How does your brain represent the environment ? The partial answer is the admittedly complex title which we will explore in this article.
您的大脑如何代表环境? 部分答案是公认的复杂标题,我们将在本文中进行探讨。
Representation is a hard problem for both Neuroscience and AI and a good explanation of these terms I think is helpful if we are to build better AIs, this is my attempt.
对于神经科学和AI来说,表示形式都是一个难题,对这些术语的良好解释我认为如果我们要构建更好的AI会有所帮助,这是我的尝试。
制图表达 (Representations)
A representation is simply that, the internal description of something, a thing, an idea or even a thought or feeling in general, but alas these later ones are very high in the hierarchy of representations and we don’t yet know exactly how they are formed in biological brains. A simpler representation is your perception of a physical thing out there, an apple for instance.
表示只是在某种程度上对事物,事物,想法甚至想法或感觉的内部描述,但是可惜这些后来的表示在表示层次中非常高,我们尚不知道它们到底是什么。在生物大脑中形成。 一个简单的表示就是您对外面的物理事物的感知,例如一个苹果。
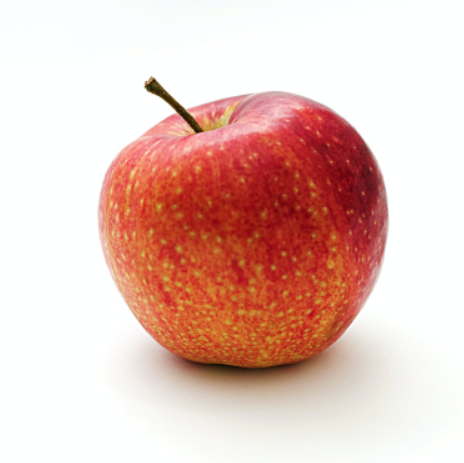
And how do we know they are representations ? We don’t definitely know, what with everyone perceiving the environment in a slightly different way, but we have some agreement and if I ask you what this is:
我们怎么知道它们是代表? 我们绝对不知道,每个人以稍微不同的方式来感知环境是什么,但是我们有一些协议,如果我问你这是什么:
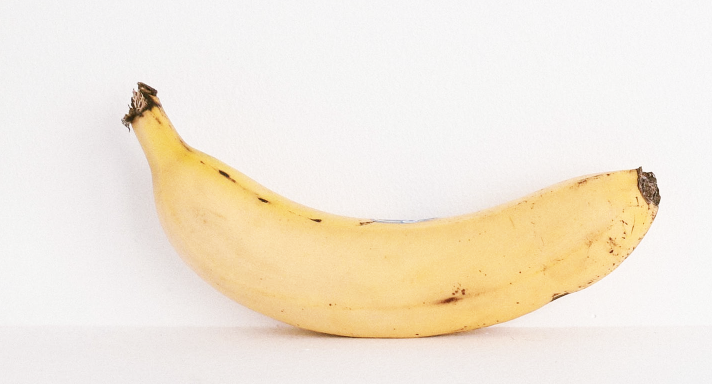
You ( and most people ) will surely answer a banana, so it follows that you had some form of stored representation of it since you recognized it.
您(和大多数人)肯定会回答一个香蕉,因此可以肯定的是,自从您识别出香蕉以来,就已经有了某种形式的存储表示形式。
疏 (Sparse)
Unlike a folder or book you store somewhere unique and specific like a bookshelf our brains seem to store things in a sparse manner, so you would break down the book and store the pieces in different places, a page here a page there, the cover over there. Memory at this level can be understood as a series of changing pointers, directions as to where you stored each element.
