分类数据。
df[‘field-group’] = pd.cut(df[‘field1’] , bins=np.linspace (min,max,bin_count))
df ['field-group'] = pd.cut(df ['field1'],bins = np.linspace(min,max,bin_count))
翻译自: https://medium.com/@duarohan18/data-cleaning-techniques-using-python-b6399f2550d5
清理数据 python