生成对抗网络生成多维数据集
介绍(Introduction)
GAN architecture has been the standard for generating content through AI, but can it actually invent new content outside what’s available in the training dataset? Or it’s just imitating the training data and mixing the features in new ways?
GAN架构一直是通过AI生成内容的标准,但它实际上可以在训练数据集中可用的范围之外发明新内容吗? 还是只是模仿训练数据并以新方式混合功能?
In this article, I will discuss “Rewriting Deep Generative Model” paper that enables editing GAN model directly to give the output that we want even if it doesn’t match the existing dataset. The image above is an example of an edit where you copy the helmet feature and pasted it on the horse contextually. I believe this possibility will open many new interesting applications in the digital industry such as generating fictitious content for animations or games where there may be no existing dataset. You can watch the presentation video at the link below.
在本文中,我将讨论“重写深度生成模型”一文,该文件可直接编辑GAN模型,以提供所需的输出,即使它与现有数据集不匹配也是如此。 上图是编辑示例,您可以在其中复制头盔功能并将其粘贴到上下文中。 我相信这种可能性将打开数字行业中许多新的有趣应用程序,例如为可能不存在现有数据集的动画或游戏生成虚拟内容。 您可以在下面的链接上观看演示视频。
甘 (GAN)
Generative Adversarial Network (GAN) is a generative model which means it can generate a similar realistic output as the training data. For example, a GAN trained on human faces would be able to generate similar looking realistic faces. GAN is able to do this by learning the distribution of the training data and generate new content that follows the same distribution.
生成对抗网络(GAN)是一种生成模型,这意味着它可以生成与训练数据类似的现实输出。 例如,经过人脸训练的GAN将能够生成相似外观的逼真的面Kong。 GAN可以通过学习训练数据的分布并生成遵循相同分布的新内容来做到这一点。
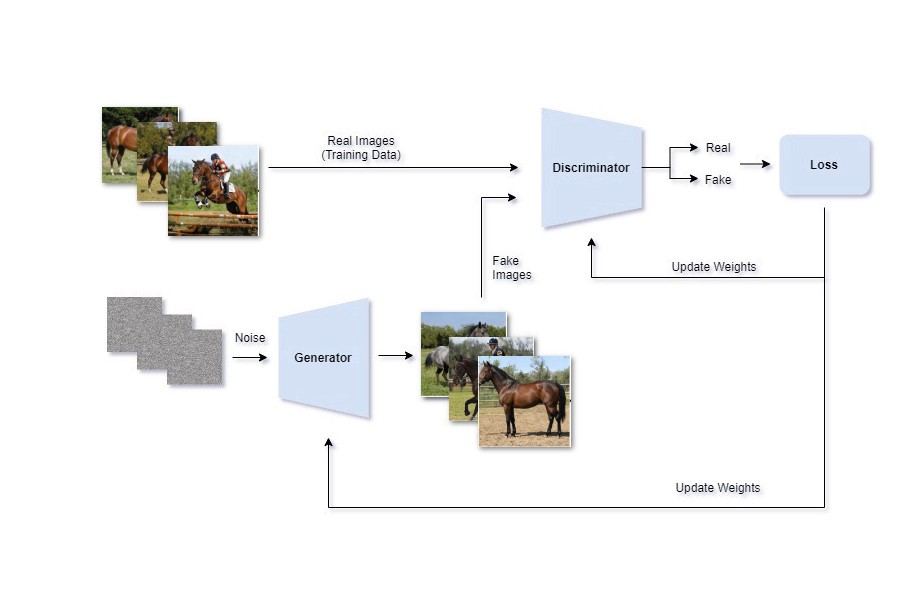
GAN “indirectly” learns the distribution by having a discriminator that tries to differentiate real and fake images and a generator that creates fake data to fool the discriminator. These 2 networks will continuously compete and learn from each other until both of them can generate and discriminate realistic images respectively.
GAN通过拥有一个试图区分真实图像和伪造图像的鉴别器以及一个创建伪造数据来欺骗鉴别器的生成器来“间接”学习分布。 这两个网络将不断竞争和相互学习,直到它们两个都可以分别生成和区分现实图像为止。
GAN的局限性 (GAN Limitations)
Although GAN is able to learn the general data distribution and generate diverse images of the dataset. It is still limited to what exists in the training data. For example, let’s take a GAN model trained on faces. Although it can generate new faces that do not exist in the dataset, it cannot invent an entirely new face with novel features. You can only expect it to combine what the model already know in new ways.
尽管GAN能够学习一般数据分布并生成数据集的各种图像。 它仍然限于训练数据中存在的内容。 例如,让我们采用经过训练的GAN模型。 尽管它可以生成数据集中不存在的新面Kong,但它不能发明具有新颖特征的全新面Kong。 您只能期望它以新的方式结合模型已经知道的内容。
Hence, there is no problem if we only want to generate normal faces. But what if we want faces with bushy eyebrows or maybe a third eye? The GAN model cannot generate this as there is no sample with bushy eyebrows or a third eye in the training data. The quick solution would be simply editing the generated face with a photo-editing tool, but it’s not feasible if we want to generate tons of images like it. Hence, GAN model would suit the problem better, but how do we make GAN generates our desired images when there is no existing dataset?
因此,如果我们只想生成法线脸就没有问题。 但是,如果我们想要眉毛浓密或第三只眼的脸怎么办? GAN模型无法生成此模型,因为在训练数据中没有带有浓密眉毛或第三只眼睛的样本。 快速的解决方案是使用照片编辑工具简单地编辑生成的面部,但是如果我们要生成大量像这样的图像&