不同于文件夹或书本,您将书本放在一个独特而又特定的地方,就像我们的大脑似乎以稀疏的方式来存储东西,因此您需要分解书本并将其碎片存储在不同的位置,这里一页一页,最后一页封面那里。 此级别的内存可以理解为一系列不断变化的指针,即有关存储每个元素的位置的方向。
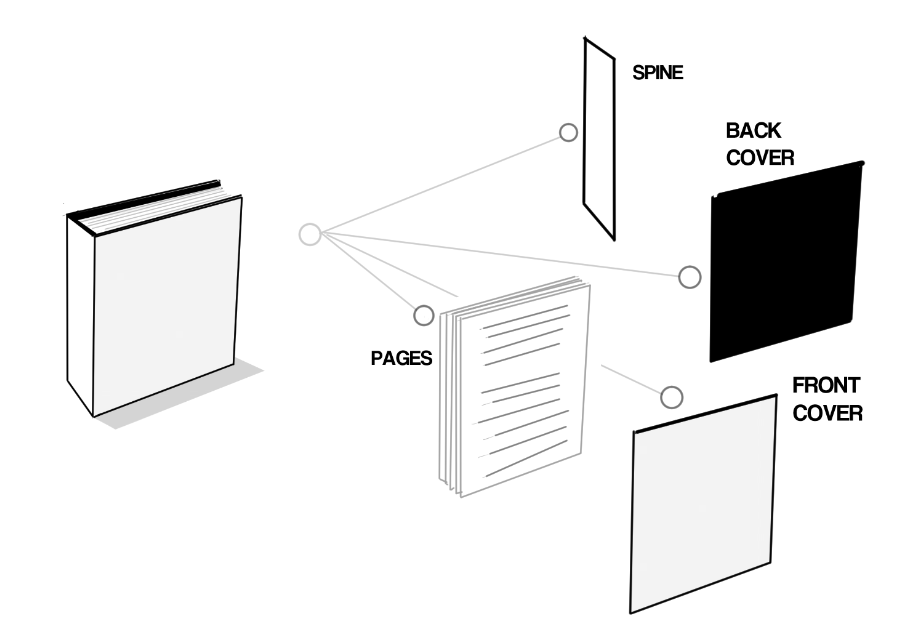
Vision is a good example since there is good research related to how we store images and movement, let’s say you want to store a symbol you have never seen:
视觉是一个很好的例子,因为有很多有关我们如何存储图像和运动的研究,假设您要存储从未见过的符号:
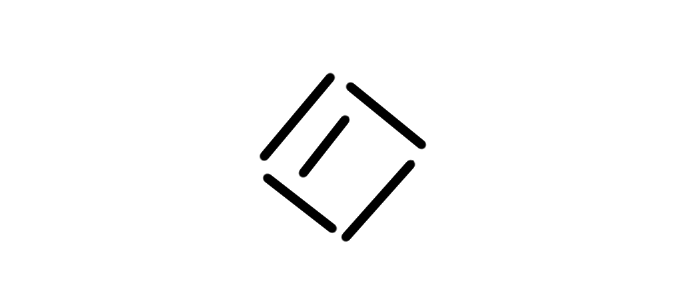
Rather than store the image as a whole, the image is broken down into parts (mainly via retinotopic maps/receptive fields), so for instance this is a simplified view of the encoding of the previous symbol:
而不是将图像作为一个整体存储,而是将其分解为多个部分(主要是通过视网膜视点贴图/接受域),因此例如,这是前一个符号编码的简化视图:
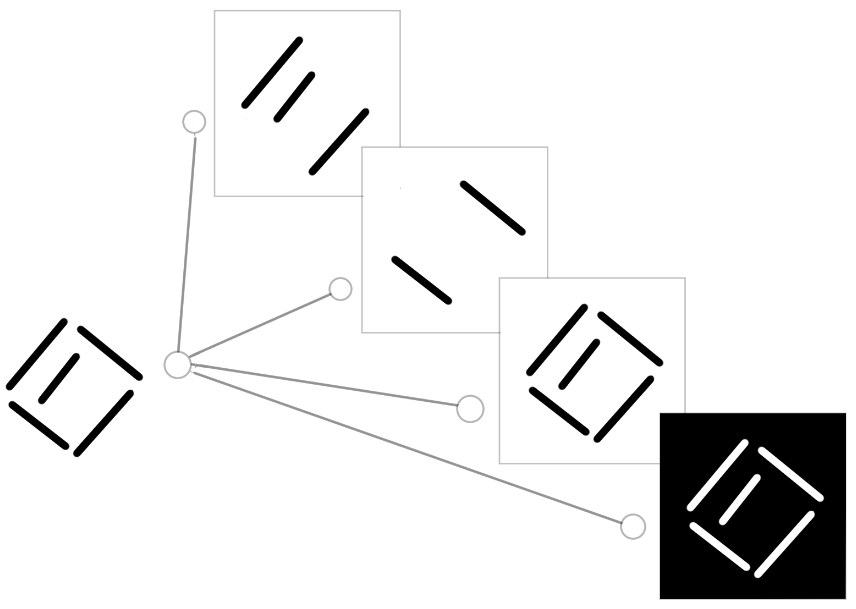
Here we have 4 different visual maps ( the blocks ), the first 2 code for lines at a certain angle, the other 2 for positive and negative space representations, in the brain the blocks are thought to be groups of neurons. How we later integrate these sparse elements into a cohesive whole is not yet known and it's sometimes called the integration problem. Intermediate, temporal and recursive maps could explain them, but that's for another article.
