数学建模时间序列分析
时间序列预测 (Time Series Forecasting)
背景 (Background)
This article is the fourth in the series on the time-series data. We started by discussing various exploratory analyses along with data preparation techniques followed by building a robust model evaluation framework. And finally, in our previous article, we discussed a wide range of classical forecasting techniques that must be explored before moving to machine learning algorithms.
本文是有关时间序列数据的系列文章中的第四篇。 我们首先讨论各种探索性分析以及数据准备技术,然后建立一个强大的模型评估框架。 最后,在我们的前一篇文章中,我们讨论了广泛的经典预测技术,在转向机器学习算法之前必须对其进行探索。
Now, in the current article, we are going to apply all these learnings to a real-life dataset. We will work through a time series forecasting project from end-to-end, from importing the dataset, analyzing and transforming the time series to training the model, and making predictions on new data. The steps of this project that we will work through are as follows:
现在,在当前文章中,我们将所有这些学习应用于实际数据集。 我们将从头到尾完成一个时间序列预测项目,从导入数据集,分析和转换时间序列到训练模型,以及对新数据进行预测。 我们将完成的该项目的步骤如下:
- Problem Description 问题描述
- Data Preparation and Analysis 数据准备与分析
- Set up an Evaluation Framework 建立评估框架
- Stationary Check: Augmented Dickey-Fuller test 固定检查:增强的Dickey-Fuller测试
- ARIMA Models ARIMA模型
- Residual Analysis 残差分析
- Bias corrected Model 偏差校正模型
- Model Validation 模型验证
问题描述 (Problem Description)
The problem is to predict the number of monthly airline passengers. We will use the Airline Passengers dataset for this exercise. This dataset describes the total number of airline passengers over time. The units are a count of the number of airline passengers in thousands. There are 144 monthly observations from 1949 to 1960. Below is a sample of the first few rows of the dataset.
问题是要预测每月的航空公司乘客数量。 我们将使用航空公司乘客数据集进行此练习。 该数据集描述了一段时间内航空公司乘客的总数。 单位是数千名航空公司乘客的总数。 从1949年到1960年,每月进行144次观测。下面是数据集前几行的样本。
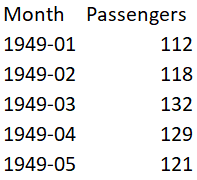
You can download this dataset from here.
您可以从此处下载该数据集。
此项目的Python库 (Python Libraries for this Project)
We need the following libraries to work on this project. These names are self-explanatory but don’t worry if you are not getting any of them. As we go along you will understand the usage of these libraries.
我们需要以下库来进行此项目。 这些名称是不言自明的,但是不用担心这些名称。 随着我们的前进,您将了解这些库的用法。
import numpy
from pandas import read_csv
from sklearn.metrics import mean_squared_error
from math import sqrt
from math import log
from math import exp
from scipy.stats import boxcox
from pandas import DataFrame
from pandas import Grouper
from pandas import Series
from pandas import concat
from pandas.plotting import lag_plot
from matplotlib import pyplot
from statsmodels.tsa.stattools import adfuller
from statsmodels.tsa.arima_model import ARIMA
from statsmodels.tsa.arima_model import ARIMAResults
from statsmodels.tsa.seasonal import seasonal_decompose
from statsmodels.graphics.tsaplots import plot_acf
from statsmodels.graphics.tsaplots import plot_pacf
from statsmodels.graphics.gofplots import qqplot
数据准备与分析 (Data Preparation and Analysis)
We will use the read_csv() function to load the time series data as a series object, a one-dimensional array with a time label for each row. It is always good to take a peek at the data to confirm that data has been loaded correctly.
我们将使用read_csv()函数将时间序列数据加载为序列对象,即一维数组,每行带有时间标签。 偷看数据以确认已正确加载数据始终是一件好事。
series = read_csv('airline-passengers.csv', header=0, index_col=0, parse_dates=True, squeeze=True)
print(series.head())
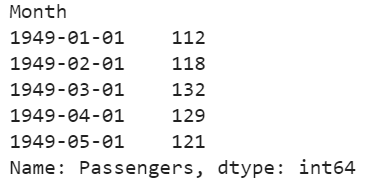
让我们通过查看汇总统计数据开始数据分析,我们将快速了解数据分布。 (Let’s begin the data analysis by looking into the summary statistics, we will get a quick idea of the data distribution.)
print(series.describe())
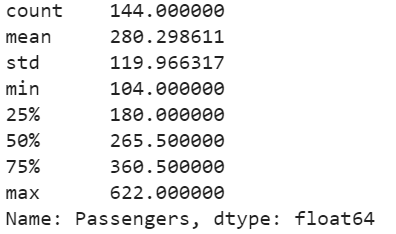
We can see the number of observations matches our expectations, the mean is about 280 which we can consider our level in this series. Other statistics like standard deviation and percentiles suggest a large spread of the data.
我们可以看到观察次数与我们的期望相符,平均数约为280,我们可以将其视为本系列的水平。 其他统计数据(例如标准差和百分位数)表明数据分布广泛。
下一步,我们将可视化折线图上的值,该工具可以为问题提供很多见解。 (As a next step, we will visualize the values on a line plot, this tool can provide a lot of insights into the problem.)
series.plot()
pyplot.show()