分层的 (Hierarchical)
A more complex shape and meaning requires the combination of several maps and their resulting simple shapes into complex objects*. Rich and complex perceptions seem to be stored in a hierarchical manner, this means that lower/simpler representations are recruited to form them:
更复杂的形状和含义需要将多个地图及其产生的简单形状组合成复杂的对象*。 丰富而复杂的感知似乎是以分层方式存储的,这意味着将招募较低/较简单的表示形式来构成它们:
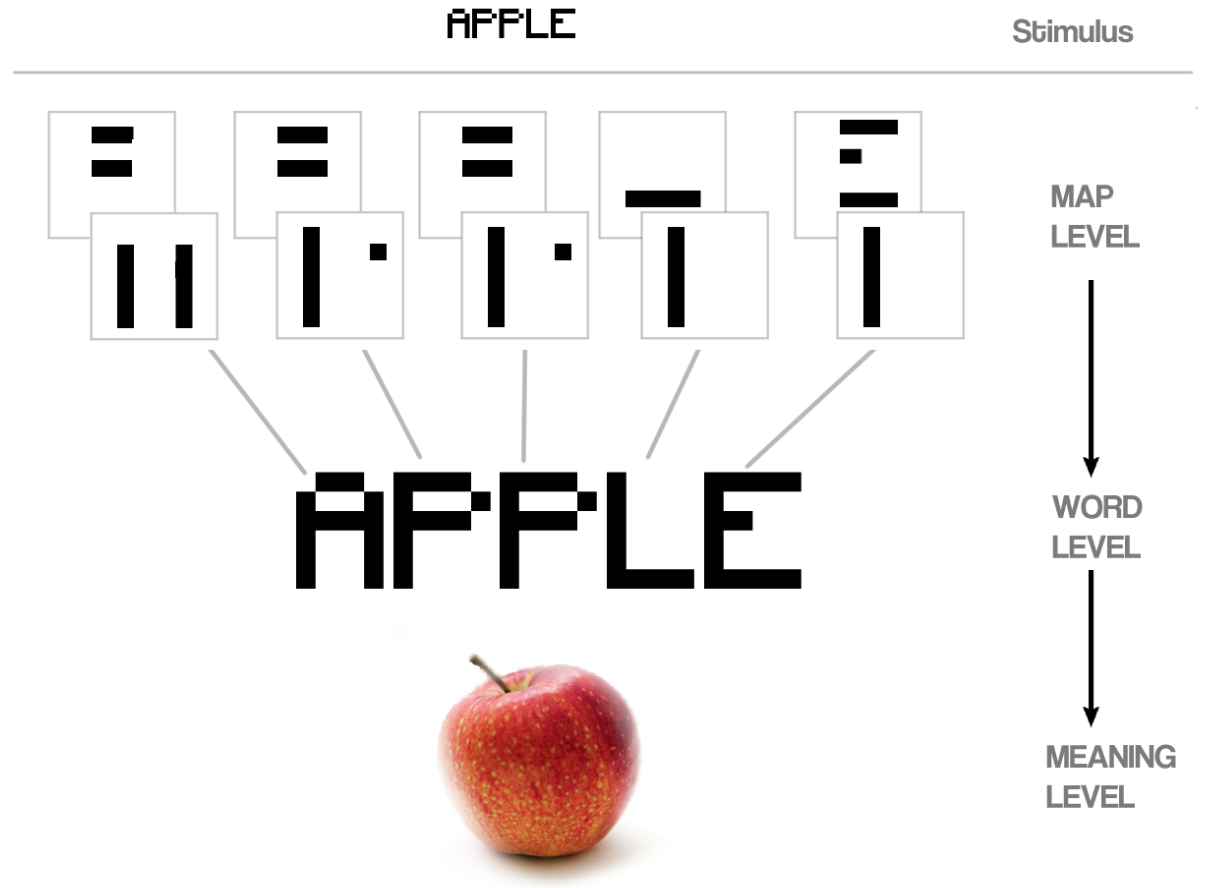
It is unknown at what level hierarchies appear or if they are the only way to encode complexity but neurons seem to code ever more explicit things as you move away from primary sensory areas, the representation of a familiar thing like a face, place or in this case food can be achieved by a few neurons, but as mentioned you need the whole brain and systems along with experience to make sense of them. We share the rough distribution of early sensory systems and bigger cognitive blocks, but the content at some point becomes particular to the individual. (ie we might share a place(s) where we store the detail of faces of our family members, but they all have different content).
*Here objects can be of a different nature than visual stimuli, hearing speech for instance combines phonemes, time features and other lower maps into words we derive meaning from :
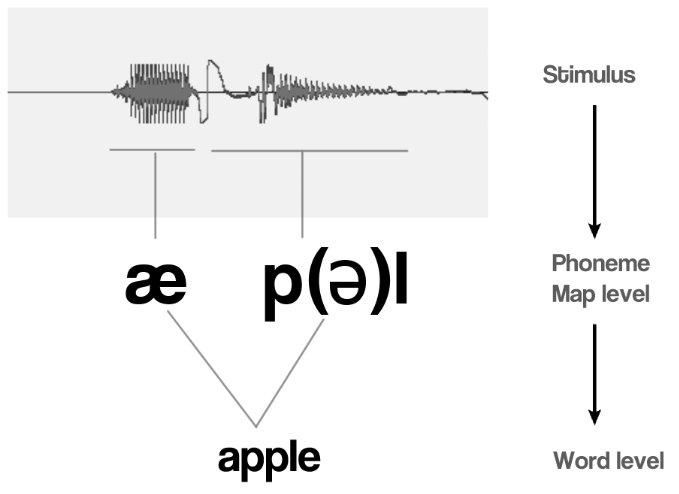
分散式 (Distributed)
It would be terrifying and not very practical to lose the memory of your nana if a few neurons went missing, so it is convenient to have representations distributed across multiple connections and areas of your brain. In how many areas and how exactly are these distributed presentations stored is still not fully understood but basic sensory representations are a good starting point, here a few of them combine to form a rich representation of an apple.
如果缺少一些神经元,丢失您的娜娜记忆会很恐怖,而且不切实际,因此将表示分布在大脑的多个连接和区域中会很方便。 这些分布的演示文稿在多少个区域以及存储的方式方面尚不完全清楚,但是基本的感官表示是一个很好的起点,在这里,其中的一些结合起来就形成了苹果的丰富表示。
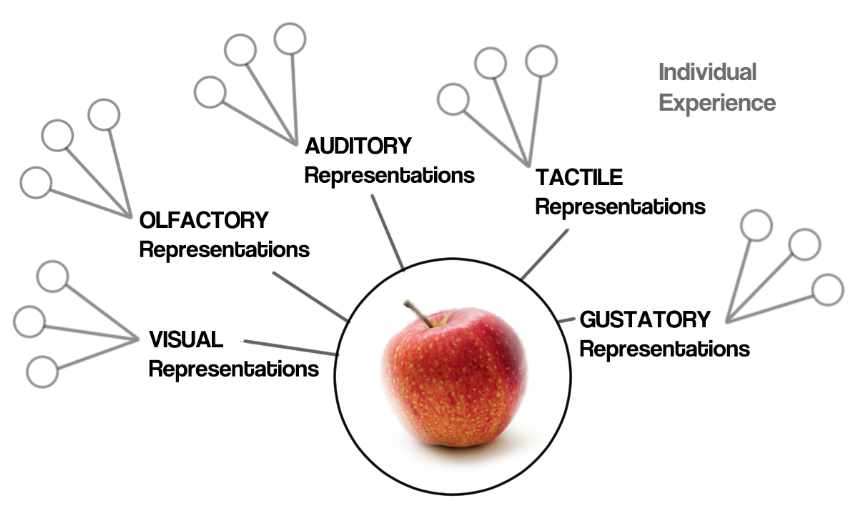
As mentioned the utility of this arrangement seems to be primarily redundancy, a deaf individual can still form a successful apple representation because there’s more to an apple than the light it reflects.
如前所述,这种安排的效用似乎主要是冗余,聋人仍然可以成功地代表苹果,因为苹果所能提供的不仅仅是反射的光线。
The distributed nature does seem to go further than basic sensory modalities, there is rich communication between them ( connections between representations ) as well as more nodes in the form of abstract hierarchical representations ( the idea of an ideal apple ) and even more connections ( Apple pies and Apple computers ).
分布式本质似乎比基本的感觉模态要走得更远,它们之间有丰富的通信(表示之间的连接),以及抽象分层表示形式(理想的苹果的想法)形式的更多节点,甚至还有更多的连接(苹果)派和Apple计算机)。
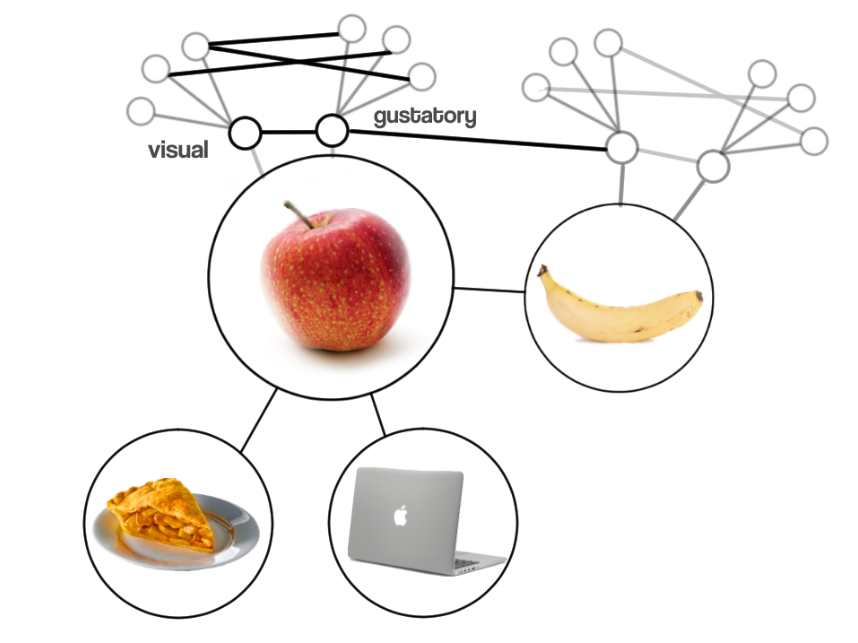
Connections galore! (in black) besides the already mentioned sensory map representations, visual representations can connect to the other modalities like gustatory ones and also to other acquired nodes like fruit, food or related things which in turn have their own connections and relationships. Thousands if not millions of connections tying representations seem to exist in your brain, the detail of how they are formed is the subject of memory acquisition and consolidation.
This nested and distributed effect is easy to experience. If you can’t remember a name, fact or other bit of information ( yet you know you know it ) it is usually helpful to start from what you remember about it, the context might be intact and you can later retrieve the missing piece.
这种嵌套和分布的效果很容易体验。 如果您不记得名称,事实或其他信息(但是您知道知道),通常从记住的内容开始会很有帮助,上下文可能是完整的,以后可以检索丢失的内容。
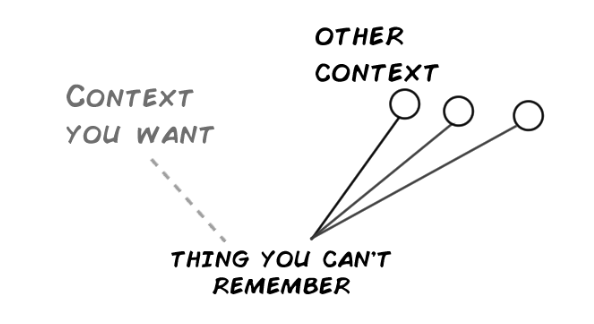
不变的 (Invariant)
We recognize numbers, letters and speech even if they are presented to us as if they had been dragged through the mud: missing pieces, blurred parts, warbled or thick pronunciations, yet remarkably we make sense of them without much effort. To code for this feature we use the sparse and distributed elements along with consolidation over time and new experiences. For instance, do you remember the symbol we just used as an example ? ( if not take another glance )
即使将数字,字母和语音呈现给我们,就像它们被拖到泥泞中一样,我们也能识别它们:丢失的片段,模糊的部分,声音混乱或浓密的发音,但值得注意的是,我们无需付出太多努力就能理解它们。 为了对此功能进行编码,我们使用稀疏和分布式元素以及随着时间的推移和新体验的整合。 例如,您还记得我们刚刚用作示例的符号吗? (如果没有再看一眼)
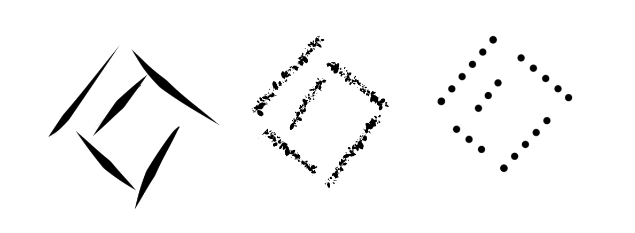
You should have no problem recognizing the same symbol yet you have never seen these specific ones, same goes for hearing the word apple in a different voice, slurred or with a heavy accent.
Another enigma we have yet to fully crack, but current AIs have made a niche in storing and retrieving invariant and useful things like text and speech.
我们还没有完全解开另一个谜团,但是当前的AI在存储和检索诸如文本和语音之类的不变和有用的东西方面已经占据了一席之地。
仿真 (Emulation)
This was just a simple overview of the building blocks of the neural code, left out are many other things. For instance all of this happens through time, be it an instant or a lifespan, it also happens in parallel and the way we perceive through sensory cells ( the computations) represents a new level of complexity, if we were to go down yet another level to the fascinating interactions and behavior of groups of neurons it can be too much, at least for an overview, but what about emulating them ?
这只是对神经代码构建块的简单概述,还有许多其他事情。 例如,所有这些都是随着时间而发生的,无论是瞬间还是生命,它也并行发生,并且如果我们要降到另一个层次,我们通过感觉细胞(计算)所感知的方式就代表了复杂性的新层次。对于神经元组的令人着迷的交互作用和行为而言,它可能太多了,至少在概述方面如此,但是如何模拟它们呢?
The good news is that we are well on our way to emulating these principles as such, in computer science and commercial applications hierarchical, sparse and distributed representations are routinely used for all kinds of tasks. The Invariant task has recently been employed along with Neural Networks and Machine Learning in better object and pattern recognition and more mundane things like search but we’ve yet to achieve the level of detail and complexity human brains posses. There is also the issue of higher cognitive functions which we have barely started understanding and emulating.
好消息是,在计算机科学和商业应用中,我们正在逐步模仿这些原理,在所有类型的任务中通常使用分层和稀疏表示。 Invariant任务最近与神经网络和机器学习一起用于更好的对象和模式识别以及诸如搜索之类的平凡事物,但我们尚未达到人脑所具备的细节和复杂性水平。 还有一个更高的认知功能问题,我们才刚刚开始理解和模仿。
Following these complexities it seems reasonable to understand that raw processing power might not be enough, the complex and mostly unknown code that is happening on biological or artificial hardware is also needed.
遵循这些复杂性,似乎有理由理解原始处理能力可能还不够,还需要生物或人工硬件上发生的复杂且几乎未知的代码。
现在到哪里? (Where to now ?)
For many the end goal is to emulate and better understand human intelligence & cognition and then translate those findings into mostly commercial AIs. Artificial Neural Networks (ANNs) for instance share a background with biological concepts but soon diverged into its own speciality and rich language, newer types of AIs might emerge from new understandings in neural code.
对于许多人来说,最终目标是模仿并更好地理解人类的智力和认知,然后将这些发现转化为大多数商业性AI。 例如,人工神经网络(ANN)具有生物学概念的背景,但不久便进入了自己的专业领域和丰富的语言中,新类型的AI可能来自对神经代码的新理解。
For now we need to work on the fundamentals and to this effect we need to understand how the brain acquires, stores and gives meaning to our world in a systems view; I hope these few paragraphs help you understand some of the building blocks and challenges.
目前,我们需要研究基础知识,为此,我们需要从系统的角度了解大脑如何获取,存储并赋予我们的世界意义; 我希望这几段内容可以帮助您理解一些构建基块和挑战。
Thanks for reading.
谢谢阅读。
进一步阅读: (Further reading :)
I think a good overview of neuroscience is helpful both for inspiration and to better understand more advanced concepts, here’s 2 such places you can start:
我认为,对神经科学的良好概述有助于启发和更好地理解更高级的概念,您可以从以下2个这样的地方开始:
Kandel, Eric R., and Sarah Mack. Principles of Neural Science. McGraw-Hill Medical, 2014.
坎德尔,埃里克·R。和莎拉·麦克。 神经科学原理。 麦格劳·希尔医学杂志,2014年。
Purves, Dale, et al. Neuroscience. Sinauer Associates, 2018.
Purves,Dale等。 神经科学。 Sinauer Associates,2018年。
The systems view of the brain is a work in progress, as such knowledge is dispersed throughout, here are 2 good starting points:
大脑的系统视图是一个正在进行的工作,因为这些知识分散在整个过程中,这里有两个很好的起点:
Rhythms of the Brain ( Buzsaki )
脑节律(Buzsaki)
Networks of the brain, Discovering the Human Connectome ( Sporns )
大脑网络,发现人类连接基因组(Sporns)
Memory is somehow better understood, but critical pieces are still missing:
以某种方式可以更好地理解内存,但是仍然缺少一些关键部分:
Gluck, Mark A., et al. Learning and Memory: from Brain to Behavior. Worth Publishers, 2008.
Gluck,Mark A.等人。 学习与记忆:从大脑到行为。 值得出版社,2008年。
On the other side of the table AI is a fast changing field especially when it comes to Neural Networks, but that’s not the whole story, the field as a whole consists of a myriad algorithms and techniques for intelligent behavior, a good introduction:
另一方面,AI是一个快速变化的领域,尤其是在涉及神经网络时,但这还不是全部,整个领域包括众多智能行为的算法和技术,很好的介绍:
Artificial Intelligence: A Modern Approach. S.n., 2010.
人工智能:一种现代方法。 锡,2010年。
For up to date Machine Learning and ANN tools and techniques the web is probably a better resource these days.
翻译自: https://medium.com/@k3no/sparse-hierarchical-distributed-invariant-representations-ff34b52bea4f